Advanced MongoDB Tutorial: Mastering Indexing & Query Optimization
MongoDB's advanced index and query optimization skills include: 1. Create single-field index optimization simple queries; 2. Use composite index optimization to optimize complex queries and sorting; 3. Use the explain() method to debug index usage; 4. Select the appropriate index type and regularly maintain index strategies to improve performance. Through these methods, the query efficiency of MongoDB can be significantly improved.
introduction
In the database world, MongoDB has become a leader in the field of NoSQL, especially when processing large-scale data, how to efficiently query and optimize has become a compulsory course for every developer. Today, we will dive into the advanced indexing and query optimization techniques of MongoDB. Through this article, you will learn how to use MongoDB's indexing mechanism to improve query performance, avoid common performance traps, and master some practical optimization strategies. Whether you are a beginner or an experienced developer, you can draw valuable knowledge from it.
Review of basic knowledge
MongoDB's index can be regarded as a tool to speed up data query, similar to directories in libraries, helping us quickly find the data we need. Indexing can not only improve query speed, but also reduce the burden on the server. Understanding index types in MongoDB, such as single-field index, composite index and text index, is the first step in mastering query optimization. At the same time, it is also essential to be familiar with MongoDB's query language (MQL) because it is the bridge for us to interact with the database.
Core concept or function analysis
Definition and function of index
Indexing plays a crucial role in MongoDB, allowing the database to quickly locate data when executing queries, rather than scanning the entire collection. Single-field indexes are the most basic index type, suitable for queries with a single field, while composite indexes can cover multiple fields, suitable for more complex query scenarios. The role of indexing is not only to accelerate querying, but also to improve the efficiency of data sorting and grouping operations.
Simple example:
// Create a single field index db.users.createIndex({ email: 1 }) // Create composite index db.users.createIndex({ email: 1, age: -1 })
How index works
When we execute a query, MongoDB first checks whether there are available indexes. If so, it uses the index to quickly locate the data. If not, it will do a full set scan, which is very inefficient for large data sets. The working principle of indexing can be simplified into a B-tree structure. MongoDB uses B-tree to organize index data, which makes the time complexity of search operations O(log n), greatly improving query efficiency.
Example of usage
Basic usage
In practical applications, creating indexes is the first step in optimizing query performance. Suppose we have a user collection, and the commonly used query is to find users based on the email address:
// Query user db.users.find({ email: "user@example.com" })
To optimize this query, we can create a single field index:
// Create mailbox index db.users.createIndex({ email: 1 })
This way, MongoDB can quickly find matching documents every time it is queryed.
Advanced Usage
Composite indexes are particularly important when dealing with complex queries. Suppose we often need to sort and query by email and age:
// Query and sort db.users.find({ email: "user@example.com" }).sort({ age: -1 })
To optimize this query, we can create a composite index:
// Create composite index db.users.createIndex({ email: 1, age: -1 })
In this way, MongoDB can leverage this index to meet the needs of query and sorting at the same time, significantly improving performance.
Common Errors and Debugging Tips
One of the common mistakes when using indexes is too many indexes. Too many indexes can increase the overhead of insertion and update operations, because every time the data changes, all relevant indexes need to be updated. Another common problem is that the index selection is improper, resulting in poor query performance. To debug these problems, you can use explain()
method to view the query plan:
// Check the query plan db.users.find({ email: "user@example.com" }).explain()
By analyzing the query plan, we can understand how MongoDB utilizes indexes and adjusts the index strategy according to actual situations.
Performance optimization and best practices
In practical applications, optimizing MongoDB's query performance requires comprehensive consideration of many factors. The first is to choose the appropriate index type. Single-field indexes are suitable for simple queries, while composite indexes are suitable for complex queries. The second is the maintenance of indexes. Regular inspection and adjustment of index strategies can avoid performance bottlenecks. Finally, query optimization, and the rational use of query operators, such as $in
, $or
, etc., can reduce query overhead.
In terms of performance optimization, it is very important to compare the effects of different index strategies. For example, suppose we have a collection of millions of records, and the commonly used queries are filtered by email and age:
// Unindexed query db.users.find({ email: "user@example.com", age: { $gt: 30 } }) // Add single field index db.users.createIndex({ email: 1 }) db.users.find({ email: "user@example.com", age: { $gt: 30 } }) // Add composite index db.users.createIndex({ email: 1, age: 1 }) db.users.find({ email: "user@example.com", age: { $gt: 30 } })
By comparing the performance of the three queries, we can find that the query speed is significantly improved after adding composite indexes. This is because composite indexes can cover the entire query condition, reducing MongoDB's scan range.
In terms of best practice, it is very important to keep the code readable and maintained. Reasonable naming and annotation can help team members quickly understand code intent, while regularly reviewing and optimizing index strategies can ensure long-term stability of the system.
In short, mastering MongoDB's advanced indexing and query optimization skills can not only improve system performance, but also add a touch of brightness to your career. I hope this article will inspire you and help you become an expert in the MongoDB field.
The above is the detailed content of Advanced MongoDB Tutorial: Mastering Indexing & Query Optimization. For more information, please follow other related articles on the PHP Chinese website!
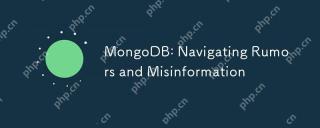
MongoDB supports relational data models, transaction processing and large-scale data processing. 1) MongoDB can handle relational data through nesting documents and $lookup operators. 2) Starting from version 4.0, MongoDB supports multi-document transactions, suitable for short-term operations. 3) Through sharding technology, MongoDB can process massive data, but it requires reasonable configuration.
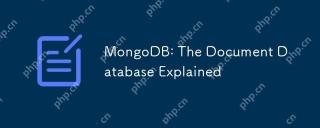
MongoDB is a NoSQL database that is suitable for handling large amounts of unstructured data. 1) It uses documents and collections to store data. Documents are similar to JSON objects and collections are similar to SQL tables. 2) MongoDB realizes efficient data operations through B-tree indexing and sharding. 3) Basic operations include connecting, inserting and querying documents; advanced operations such as aggregated pipelines can perform complex data processing. 4) Common errors include improper handling of ObjectId and improper use of indexes. 5) Performance optimization includes index optimization, sharding, read-write separation and data modeling.
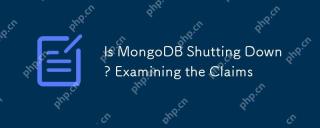
No,MongoDBisnotshuttingdown.Itcontinuestothrivewithsteadygrowth,anexpandinguserbase,andongoingdevelopment.Thecompany'ssuccesswithMongoDBAtlasanditsvibrantcommunityfurtherdemonstrateitsvitalityandfutureprospects.
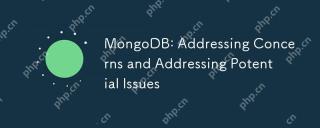
Common problems with MongoDB include data consistency, query performance, and security. The solutions are: 1) Use write and read attention mechanisms to ensure data consistency; 2) Optimize query performance through indexing, aggregation pipelines and sharding; 3) Use encryption, authentication and audit measures to improve security.
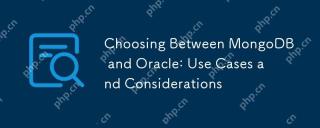
MongoDB is suitable for processing large-scale, unstructured data, and Oracle is suitable for scenarios that require strict data consistency and complex queries. 1.MongoDB provides flexibility and scalability, suitable for variable data structures. 2. Oracle provides strong transaction support and data consistency, suitable for enterprise-level applications. Data structure, scalability and performance requirements need to be considered when choosing.
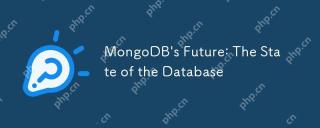
MongoDB's future is full of possibilities: 1. The development of cloud-native databases, 2. The fields of artificial intelligence and big data are focused, 3. The improvement of security and compliance. MongoDB continues to advance and make breakthroughs in technological innovation, market position and future development direction.
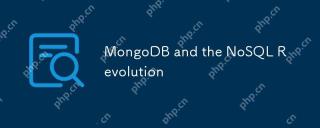
MongoDB is a document-based NoSQL database designed to provide high-performance, scalable and flexible data storage solutions. 1) It uses BSON format to store data, which is suitable for processing semi-structured or unstructured data. 2) Realize horizontal expansion through sharding technology and support complex queries and data processing. 3) Pay attention to index optimization, data modeling and performance monitoring when using it to give full play to its advantages.
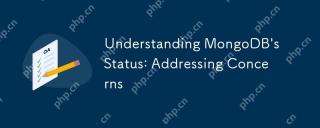
MongoDB is suitable for project needs, but it needs to be used optimized. 1) Performance: Optimize indexing strategies and use sharding technology. 2) Security: Enable authentication and data encryption. 3) Scalability: Use replica sets and sharding technologies.


Hot AI Tools

Undresser.AI Undress
AI-powered app for creating realistic nude photos

AI Clothes Remover
Online AI tool for removing clothes from photos.

Undress AI Tool
Undress images for free

Clothoff.io
AI clothes remover

Video Face Swap
Swap faces in any video effortlessly with our completely free AI face swap tool!

Hot Article

Hot Tools

PhpStorm Mac version
The latest (2018.2.1) professional PHP integrated development tool
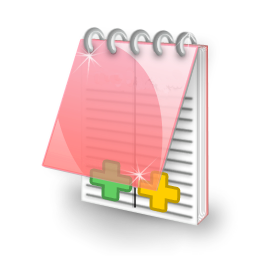
EditPlus Chinese cracked version
Small size, syntax highlighting, does not support code prompt function

Atom editor mac version download
The most popular open source editor
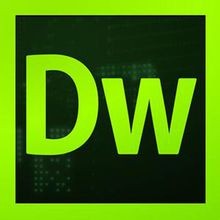
Dreamweaver CS6
Visual web development tools

MinGW - Minimalist GNU for Windows
This project is in the process of being migrated to osdn.net/projects/mingw, you can continue to follow us there. MinGW: A native Windows port of the GNU Compiler Collection (GCC), freely distributable import libraries and header files for building native Windows applications; includes extensions to the MSVC runtime to support C99 functionality. All MinGW software can run on 64-bit Windows platforms.
