Introduction
Don’t want to spend money on APIs, or are you concerned about privacy? Or do you just want to run LLMs locally? Don’t worry; this guide will help you build agents and multi-agent frameworks with local LLMs that are completely free. We’ll explore how to build a multi-agent system with CrewAI and Ollama and look at the multiple LLMs available from Ollama.
Overview
- This guide focuses on building agentic frameworks and multi-agent systems using local LLMs with CrewAI and Ollama, providing a cost-free and privacy-preserving solution.
- It introduces key concepts of agents and Multi-Agentic Framework with CrewAI and Ollama, emphasizing their role in autonomous, collaborative problem-solving across various industries.
- CrewAI is highlighted as an advanced framework for orchestrating tasks between agents. It uses structured roles, goals, and memory management to improve task execution.
- Ollama enables running language models like Llama2, Llama3, and LLaVA locally, allowing users to bypass cloud services for AI tasks.
- The article includes a practical example of building a Multi-Agent System for image classification, description, and information retrieval using CrewAI and Ollama.
- The conclusion underscores the benefits of using different LLMs for specialized tasks and showcases the flexibility of combining CrewAI and Ollama in local environments.
Table of contents
- Introduction
- Agents, Agentic Frameworks, and CrewAI
- Agentic Frameworks
- CrewAI Framework
- Key Strengths of CrewAI
- Ollama
- Building a Multi-Agent System
- Objectives
- Components
- Let’s Build our Multi-Agent System
- Import Required Libraries
- Define the Agents
- Define Tasks for Each Agent
- Managing Agents and Tasks with a Crew
- Conclusion
- Frequently Asked Questions
Agents, Agentic Frameworks, and CrewAI
Generative AI has transitioned from basic large language models (LLM) to advanced multi-agent systems. In theory, Agents are autonomous systems capable of planning, reasoning, and acting without human input. These agents aim to reduce human involvement while expanding functionality.
Also Read: Top 5 Frameworks for Building AI Agents in 2024
Agentic Frameworks
These frameworks utilize multiple agents working in concert, allowing for collaboration, communication, and problem-solving that exceed the capabilities of single-use agents. In these frameworks, agents have distinct roles and goals and can perform complex tasks. Multi-Agentic Framework such as CrewAI and Ollama are essential for large-scale, dynamic, and distributed problem-solving, making them adaptable across industries like robotics, finance, healthcare, and beyond.
Key Components of Agentic Frameworks
- Agent Architecture: Defines the internal structure of agents, including planning, reasoning, and communication protocols.
- Communication Protocols: Methods for agent collaboration through messaging and data exchange.
- Agent Interaction Design: Mechanisms for agent collaboration, including task allocation and conflict resolution.
- Environment: The setting where agents interact, often including external tools and resources.
These frameworks enable modular and scalable systems, making modifying or adding agents to adapt to evolving requirements easy.
CrewAI Framework
crewAI is an advanced multi-agentic framework, enabling multiple agents (called a “crew”) to collaborate through task orchestration. The framework divides agents into three attributes—role, goal, and backstory—ensuring a thorough understanding of each agent’s function. This structured approach mitigates under-specification risk, improving task definition and execution.
Key Strengths of CrewAI
- Explicit Task Definition: Tasks are well-defined, ensuring clarity in what each agent does.
- Tool Use: Task-specific tools precede agent-level tools, creating a more granular and controlled toolset.
- Agent Interaction Processes: crewAI supports sequential and hierarchical agent collaboration processes.
- Advanced Memory Management: The framework provides short-term, long-term, entity, and contextual memory, facilitating sophisticated reasoning and learning.
Also Read: Building Collaborative AI Agents With CrewAI
Ollama
Ollama is a framework for building and running language models on local machines. It’s easy to use, as we can run models directly on devices without needing cloud-based services. There’s no concern about privacy.
To interact with Ollama:
We can run the pip install ollama command to integrate Ollama with Python.
Now, we can download models with the ollama pull command to download the models.
Let’s run these:
ollama pull llama2 ollama pull llama3 ollama pull llava
Now, we have 3 of the Large Language Models (LLMs) locally:
- Llama 2: An open-source large language model from Meta.
- Llama 3: The latest iteration of Meta’s Llama series, further refining capabilities for complex language generation tasks with increased parameter size and efficiency.
- LLaVA: A vision-language model designed for image and text understanding tasks.
We can use these models locally by running ollama run model-name, here’s an example:
You can press ctrl d to exit.
Also read: How to Run LLM Models Locally with Ollama?
Building a Multi-Agent System
Let’s work on building an Agentic system that takes an image as an input and gives a few interesting facts about the animal in the system.
Objectives
- Build a multi-agent system for image classification, description, and information retrieval using CrewAI.
- Automate decision-making: Agents perform specific tasks like identifying animals in images, describing them, and fetching relevant facts.
- Task sequencing: Coordinate agents through tasks in a stepwise, agentic system.
Components
- Classifier Agent: Identifies whether the input image contains an animal using the llava:7b model.
- Description Agent: Describes the animal in the image, also powered by llava:7b.
- Information Retrieval Agent: Fetches additional facts about the animal using llama2.
- Task Definitions: Each task is tied to a specific agent, guiding its action.
- Crew Management: The Crew coordinates agent actions, executes tasks, and aggregates results based on the input image
By default, tasks are executed sequentially in CrewAI. You can add a task manager to control the order of execution. Additionally, the allow_delegation feature allows an agent to ask its preceding agent to regenerate a response if needed. Setting memory to True enables agents to learn from past interactions, and you can optionally configure tasks to ask for human feedback about the output.
Also read: Building Collaborative AI Agents With CrewAI
Let’s Build our Multi-Agent System
Before we start, let’s install all the necessary packages:
pip install crewai pip install 'crewai[tools]' pip install ollama
Import Required Libraries
from crewai import Agent, Task, Crew import pkg_resources # Get the version of CrewAI crewai_version = pkg_resources.get_distribution("crewai").version print(crewai_version) 0.61.0
Define the Agents
Here, we define three agents with specific roles and goals. Each agent is responsible for a task related to image classification and description.
- Classifier Agent: Checks if the image contains an animal, uses llava:7b model to classify the animal.
- Description Agent: Describes the animal in the image. This also uses the same llava:7b model like the preceding agent.
- Information Retrieval Agent: This agent retrieves additional information or interesting facts about the animal. It uses llama2 to provide this information.
# 1. Image Classifier Agent (to check if the image is an animal) classifier_agent = Agent( role="Image Classifier Agent", goal="Determine if the image is of an animal or not", backstory=""" You have an eye for animals! Your job is to identify whether the input image is of an animal or something else. """, llm='ollama/llava:7b' # Model for image-related tasks ) # 2. Animal Description Agent (to describe the animal in the image) description_agent = Agent( role="Animal Description Agent {image_path}", goal="Describe the animal in the image", backstory=""" You love nature and animals. Your task is to describe any animal based on an image. """, llm='ollama/llava:7b' # Model for image-related tasks ) # 3. Information Retrieval Agent (to fetch additional info about the animal) info_agent = Agent( role="Information Agent", goal="Give compelling information about a certain animal", backstory=""" You are very good at telling interesting facts. You don't give any wrong information if you don't know it. """, llm='ollama/llama2' # Model for general knowledge retrieval )
Also Read: Agentic Frameworks for Generative AI Applications
Define Tasks for Each Agent
Each task is tied to one of the agents. Tasks describe the input, the expected output, and which agent should handle it.
- Task 1: Classify whether the image contains an animal.
- Task 2: If the image is classified as an animal, describe it.
- Task 3: Provide additional information about the animal based on the description.
# Task 1: Check if the image is an animal task1 = Task( description="Classify the image ({image_path}) and tell me if it's an animal.", expected_output="If it's an animal, say 'animal'; otherwise, say 'not an animal'.", agent=classifier_agent ) # Task 2: If it's an animal, describe it task2 = Task( description="Describe the animal in the image.({image_path})", expected_output="Give a detailed description of the animal.", agent=description_agent ) # Task 3: Provide more information about the animal task3 = Task( description="Give additional information about the described animal.", expected_output="Provide at least 5 interesting facts or information about the animal.", agent=info_agent )
Managing Agents and Tasks with a Crew
A Crew is set up to manage the agents and tasks. It coordinates the tasks sequentially and provides the results based on the agents’ chains of thought.
# Crew to manage the agents and tasks crew = Crew( agents=[classifier_agent, description_agent, info_agent], tasks=[task1, task2, task3], verbose=True ) # Execute the tasks with the provided image path result = crew.kickoff(inputs={'image_path': 'racoon.jpg'})
I’ve given an image of a racoon to the crewAI framework, and this is the output that I got:
Note: Ensure the image is in the working directory, or you can give the full path.
OUTPUT
# Agent: Image Classifier Agent<br><br>## Task: Classify the image (racoon.jpg) and tell me if it's an animal.<br><br># Agent: Image Classifier Agent<br><br>## Final Answer:<br><br>Based on my analysis, the image (racoon.jpg) contains a raccoon, which is<br> indeed an animal. Therefore, the final answer is 'animal'.<br><br># Agent: Animal Description Agent racoon.jpg<br><br>## Task: Describe the animal in the image.(racoon.jpg)<br><br># Agent: Animal Description Agent racoon.jpg<br><br>## Final Answer:<br><br>The image (racoon.jpg) features a raccoon, which is a mammal known for its<br> agility and adaptability to various environments. Raccoons are characterized<br> by their distinct black "mask" around the eyes and ears, as well as a<br> grayish or brownish coat with white markings on the face and paws. They have<br> a relatively short tail and small rounded ears. Raccoons are omnivorous and<br> have a highly dexterous front paw that they use to manipulate objects. They<br> are also known for their intelligence and ability to solve problems, such as<br> opening containers or climbing trees.<br><br># Agent: Information Agent<br><br>## Task: Give additional information about the described animal.<br><br># Agent: Information Agent<br><br>## Final Answer:<br><br>Here are 5 fascinating facts about the raccoon:<br><br>1. Raccoons have exceptional dexterity in their front paws, which they use to<br> manipulate objects with remarkable precision. In fact, studies have shown<br> that raccoons are able to open containers and perform other tasks with a<br> level of skill rivaling that of humans!<br><br>2. Despite their cute appearance, raccoons are formidable hunters and can<br> catch a wide variety of prey, including fish, insects, and small mammals.<br> Their sensitive snouts help them locate food in the dark waters or<br> underbrush.<br><br>3. Raccoons are highly adaptable and can be found in a range of habitats,<br> from forests to marshes to urban areas. They are even known to climb trees<br> and swim in water!<br><br>4. In addition to their intelligence and problem-solving skills, raccoons<br> have an excellent memory and are able to recognize and interact with<br> individual humans and other animals. They can also learn to perform tricks<br> and tasks through training.<br><br>5. Unlike many other mammals, raccoons do not hibernate during the winter<br> months. Instead, they enter a state of dormancy known as torpor, which<br> allows them to conserve energy and survive harsh weather conditions. During<br> this time, their heart rate slows dramatically, from around 70-80 beats per<br> minute to just 10-20!<br><br>I hope these interesting facts will provide a comprehensive understanding of<br> the fascinating raccoon species!
The classifier confirmed that it was an animal, and then the agent with the llava:7b model described the animal and image and sequentially passed it to the information agent. Despite the information agent using llama2, a text-based model, it could use the context from the previous agent and give information about a raccoon.
Also read: Building a Responsive Chatbot with Llama 3.1, Ollama and LangChain
Conclusion
Using multiple LLMs according to their strengths is good because different models excel at different tasks. We have used CrewAI and Ollama to showcase multi-agent collaboration and also used LLMs locally from Ollama. Yes, the Ollama models might be slower than cloud-based models for obvious reasons, but both have pros and cons. The effectiveness of the agentic framework depends on the workflows and the use of the right tools and LLMs to optimize the results.
Frequently Asked Questions
Q1. What’s allow_delegation in CrewAI?Ans. When set to True, this crewAI parameter lets agents assign tasks to others, enabling complex task flows and collaboration.
Q2. How does crewAI use Pydantic objects?Ans. crewAI uses Pydantic objects to define and validate task input/output data structures, ensuring agents receive and produce data in the expected format.
Q3. How does crewAI manage task flow and agent collaboration?Ans. crewAI manages this by organizing agents and tasks into a ‘Crew’ object, coordinating tasks sequentially based on user-defined dependencies.
Q4. Can I use custom LLMs with crewAI and Ollama?Ans. Yes, both support custom LLMs. For crewAI, specify the model path/name when creating an Agent. For Ollama, follow their docs to build and run custom models.
The above is the detailed content of How to Build Multi-Agent System with CrewAI and Ollama?. For more information, please follow other related articles on the PHP Chinese website!
![Can't use ChatGPT! Explaining the causes and solutions that can be tested immediately [Latest 2025]](https://img.php.cn/upload/article/001/242/473/174717025174979.jpg?x-oss-process=image/resize,p_40)
ChatGPT is not accessible? This article provides a variety of practical solutions! Many users may encounter problems such as inaccessibility or slow response when using ChatGPT on a daily basis. This article will guide you to solve these problems step by step based on different situations. Causes of ChatGPT's inaccessibility and preliminary troubleshooting First, we need to determine whether the problem lies in the OpenAI server side, or the user's own network or device problems. Please follow the steps below to troubleshoot: Step 1: Check the official status of OpenAI Visit the OpenAI Status page (status.openai.com) to see if the ChatGPT service is running normally. If a red or yellow alarm is displayed, it means Open
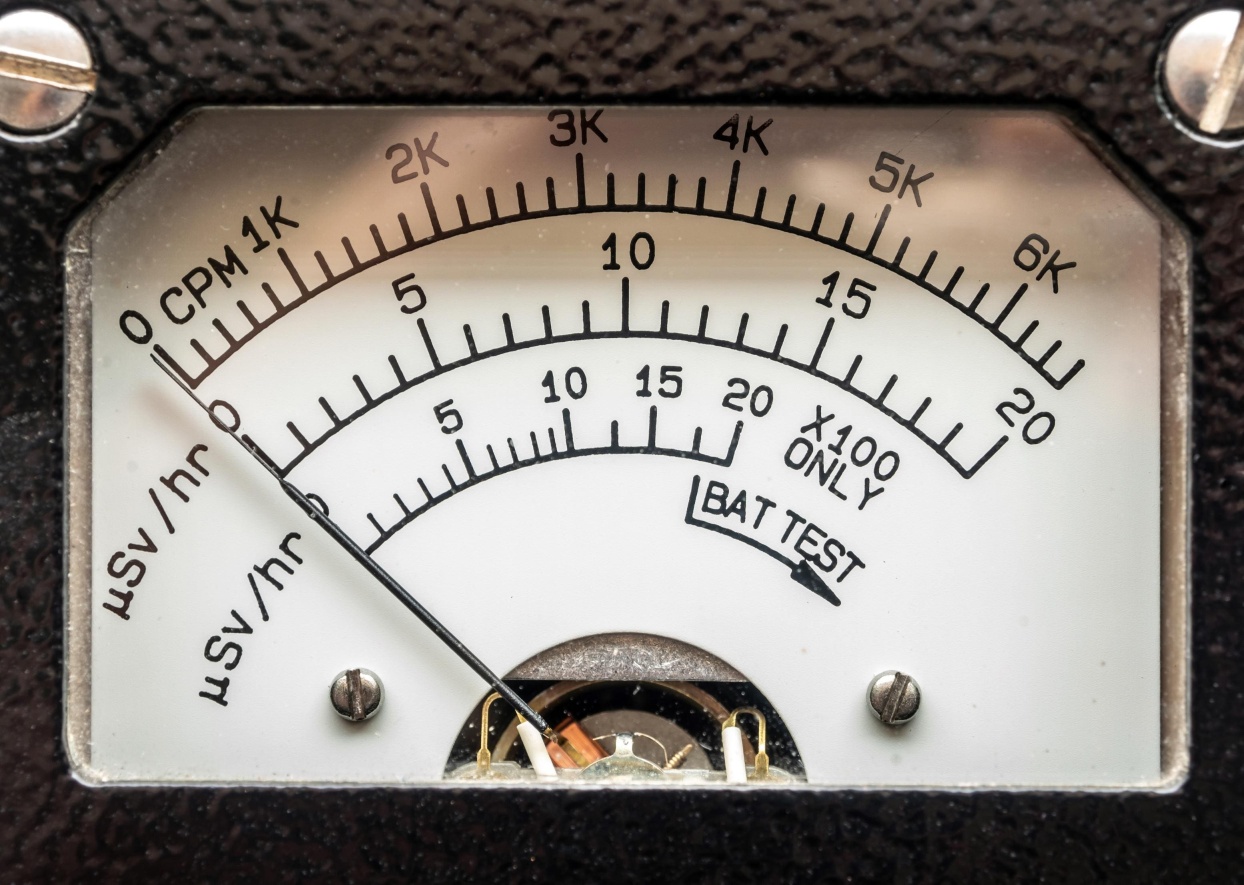
On 10 May 2025, MIT physicist Max Tegmark told The Guardian that AI labs should emulate Oppenheimer’s Trinity-test calculus before releasing Artificial Super-Intelligence. “My assessment is that the 'Compton constant', the probability that a race to
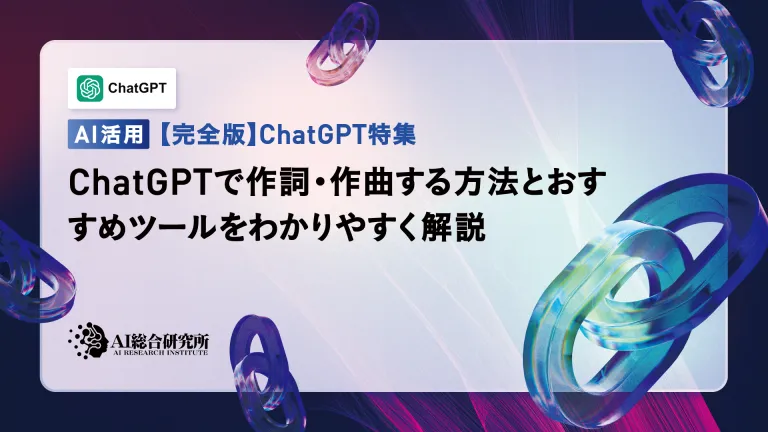
AI music creation technology is changing with each passing day. This article will use AI models such as ChatGPT as an example to explain in detail how to use AI to assist music creation, and explain it with actual cases. We will introduce how to create music through SunoAI, AI jukebox on Hugging Face, and Python's Music21 library. Through these technologies, everyone can easily create original music. However, it should be noted that the copyright issue of AI-generated content cannot be ignored, and you must be cautious when using it. Let’s explore the infinite possibilities of AI in the music field together! OpenAI's latest AI agent "OpenAI Deep Research" introduces: [ChatGPT]Ope
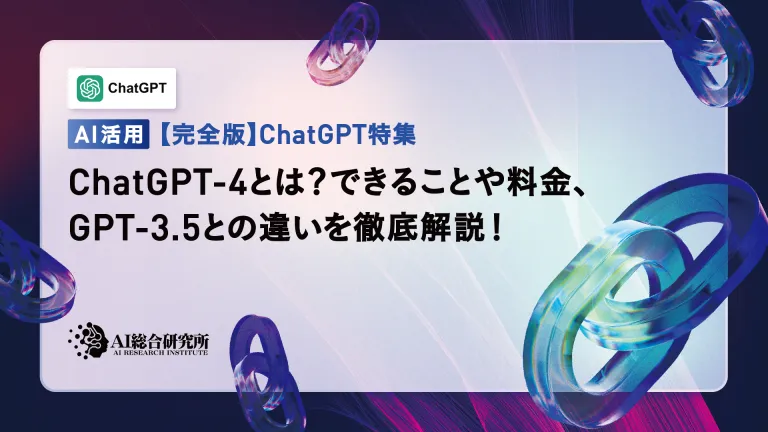
The emergence of ChatGPT-4 has greatly expanded the possibility of AI applications. Compared with GPT-3.5, ChatGPT-4 has significantly improved. It has powerful context comprehension capabilities and can also recognize and generate images. It is a universal AI assistant. It has shown great potential in many fields such as improving business efficiency and assisting creation. However, at the same time, we must also pay attention to the precautions in its use. This article will explain the characteristics of ChatGPT-4 in detail and introduce effective usage methods for different scenarios. The article contains skills to make full use of the latest AI technologies, please refer to it. OpenAI's latest AI agent, please click the link below for details of "OpenAI Deep Research"
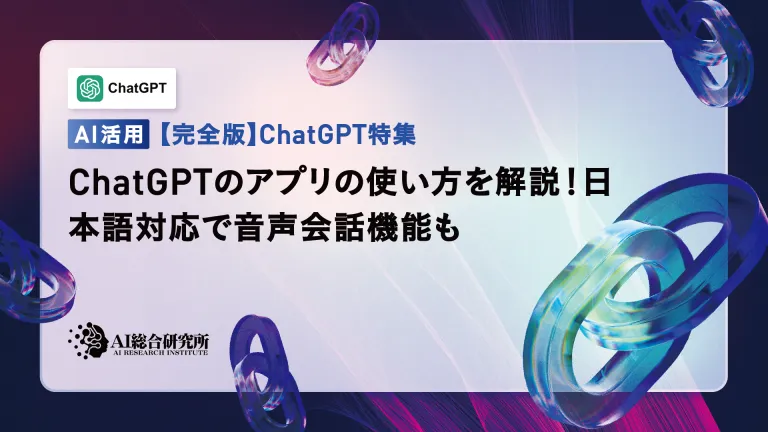
ChatGPT App: Unleash your creativity with the AI assistant! Beginner's Guide The ChatGPT app is an innovative AI assistant that handles a wide range of tasks, including writing, translation, and question answering. It is a tool with endless possibilities that is useful for creative activities and information gathering. In this article, we will explain in an easy-to-understand way for beginners, from how to install the ChatGPT smartphone app, to the features unique to apps such as voice input functions and plugins, as well as the points to keep in mind when using the app. We'll also be taking a closer look at plugin restrictions and device-to-device configuration synchronization
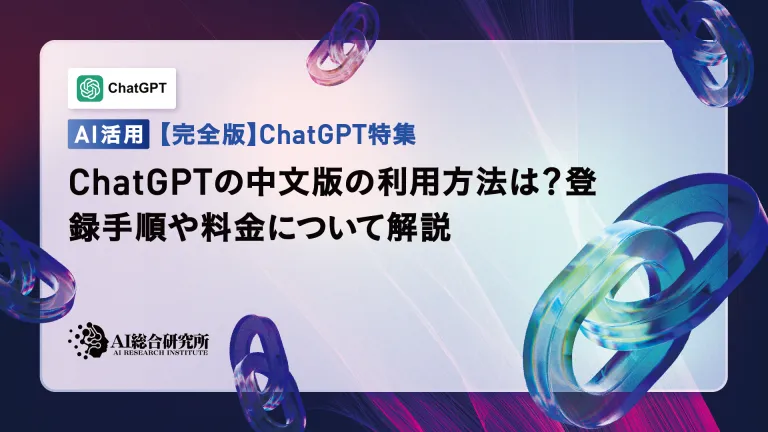
ChatGPT Chinese version: Unlock new experience of Chinese AI dialogue ChatGPT is popular all over the world, did you know it also offers a Chinese version? This powerful AI tool not only supports daily conversations, but also handles professional content and is compatible with Simplified and Traditional Chinese. Whether it is a user in China or a friend who is learning Chinese, you can benefit from it. This article will introduce in detail how to use ChatGPT Chinese version, including account settings, Chinese prompt word input, filter use, and selection of different packages, and analyze potential risks and response strategies. In addition, we will also compare ChatGPT Chinese version with other Chinese AI tools to help you better understand its advantages and application scenarios. OpenAI's latest AI intelligence
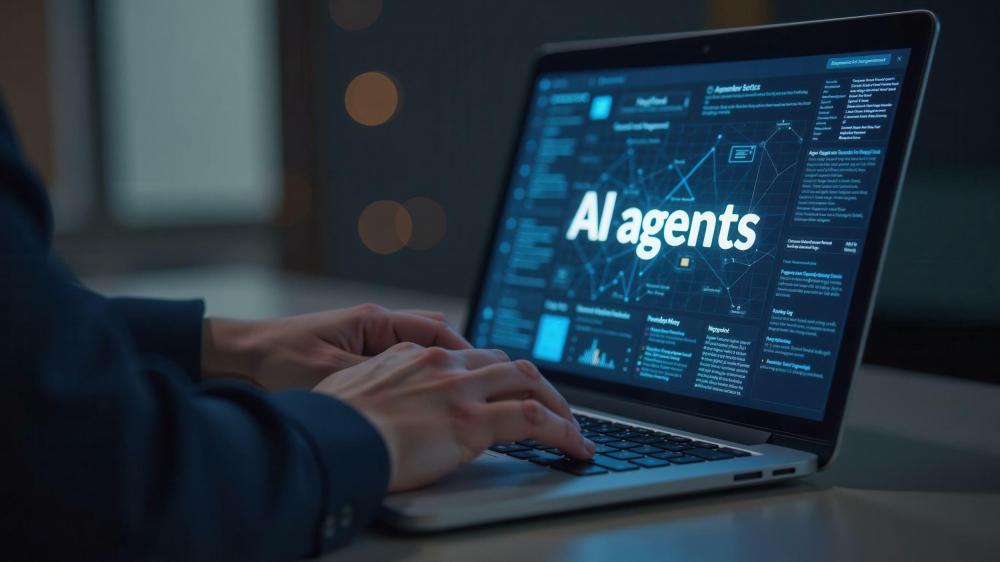
These can be thought of as the next leap forward in the field of generative AI, which gave us ChatGPT and other large-language-model chatbots. Rather than simply answering questions or generating information, they can take action on our behalf, inter
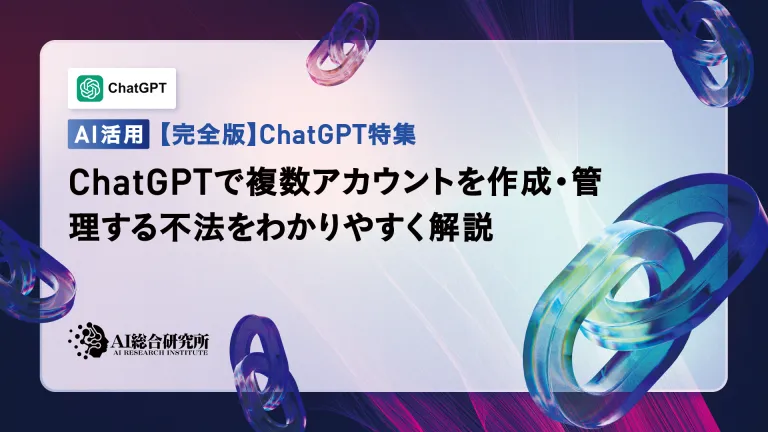
Efficient multiple account management techniques using ChatGPT | A thorough explanation of how to use business and private life! ChatGPT is used in a variety of situations, but some people may be worried about managing multiple accounts. This article will explain in detail how to create multiple accounts for ChatGPT, what to do when using it, and how to operate it safely and efficiently. We also cover important points such as the difference in business and private use, and complying with OpenAI's terms of use, and provide a guide to help you safely utilize multiple accounts. OpenAI


Hot AI Tools

Undresser.AI Undress
AI-powered app for creating realistic nude photos

AI Clothes Remover
Online AI tool for removing clothes from photos.

Undress AI Tool
Undress images for free

Clothoff.io
AI clothes remover

Video Face Swap
Swap faces in any video effortlessly with our completely free AI face swap tool!

Hot Article

Hot Tools

Safe Exam Browser
Safe Exam Browser is a secure browser environment for taking online exams securely. This software turns any computer into a secure workstation. It controls access to any utility and prevents students from using unauthorized resources.

DVWA
Damn Vulnerable Web App (DVWA) is a PHP/MySQL web application that is very vulnerable. Its main goals are to be an aid for security professionals to test their skills and tools in a legal environment, to help web developers better understand the process of securing web applications, and to help teachers/students teach/learn in a classroom environment Web application security. The goal of DVWA is to practice some of the most common web vulnerabilities through a simple and straightforward interface, with varying degrees of difficulty. Please note that this software
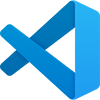
VSCode Windows 64-bit Download
A free and powerful IDE editor launched by Microsoft

SublimeText3 Chinese version
Chinese version, very easy to use
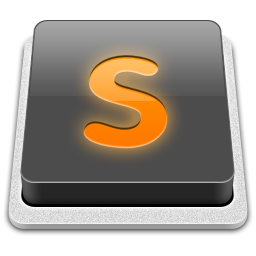
SublimeText3 Mac version
God-level code editing software (SublimeText3)
