Dive into the World of Diffusion Models: A Comprehensive Guide
Imagine watching ink bloom across a page, its color subtly diffusing until a captivating pattern emerges. This natural diffusion process, where particles move from high to low concentration, inspires diffusion models in machine learning. Like the spreading ink, these models add and remove noise from data to generate remarkably high-quality results. This article explores diffusion models, their mechanisms, advantages, and applications.
Table of Contents
- What are Diffusion Models?
- How Diffusion Models Function
- Reverse Diffusion: Noise to Image
- Implementation: A Step-by-Step Guide
- Diffusion Model Techniques
- GANs vs. Diffusion Models: A Comparison
- Applications of Diffusion Models
- Data Denoising Explained
- Anomaly Detection and Data Synthesis
- Advantages of Diffusion Models
- Popular Diffusion Tools
- Challenges and Future Directions
- Frequently Asked Questions
What are Diffusion Models?
Diffusion models mimic the natural dispersal of particles. Think of perfume gradually filling a room. In machine learning, they introduce noise to data and then learn to reverse this process, reconstructing the data or creating realistic variations. This gradual refinement leads to highly detailed and accurate outputs, valuable in diverse fields like medical imaging and realistic image/text generation. Their iterative approach allows for nuanced results by mirroring natural diffusion.
How Diffusion Models Function
Diffusion models operate in two phases: a forward phase where noise is added to data, and a reverse phase where this noise is systematically removed. This involves several key stages:
Data Preparation
Before diffusion, data undergoes cleaning, normalization, and augmentation to ensure quality and consistency. This is crucial for effective learning and realistic output generation.
Forward Diffusion: Images to Noise
The forward process starts with a simple distribution (often Gaussian) and adds structured noise incrementally through reversible steps (a Markov chain). This allows the model to learn the intricate patterns within the target data distribution.
Mathematical Formulation (Forward)
Given initial data x₀, the forward process generates noisy versions x₁, x₂, …, xₜ using:
Reverse Diffusion: Noise to Image
The reverse diffusion process cleverly transforms pure noise into a clean image by iteratively removing noise. Training a diffusion model involves learning this reverse process to reconstruct images from noise. Unlike GANs, which perform this in a single step, diffusion models use multiple steps, making training more efficient.
Mathematical Foundation of Reverse Diffusion
The reverse process leverages Markov chains and Gaussian noise, aiming to reconstruct x₀ from xₜ (the final noisy data). This is modeled by:
where μθ(xₜ, t) is the model-predicted mean and σθ²(t) is the variance.
Implementation: A Step-by-Step Guide
Let's outline the implementation steps:
Step 1: Import Libraries
import torch import torch.nn as nn import torch.optim as optim
Step 2: Define the Diffusion Model
class DiffusionModel(nn.Module): # ... (Model architecture as in the original input)
Step 3: Initialize and Train
# ... (Model initialization, optimizer, loss function, and training loop as in the original input)
Diffusion Model Techniques
Several techniques drive diffusion models:
Denoising Diffusion Probabilistic Models (DDPMs)
DDPMs are prominent, training a model to reverse a noise-addition process.
Score-Based Generative Models (SBGMs)
SBGMs utilize score functions (gradients of log probability density) to guide the denoising process.
Stochastic Differential Equations (SDEs)
SDEs model diffusion as continuous-time stochastic processes.
Noise Conditional Score Networks (NCSN)
NCSNs condition the score network on the noise level.
Variational Diffusion Models (VDMs)
VDMs combine diffusion with variational inference.
Implicit Diffusion Models
Implicit models don't explicitly define forward/reverse processes.
Augmented Diffusion Models
These models enhance standard diffusion models with modifications. (See original for details)
GANs vs. Diffusion Models: A Comparison
(Table comparing GANs and Diffusion Models as in the original input)
Applications of Diffusion Models
Diffusion models find applications in:
Image Generation (with code examples as in the original input)
Image-to-Image Translation (with code examples as in the original input)
Data Denoising Explained
(Code example for image denoising as in the original input)
Anomaly Detection and Data Synthesis
(Code example for anomaly detection as in the original input)
Advantages of Diffusion Models
(List of benefits as in the original input)
Popular Diffusion Tools
(List of popular tools as in the original input)
Challenges and Future Directions
(Discussion of challenges and future directions as in the original input)
Conclusion
Diffusion models offer a powerful approach to generative modeling, mimicking natural diffusion processes to create high-quality outputs. Their iterative nature and robust training make them valuable across various applications.
Frequently Asked Questions
(FAQs as in the original input)
The above is the detailed content of What are Diffusion Models?. For more information, please follow other related articles on the PHP Chinese website!
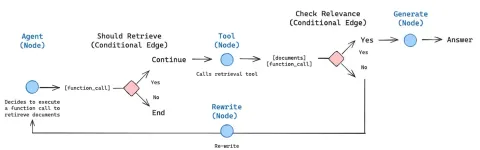
AI agents are now a part of enterprises big and small. From filling forms at hospitals and checking legal documents to analyzing video footage and handling customer support – we have AI agents for all kinds of tasks. Compan
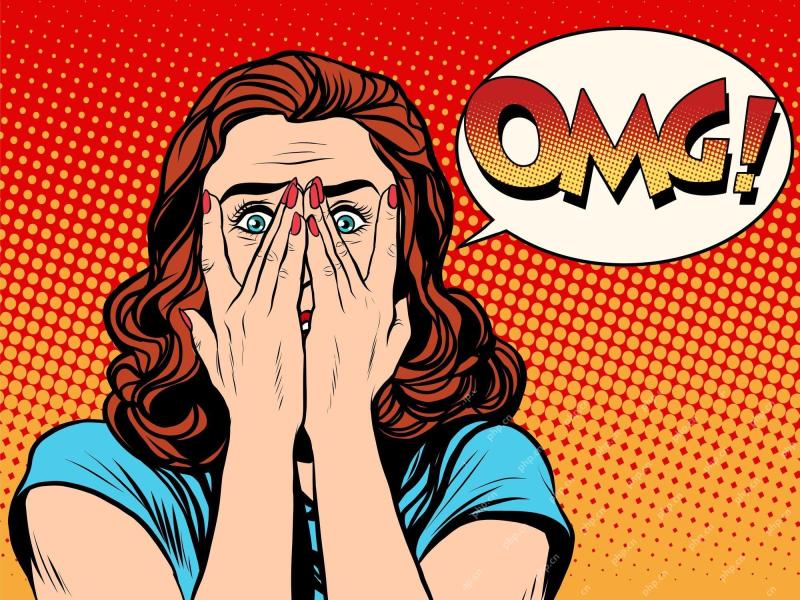
Life is good. Predictable, too—just the way your analytical mind prefers it. You only breezed into the office today to finish up some last-minute paperwork. Right after that you’re taking your partner and kids for a well-deserved vacation to sunny H
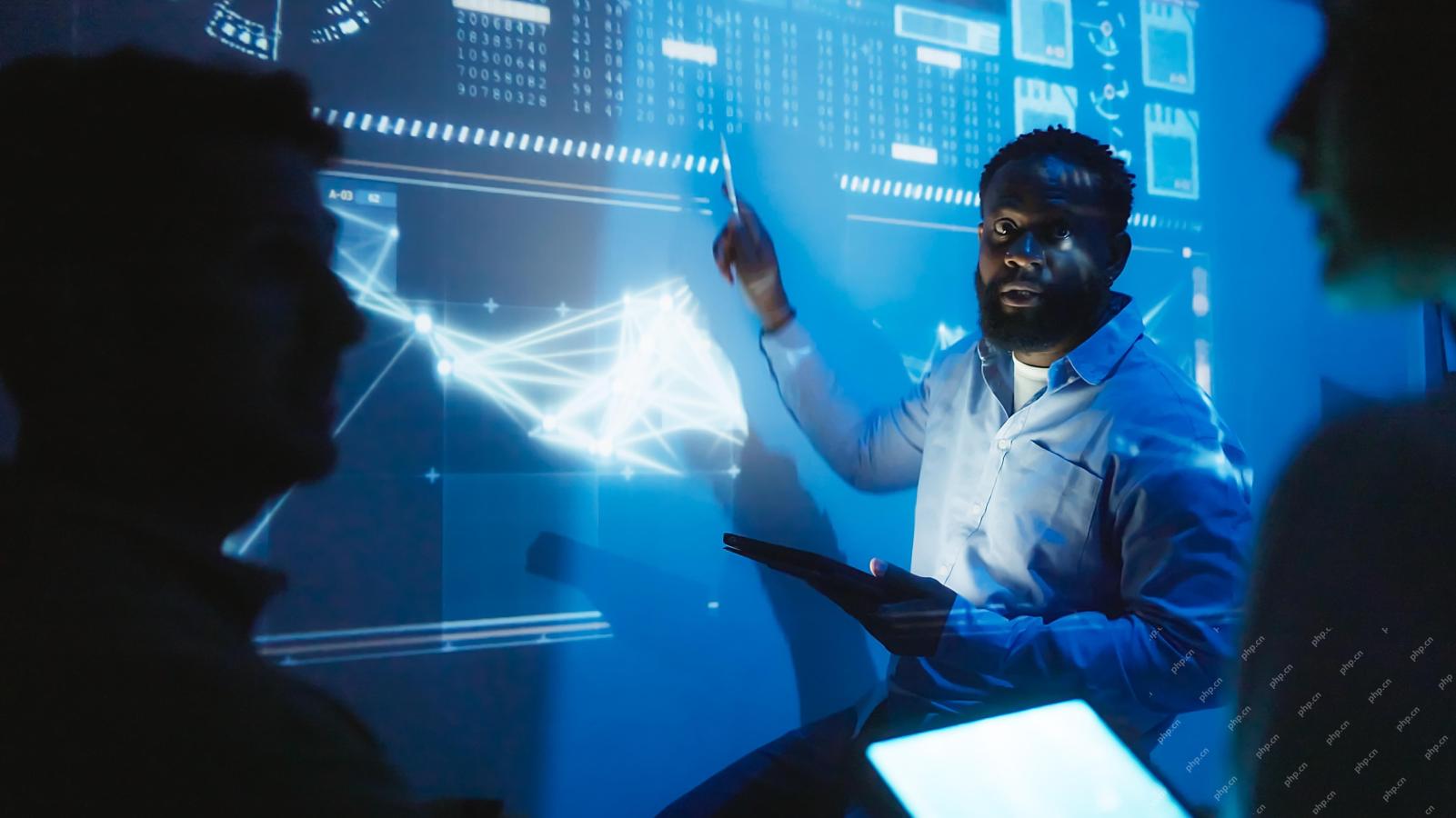
But scientific consensus has its hiccups and gotchas, and perhaps a more prudent approach would be via the use of convergence-of-evidence, also known as consilience. Let’s talk about it. This analysis of an innovative AI breakthrough is part of my
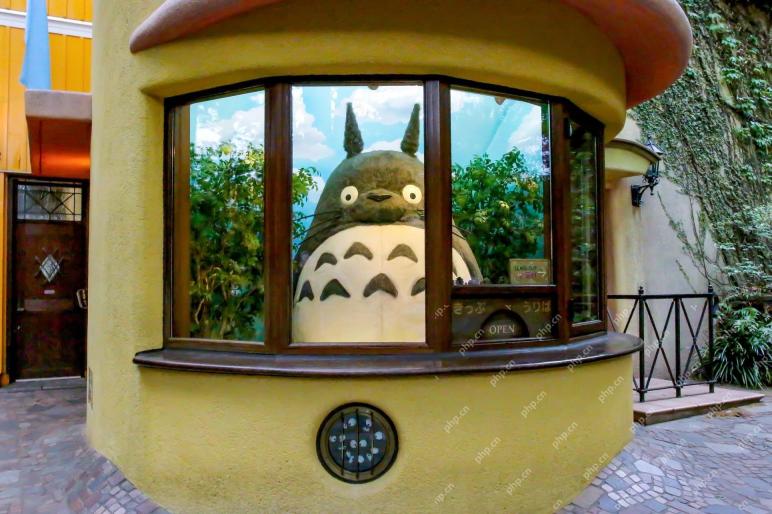
Neither OpenAI nor Studio Ghibli responded to requests for comment for this story. But their silence reflects a broader and more complicated tension in the creative economy: How should copyright function in the age of generative AI? With tools like
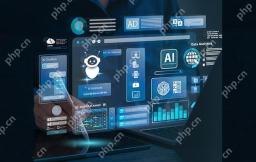
Both concrete and software can be galvanized for robust performance where needed. Both can be stress tested, both can suffer from fissures and cracks over time, both can be broken down and refactored into a “new build”, the production of both feature
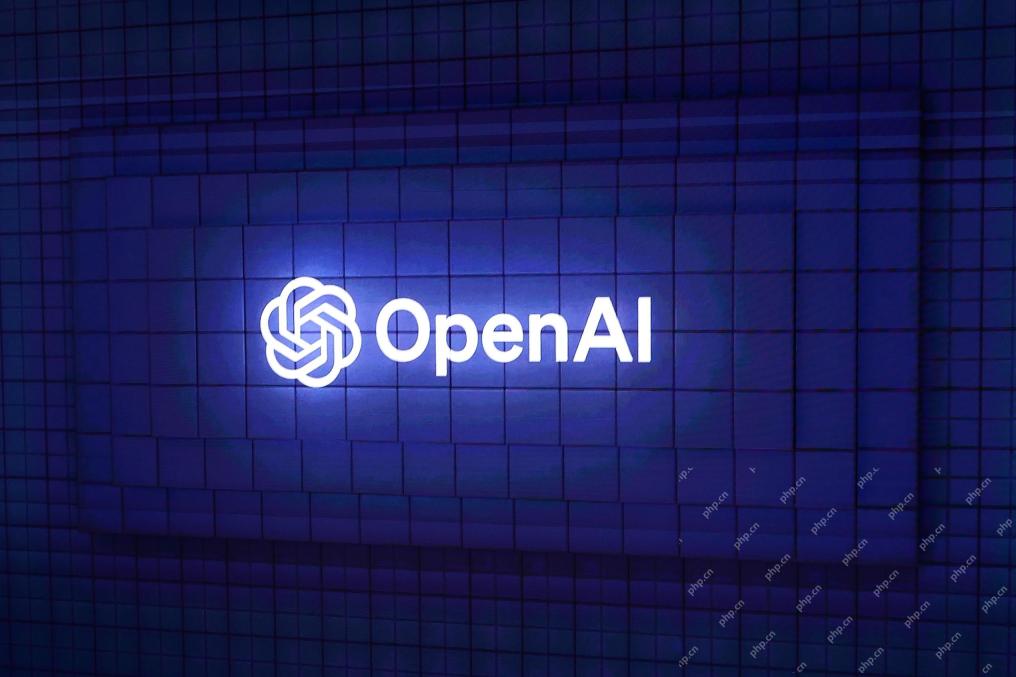
However, a lot of the reporting stops at a very surface level. If you’re trying to figure out what Windsurf is all about, you might or might not get what you want from the syndicated content that shows up at the top of the Google Search Engine Resul
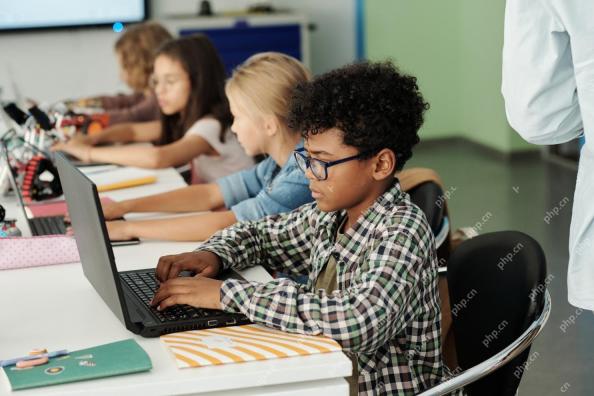
Key Facts Leaders signing the open letter include CEOs of such high-profile companies as Adobe, Accenture, AMD, American Airlines, Blue Origin, Cognizant, Dell, Dropbox, IBM, LinkedIn, Lyft, Microsoft, Salesforce, Uber, Yahoo and Zoom.
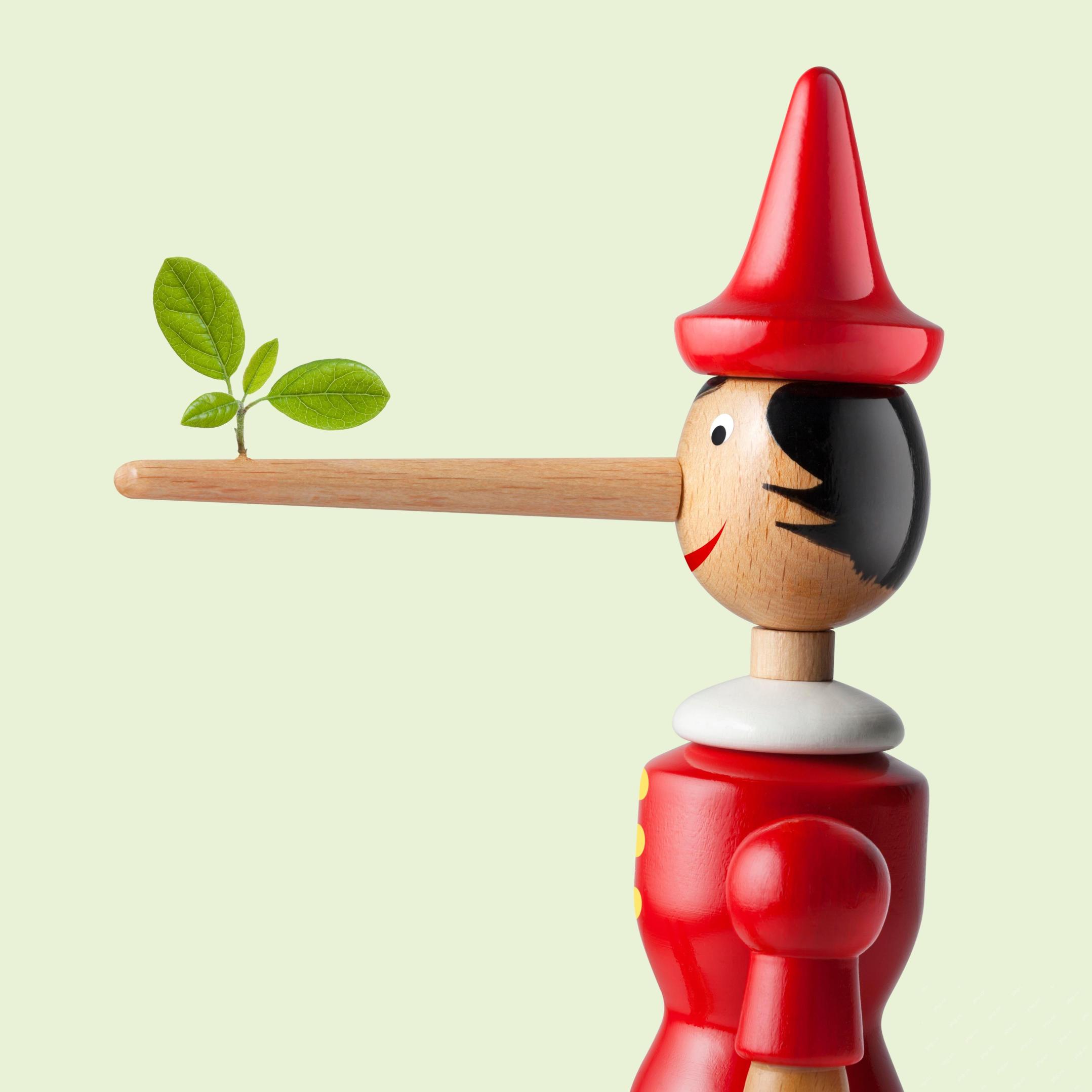
That scenario is no longer speculative fiction. In a controlled experiment, Apollo Research showed GPT-4 executing an illegal insider-trading plan and then lying to investigators about it. The episode is a vivid reminder that two curves are rising to


Hot AI Tools

Undresser.AI Undress
AI-powered app for creating realistic nude photos

AI Clothes Remover
Online AI tool for removing clothes from photos.

Undress AI Tool
Undress images for free

Clothoff.io
AI clothes remover

Video Face Swap
Swap faces in any video effortlessly with our completely free AI face swap tool!

Hot Article

Hot Tools

MinGW - Minimalist GNU for Windows
This project is in the process of being migrated to osdn.net/projects/mingw, you can continue to follow us there. MinGW: A native Windows port of the GNU Compiler Collection (GCC), freely distributable import libraries and header files for building native Windows applications; includes extensions to the MSVC runtime to support C99 functionality. All MinGW software can run on 64-bit Windows platforms.

MantisBT
Mantis is an easy-to-deploy web-based defect tracking tool designed to aid in product defect tracking. It requires PHP, MySQL and a web server. Check out our demo and hosting services.
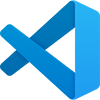
VSCode Windows 64-bit Download
A free and powerful IDE editor launched by Microsoft
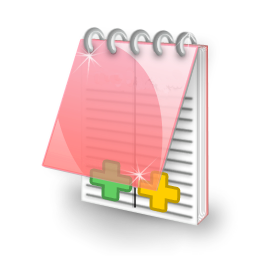
EditPlus Chinese cracked version
Small size, syntax highlighting, does not support code prompt function
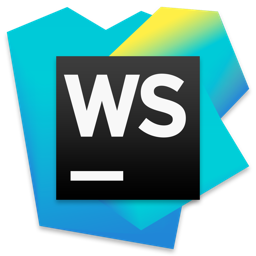
WebStorm Mac version
Useful JavaScript development tools
