Introduction
Vector streaming in EmbedAnything is being introduced, a feature designed to optimize large-scale document embedding. Enabling asynchronous chunking and embedding using Rust’s concurrency reduces memory usage and speeds up the process. Today, I will show how to integrate it with the Weaviate Vector Database for seamless image embedding and search.
In my previous article, Supercharge Your Embeddings Pipeline with EmbedAnything, I discussed the idea behind EmbedAnything and how it makes creating embeddings from multiple modalities easy. In this article, I want to introduce a new feature of EmbedAnything called vector streaming and see how it works with Weaviate Vector Database.
Overview
- Vector streaming in EmbedAnything optimizes embedding large-scale documents using asynchronous chunking with Rust’s concurrency.
- It solves memory and efficiency issues in traditional embedding methods by processing chunks in parallel.
- Integration with Weaviate enables seamless embedding and searching in a vector database.
- Implementing vector streaming involves creating a database adapter, initiating an embedding model, and embedding data.
- This approach offers a more efficient, scalable, and flexible solution for large-scale document embedding.
Table of contents
- What is the problem?
- Our Solution to the Problem
- Example Use Case with EmbedAnything
- Step 1: Create the Adapter
- Step 2: Create the Embedding Model
- Step 3: Embed the Directory
- Step 4: Query the Vector Database
- Step 5: Query the Vector Database
- Output
- Frequently Asked Questions
What is the problem?
First, examine the current problem with creating embeddings, especially in large-scale documents. The current embedding frameworks operate on a two-step process: chunking and embedding. First, the text is extracted from all the files, and chunks/nodes are created. Then, these chunks are fed to an embedding model with a specific batch size to process the embeddings. While this is done, the chunks and the embeddings stay on the system memory.
This is not a problem when the files and embedding dimensions are small. But this becomes a problem when there are many files, and you are working with large models and, even worse, multi-vector embeddings. Thus, to work with this, a high RAM is required to process the embeddings. Also, if this is done synchronously, a lot of time is wasted while the chunks are being created, as chunking is not a compute-heavy operation. As the chunks are being made, passing them to the embedding model would be efficient.
Our Solution to the Problem
The solution is to create an asynchronous chunking and embedding task. We can effectively spawn threads to handle this task using Rust’s concurrency patterns and thread safety. This is done using Rust’s MPSC (Multi-producer Single Consumer) module, which passes messages between threads. Thus, this creates a stream of chunks passed into the embedding thread with a buffer. Once the buffer is complete, it embeds the chunks and sends the embeddings back to the main thread, which then sends them to the vector database. This ensures no time is wasted on a single operation and no bottlenecks. Moreover, the system stores only the chunks and embeddings in the buffer, erasing them from memory once they are moved to the vector database.
Example Use Case with EmbedAnything
Now, let’s see this feature in action:
With EmbedAnything, streaming the vectors from a directory of files to the vector database is a simple three-step process.
- Create an adapter for your vector database: This is a wrapper around the database’s functions that allows you to create an index, convert metadata from EmbedAnything’s format to the format required by the database, and the function to insert the embeddings in the index. Adapters for the prominent databases have already been created and are present here.
- Initiate an embedding model of your choice: You can choose from different local models or even cloud models. The configuration can also be determined by setting the chunk size and buffer size for how many embeddings need to be streamed at once. Ideally, this should be as high as possible, but the system RAM limits this.
- Call the embedding function from EmbedAnything: Just pass the directory path to be embedded, the embedding model, the adapter, and the configuration.
In this example, we will embed a directory of images and send it to the vector databases.
Step 1: Create the Adapter
In EmbedAnything, the adapters are created outside so as not to make the library heavy and you get to choose which database you want to work with. Here is a simple adapter for Weaviate:
from embed_anything import EmbedData from embed_anything.vectordb import Adapter class WeaviateAdapter(Adapter): def __init__(self, api_key, url): super().__init__(api_key) self.client = weaviate.connect_to_weaviate_cloud( cluster_url=url, auth_credentials=wvc.init.Auth.api_key(api_key) ) if self.client.is_ready(): print("Weaviate is ready") def create_index(self, index_name: str): self.index_name = index_name self.collection = self.client.collections.create( index_name, vectorizer_config=wvc.config.Configure.Vectorizer.none() ) return self.collection def convert(self, embeddings: List[EmbedData]): data = [] for embedding in embeddings: property = embedding.metadata property["text"] = embedding.text data.append( wvc.data.DataObject(properties=property, vector=embedding.embedding) ) return data def upsert(self, embeddings): data = self.convert(embeddings) self.client.collections.get(self.index_name).data.insert_many(data) def delete_index(self, index_name: str): self.client.collections.delete(index_name) ### Start the client and index URL = "your-weaviate-url" API_KEY = "your-weaviate-api-key" weaviate_adapter = WeaviateAdapter(API_KEY, URL) index_name = "Test_index" if index_name in weaviate_adapter.client.collections.list_all(): weaviate_adapter.delete_index(index_name) weaviate_adapter.create_index("Test_index")
Step 2: Create the Embedding Model
Here, since we are embedding images, we can use the clip model
import embed_anything import WhichModel model = embed_anything.EmbeddingModel.from_pretrained_cloud( embed_anything.WhichModel.Clip, model_)
Step 3: Embed the Directory
data = embed_anything.embed_image_directory( "\image_directory", embeder=model, adapter=weaviate_adapter, config=embed_anything.ImageEmbedConfig(buffer_size=100), )
Step 4: Query the Vector Database
query_vector = embed_anything.embed_query(["image of a cat"], embeder=model)[0].embedding
Step 5: Query the Vector Database
response = weaviate_adapter.collection.query.near_vector( near_vector=query_vector, limit=2, return_metadata=wvc.query.MetadataQuery(certainty=True), ) Check the response;
Output
Using the Clip model, we vectorized the whole directory with pictures of cats, dogs, and monkeys. With the simple query “images of cats, ” we were able to search all the files for images of cats.
Check out the notebook for the code here on colab.
Conclusion
I think vector streaming is one of the features that will empower many engineers to opt for a more optimized and no-tech debt solution. Instead of using bulky frameworks on the cloud, you can use a lightweight streaming option.
Check out the GitHub repo over here: EmbedAnything Repo.
Frequently Asked Questions
Q1. What is vector streaming in EmbedAnything?Ans. Vector streaming is a feature that optimizes large-scale document embedding by using Rust’s concurrency for asynchronous chunking and embedding, reducing memory usage and speeding up the process.
Q2. What problem does vector streaming solve?Ans. It addresses high memory usage and inefficiency in traditional embedding methods by processing chunks asynchronously, reducing bottlenecks and optimizing resource use.
Q3. How does vector streaming work with Weaviate?Ans. It uses an adapter to connect EmbedAnything with the Weaviate Vector Database, allowing seamless embedding and querying of data.
Q4. What are the steps for using vector streaming?Ans. Here are steps:
1. Create a database adapter.
2. Initiate an embedding model.
3. Embed the directory.
4. Query the vector database.
Ans. It offers better efficiency, reduced memory usage, scalability, and flexibility compared to traditional embedding methods.
The above is the detailed content of Vector Streaming: Memory-efficient Indexing with Rust. For more information, please follow other related articles on the PHP Chinese website!
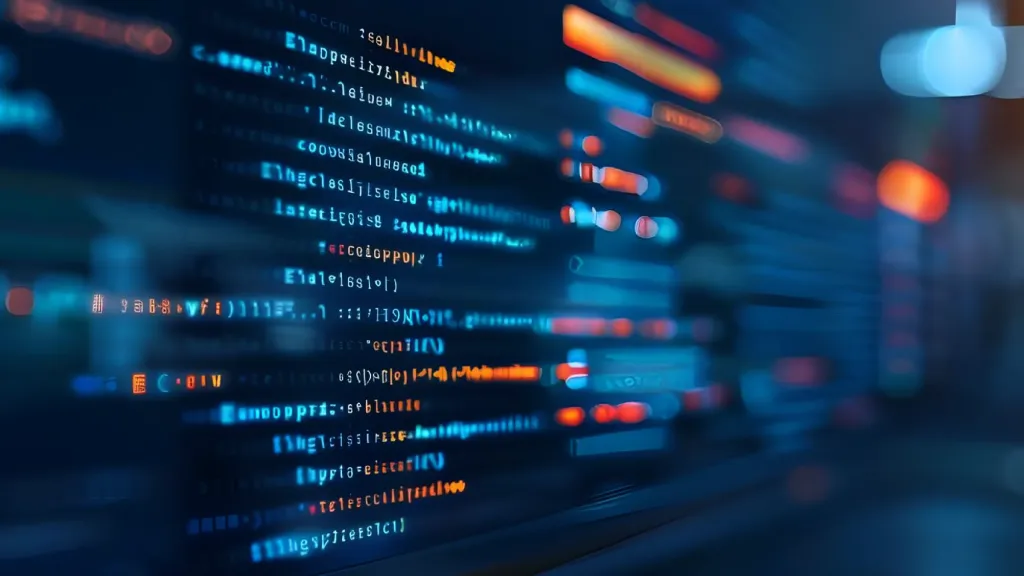
Running large language models at home with ease: LM Studio User Guide In recent years, advances in software and hardware have made it possible to run large language models (LLMs) on personal computers. LM Studio is an excellent tool to make this process easy and convenient. This article will dive into how to run LLM locally using LM Studio, covering key steps, potential challenges, and the benefits of having LLM locally. Whether you are a tech enthusiast or are curious about the latest AI technologies, this guide will provide valuable insights and practical tips. Let's get started! Overview Understand the basic requirements for running LLM locally. Set up LM Studi on your computer
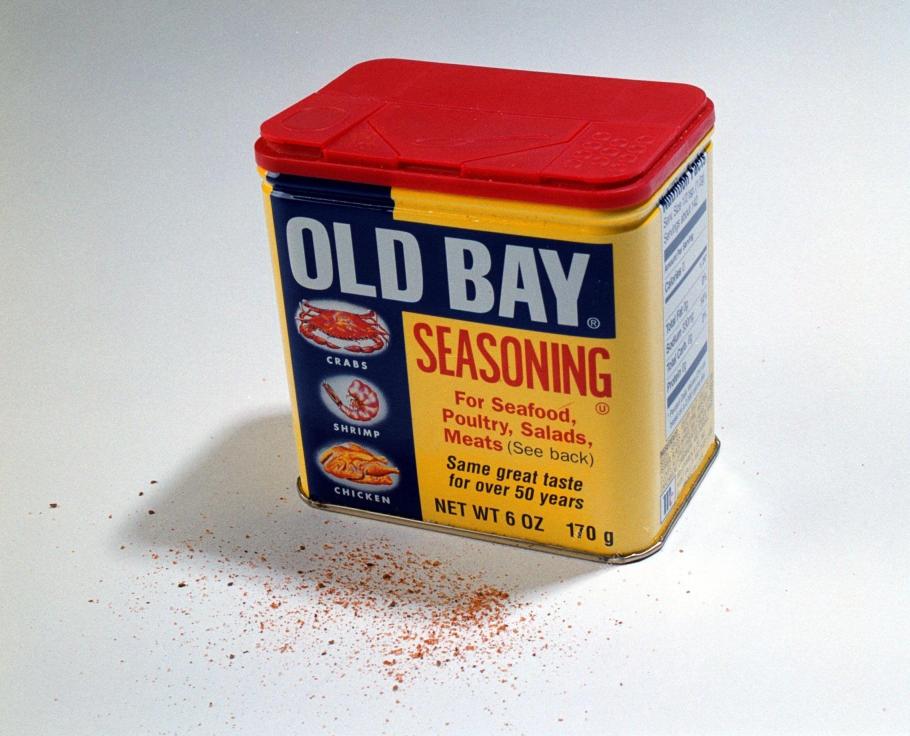
Guy Peri is McCormick’s Chief Information and Digital Officer. Though only seven months into his role, Peri is rapidly advancing a comprehensive transformation of the company’s digital capabilities. His career-long focus on data and analytics informs
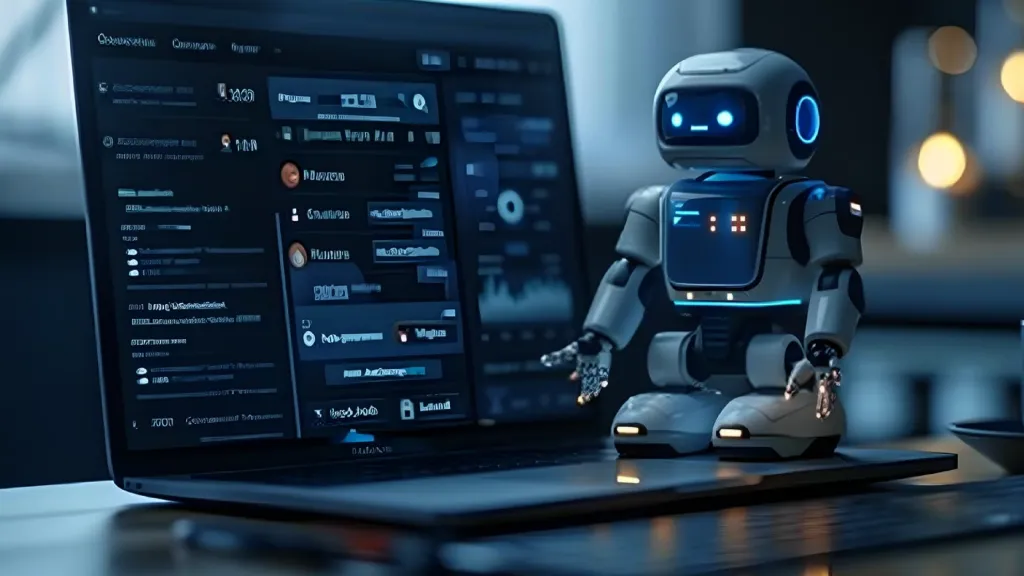
Introduction Artificial intelligence (AI) is evolving to understand not just words, but also emotions, responding with a human touch. This sophisticated interaction is crucial in the rapidly advancing field of AI and natural language processing. Th
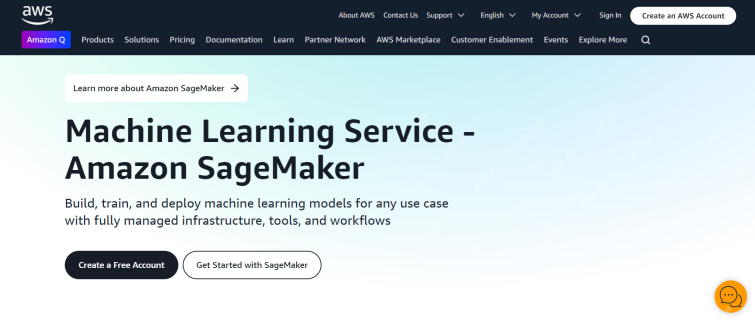
Introduction In today's data-centric world, leveraging advanced AI technologies is crucial for businesses seeking a competitive edge and enhanced efficiency. A range of powerful tools empowers data scientists, analysts, and developers to build, depl
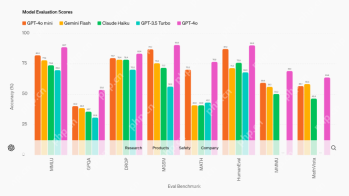
This week's AI landscape exploded with groundbreaking releases from industry giants like OpenAI, Mistral AI, NVIDIA, DeepSeek, and Hugging Face. These new models promise increased power, affordability, and accessibility, fueled by advancements in tr
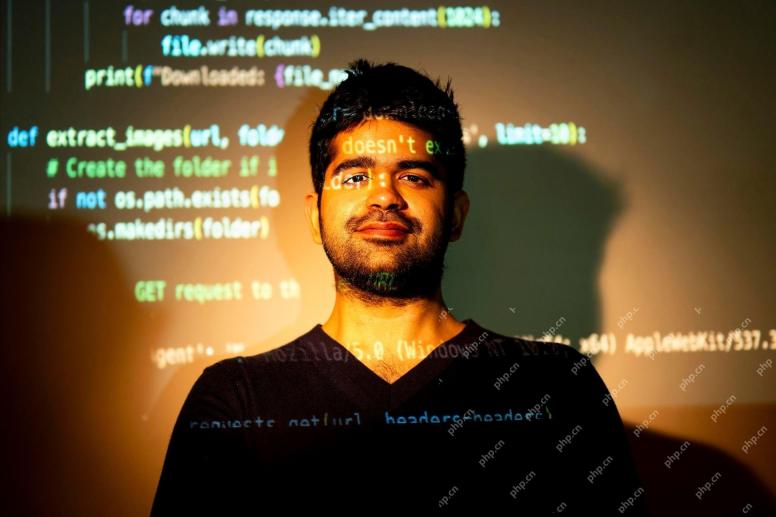
But the company’s Android app, which offers not only search capabilities but also acts as an AI assistant, is riddled with a host of security issues that could expose its users to data theft, account takeovers and impersonation attacks from malicious
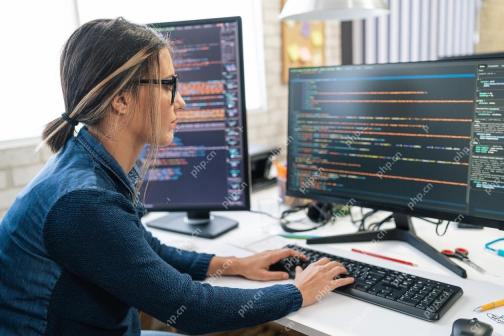
You can look at what’s happening in conferences and at trade shows. You can ask engineers what they’re doing, or consult with a CEO. Everywhere you look, things are changing at breakneck speed. Engineers, and Non-Engineers What’s the difference be
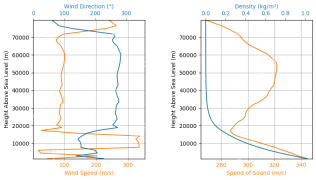
Simulate Rocket Launches with RocketPy: A Comprehensive Guide This article guides you through simulating high-power rocket launches using RocketPy, a powerful Python library. We'll cover everything from defining rocket components to analyzing simula


Hot AI Tools

Undresser.AI Undress
AI-powered app for creating realistic nude photos

AI Clothes Remover
Online AI tool for removing clothes from photos.

Undress AI Tool
Undress images for free

Clothoff.io
AI clothes remover

Video Face Swap
Swap faces in any video effortlessly with our completely free AI face swap tool!

Hot Article

Hot Tools

SublimeText3 Linux new version
SublimeText3 Linux latest version
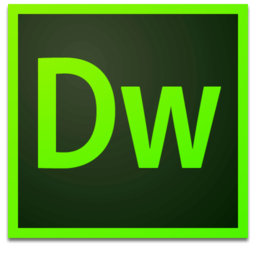
Dreamweaver Mac version
Visual web development tools
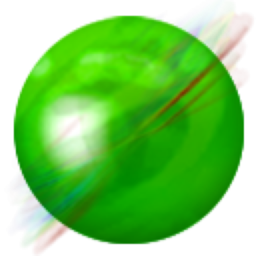
ZendStudio 13.5.1 Mac
Powerful PHP integrated development environment

SecLists
SecLists is the ultimate security tester's companion. It is a collection of various types of lists that are frequently used during security assessments, all in one place. SecLists helps make security testing more efficient and productive by conveniently providing all the lists a security tester might need. List types include usernames, passwords, URLs, fuzzing payloads, sensitive data patterns, web shells, and more. The tester can simply pull this repository onto a new test machine and he will have access to every type of list he needs.
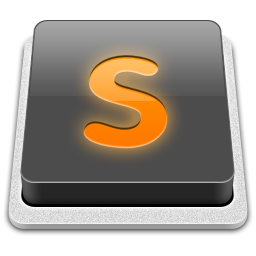
SublimeText3 Mac version
God-level code editing software (SublimeText3)