This blog post explores efficient memory management techniques for loading large PyTorch models, especially beneficial when dealing with limited GPU or CPU resources. The author focuses on scenarios where models are saved using torch.save(model.state_dict(), "model.pth")
. While the examples use a large language model (LLM), the techniques are applicable to any PyTorch model.
Key Strategies for Efficient Model Loading:
The article details several methods to optimize memory usage during model loading:
-
Sequential Weight Loading: This technique loads the model architecture onto the GPU and then iteratively copies individual weights from CPU memory to the GPU. This prevents the simultaneous presence of both the full model and weights in GPU memory, significantly reducing peak memory consumption.
-
Meta Device: PyTorch's "meta" device enables tensor creation without immediate memory allocation. The model is initialized on the meta device, then transferred to the GPU, and weights are loaded directly onto the GPU, minimizing CPU memory usage. This is particularly useful for systems with limited CPU RAM.
-
mmap=True
intorch.load()
: This option uses memory-mapped file I/O, allowing PyTorch to read model data directly from disk on demand, rather than loading everything into RAM. This is ideal for systems with limited CPU memory and fast disk I/O. -
Individual Weight Saving and Loading: As a last resort for extremely limited resources, the article suggests saving each model parameter (tensor) as a separate file. Loading then occurs one parameter at a time, minimizing the memory footprint at any given moment. This comes at the cost of increased I/O overhead.
Practical Implementation and Benchmarking:
The post provides Python code snippets demonstrating each technique, including utility functions for tracking GPU and CPU memory usage. These benchmarks illustrate the memory savings achieved by each method. The author compares the memory usage of each approach, highlighting the trade-offs between memory efficiency and potential performance impacts.
Conclusion:
The article concludes by emphasizing the importance of memory-efficient model loading, especially for large models. It recommends selecting the most appropriate technique based on the specific hardware limitations (CPU RAM, GPU VRAM) and I/O speeds. The mmap=True
approach is generally preferred for limited CPU RAM, while individual weight loading is a last resort for extremely constrained environments. The sequential loading method offers a good balance for many scenarios.
The above is the detailed content of Memory-Efficient Model Weight Loading in PyTorch. For more information, please follow other related articles on the PHP Chinese website!
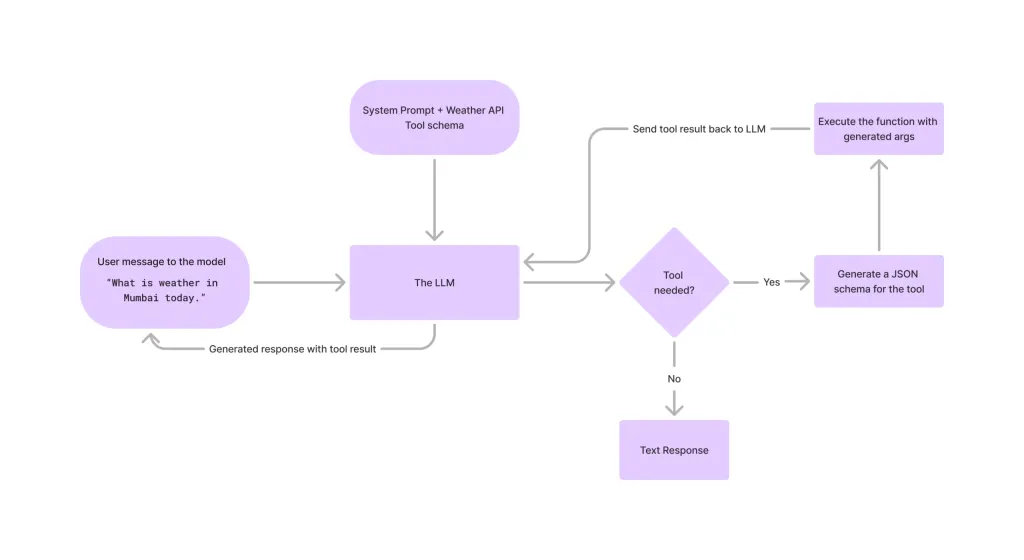
Large language models (LLMs) have surged in popularity, with the tool-calling feature dramatically expanding their capabilities beyond simple text generation. Now, LLMs can handle complex automation tasks such as dynamic UI creation and autonomous a
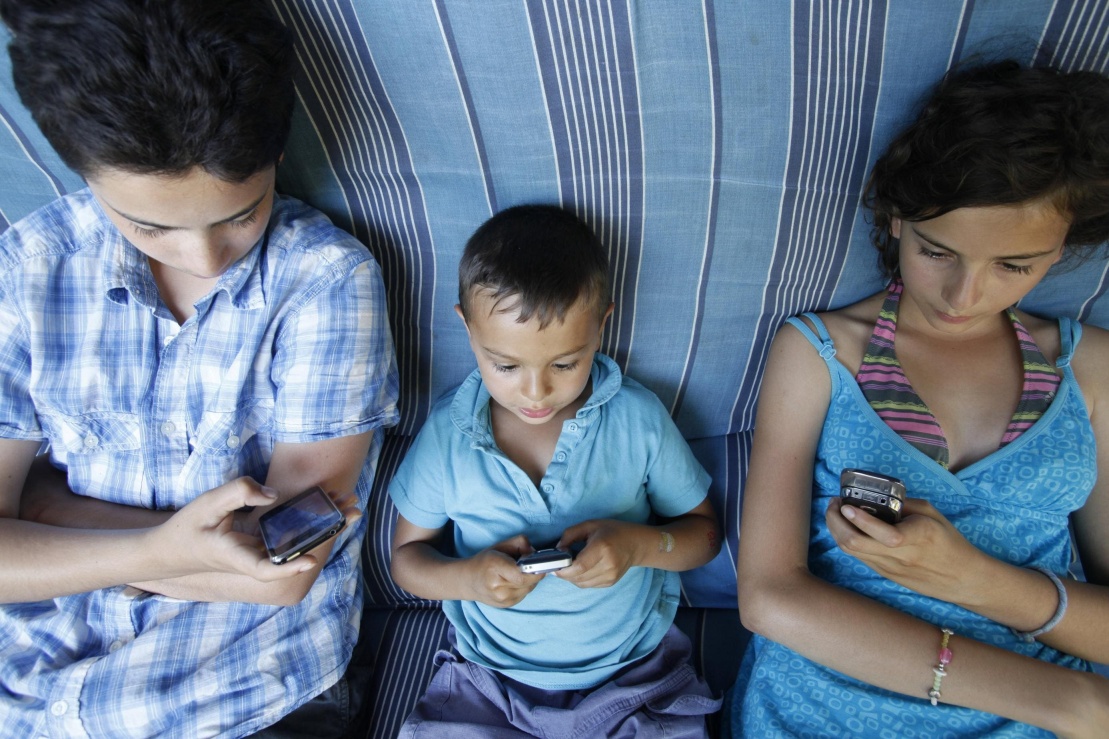
Can a video game ease anxiety, build focus, or support a child with ADHD? As healthcare challenges surge globally — especially among youth — innovators are turning to an unlikely tool: video games. Now one of the world’s largest entertainment indus
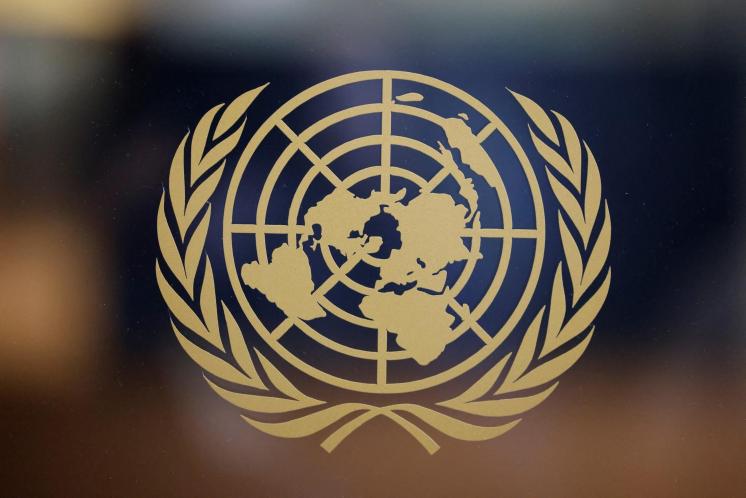
“History has shown that while technological progress drives economic growth, it does not on its own ensure equitable income distribution or promote inclusive human development,” writes Rebeca Grynspan, Secretary-General of UNCTAD, in the preamble.
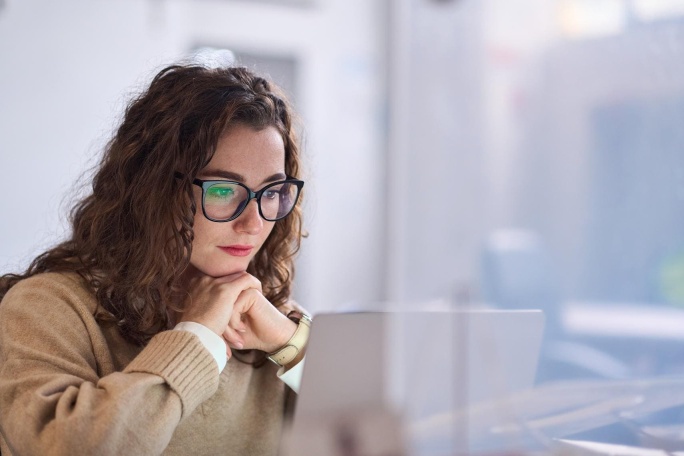
Easy-peasy, use generative AI as your negotiation tutor and sparring partner. Let’s talk about it. This analysis of an innovative AI breakthrough is part of my ongoing Forbes column coverage on the latest in AI, including identifying and explaining
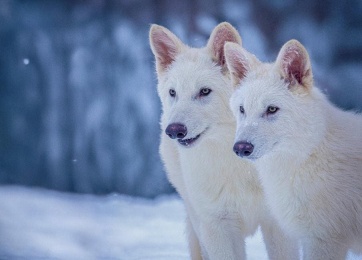
The TED2025 Conference, held in Vancouver, wrapped its 36th edition yesterday, April 11. It featured 80 speakers from more than 60 countries, including Sam Altman, Eric Schmidt, and Palmer Luckey. TED’s theme, “humanity reimagined,” was tailor made
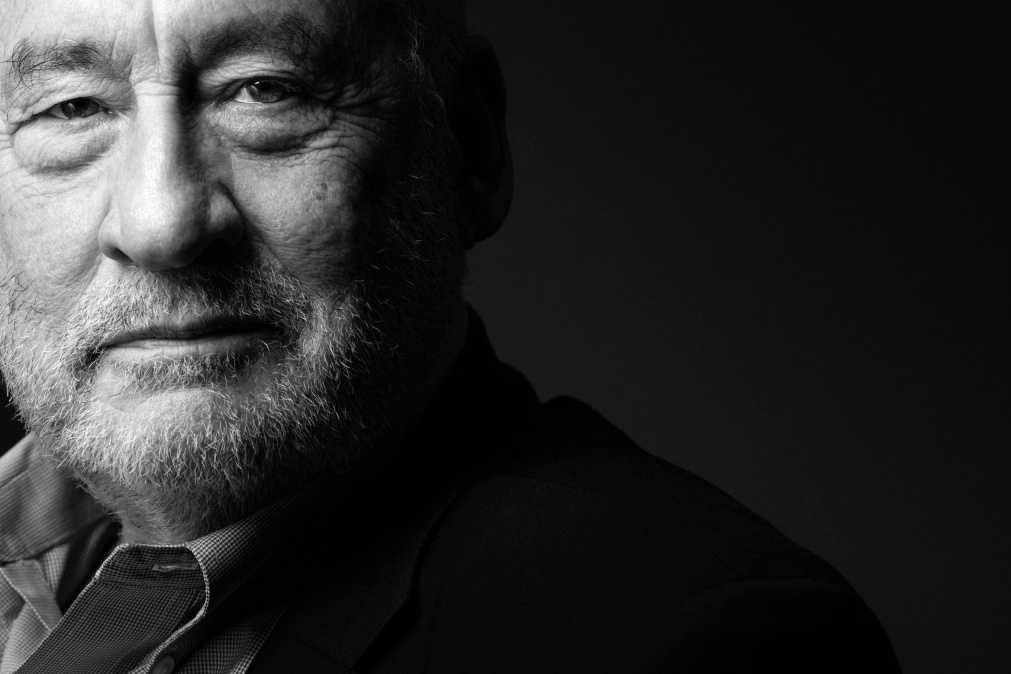
Joseph Stiglitz is renowned economist and recipient of the Nobel Prize in Economics in 2001. Stiglitz posits that AI can worsen existing inequalities and consolidated power in the hands of a few dominant corporations, ultimately undermining economic
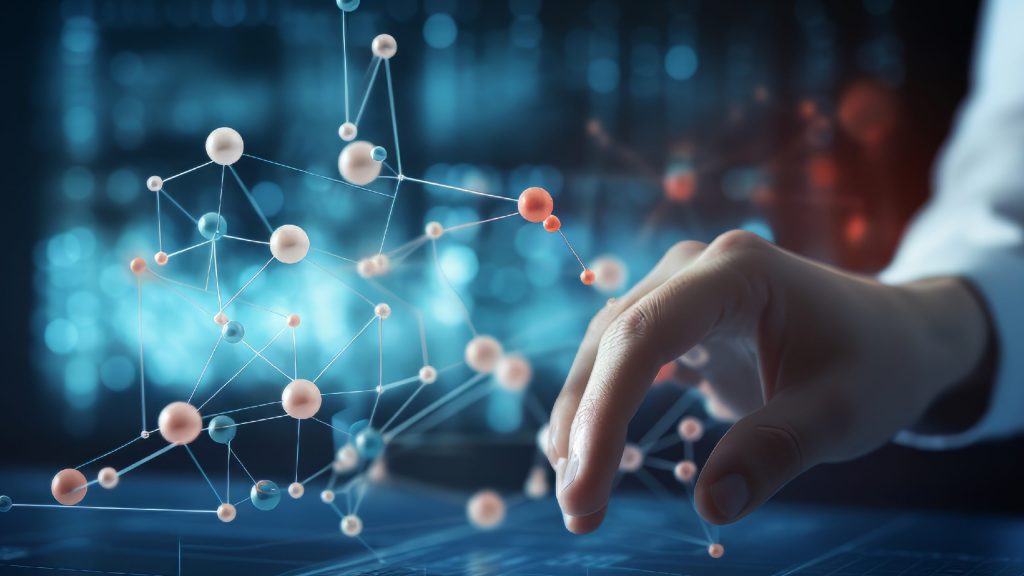
Graph Databases: Revolutionizing Data Management Through Relationships As data expands and its characteristics evolve across various fields, graph databases are emerging as transformative solutions for managing interconnected data. Unlike traditional
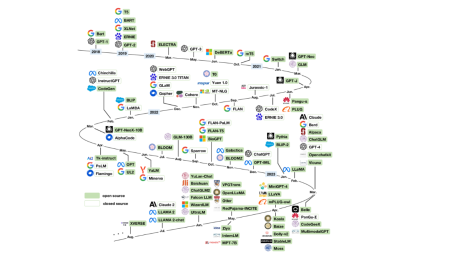
Large Language Model (LLM) Routing: Optimizing Performance Through Intelligent Task Distribution The rapidly evolving landscape of LLMs presents a diverse range of models, each with unique strengths and weaknesses. Some excel at creative content gen


Hot AI Tools

Undresser.AI Undress
AI-powered app for creating realistic nude photos

AI Clothes Remover
Online AI tool for removing clothes from photos.

Undress AI Tool
Undress images for free

Clothoff.io
AI clothes remover

AI Hentai Generator
Generate AI Hentai for free.

Hot Article

Hot Tools
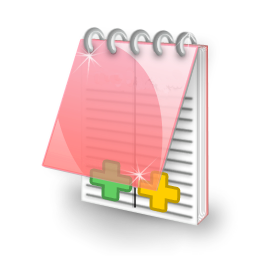
EditPlus Chinese cracked version
Small size, syntax highlighting, does not support code prompt function
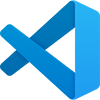
VSCode Windows 64-bit Download
A free and powerful IDE editor launched by Microsoft

MinGW - Minimalist GNU for Windows
This project is in the process of being migrated to osdn.net/projects/mingw, you can continue to follow us there. MinGW: A native Windows port of the GNU Compiler Collection (GCC), freely distributable import libraries and header files for building native Windows applications; includes extensions to the MSVC runtime to support C99 functionality. All MinGW software can run on 64-bit Windows platforms.

SublimeText3 Linux new version
SublimeText3 Linux latest version

DVWA
Damn Vulnerable Web App (DVWA) is a PHP/MySQL web application that is very vulnerable. Its main goals are to be an aid for security professionals to test their skills and tools in a legal environment, to help web developers better understand the process of securing web applications, and to help teachers/students teach/learn in a classroom environment Web application security. The goal of DVWA is to practice some of the most common web vulnerabilities through a simple and straightforward interface, with varying degrees of difficulty. Please note that this software