


How do you profile Python code to identify performance bottlenecks?
To profile Python code and identify performance bottlenecks, you can use several approaches and tools. The general process involves:
-
Choosing a Profiler: Select an appropriate profiling tool based on your needs. Common tools include
cProfile
andline_profiler
. -
Running the Profiler: Execute your code with the profiler enabled. For example, using
cProfile
in the command line:<code>python -m cProfile -o output.prof your_script.py</code>
This command runs your script and outputs profiling data to
output.profile
. -
Analyzing the Output: Use tools to interpret the profiling data. For
cProfile
, you can usepstats
or external tools likesnakeviz
to visualize the results. - Identifying Bottlenecks: Look for functions or code sections that consume the most time. These are your performance bottlenecks.
- Optimization: Once identified, optimize the bottlenecks using techniques such as algorithmic improvements, caching, or using more efficient data structures.
Which Python profiling tools are most effective for pinpointing performance issues?
Several Python profiling tools are effective for pinpointing performance issues:
- cProfile: A built-in deterministic profiler in Python that provides detailed statistics about the time spent in various parts of the program. It's useful for identifying time-consuming functions but doesn't give line-by-line performance data.
- line_profiler: This tool extends profiling to a line-by-line basis, which is helpful for pinpointing exact locations of performance issues within functions. It can be particularly useful for optimizing loops and complex algorithms.
- memory_profiler: While primarily focused on memory usage, it can also help identify performance bottlenecks related to memory allocation and deallocation.
-
Yappi: A powerful profiler that supports multi-threading and can profile both CPU time and wall time. It's more advanced than
cProfile
and can provide more detailed insights in complex applications. -
Snakeviz: A browser-based graphical viewer for profiling data, often used in conjunction with
cProfile
. It makes it easier to visualize and understand profiling results.
Each of these tools has its strengths, and the most effective one will depend on the specific needs of your project, such as whether you're more concerned with time, memory, or both.
Can profiling help optimize memory usage in Python applications?
Yes, profiling can significantly help in optimizing memory usage in Python applications. While many profiling tools focus on execution time, specific tools like memory_profiler
are designed to monitor and analyze memory consumption.
-
Tracking Memory Usage:
memory_profiler
can track the memory consumption of your code line by line, helping you identify where memory is being allocated and deallocated. - Identifying Leaks: By analyzing memory usage over time, you can detect memory leaks, where memory is allocated but not freed, leading to increased memory usage over time.
- Optimizing Data Structures: Profiling can reveal inefficient use of data structures. For example, using lists when sets would be more memory-efficient can be identified through memory profiling.
- Improving Code Efficiency: Sometimes, performance bottlenecks are directly related to memory usage. Optimizing these sections can lead to better memory management.
By using memory profiling tools, you can gain insights into how your application uses memory and make informed decisions to optimize it, resulting in more efficient and scalable applications.
What are the best practices for interpreting profiling results to improve Python code efficiency?
Interpreting profiling results effectively is key to improving Python code efficiency. Here are some best practices:
- Focus on Hotspots: Identify the functions or code sections that consume the most time. These are your primary targets for optimization.
- Understand the Context: Consider the context in which the bottlenecks occur. Sometimes, improving the performance of a function requires understanding how it's used in the broader application.
-
Use Visual Aids: Tools like
Snakeviz
orKCacheGrind
can help visualize profiling data, making it easier to spot trends and patterns. - Iterative Optimization: Optimize incrementally. After making changes, profile again to see the impact of your optimizations. This iterative approach helps refine improvements and avoid over-optimization.
- Avoid Premature Optimization: Only optimize code that profiling has identified as a bottleneck. Premature optimization can lead to unnecessarily complex code with little performance gain.
- Consider the Trade-offs: Be aware of trade-offs between time and space complexity. Sometimes, increasing memory usage can lead to better performance, and vice versa.
- Document Findings and Changes: Keep a record of your profiling results and the optimizations you make. This documentation can be invaluable for future maintenance and optimization efforts.
By following these best practices, you can systematically improve your Python code's efficiency based on concrete profiling data.
The above is the detailed content of How do you profile Python code to identify performance bottlenecks?. For more information, please follow other related articles on the PHP Chinese website!
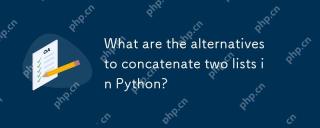
There are many methods to connect two lists in Python: 1. Use operators, which are simple but inefficient in large lists; 2. Use extend method, which is efficient but will modify the original list; 3. Use the = operator, which is both efficient and readable; 4. Use itertools.chain function, which is memory efficient but requires additional import; 5. Use list parsing, which is elegant but may be too complex. The selection method should be based on the code context and requirements.
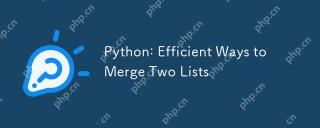
There are many ways to merge Python lists: 1. Use operators, which are simple but not memory efficient for large lists; 2. Use extend method, which is efficient but will modify the original list; 3. Use itertools.chain, which is suitable for large data sets; 4. Use * operator, merge small to medium-sized lists in one line of code; 5. Use numpy.concatenate, which is suitable for large data sets and scenarios with high performance requirements; 6. Use append method, which is suitable for small lists but is inefficient. When selecting a method, you need to consider the list size and application scenarios.
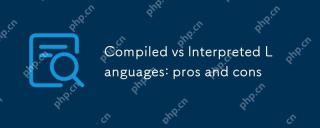
Compiledlanguagesofferspeedandsecurity,whileinterpretedlanguagesprovideeaseofuseandportability.1)CompiledlanguageslikeC arefasterandsecurebuthavelongerdevelopmentcyclesandplatformdependency.2)InterpretedlanguageslikePythonareeasiertouseandmoreportab
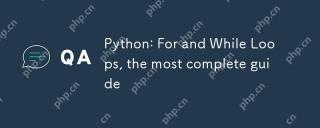
In Python, a for loop is used to traverse iterable objects, and a while loop is used to perform operations repeatedly when the condition is satisfied. 1) For loop example: traverse the list and print the elements. 2) While loop example: guess the number game until you guess it right. Mastering cycle principles and optimization techniques can improve code efficiency and reliability.
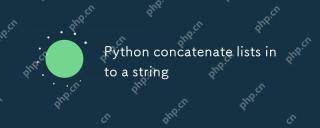
To concatenate a list into a string, using the join() method in Python is the best choice. 1) Use the join() method to concatenate the list elements into a string, such as ''.join(my_list). 2) For a list containing numbers, convert map(str, numbers) into a string before concatenating. 3) You can use generator expressions for complex formatting, such as ','.join(f'({fruit})'forfruitinfruits). 4) When processing mixed data types, use map(str, mixed_list) to ensure that all elements can be converted into strings. 5) For large lists, use ''.join(large_li
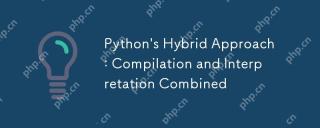
Pythonusesahybridapproach,combiningcompilationtobytecodeandinterpretation.1)Codeiscompiledtoplatform-independentbytecode.2)BytecodeisinterpretedbythePythonVirtualMachine,enhancingefficiencyandportability.
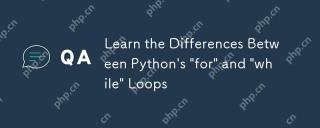
ThekeydifferencesbetweenPython's"for"and"while"loopsare:1)"For"loopsareidealforiteratingoversequencesorknowniterations,while2)"while"loopsarebetterforcontinuinguntilaconditionismetwithoutpredefinediterations.Un
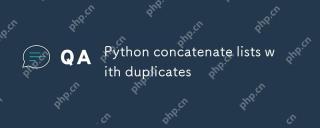
In Python, you can connect lists and manage duplicate elements through a variety of methods: 1) Use operators or extend() to retain all duplicate elements; 2) Convert to sets and then return to lists to remove all duplicate elements, but the original order will be lost; 3) Use loops or list comprehensions to combine sets to remove duplicate elements and maintain the original order.


Hot AI Tools

Undresser.AI Undress
AI-powered app for creating realistic nude photos

AI Clothes Remover
Online AI tool for removing clothes from photos.

Undress AI Tool
Undress images for free

Clothoff.io
AI clothes remover

Video Face Swap
Swap faces in any video effortlessly with our completely free AI face swap tool!

Hot Article

Hot Tools

DVWA
Damn Vulnerable Web App (DVWA) is a PHP/MySQL web application that is very vulnerable. Its main goals are to be an aid for security professionals to test their skills and tools in a legal environment, to help web developers better understand the process of securing web applications, and to help teachers/students teach/learn in a classroom environment Web application security. The goal of DVWA is to practice some of the most common web vulnerabilities through a simple and straightforward interface, with varying degrees of difficulty. Please note that this software

Atom editor mac version download
The most popular open source editor
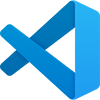
VSCode Windows 64-bit Download
A free and powerful IDE editor launched by Microsoft
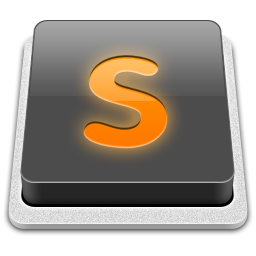
SublimeText3 Mac version
God-level code editing software (SublimeText3)
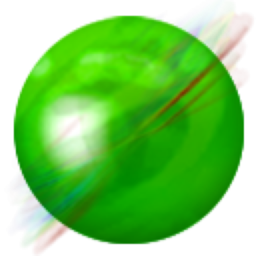
ZendStudio 13.5.1 Mac
Powerful PHP integrated development environment
