


Explain the different sorting algorithms (e.g., bubble sort, insertion sort, merge sort, quicksort, heapsort). What are their time complexities?
Bubble Sort:
Bubble sort is a simple sorting algorithm that repeatedly steps through the list, compares adjacent elements, and swaps them if they are in the wrong order. The pass through the list is repeated until the list is sorted. The time complexity of bubble sort is O(n^2) in the average and worst cases, where n is the number of items being sorted. In the best case, where the list is already sorted, the time complexity is O(n).
Insertion Sort:
Insertion sort builds the final sorted array one item at a time. It is much less efficient on large lists than more advanced algorithms such as quicksort, heapsort, or merge sort. However, it performs well for small lists or nearly sorted lists. The time complexity of insertion sort is O(n^2) in the average and worst cases, and O(n) in the best case.
Merge Sort:
Merge sort is a divide-and-conquer algorithm that divides the unsorted list into n sublists, each containing one element (a list of one element is considered sorted), and repeatedly merges sublists to produce new sorted sublists until there is only one sublist remaining. The time complexity of merge sort is O(n log n) in all cases (best, average, and worst).
Quicksort:
Quicksort is also a divide-and-conquer algorithm that works by selecting a 'pivot' element from the array and partitioning the other elements into two sub-arrays, according to whether they are less than or greater than the pivot. The sub-arrays are then sorted recursively. The time complexity of quicksort is O(n log n) on average and in the best case, but it can degrade to O(n^2) in the worst case.
Heapsort:
Heapsort involves building a max-heap from the list, then repeatedly extracting the maximum element from the heap and placing it at the end of the sorted array. The time complexity of heapsort is O(n log n) in all cases (best, average, and worst).
Which sorting algorithm is most efficient for small datasets and why?
For small datasets, insertion sort is often the most efficient sorting algorithm. This is because insertion sort has a best-case time complexity of O(n), which occurs when the input is already sorted or nearly sorted. For small datasets, the overhead of more complex algorithms like quicksort or merge sort may outweigh their benefits, making insertion sort a good choice due to its simplicity and efficiency in these scenarios.
How does the choice of pivot affect the performance of quicksort?
The choice of pivot in quicksort significantly affects its performance. The pivot is used to partition the array into two sub-arrays, and the efficiency of this partitioning directly impacts the overall performance of the algorithm.
- Best Case: If the pivot chosen always divides the array into two equal halves, quicksort achieves its best-case time complexity of O(n log n). This happens when the pivot is the median of the array.
- Average Case: In practice, choosing a random pivot or the middle element often results in an average-case time complexity of O(n log n), as it tends to divide the array into roughly equal parts over multiple iterations.
- Worst Case: The worst-case scenario occurs when the pivot chosen is always the smallest or largest element in the array, leading to unbalanced partitions. This results in a time complexity of O(n^2). This can happen, for example, if the array is already sorted and the first or last element is chosen as the pivot.
Therefore, strategies like choosing a random pivot or using the median-of-three method (selecting the median of the first, middle, and last elements) can help mitigate the risk of encountering the worst-case scenario.
Can you recommend a sorting algorithm for large datasets and explain its advantages?
For large datasets, I recommend using mergesort. Mergesort has several advantages that make it suitable for sorting large datasets:
- Stable and Consistent Performance: Mergesort has a time complexity of O(n log n) in all cases (best, average, and worst), making its performance predictable and reliable regardless of the input data's initial order.
- Efficient Use of Memory: While mergesort does require additional memory for the merging process, it can be implemented in a way that minimizes memory usage, such as using an in-place merge or external sorting for extremely large datasets that do not fit in memory.
- Parallelization: Mergesort is well-suited for parallel processing, as the divide-and-conquer approach allows different parts of the array to be sorted independently before being merged. This can significantly speed up the sorting process on multi-core systems or distributed computing environments.
- Stability: Mergesort is a stable sorting algorithm, meaning that it preserves the relative order of equal elements. This can be important in applications where the order of equal elements matters.
Overall, the consistent O(n log n) time complexity, potential for parallelization, and stability make mergesort an excellent choice for sorting large datasets.
The above is the detailed content of Explain the different sorting algorithms (e.g., bubble sort, insertion sort, merge sort, quicksort, heapsort). What are their time complexities?. For more information, please follow other related articles on the PHP Chinese website!
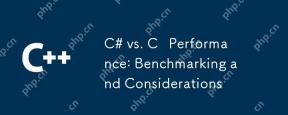
The performance differences between C# and C are mainly reflected in execution speed and resource management: 1) C usually performs better in numerical calculations and string operations because it is closer to hardware and has no additional overhead such as garbage collection; 2) C# is more concise in multi-threaded programming, but its performance is slightly inferior to C; 3) Which language to choose should be determined based on project requirements and team technology stack.
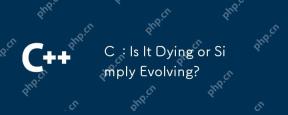
C isnotdying;it'sevolving.1)C remainsrelevantduetoitsversatilityandefficiencyinperformance-criticalapplications.2)Thelanguageiscontinuouslyupdated,withC 20introducingfeatureslikemodulesandcoroutinestoimproveusabilityandperformance.3)Despitechallen
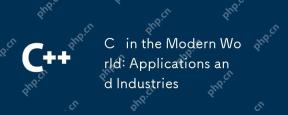
C is widely used and important in the modern world. 1) In game development, C is widely used for its high performance and polymorphism, such as UnrealEngine and Unity. 2) In financial trading systems, C's low latency and high throughput make it the first choice, suitable for high-frequency trading and real-time data analysis.
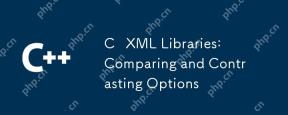
There are four commonly used XML libraries in C: TinyXML-2, PugiXML, Xerces-C, and RapidXML. 1.TinyXML-2 is suitable for environments with limited resources, lightweight but limited functions. 2. PugiXML is fast and supports XPath query, suitable for complex XML structures. 3.Xerces-C is powerful, supports DOM and SAX resolution, and is suitable for complex processing. 4. RapidXML focuses on performance and parses extremely fast, but does not support XPath queries.
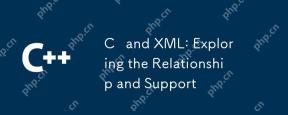
C interacts with XML through third-party libraries (such as TinyXML, Pugixml, Xerces-C). 1) Use the library to parse XML files and convert them into C-processable data structures. 2) When generating XML, convert the C data structure to XML format. 3) In practical applications, XML is often used for configuration files and data exchange to improve development efficiency.
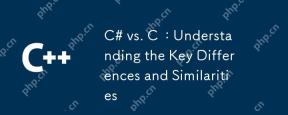
The main differences between C# and C are syntax, performance and application scenarios. 1) The C# syntax is more concise, supports garbage collection, and is suitable for .NET framework development. 2) C has higher performance and requires manual memory management, which is often used in system programming and game development.
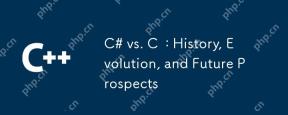
The history and evolution of C# and C are unique, and the future prospects are also different. 1.C was invented by BjarneStroustrup in 1983 to introduce object-oriented programming into the C language. Its evolution process includes multiple standardizations, such as C 11 introducing auto keywords and lambda expressions, C 20 introducing concepts and coroutines, and will focus on performance and system-level programming in the future. 2.C# was released by Microsoft in 2000. Combining the advantages of C and Java, its evolution focuses on simplicity and productivity. For example, C#2.0 introduced generics and C#5.0 introduced asynchronous programming, which will focus on developers' productivity and cloud computing in the future.
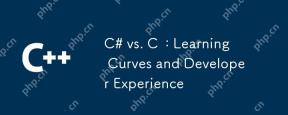
There are significant differences in the learning curves of C# and C and developer experience. 1) The learning curve of C# is relatively flat and is suitable for rapid development and enterprise-level applications. 2) The learning curve of C is steep and is suitable for high-performance and low-level control scenarios.


Hot AI Tools

Undresser.AI Undress
AI-powered app for creating realistic nude photos

AI Clothes Remover
Online AI tool for removing clothes from photos.

Undress AI Tool
Undress images for free

Clothoff.io
AI clothes remover

Video Face Swap
Swap faces in any video effortlessly with our completely free AI face swap tool!

Hot Article

Hot Tools

SublimeText3 Linux new version
SublimeText3 Linux latest version
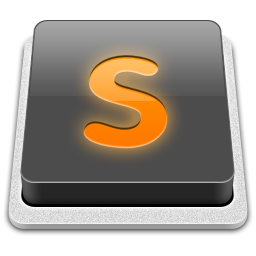
SublimeText3 Mac version
God-level code editing software (SublimeText3)

Atom editor mac version download
The most popular open source editor
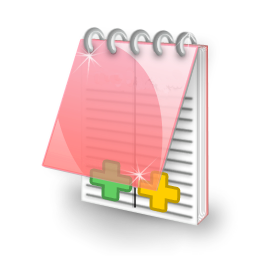
EditPlus Chinese cracked version
Small size, syntax highlighting, does not support code prompt function

Zend Studio 13.0.1
Powerful PHP integrated development environment
