Understanding Generative AI: How It Works and Key Examples
Generative AI refers to a subset of artificial intelligence focused on creating new content, ranging from images and text to music and even synthetic data. It operates by learning the patterns and structures within existing datasets and then using that knowledge to generate new, original outputs that mimic the style and characteristics of the training data.
At the core of generative AI are models such as Generative Adversarial Networks (GANs), Variational Autoencoders (VAEs), and more recently, transformer-based architectures like those used in large language models. For instance, a GAN consists of two neural networks, the generator and the discriminator, which work in tandem. The generator creates new content, while the discriminator evaluates it against real data, refining the generator's output over time to improve realism.
Key examples of generative AI in action include:
- DALL-E and Midjourney: These tools generate high-quality images from textual descriptions, showcasing the ability of generative AI to create visual content that aligns with specific user prompts.
- Jukebox by OpenAI: This system generates music across various genres and styles, illustrating the potential of generative AI in the music industry.
- GPT-3 and subsequent models: These language models generate human-like text, enabling applications in content creation, chatbots, and more.
What are the practical applications of generative AI in various industries?
Generative AI has found practical applications across numerous industries, revolutionizing how businesses operate and create value:
- Healthcare: Generative AI can create synthetic medical data for training purposes, assist in drug discovery by generating potential molecular structures, and even produce personalized treatment plans based on patient data.
- Entertainment and Media: In the film and gaming industries, generative AI can create realistic characters, scenes, and soundtracks, significantly reducing production costs and time. It can also generate scripts or dialogue, adding a new dimension to content creation.
- Fashion and Design: Generative models can design new clothing and accessories by learning from existing styles and trends, enabling rapid prototyping and customization.
- Advertising and Marketing: AI-generated content can be used to create personalized ad campaigns and marketing materials. It can also generate diverse sets of images for social media to engage different demographics.
- Automotive and Manufacturing: Generative design tools can optimize parts and components, leading to lighter, stronger, and more efficient designs. This is particularly useful in industries where weight and performance are critical.
- Finance: In the financial sector, generative AI can be used to detect fraud through the generation of synthetic data for training fraud detection models, and it can also assist in creating personalized financial advice or investment strategies.
Can you explain the underlying technology that powers generative AI?
The underlying technology of generative AI primarily involves advanced machine learning techniques, with several key methods standing out:
- Generative Adversarial Networks (GANs): As mentioned earlier, GANs consist of two neural networks. The generator produces new data samples, while the discriminator assesses their authenticity. Through iterative competition, the generator improves its outputs to fool the discriminator, resulting in increasingly realistic data.
- Variational Autoencoders (VAEs): VAEs work by encoding input data into a latent space and then decoding it to generate new samples. They are particularly useful for learning the distribution of data, allowing for controlled generation of new instances.
- Transformer Models: Particularly in natural language processing, transformers like those used in models such as GPT-3 leverage attention mechanisms to understand and generate text. These models can capture long-range dependencies in data, making them highly effective for tasks like language translation and content creation.
- Diffusion Models: A more recent approach, diffusion models generate data by reversing a process that adds noise to the data. They have shown promising results in generating high-quality images and other types of data.
How does generative AI differ from traditional AI in terms of functionality and output?
Generative AI and traditional AI differ significantly in both functionality and output, reflecting their distinct approaches and applications:
-
Functionality:
- Generative AI: Focuses on creating new data or content. It learns patterns and structures from existing datasets and uses this knowledge to generate new samples that are original yet consistent with the learned patterns.
- Traditional AI: Typically involves classification, prediction, and decision-making based on existing data. Traditional models like decision trees, logistic regression, or neural networks are often used for tasks such as spam detection, recommendation systems, and autonomous driving.
-
Output:
- Generative AI: Produces outputs that are novel and not directly present in the training data. Examples include new images, text, music, and synthetic data that can be used for further training or simulation.
- Traditional AI: Outputs predictions, classifications, or decisions based on the patterns it has identified in the data it was trained on. The output is a response or action based on what the model has learned.
In essence, while traditional AI excels at understanding and interpreting existing data to make decisions or predictions, generative AI goes a step further by creating new, original content. This capability opens up new avenues for innovation and creativity across various domains.
The above is the detailed content of Understanding Generative AI: How It Works and Key Examples. For more information, please follow other related articles on the PHP Chinese website!
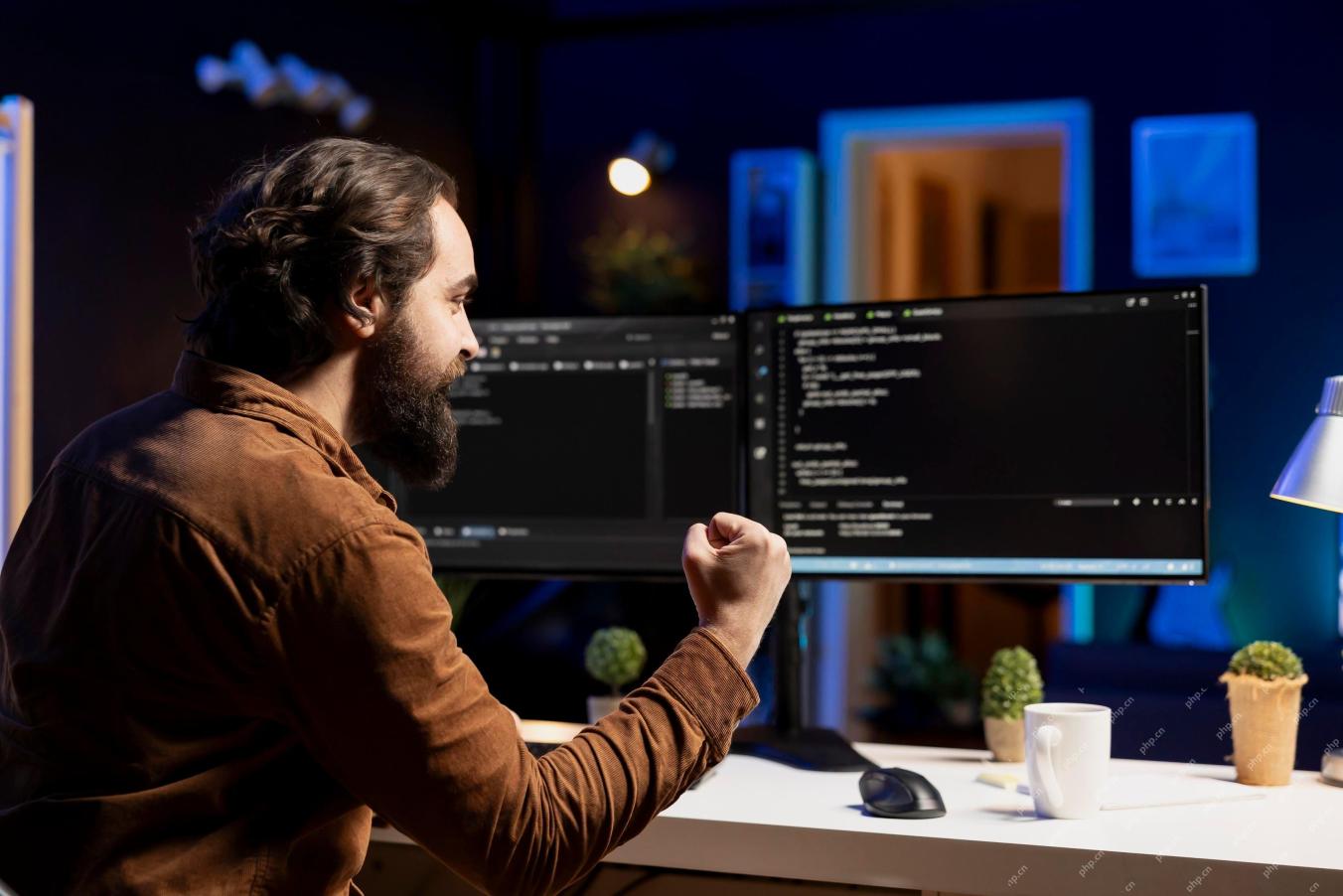
Let's discuss the rising use of "vibes" as an evaluation metric in the AI field. This analysis is part of my ongoing Forbes column on AI advancements, exploring complex aspects of AI development (see link here). Vibes in AI Assessment Tradi
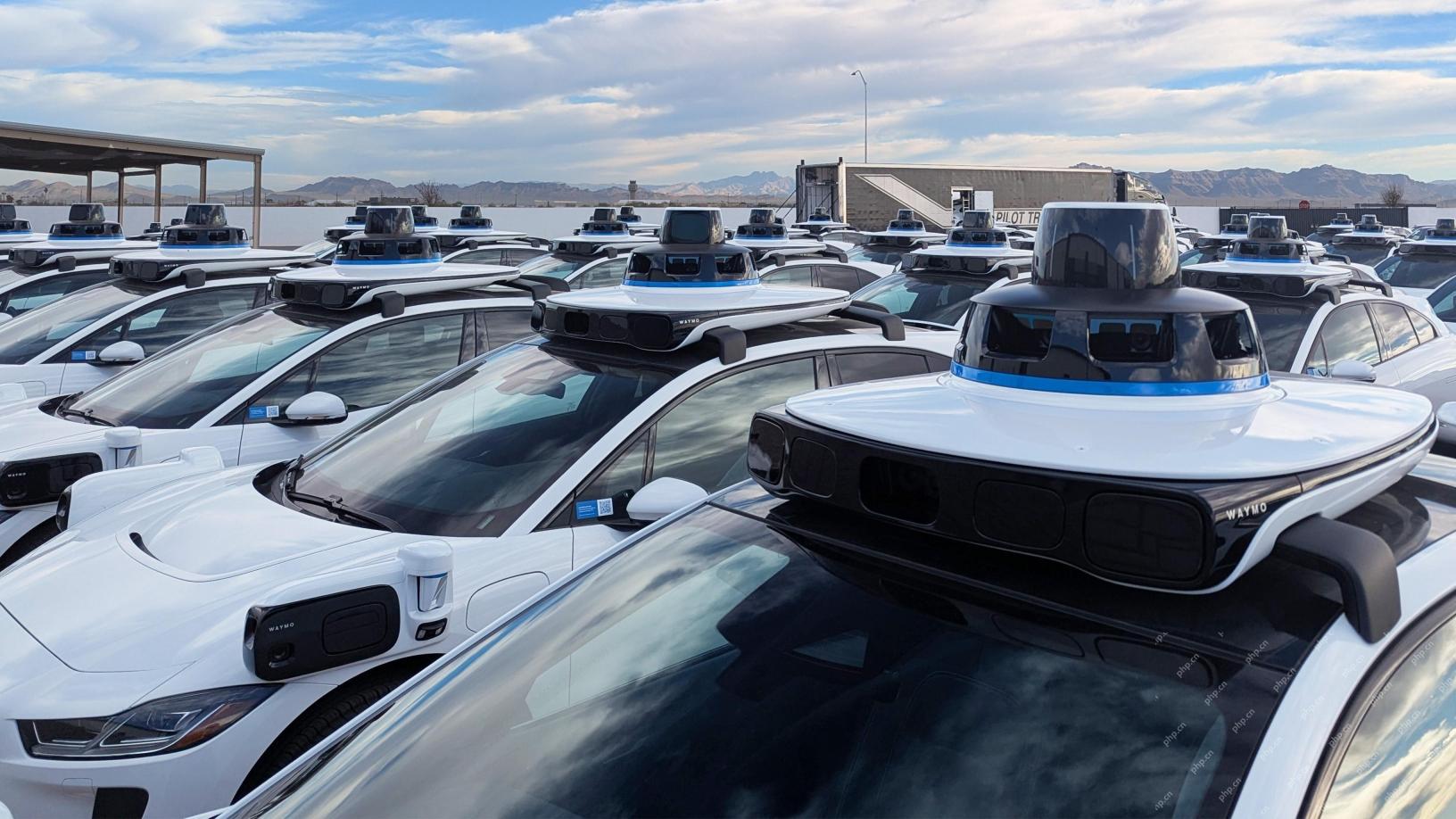
Waymo's Arizona Factory: Mass-Producing Self-Driving Jaguars and Beyond Located near Phoenix, Arizona, Waymo operates a state-of-the-art facility producing its fleet of autonomous Jaguar I-PACE electric SUVs. This 239,000-square-foot factory, opened
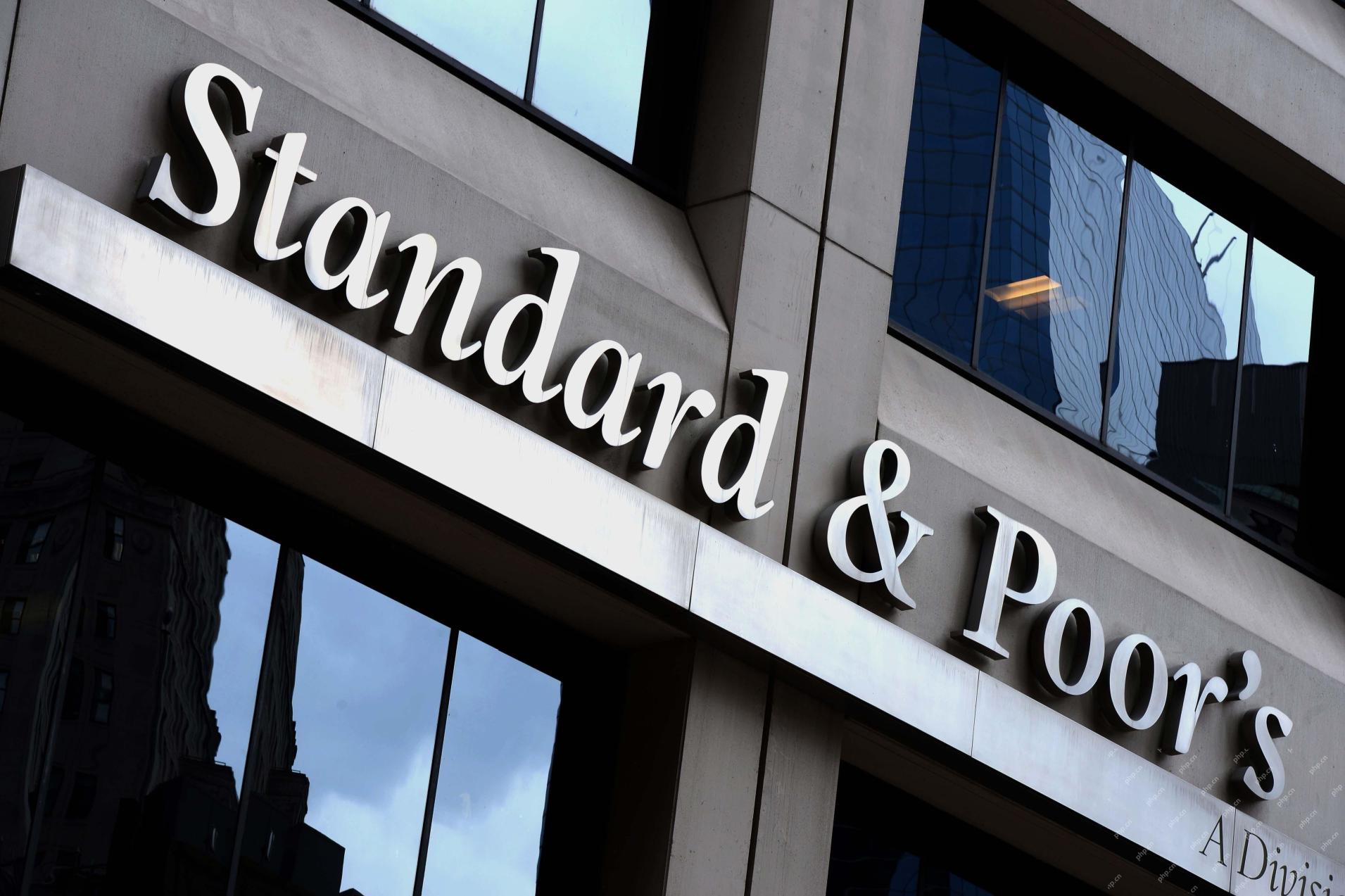
S&P Global's Chief Digital Solutions Officer, Jigar Kocherlakota, discusses the company's AI journey, strategic acquisitions, and future-focused digital transformation. A Transformative Leadership Role and a Future-Ready Team Kocherlakota's role
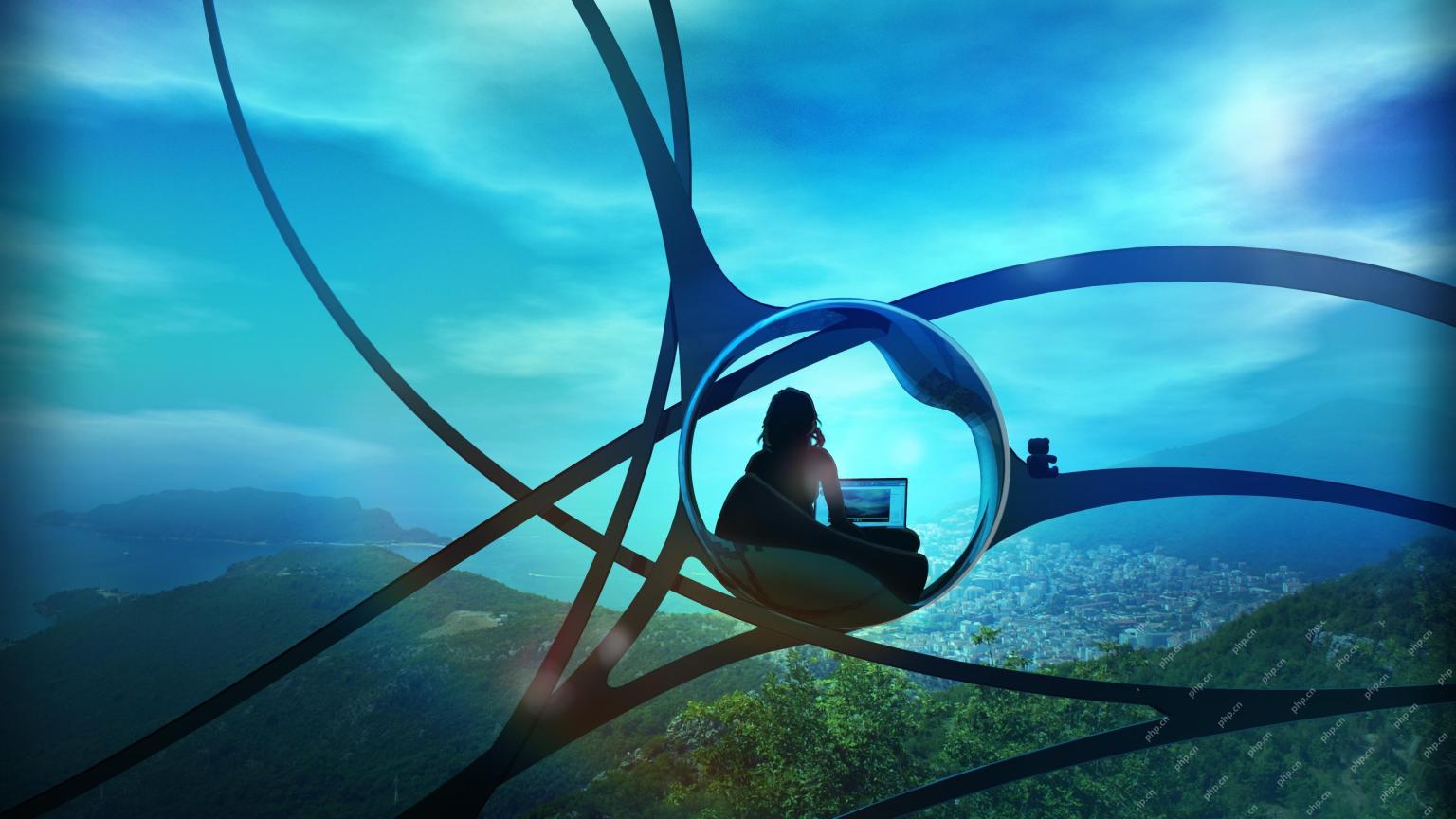
From Apps to Ecosystems: Navigating the Digital Landscape The digital revolution extends far beyond social media and AI. We're witnessing the rise of "everything apps"—comprehensive digital ecosystems integrating all aspects of life. Sam A
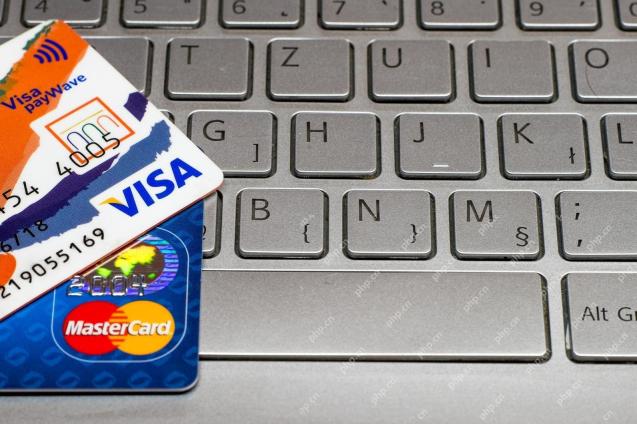
Mastercard's Agent Pay: AI-Powered Payments Revolutionize Commerce While Visa's AI-powered transaction capabilities made headlines, Mastercard has unveiled Agent Pay, a more advanced AI-native payment system built on tokenization, trust, and agentic
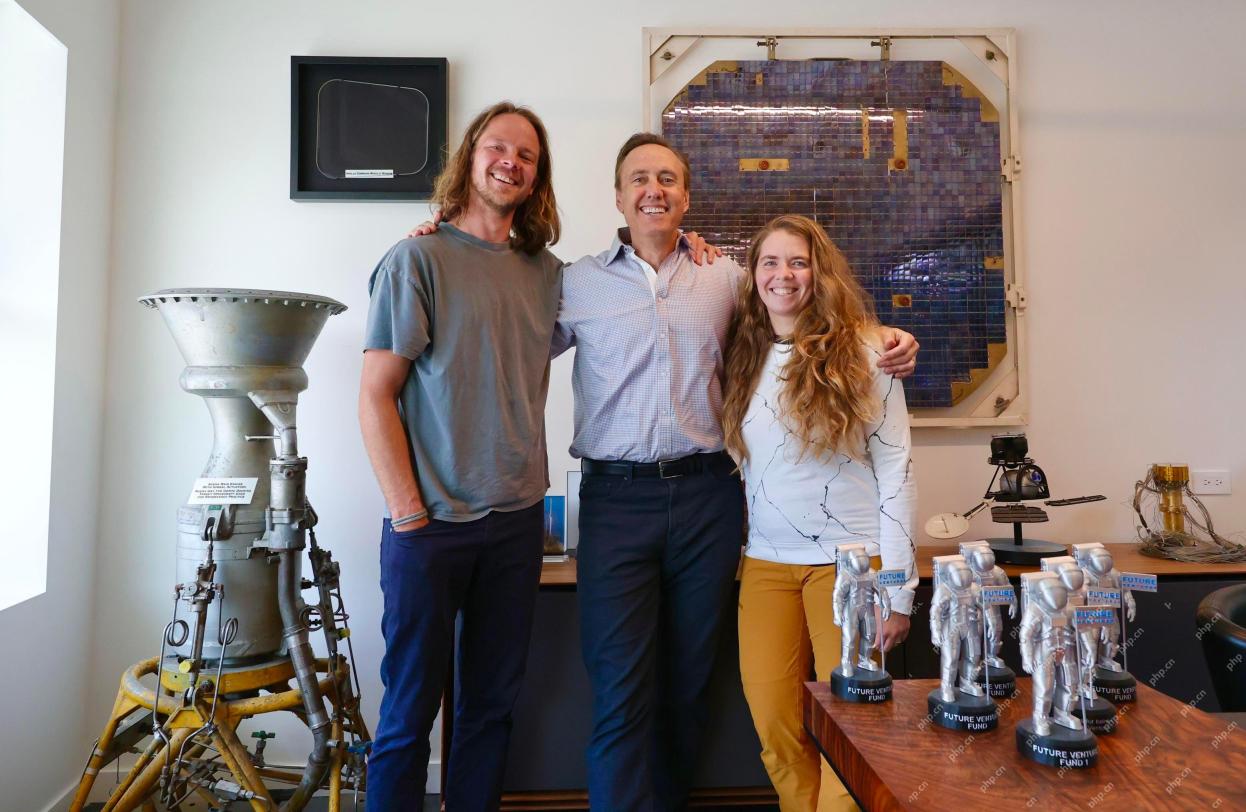
Future Ventures Fund IV: A $200M Bet on Novel Technologies Future Ventures recently closed its oversubscribed Fund IV, totaling $200 million. This new fund, managed by Steve Jurvetson, Maryanna Saenko, and Nico Enriquez, represents a significant inv
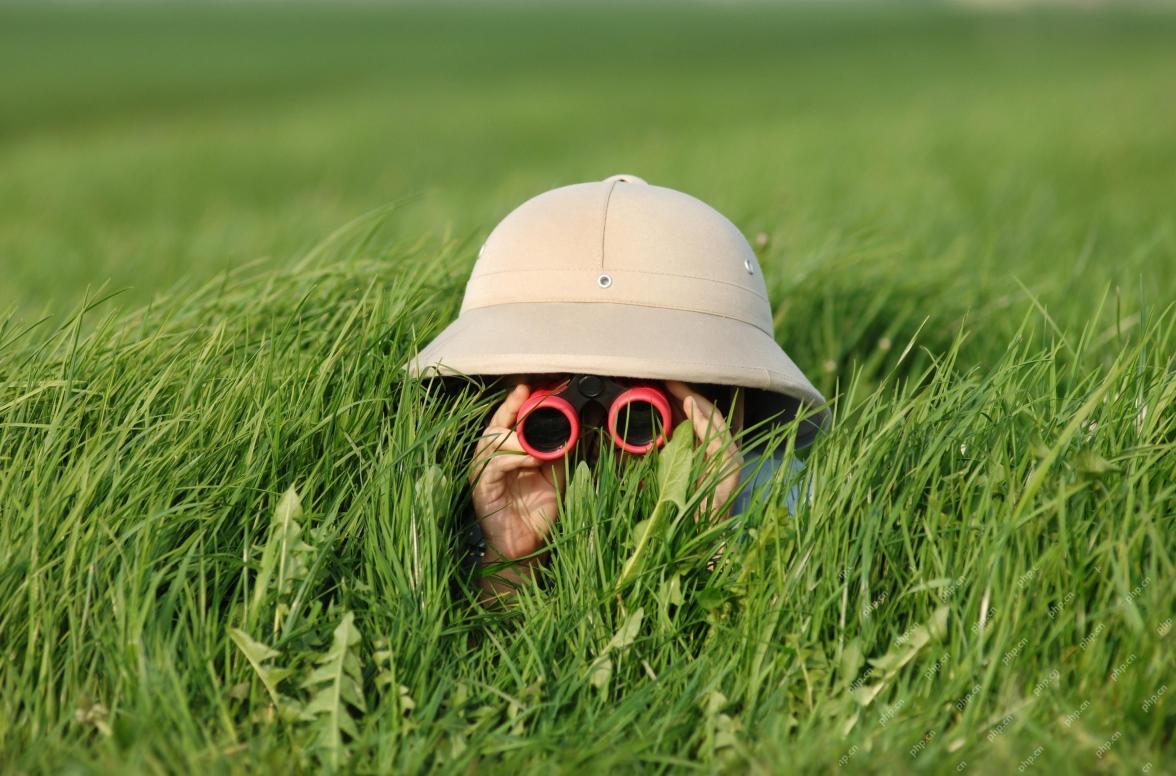
With the explosion of AI applications, enterprises are shifting from traditional search engine optimization (SEO) to generative engine optimization (GEO). Google is leading the shift. Its "AI Overview" feature has served over a billion users, providing full answers before users click on the link. [^2] Other participants are also rapidly rising. ChatGPT, Microsoft Copilot and Perplexity are creating a new “answer engine” category that completely bypasses traditional search results. If your business doesn't show up in these AI-generated answers, potential customers may never find you—even if you rank high in traditional search results. From SEO to GEO – What exactly does this mean? For decades
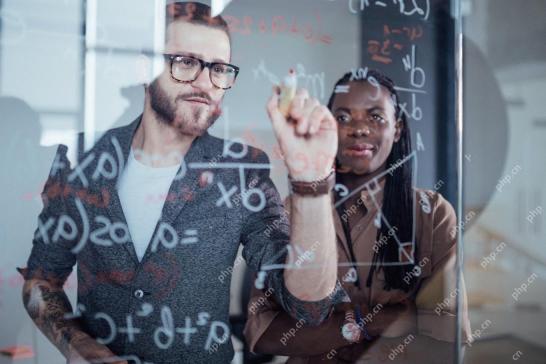
Let's explore the potential paths to Artificial General Intelligence (AGI). This analysis is part of my ongoing Forbes column on AI advancements, delving into the complexities of achieving AGI and Artificial Superintelligence (ASI). (See related art


Hot AI Tools

Undresser.AI Undress
AI-powered app for creating realistic nude photos

AI Clothes Remover
Online AI tool for removing clothes from photos.

Undress AI Tool
Undress images for free

Clothoff.io
AI clothes remover

Video Face Swap
Swap faces in any video effortlessly with our completely free AI face swap tool!

Hot Article

Hot Tools

SublimeText3 Linux new version
SublimeText3 Linux latest version
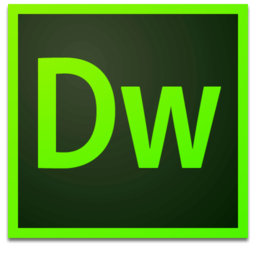
Dreamweaver Mac version
Visual web development tools
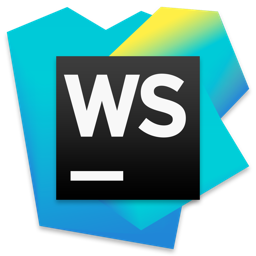
WebStorm Mac version
Useful JavaScript development tools

PhpStorm Mac version
The latest (2018.2.1) professional PHP integrated development tool

DVWA
Damn Vulnerable Web App (DVWA) is a PHP/MySQL web application that is very vulnerable. Its main goals are to be an aid for security professionals to test their skills and tools in a legal environment, to help web developers better understand the process of securing web applications, and to help teachers/students teach/learn in a classroom environment Web application security. The goal of DVWA is to practice some of the most common web vulnerabilities through a simple and straightforward interface, with varying degrees of difficulty. Please note that this software
