What is pickling and unpickling in Python?
Pickling and unpickling are processes in Python used for serializing and deserializing objects, respectively. Serialization is the process of converting an object into a byte stream, which can be stored in a file or transmitted over a network. This byte stream can later be deserialized, or unpickled, to reconstruct the original object.
In Python, the pickle
module is used for these operations. Pickling converts Python objects into a binary format that can be stored or transmitted, and unpickling retrieves the original object from this binary format. This is useful for persisting objects or sending complex data structures between different parts of a program or different machines.
The pickle
module supports most Python data types, including custom class instances, but it is specific to Python and may not be compatible with other programming languages.
How can I use pickling to save Python objects?
To use pickling to save Python objects, you can follow these steps:
-
Import the
pickle
module:import pickle
-
Create or obtain the object you want to pickle:
For example, a list or a dictionary:data = {'key': 'value', 'number': 42}
-
Open a file in binary write mode:
with open('data.pickle', 'wb') as file: # Use pickle.dump to serialize the object to the file pickle.dump(data, file)
In this example,
data.pickle
is the file where the serialized data will be saved. -
To unpickle and retrieve the object, open the file in binary read mode:
with open('data.pickle', 'rb') as file: # Use pickle.load to deserialize the object from the file loaded_data = pickle.load(file)
Now,
loaded_data
will contain the original object.
Here's a complete example demonstrating pickling and unpickling:
import pickle # Object to be pickled data = {'key': 'value', 'number': 42} # Pickling with open('data.pickle', 'wb') as file: pickle.dump(data, file) # Unpickling with open('data.pickle', 'rb') as file: loaded_data = pickle.load(file) print(loaded_data) # Output: {'key': 'value', 'number': 42}
What are the security considerations when unpickling data in Python?
Unpickling data in Python can pose significant security risks if the data comes from an untrusted source. Here are some key considerations:
-
Arbitrary Code Execution:
Thepickle
module can execute arbitrary Python code during unpickling. If an attacker manipulates the pickled data, they can inject malicious code that will be executed when the data is unpickled. This is particularly dangerous in networked applications where the data might be received from an untrusted source. -
Data Validation:
Always validate the source and integrity of the pickled data before unpickling. If the data is not from a trusted source, it should not be unpickled. -
Use of Safer Alternatives:
Consider using safer serialization formats like JSON or MessagePack, which do not allow arbitrary code execution. Thejson
module in Python is a secure alternative for serializing basic data types. -
Access Controls:
If unpickling cannot be avoided, ensure that the application runs with minimal privileges and uses strict access controls to limit the potential damage from malicious code. -
Error Handling:
Implement robust error handling to catch and handle any exceptions that occur during unpickling, which might indicate an attempt to execute malicious code.
Here’s an example of how you might safely handle unpickling:
import pickle def safe_unpickle(file_path): try: with open(file_path, 'rb') as file: data = pickle.load(file) # Validate data here if necessary return data except (pickle.UnpicklingError, EOFError, ImportError, AttributeError) as e: print(f"Error unpickling: {e}") return None # Use the function loaded_data = safe_unpickle('data.pickle') if loaded_data is not None: print(loaded_data)
By following these security considerations, you can mitigate the risks associated with unpickling data in Python.
The above is the detailed content of What is pickling and unpickling in Python?. For more information, please follow other related articles on the PHP Chinese website!
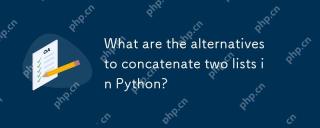
There are many methods to connect two lists in Python: 1. Use operators, which are simple but inefficient in large lists; 2. Use extend method, which is efficient but will modify the original list; 3. Use the = operator, which is both efficient and readable; 4. Use itertools.chain function, which is memory efficient but requires additional import; 5. Use list parsing, which is elegant but may be too complex. The selection method should be based on the code context and requirements.
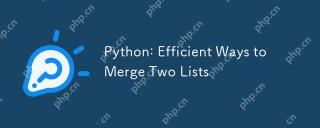
There are many ways to merge Python lists: 1. Use operators, which are simple but not memory efficient for large lists; 2. Use extend method, which is efficient but will modify the original list; 3. Use itertools.chain, which is suitable for large data sets; 4. Use * operator, merge small to medium-sized lists in one line of code; 5. Use numpy.concatenate, which is suitable for large data sets and scenarios with high performance requirements; 6. Use append method, which is suitable for small lists but is inefficient. When selecting a method, you need to consider the list size and application scenarios.
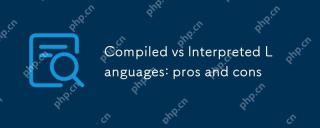
Compiledlanguagesofferspeedandsecurity,whileinterpretedlanguagesprovideeaseofuseandportability.1)CompiledlanguageslikeC arefasterandsecurebuthavelongerdevelopmentcyclesandplatformdependency.2)InterpretedlanguageslikePythonareeasiertouseandmoreportab
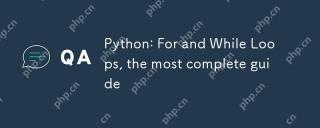
In Python, a for loop is used to traverse iterable objects, and a while loop is used to perform operations repeatedly when the condition is satisfied. 1) For loop example: traverse the list and print the elements. 2) While loop example: guess the number game until you guess it right. Mastering cycle principles and optimization techniques can improve code efficiency and reliability.
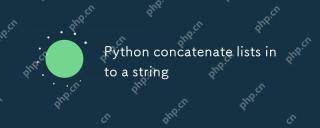
To concatenate a list into a string, using the join() method in Python is the best choice. 1) Use the join() method to concatenate the list elements into a string, such as ''.join(my_list). 2) For a list containing numbers, convert map(str, numbers) into a string before concatenating. 3) You can use generator expressions for complex formatting, such as ','.join(f'({fruit})'forfruitinfruits). 4) When processing mixed data types, use map(str, mixed_list) to ensure that all elements can be converted into strings. 5) For large lists, use ''.join(large_li
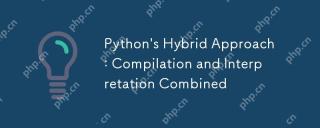
Pythonusesahybridapproach,combiningcompilationtobytecodeandinterpretation.1)Codeiscompiledtoplatform-independentbytecode.2)BytecodeisinterpretedbythePythonVirtualMachine,enhancingefficiencyandportability.
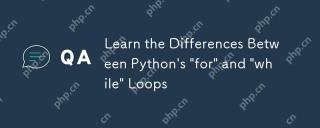
ThekeydifferencesbetweenPython's"for"and"while"loopsare:1)"For"loopsareidealforiteratingoversequencesorknowniterations,while2)"while"loopsarebetterforcontinuinguntilaconditionismetwithoutpredefinediterations.Un
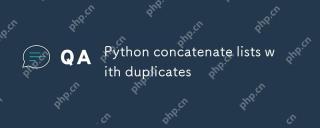
In Python, you can connect lists and manage duplicate elements through a variety of methods: 1) Use operators or extend() to retain all duplicate elements; 2) Convert to sets and then return to lists to remove all duplicate elements, but the original order will be lost; 3) Use loops or list comprehensions to combine sets to remove duplicate elements and maintain the original order.


Hot AI Tools

Undresser.AI Undress
AI-powered app for creating realistic nude photos

AI Clothes Remover
Online AI tool for removing clothes from photos.

Undress AI Tool
Undress images for free

Clothoff.io
AI clothes remover

Video Face Swap
Swap faces in any video effortlessly with our completely free AI face swap tool!

Hot Article

Hot Tools

Safe Exam Browser
Safe Exam Browser is a secure browser environment for taking online exams securely. This software turns any computer into a secure workstation. It controls access to any utility and prevents students from using unauthorized resources.
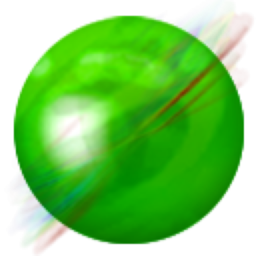
ZendStudio 13.5.1 Mac
Powerful PHP integrated development environment

SecLists
SecLists is the ultimate security tester's companion. It is a collection of various types of lists that are frequently used during security assessments, all in one place. SecLists helps make security testing more efficient and productive by conveniently providing all the lists a security tester might need. List types include usernames, passwords, URLs, fuzzing payloads, sensitive data patterns, web shells, and more. The tester can simply pull this repository onto a new test machine and he will have access to every type of list he needs.

PhpStorm Mac version
The latest (2018.2.1) professional PHP integrated development tool

MinGW - Minimalist GNU for Windows
This project is in the process of being migrated to osdn.net/projects/mingw, you can continue to follow us there. MinGW: A native Windows port of the GNU Compiler Collection (GCC), freely distributable import libraries and header files for building native Windows applications; includes extensions to the MSVC runtime to support C99 functionality. All MinGW software can run on 64-bit Windows platforms.
