This article explores the evolution of AI models, focusing on the transition from traditional LLMs to Retrieval-Augmented Generation (RAG) and finally, Agentic RAG. It highlights the limitations of traditional LLMs in performing real-world actions and the advancements offered by RAG and Agentic RAG in addressing these limitations.
Key advancements covered:
-
From LLMs to RAG: The article details how RAG enhances LLMs by integrating external knowledge bases, leading to more accurate and contextually rich responses. It explains the process of query management, information retrieval, and response generation within a RAG system.
-
The emergence of Agentic RAG: Agentic RAG builds upon RAG by adding an autonomous decision-making layer. This allows the system to not only retrieve information but also strategically select and utilize appropriate tools to optimize responses and perform complex tasks.
-
Improvements in RAG technology: Recent advancements like improved retrieval algorithms, semantic caching, and multimodal integration are discussed, showcasing the ongoing development in this field.
-
Comparing RAG and AI Agents: A clear comparison highlights the key differences between RAG (focused on knowledge augmentation) and AI Agents (focused on action and interaction).
-
Architectural differences: A table provides a concise comparison of the architectures of Long Context LLMs, RAG, and Agentic RAG, emphasizing their distinct components and capabilities. The article explains the benefits of Long Context LLMs in handling extensive text, while highlighting RAG's cost-effectiveness.
- Self-Route: A Hybrid Approach: The article introduces Self-Route, a hybrid system that combines RAG and Long Context LLMs to achieve a balance between cost and performance. It dynamically routes queries to either RAG or the Long Context LLM based on complexity. This offers a practical solution for diverse query types.
The article concludes by summarizing the key differences and use cases for each type of model, emphasizing that the optimal choice depends on specific application needs and resource constraints. A FAQ section further clarifies key concepts.
The above is the detailed content of Evolution of RAG, Long Context LLMs to Agentic RAG - Analytics Vidhya. For more information, please follow other related articles on the PHP Chinese website!
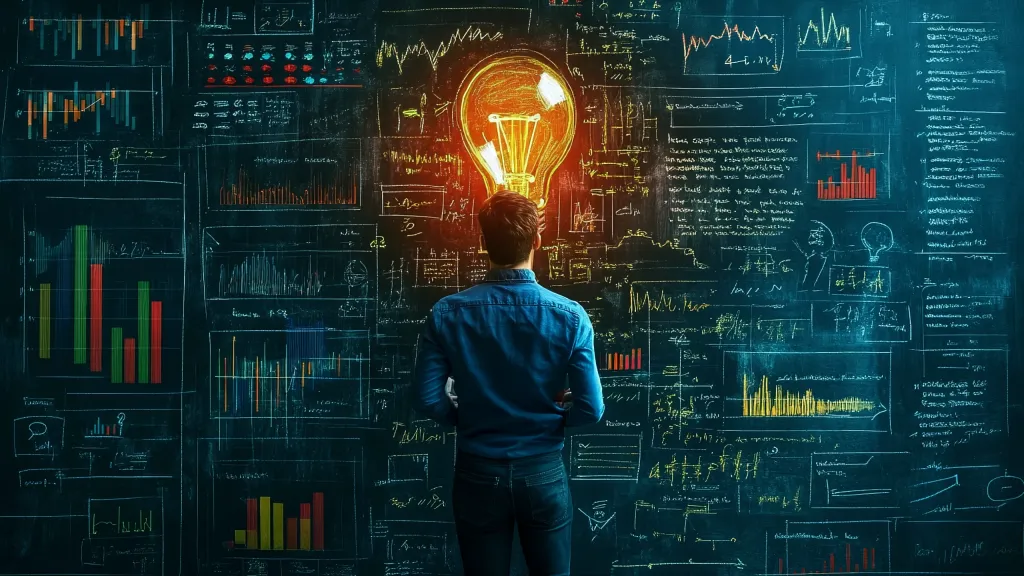
Introduction In prompt engineering, “Graph of Thought” refers to a novel approach that uses graph theory to structure and guide AI’s reasoning process. Unlike traditional methods, which often involve linear s
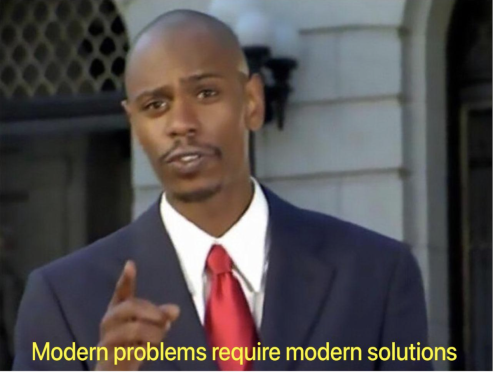
Introduction Congratulations! You run a successful business. Through your web pages, social media campaigns, webinars, conferences, free resources, and other sources, you collect 5000 email IDs daily. The next obvious step is
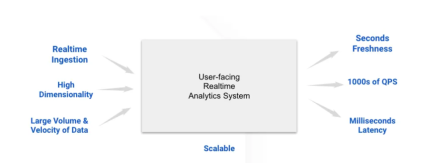
Introduction In today’s fast-paced software development environment, ensuring optimal application performance is crucial. Monitoring real-time metrics such as response times, error rates, and resource utilization can help main
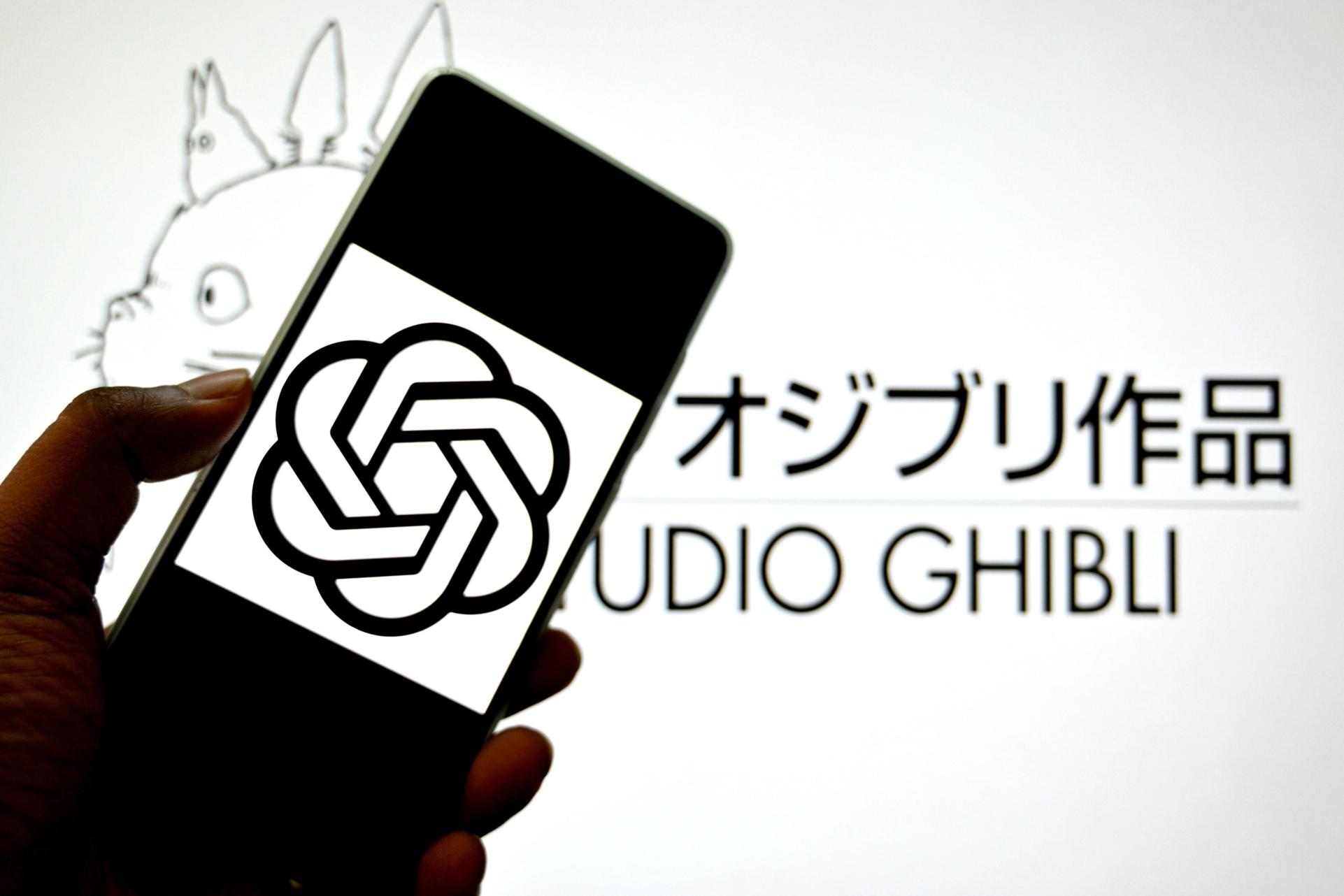
“How many users do you have?” he prodded. “I think the last time we said was 500 million weekly actives, and it is growing very rapidly,” replied Altman. “You told me that it like doubled in just a few weeks,” Anderson continued. “I said that priv
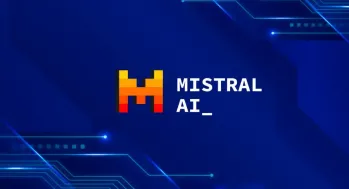
Introduction Mistral has released its very first multimodal model, namely the Pixtral-12B-2409. This model is built upon Mistral’s 12 Billion parameter, Nemo 12B. What sets this model apart? It can now take both images and tex
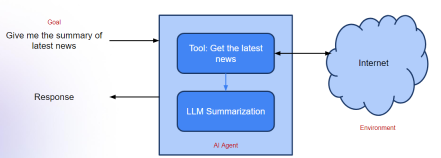
Imagine having an AI-powered assistant that not only responds to your queries but also autonomously gathers information, executes tasks, and even handles multiple types of data—text, images, and code. Sounds futuristic? In this a
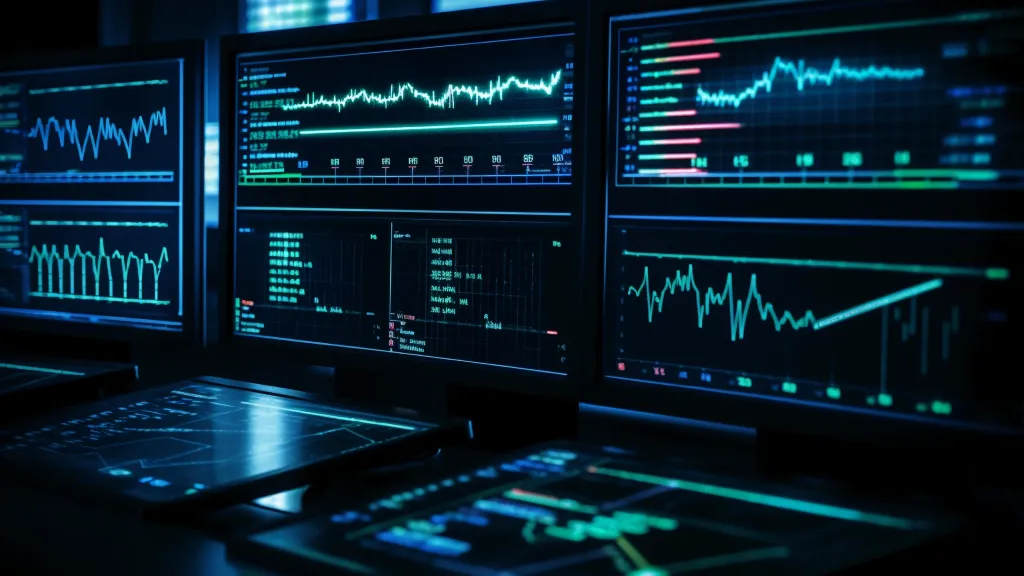
Introduction The finance industry is the cornerstone of any country’s development, as it drives economic growth by facilitating efficient transactions and credit availability. The ease with which transactions occur and credit

Introduction Data is being generated at an unprecedented rate from sources such as social media, financial transactions, and e-commerce platforms. Handling this continuous stream of information is a challenge, but it offers an


Hot AI Tools

Undresser.AI Undress
AI-powered app for creating realistic nude photos

AI Clothes Remover
Online AI tool for removing clothes from photos.

Undress AI Tool
Undress images for free

Clothoff.io
AI clothes remover

AI Hentai Generator
Generate AI Hentai for free.

Hot Article

Hot Tools

SublimeText3 Chinese version
Chinese version, very easy to use

mPDF
mPDF is a PHP library that can generate PDF files from UTF-8 encoded HTML. The original author, Ian Back, wrote mPDF to output PDF files "on the fly" from his website and handle different languages. It is slower than original scripts like HTML2FPDF and produces larger files when using Unicode fonts, but supports CSS styles etc. and has a lot of enhancements. Supports almost all languages, including RTL (Arabic and Hebrew) and CJK (Chinese, Japanese and Korean). Supports nested block-level elements (such as P, DIV),

DVWA
Damn Vulnerable Web App (DVWA) is a PHP/MySQL web application that is very vulnerable. Its main goals are to be an aid for security professionals to test their skills and tools in a legal environment, to help web developers better understand the process of securing web applications, and to help teachers/students teach/learn in a classroom environment Web application security. The goal of DVWA is to practice some of the most common web vulnerabilities through a simple and straightforward interface, with varying degrees of difficulty. Please note that this software
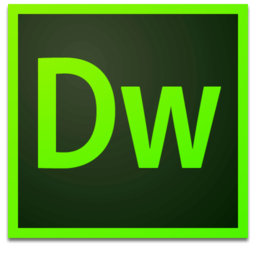
Dreamweaver Mac version
Visual web development tools

SecLists
SecLists is the ultimate security tester's companion. It is a collection of various types of lists that are frequently used during security assessments, all in one place. SecLists helps make security testing more efficient and productive by conveniently providing all the lists a security tester might need. List types include usernames, passwords, URLs, fuzzing payloads, sensitive data patterns, web shells, and more. The tester can simply pull this repository onto a new test machine and he will have access to every type of list he needs.