LangChain Retrieval: Efficient and Flexible Access to Documents
The searcher in the LangChain framework plays a crucial role, providing a flexible interface for returning documents based on unstructured queries. Unlike vector databases, the searcher does not need to store documents; its main function is to retrieve relevant information from a large amount of data. While vector databases can serve as the basis for retriers, there are various types of retriers, each customizable for a specific use case.
Learning Objectives
- Understand the key role of searchers in LangChain and achieve efficient and flexible document retrieval to meet various application needs.
- Learn how LangChain's searcher (from vector databases to multi-queries and context compression) simplifies access to relevant information.
- This guide covers various searcher types in LangChain and explains how each searcher is customized to optimize query processing and data access.
- Dig into LangChain's searcher capabilities and check out tools for enhancing document retrieval accuracy and relevance.
- Learn how LangChain's custom retriever adapts to specific needs, enabling developers to create highly responsive applications.
- Explore LangChain's search techniques that integrate language models and vector databases to obtain more accurate and efficient search results.
Table of contents
- Learning Objectives
- Searcher in LangChain
- Use vector database as a searcher
- Using MultiQueryRetriever
- Build a sample vector database
- Simple usage
- Custom Tips
- How to search using context compression
- Overview of context compression
- Create a custom searcher
- interface
- Example
- in conclusion
- Frequently Asked Questions
Searcher in LangChain
The searcher receives a string query as input and outputs a list of Document objects. This mechanism allows applications to efficiently acquire relevant information, enabling advanced interactions with large data sets or knowledge bases.
- Use vector database as a searcher
The vector database retriever efficiently retrieves documents by utilizing vector representations. It acts as a lightweight wrapper for vector storage classes, conforms to the searcher interface and uses methods such as similarity search and maximum marginal correlation (MMR).
To create a retriever from a vector database, use the .as_retriever
method. For example, for a Pinecone vector database based on customer reviews, we can set it up as follows:
from langchain_community.document_loaders import CSVLoader from langchain_community.vectorstores import Pinecone from langchain_openai import OpenAIEmbeddings from langchain_text_splitters import CharacterTextSplitter loader = CSVLoader("customer_reviews.csv") documents = loader.load() text_splitter = CharacterTextSplitter(chunk_size=500, chunk_overlap=50) texts = text_splitter.split_documents(documents) embeddings = OpenAIEmbeddings() vectorstore = Pinecone.from_documents(texts, embeddeds) retriever = vectorstore.as_retriever()
We can now use this searcher to query related comments:
docs = retriever.invoke("What do customers think about the battery life?")
By default, the searcher uses similarity search, but we can specify MMR as the search type:
retriever = vectorstore.as_retriever(search_type="mmr")
Additionally, we can pass parameters such as similarity score thresholds, or use top-k to limit the number of results:
retriever = vectorstore.as_retriever(search_kwargs={"k": 2, "score_threshold": 0.6})
Output:
Using a vector database as a searcher can enhance document retrieval by ensuring efficient access to relevant information.
- Using MultiQueryRetriever
MultiQueryRetriever enhances distance-based vector database retrieval by addressing common limitations such as changes in query wording and suboptimal embedding. Using large language model (LLM) automation prompt adjustments, multiple queries can be generated for a given user input from different angles. This process allows retrieval of relevant documents for each query and combine the results to produce a richer set of potential documents.
Build a sample vector database
To demonstrate MultiQueryRetriever, let's create a vector store using the product description from the CSV file:
from langchain_community.document_loaders import CSVLoader from langchain_community.vectorstores import FAISS from langchain_openai import OpenAIEmbeddings from langchain_text_splitters import CharacterTextSplitter # Load product description loader = CSVLoader("product_descriptions.csv") data = loader.load() # Split text into blocks text_splitter = CharacterTextSplitter(chunk_size=300, chunk_overlap=50) documents = text_splitter.split_documents(data) # Create vector storage embeddings = OpenAIEmbeddings() vectordb = FAISS.from_documents(documents, embeddeds)
Simple usage
To use MultiQueryRetriever, specify the LLM used for query generation:
from langchain.retrievers.multi_query import MultiQueryRetriever from langchain_openai import ChatOpenAI question = "What features do customers value in smartphones?" llm = ChatOpenAI(temperature=0) retriever_from_llm = MultiQueryRetriever.from_llm( retriever=vectordb.as_retriever(), llm=llm ) unique_docs = retriever_from_llm.invoke(question) len(unique_docs) # Number of unique documents retrieved
Output:
MultiQueryRetriever generates multiple queries, enhancing the diversity and relevance of retrieved documents.
Custom Tips
To adjust the generated query, you can create a custom PromptTemplate and an output parser:
from langchain_core.output_parsers import BaseOutputParser from langchain_core.prompts import PromptTemplate from typing import List # Custom output parser class LineListOutputParser(BaseOutputParser[List[str]]): def parse(self, text: str) -> List[str]: return list(filter(None, text.strip().split("\n"))) output_parser = LineListOutputParser() # Custom prompts for query generation QUERY_PROMPT = PromptTemplate( input_variables=["question"], template="""Generate five different versions of the question: {question}""" ) llm_chain = QUERY_PROMPT | llm | output_parser # Initialize the retriever retriever = MultiQueryRetriever( retriever=vectordb.as_retriever(), llm_chain=llm_chain, parser_key="lines" ) unique_docs = retriever.invoke("What features do customers value in smartphones?") len(unique_docs) # Number of unique documents retrieved
Output
Using MultiQueryRetriever can achieve a more efficient search process, ensuring diverse and comprehensive results based on user queries.
- How to search using context compression
Retrieving relevant information from a large collection of documents can be challenging, especially when data ingestion is not known about the specific query the user will make. Often, valuable insights are hidden in lengthy documents, resulting in inefficient and costly calls to the language model (LLM) while providing less responsiveness than ideal. Context compression solves this problem by improving the search process, ensuring that relevant information is returned only based on the user's query. This compression includes reducing the content of a single document and filtering out irrelevant documents.
Overview of context compression
The context compression searcher runs by integrating the basic searcher with the document compressor. This method does not return the document in its entirety, but compresses the document based on the context provided by the query. This compression includes reducing the content of a single document and filtering out irrelevant documents.
Implementation steps
- Initialize the basic searcher: First set up a normal vector storage searcher. For example, consider a news article about climate change policy:
from langchain_community.document_loaders import TextLoader from langchain_community.vectorstores import FAISS from langchain_openai import OpenAIEmbeddings from langchain_text_splitters import CharacterTextSplitter # Load and split the article documents = TextLoader("climate_change_policy.txt").load() text_splitter = CharacterTextSplitter(chunk_size=1000, chunk_overlap=0) texts = text_splitter.split_documents(documents) # Initialize vector storage retriever retriever = FAISS.from_documents(texts, OpenAIEmbeddings()).as_retriever()
- Execute an initial query: Execute a query to view the results returned by the basic retriever, which may include relevant and unrelated information.
docs = retriever.invoke("What actions are being proposed to combat climate change?")
- Enhanced search using context compression: wrap the basic searcher with ContextualCompressionRetriever, and extract relevant content using LLMChainExtractor:
from langchain.retrievers import ContextualCompressionRetriever from langchain.retrievers.document_compressors import LLMChainExtractor from langchain_openai import OpenAI llm = OpenAI(temperature=0) compressor = LLMChainExtractor.from_llm(llm) compression_retriever = ContextualCompressionRetriever( base_compressor=compressor, base_retriever=retriever ) # Perform compressed_docs = compression_retriever.invoke("What actions are being proposed to combat climate change?")
View the compressed results: ContextualCompressionRetriever processes the initial document and extracts only relevant information related to the query, thereby optimizing the response.
Create a custom searcher
Retrieval is essential in many LLM applications. Its task is to obtain relevant documents based on user queries. These documents are formatted as LLM prompts, enabling them to generate appropriate responses.
interface
To create a custom retriever, extend the BaseRetriever class and implement the following:
method | describe | Required/Optional |
---|---|---|
_get_relevant_documents
|
Search documents related to the query. | Required |
_aget_relevant_documents
|
Asynchronous implementation for native support. | Optional |
Inherited from BaseRetriever will provide standard Runnable functionality to your retriever.
Example
Here is an example of a simple retriever:
from typing import List from langchain_core.documents import Document from langchain_core.retrievers import BaseRetriever class ToyRetriever(BaseRetriever): """A simple retriever that returns the first k documents containing the user's query.""" documents: List[Document] k: int def _get_relevant_documents(self, query: str) -> List[Document]: matching_documents = [doc for doc in self.documents if query.lower() in doc.page_content.lower()] return matching_documents[:self.k] # Example usage documents = [ Document("Dogs are great companies.", {"type": "dog"}), Document("Cats are independent pets.", {"type": "cat"}), ] retriever = ToyRetriever(documents=documents, k=1) result = retriever.invoke("dog") print(result[0].page_content)
Output
This implementation provides a simple way to retrieve documents based on user input, illustrating the core functionality of a custom searcher in LangChain.
in conclusion
In the LangChain framework, the searcher is a powerful tool that can effectively access relevant information in various document types and use cases. By understanding and implementing different searcher types (such as vector storage retrievers, MultiQueryRetriever, and context compression retrievers), developers can customize document retrievals based on the specific needs of their applications.
Each retriever type has unique advantages, from using MultiQueryRetriever to using context compression to optimizing responses. Additionally, creating a custom searcher provides greater flexibility to accommodate special requirements that built-in options may not meet. Mastering these search techniques allows developers to build more efficient and responsive applications that leverage the potential of language models and large data sets.
Frequently Asked Questions
Q1. What is the main function of the searcher in LangChain? A1. The main function of the searcher is to obtain relevant documents based on the query. This helps the application effectively access necessary information in large datasets without storing documents themselves.
Q2. How is the difference between a searcher and a vector database? A2. A vector database is used to store documents in a way that allows similarity-based searches, and the searcher is an interface for searching documents based on queries. Although a vector database can be part of a searcher, the searcher's task focuses on obtaining relevant information.
Q3. What is MultiQueryRetriever and how does it work? A3. MultiQueryRetriever improves search results by creating multiple variants of queries using language models. This method captures a wider range of documents that may be related to different wording issues, thereby enhancing the richness of the search information.
Q4. Why is context compression important? A4. Context compression optimizes search results by reducing the content of the document to only relevant parts and filtering out unrelated information. This is especially useful in large collections, as the complete documentation may contain unrelated details, saving resources and providing a more centralized response.
Q5. What are the requirements for setting MultiQueryRetriever? A5. To set up MultiQueryRetriever, you need a vector database for document storage, a language model (LLM) for generating multiple query perspectives, and optional custom prompt templates to further optimize query generation.
The above is the detailed content of 3 Advanced Strategies for Retrievers in LangChain. For more information, please follow other related articles on the PHP Chinese website!
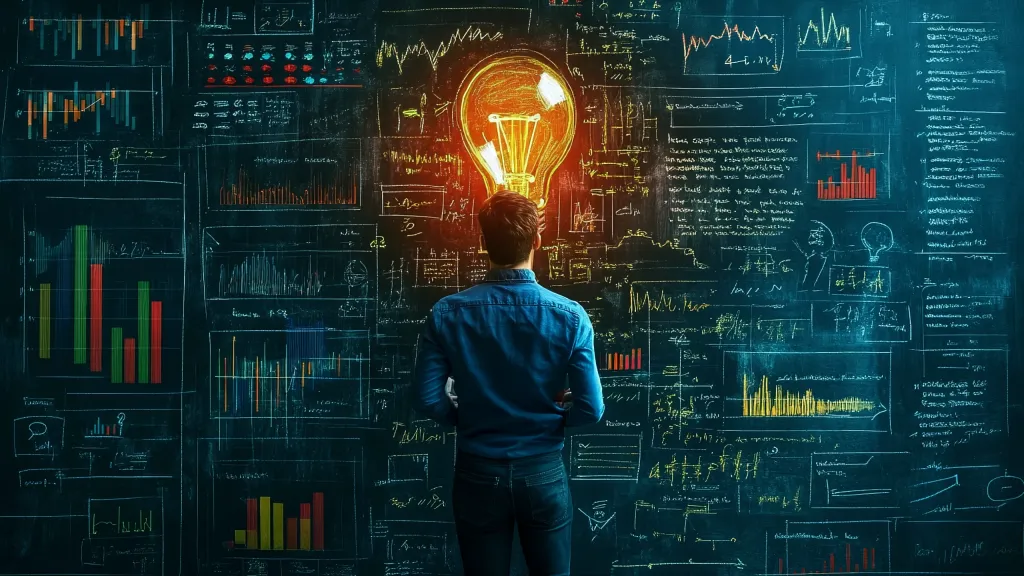
Introduction In prompt engineering, “Graph of Thought” refers to a novel approach that uses graph theory to structure and guide AI’s reasoning process. Unlike traditional methods, which often involve linear s
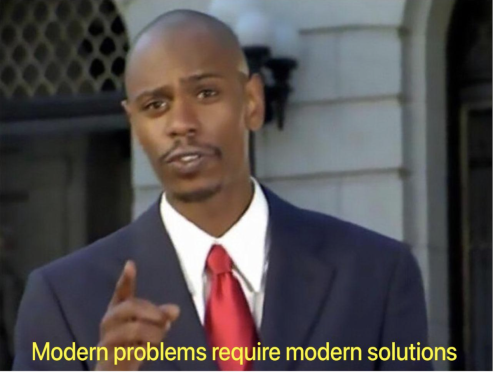
Introduction Congratulations! You run a successful business. Through your web pages, social media campaigns, webinars, conferences, free resources, and other sources, you collect 5000 email IDs daily. The next obvious step is
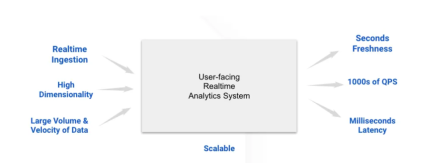
Introduction In today’s fast-paced software development environment, ensuring optimal application performance is crucial. Monitoring real-time metrics such as response times, error rates, and resource utilization can help main
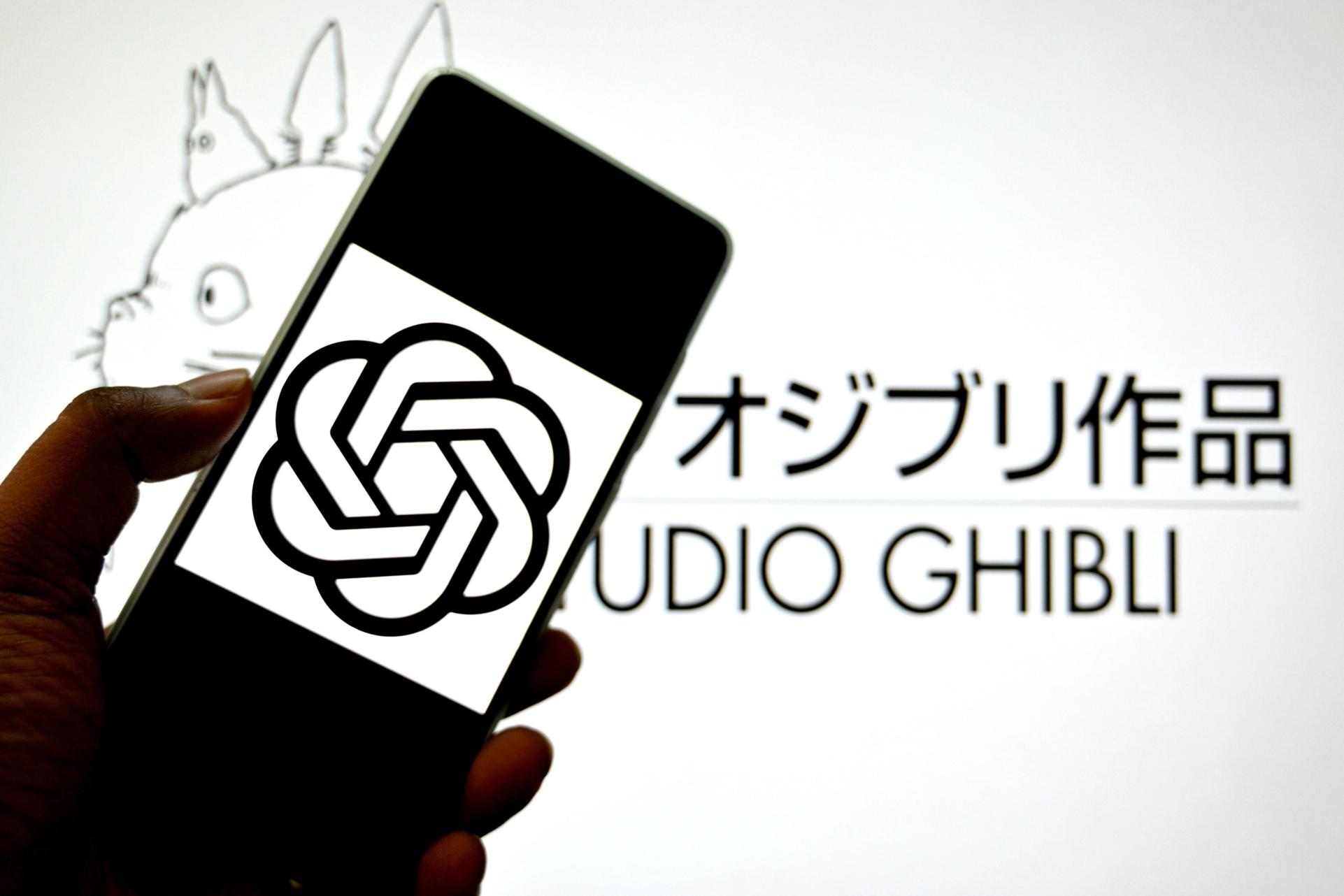
“How many users do you have?” he prodded. “I think the last time we said was 500 million weekly actives, and it is growing very rapidly,” replied Altman. “You told me that it like doubled in just a few weeks,” Anderson continued. “I said that priv
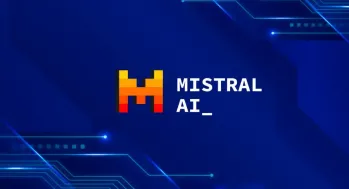
Introduction Mistral has released its very first multimodal model, namely the Pixtral-12B-2409. This model is built upon Mistral’s 12 Billion parameter, Nemo 12B. What sets this model apart? It can now take both images and tex
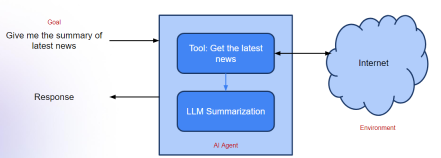
Imagine having an AI-powered assistant that not only responds to your queries but also autonomously gathers information, executes tasks, and even handles multiple types of data—text, images, and code. Sounds futuristic? In this a
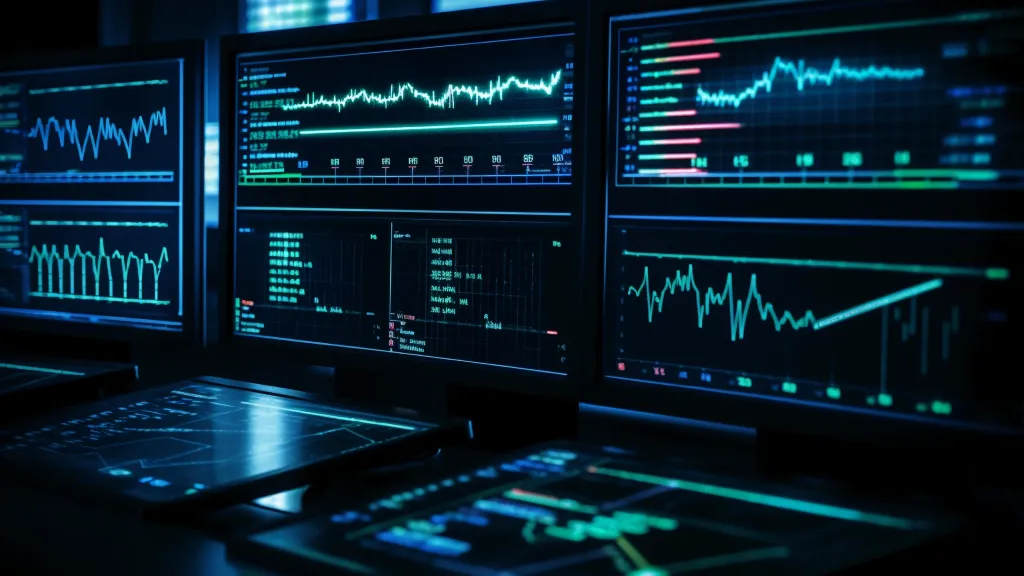
Introduction The finance industry is the cornerstone of any country’s development, as it drives economic growth by facilitating efficient transactions and credit availability. The ease with which transactions occur and credit

Introduction Data is being generated at an unprecedented rate from sources such as social media, financial transactions, and e-commerce platforms. Handling this continuous stream of information is a challenge, but it offers an


Hot AI Tools

Undresser.AI Undress
AI-powered app for creating realistic nude photos

AI Clothes Remover
Online AI tool for removing clothes from photos.

Undress AI Tool
Undress images for free

Clothoff.io
AI clothes remover

AI Hentai Generator
Generate AI Hentai for free.

Hot Article

Hot Tools

Safe Exam Browser
Safe Exam Browser is a secure browser environment for taking online exams securely. This software turns any computer into a secure workstation. It controls access to any utility and prevents students from using unauthorized resources.

MantisBT
Mantis is an easy-to-deploy web-based defect tracking tool designed to aid in product defect tracking. It requires PHP, MySQL and a web server. Check out our demo and hosting services.

SAP NetWeaver Server Adapter for Eclipse
Integrate Eclipse with SAP NetWeaver application server.

SublimeText3 English version
Recommended: Win version, supports code prompts!
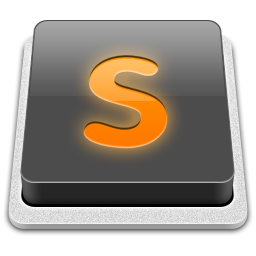
SublimeText3 Mac version
God-level code editing software (SublimeText3)