Introduction
Large Language Models (LLMs) contributed to the progress of Natural Language Processing (NLP), but they also raised some important questions about computational efficiency. These models have become too large, so the training and inference cost is no longer within reasonable limits.
To address this, the Chinchilla Scaling Law, introduced by Hoffmann et al. in 2022, provides a groundbreaking framework for optimizing the training of LLMs. The Chinchilla Scaling Law offers an essential guide to efficiently scaling LLMs without compromising performance by establishing relationships between model size, training data, and computational resources. We will discuss it in detail in this article.
Overview
- The Chinchilla Scaling Law optimizes LLM training by balancing model size and data volume for enhanced efficiency.
- New scaling insights suggest that smaller language models like Chinchilla can outperform larger ones when trained on more data.
- Chinchilla’s approach challenges traditional LLM scaling by prioritizing data quantity over model size for compute efficiency.
- The Chinchilla Scaling Law offers a new roadmap for NLP, guiding the development of high-performing, resource-efficient models.
- The Chinchilla Scaling Law maximizes language model performance with minimal compute costs by doubling the model size and training data.
Table of contents
- What is Chinchilla Scaling Law?
- A Shift in Focus: From Model Size to Data
- Overview of the Chinchilla Scaling Law
- Key Findings of the Chinchilla Scaling Law
- Compute-Optimal Training
- Empirical Evidence from Over 400 Models
- Revised Estimates and Continuous Improvement
- Benefits of the Chinchilla Approach
- Improved Performance
- Lower Computational Costs
- Implications for Future Research and Model Development
- Challenges and Considerations
- Frequently Asked Questions
What is Chinchilla Scaling Law?
The paper “Training Compute-Optimal Large Language Models,” published in 2022, focuses on identifying the relationship between three key factors: model size, number of tokens, and compute budget. The authors found that existing large language models (LLMs) like GPT-3 (175B parameters), Gopher (280B), and Megatron (530B) are significantly undertrained. While these models increased in size, the amount of training data remained largely constant, leading to suboptimal performance. The authors propose that model size and the number of training tokens must be scaled equally for compute-optimal training. To prove this, they trained around 400 models, ranging from 70 million to over 16 billion parameters, using between 5 and 500 billion tokens.
Based on these findings, the authors trained a new model called Chinchilla, which uses the same compute budget as Gopher (280B) but with only 70B parameters and four times more training data. Chinchilla outperformed several well-known LLMs, including Gopher (280B), GPT-3 (175B), Jurassic-1 (178B), and Megatron (530B). This result contradicts the scaling laws proposed by OpenAI in “Scaling Laws for LLMs,” which suggested that larger models would always perform better. The Chinchilla Scaling Laws demonstrate that smaller models when trained on more data, can achieve superior performance. This approach also makes smaller models easier to fine-tune and reduces inference latency.
The graph shows that, despite being smaller, Chinchilla (70B) follows a different compute-to-parameter ratio and outperforms larger models like Gopher and GPT-3.
The other approaches (1, 2, and 3) explore different ways to optimize model performance based on compute allocation.
From this figure we can see Chinchilla’s Advantage even though Chinchilla is smaller in size (70B parameters), it was trained on a much larger dataset (1.4 trillion tokens), which follows the principle introduced in the Chinchilla Scaling Laws—smaller models can outperform larger ones if they are trained on more data.Other models like Gopher, GPT-3, and MT-NLG 530B have significantly more parameters but were trained on relatively fewer tokens, suggesting that these models may not have fully optimized their compute potential.
A Shift in Focus: From Model Size to Data
Historically, the focus in enhancing LLM performance has been on increasing model size, as seen in models like GPT-3 and Gopher. This was driven by the research of Kaplan et al. (2020), which proposed a power-law relationship between model size and performance. However, as models grew larger, the amount of training data did not scale accordingly, resulting in underutilized compute potential. The Chinchilla Scaling Laws challenge this by showing that a more balanced allocation of resources, particularly in terms of data and model size, can lead to compute-optimal models that perform better without reaching their lowest possible loss.
Overview of the Chinchilla Scaling Law
The trade-off between model size, training tokens, and computational cost is at the core of the Chinchilla Scaling Law. The law establishes a compute-optimal balance between these three parameters:
- Model Size (N):The number of parameters in the model.
- Training Tokens (D):The total number of tokens used during training.
- Computational Cost (C): The total compute resources allocated for training, usually measured in FLOPs (floating point operations per second).
The Chinchilla Scaling Law suggests that for optimal performance, both model size and the amount of training data should scale at equal rates. Specifically, the number of training tokens should also double for every doubling of model size. This approach contrasts earlier methods, which emphasized increasing model size without sufficiently increasing the training data.
This relationship is mathematically expressed as:
Where:
- L is the model’s final loss.
- L_0 is the irreducible loss, representing the best possible performance.
- A and B are constants that capture the model’s underperformance compared to an ideal generative process.
- α and β are exponents that describe how loss scales with respect to model size and data size, respectively.
Key Findings of the Chinchilla Scaling Law
Here are the key findings of the Chinchilla scaling law:
Compute-Optimal Training
The Chinchilla Scaling Law highlights an optimal balance between model size and the amount of training data. Specifically, the study found that an approximate ratio of 20 training tokens per model parameter is ideal for achieving the best performance with a given compute budget. For example, the Chinchilla model, with 70 billion parameters, was trained on 1.4 trillion tokens—four times more than Gopher but with far fewer parameters. This balance resulted in a model significantly outperforming larger models on several benchmarks.
Empirical Evidence from Over 400 Models
To derive the Chinchilla Scaling Laws, Hoffmann et al. trained over 400 transformer models, ranging in size from 70 million to 16 billion parameters, on datasets of up to 500 billion tokens. The empirical evidence strongly supported the hypothesis that models trained with more data (at a fixed compute budget) perform better than simply increasing model size alone.
Revised Estimates and Continuous Improvement
Subsequent research has sought to refine Hoffmann et al.’s initial findings, identifying possible adjustments in the parameter estimates. Some studies have suggested minor inconsistencies in the original results and have proposed revised estimates to fit the observed data better. These adjustments indicate that further research is needed to understand the dynamics of model scaling fully, but the core insights of the Chinchilla Scaling Law remain a valuable guideline.
Benefits of the Chinchilla Approach
Here are the benefits of the Chinchilla approach:
Improved Performance
Chinchilla’s equal scaling of model size and training data yielded remarkable results. Despite being smaller than many other large models, Chinchilla outperformed GPT-3, Gopher, and even the massive Megatron-Turing NLG model (530 billion parameters) on various benchmarks. For instance, on the Massive Multitask Language Understanding (MMLU) benchmark, Chinchilla achieved an average accuracy of 67.5%, a significant improvement over Gopher’s 60%.
Lower Computational Costs
The Chinchilla approach optimizes performance and reduces computational and energy costs for training and inference. Training models like GPT-3 and Gopher require enormous computing resources, making their use in real-world applications prohibitively expensive. In contrast, Chinchilla’s smaller model size and more extensive training data result in lower compute requirements for fine-tuning and inference, making it more accessible for downstream applications.
Implications for Future Research and Model Development
The Chinchilla Scaling Laws offer valuable insights for the future of LLM development. Key implications include:
- Guiding Model Design:Understanding how to balance model size and training data allows researchers and developers to make more informed decisions when designing new models. By adhering to the principles outlined in the Chinchilla Scaling Law, developers can ensure that their models are both compute-efficient and high-performing.
- Guiding Model Design:Knowledge on optimizing the volume and so the training data informs the models’ research and design. Within this guideline scale, the development of their ideas will operate within broad definitions of high efficiency without excessive consumption of computer resources.
- Performance Optimization:The Chinchilla Scaling Law provides a roadmap for optimizing LLMs. By focusing on equal scaling, developers can avoid the pitfalls of under-training large models and ensure that models are optimized for training and inference tasks.
- Exploration Beyond Chinchilla:As research continues, new strategies are emerging to extend the ideas of the Chinchilla Scaling Law. For example, some researchers are investigating ways to achieve similar performance levels with fewer computational resources or to further enhance model performance in data-constrained environments. These explorations are likely to result in even more efficient training pipelines.
Challenges and Considerations
While the Chinchilla Scaling Law marks a significant step forward in understanding LLM scaling, it also raises new questions and challenges:
- Data Collection: As was the case for Chinchilla, training a model with 1.4 trillion tokens implies the availability of many high-quality datasets. However, such a scale of data collection and processing raises organizational problems for researchers and developers, as well as ethical problems, such as privacy and bias.
- Bias and Toxicity:However, proportional reduction of regular bias and toxicity of a model trained using the Chinchilla Scaling law is easier and more efficient than all these inefficiency issues. As LLMs grow in power and reach, ensuring fairness and mitigating harmful outputs will be crucial focus areas for future research.
Conclusion
The Chinchilla Scaling Law represents a pivotal advancement in our understanding of optimizing the training of large language models. By establishing clear relationships between model size, training data, and computational cost, the law provides a compute-optimal framework for efficiently scaling LLMs. The success of the Chinchilla model demonstrates the practical benefits of this approach, both in terms of performance and resource efficiency.
As research in this area continues, the principles of the Chinchilla Scaling Law will likely shape the future of LLM development, guiding the design of models that push the boundaries of what’s possible in natural language processing while maintaining sustainability and accessibility.
Also, if you are looking for a Generative AI course online, then explore: the GenAI Pinnacle Program!
Frequently Asked Questions
Q1. What is the Chinchilla scaling law?Ans. The Chinchilla scaling law is an empirical framework that describes the optimal relationship between the size of a language model (number of parameters), the amount of training data (tokens), and the computational resources required for training. It aims to minimize training compute while maximizing model performance.
Q2. What are the key parameters in the Chinchilla scaling law?Ans. The key parameters include:
1. N: Number of parameters in the model.
2. D: Number of training tokens.
3. C: Total computational cost in FLOPS.
4. L: Average loss achieved by the model on a test dataset.
5. A and B: Constants reflecting underperformance compared to an ideal generative process.
6. α and β: Exponents describing how loss scales concerning model and data size, respectively.
Ans. The law suggests that both model size and training tokens should scale at equal rates for optimal performance. Specifically, for every doubling of model size, the number of training tokens should also double, typically aiming for a ratio of around 20 tokens per parameter.
Q4. What are some criticisms or limitations of the Chinchilla scaling law?Ans. Recent studies have indicated potential issues with Hoffmann et al.’s original estimates, including inconsistencies in reported data and overly tight confidence intervals. Some researchers argue that the scaling law may be too simplistic and does not account for various practical considerations in model training.
Q5. How has the Chinchilla scaling law influenced recent language model development?Ans. The findings from the Chinchilla scaling law have informed several notable models’ design and training processes, including Google’s Gemini suite. It has also prompted discussions about “beyond Chinchilla” strategies, where researchers explore training models larger than optimal according to the original scaling laws.
The above is the detailed content of What is the Chinchilla Scaling Law?. For more information, please follow other related articles on the PHP Chinese website!
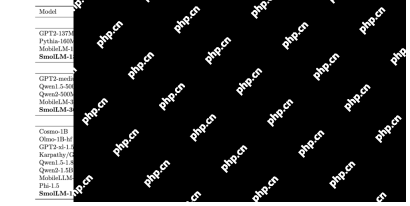
Harness the Power of On-Device AI: Building a Personal Chatbot CLI In the recent past, the concept of a personal AI assistant seemed like science fiction. Imagine Alex, a tech enthusiast, dreaming of a smart, local AI companion—one that doesn't rely
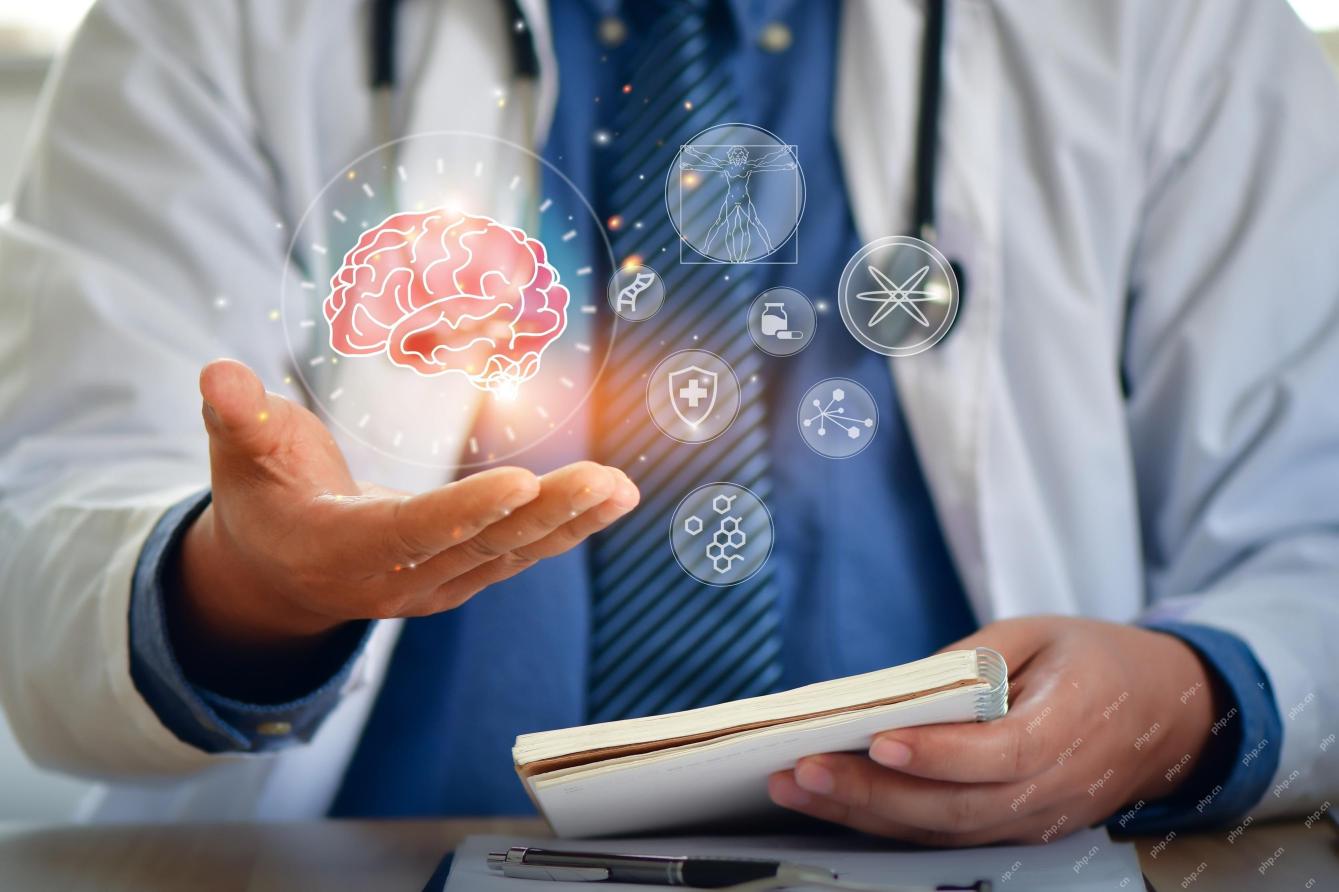
Their inaugural launch of AI4MH took place on April 15, 2025, and luminary Dr. Tom Insel, M.D., famed psychiatrist and neuroscientist, served as the kick-off speaker. Dr. Insel is renowned for his outstanding work in mental health research and techno
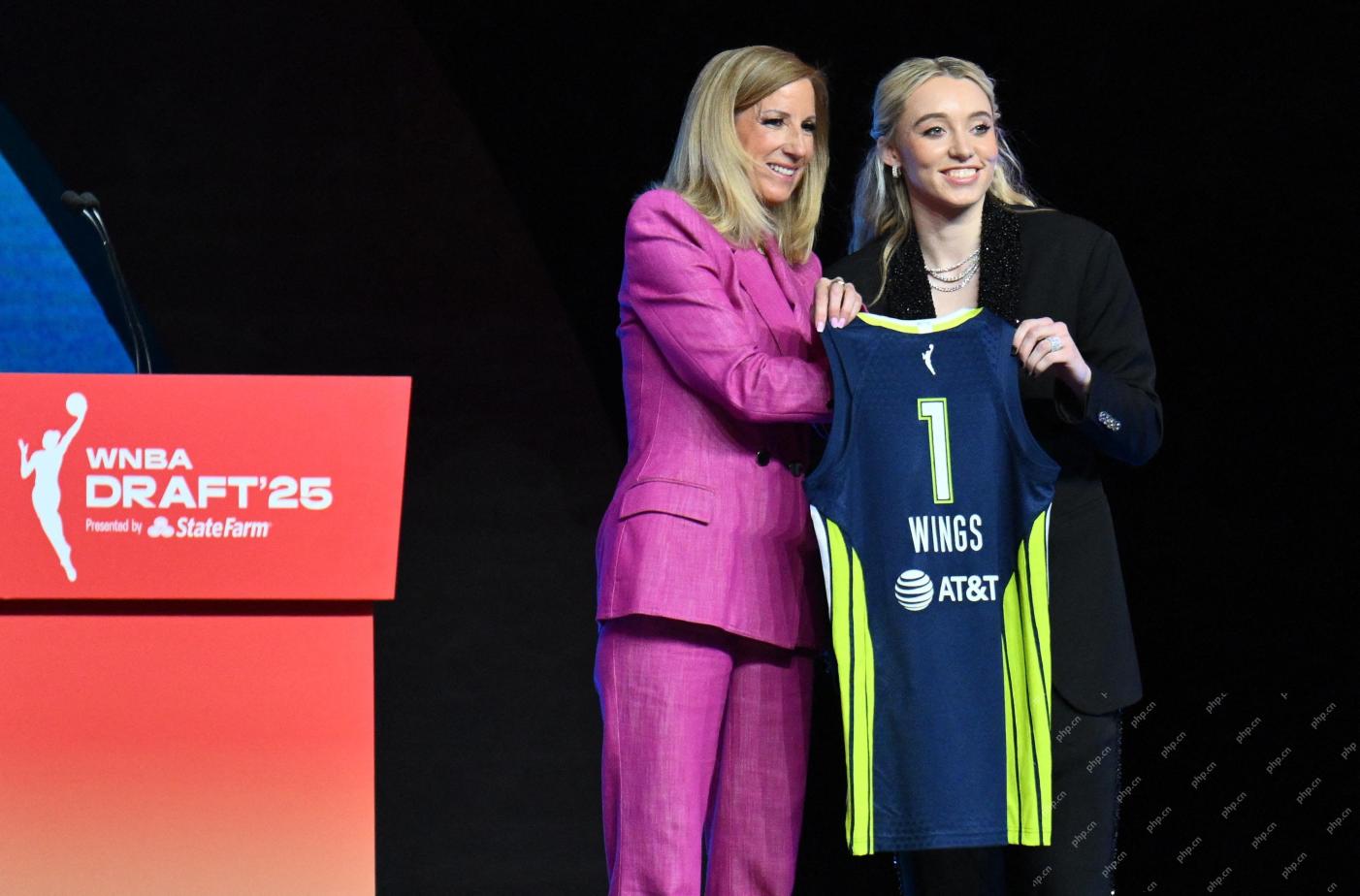
"We want to ensure that the WNBA remains a space where everyone, players, fans and corporate partners, feel safe, valued and empowered," Engelbert stated, addressing what has become one of women's sports' most damaging challenges. The anno
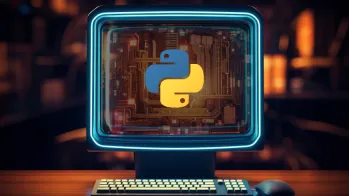
Introduction Python excels as a programming language, particularly in data science and generative AI. Efficient data manipulation (storage, management, and access) is crucial when dealing with large datasets. We've previously covered numbers and st
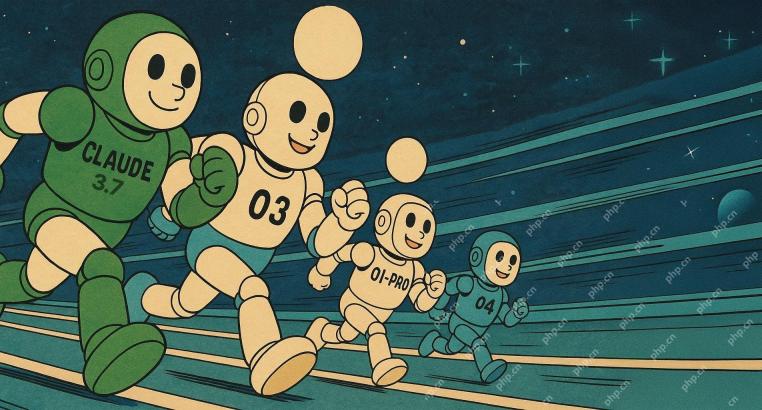
Before diving in, an important caveat: AI performance is non-deterministic and highly use-case specific. In simpler terms, Your Mileage May Vary. Don't take this (or any other) article as the final word—instead, test these models on your own scenario
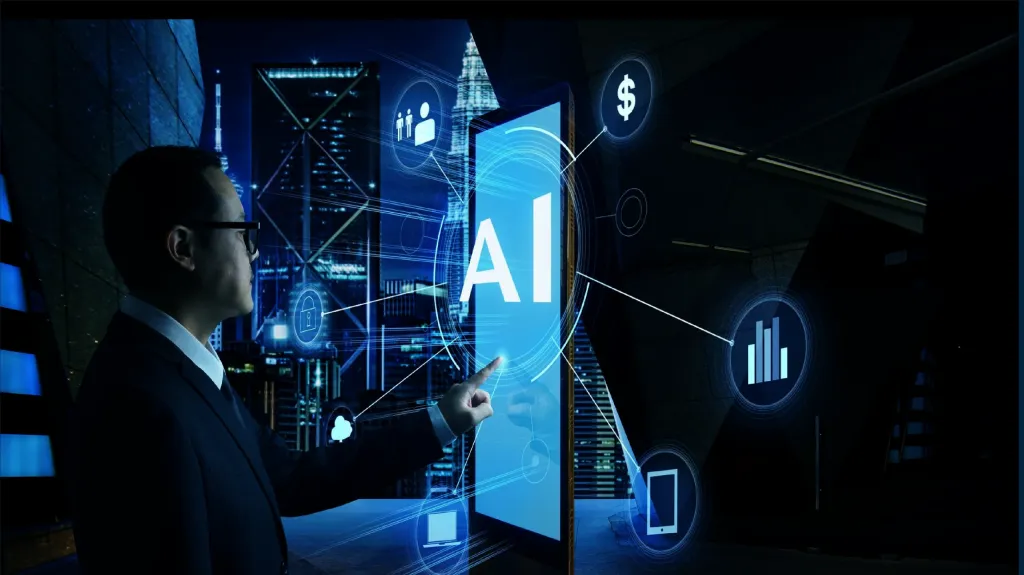
Building a Standout AI/ML Portfolio: A Guide for Beginners and Professionals Creating a compelling portfolio is crucial for securing roles in artificial intelligence (AI) and machine learning (ML). This guide provides advice for building a portfolio
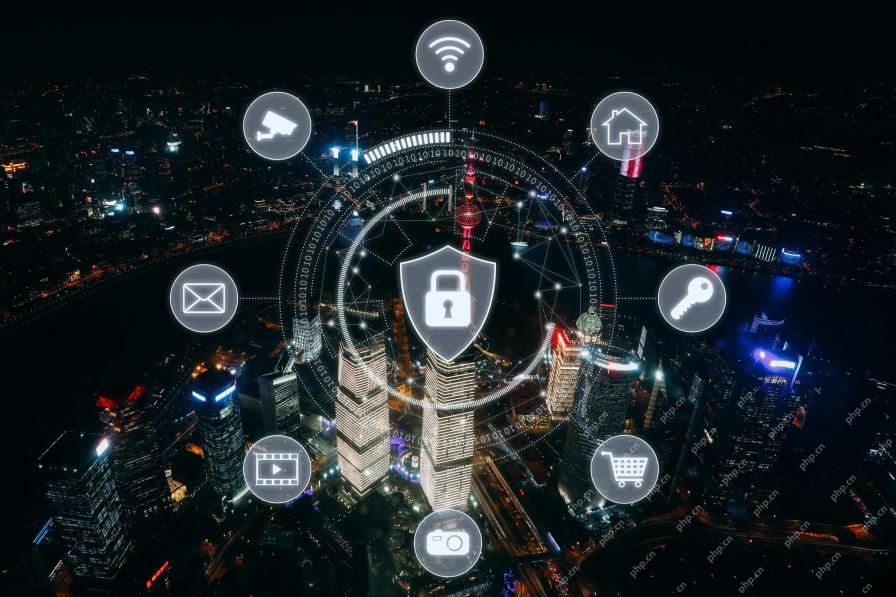
The result? Burnout, inefficiency, and a widening gap between detection and action. None of this should come as a shock to anyone who works in cybersecurity. The promise of agentic AI has emerged as a potential turning point, though. This new class
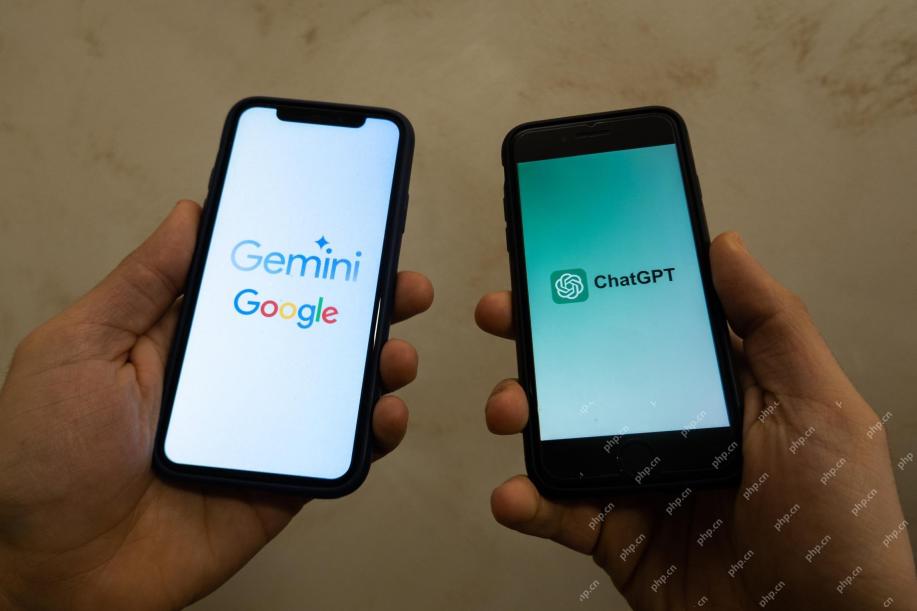
Immediate Impact versus Long-Term Partnership? Two weeks ago OpenAI stepped forward with a powerful short-term offer, granting U.S. and Canadian college students free access to ChatGPT Plus through the end of May 2025. This tool includes GPT‑4o, an a


Hot AI Tools

Undresser.AI Undress
AI-powered app for creating realistic nude photos

AI Clothes Remover
Online AI tool for removing clothes from photos.

Undress AI Tool
Undress images for free

Clothoff.io
AI clothes remover

AI Hentai Generator
Generate AI Hentai for free.

Hot Article

Hot Tools
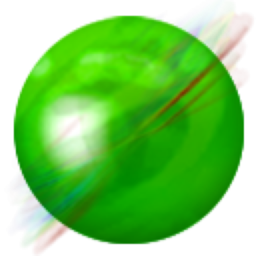
ZendStudio 13.5.1 Mac
Powerful PHP integrated development environment

Notepad++7.3.1
Easy-to-use and free code editor

mPDF
mPDF is a PHP library that can generate PDF files from UTF-8 encoded HTML. The original author, Ian Back, wrote mPDF to output PDF files "on the fly" from his website and handle different languages. It is slower than original scripts like HTML2FPDF and produces larger files when using Unicode fonts, but supports CSS styles etc. and has a lot of enhancements. Supports almost all languages, including RTL (Arabic and Hebrew) and CJK (Chinese, Japanese and Korean). Supports nested block-level elements (such as P, DIV),
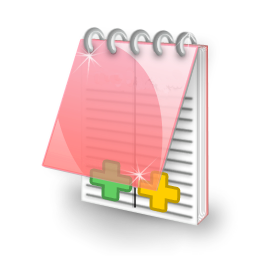
EditPlus Chinese cracked version
Small size, syntax highlighting, does not support code prompt function
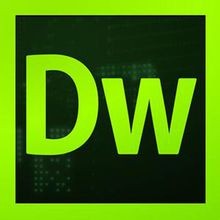
Dreamweaver CS6
Visual web development tools