Case Studies or References Showing Performance Gains with RoPE and ALiBi
Rotary Position Embedding (RoPE):
-
Case Study 1: In the paper “RoFormer: Rotary Position Embedding for Transformer Models,” the authors demonstrated that RoPE significantly improved performance on long-sequence tasks like language modeling. The ability of RoPE to generalize better over long sequences without requiring extra computational resources made it a more efficient choice over traditional embeddings.
-
Performance Gain: RoPE provided up to 4-6% better accuracy in handling sequences longer than 512 tokens, compared to models using traditional positional encodings.
ALiBi (Attention with Linear Biases):
-
Case Study 2: In “ALiBi: Attention with Linear Biases for Efficient Long-Range Sequence Modeling,” the introduction of linear bias in the attention mechanism allowed the model to efficiently process sequences without relying on positional encoding. ALiBi reduced the memory overhead and improved the scalability of the model for tasks like machine translation and summarization.
-
Performance Gain: ALiBi demonstrated up to 8% faster training times and significant reductions in memory usage while maintaining or improving model performance on long-sequence benchmarks.
These advancements showcase how modern positional embedding techniques like RoPE and ALiBi not only address the limitations of traditional methods but also enhance the scalability and efficiency of large language models, especially when dealing with long inputs.
Harnessing the Power of Lower Precision
LLMs are composed of vast matrices and vectors representing their weights. These weights are typically stored in float32, bfloat16, or float16 precision. Memory requirements can be estimated as follows:
-
Float32 Precision: Memory required = 4 * X GB, where X is the number of model parameters (in billions).
-
bfloat16/Float16 Precision: Memory required = 2 * X GB.
Examples of Memory Usage in bfloat16 Precision:
-
GPT-3: 175 billion parameters, ~350 GB VRAM.
-
Bloom: 176 billion parameters, ~352 GB VRAM.
-
LLaMA-2-70B: 70 billion parameters, ~140 GB VRAM.
-
Falcon-40B: 40 billion parameters, ~80 GB VRAM.
-
MPT-30B: 30 billion parameters, ~60 GB VRAM.
-
Starcoder: 15.5 billion parameters, ~31 GB VRAM.
Given that the NVIDIA A100 GPU has a maximum of 80 GB VRAM, larger models need tensor parallelism or pipeline parallelism to operate efficiently.
Practical Example
Loading BLOOM on an 8 x 80GB A100 node:
!pip install transformers accelerate bitsandbytes optimum
# from transformers import AutoModelForCausalLM
# model = AutoModelForCausalLM.from_pretrained("bigscience/bloom", device_map="auto")
from transformers import AutoModelForCausalLM, AutoTokenizer, pipeline
import torch
model = AutoModelForCausalLM.from_pretrained("bigcode/octocoder", torch_dtype=torch.bfloat16, device_map="auto", pad_token_id=0)
tokenizer = AutoTokenizer.from_pretrained("bigcode/octocoder")
pipe = pipeline("text-generation", model=model, tokenizer=tokenizer)
prompt = "Question: Please write a function in Python that transforms bytes to Giga bytes.\n\nAnswer:"
result = pipe(prompt, max_new_tokens=60)[0]["generated_text"][len(prompt):]
result
def bytes_to_giga_bytes(bytes):
return bytes / 1024 / 1024 / 1024
bytes_to_giga_bytes(torch.cuda.max_memory_allocated())
model.to("cpu")
del pipe
del model
import gc
import torch
def flush():
gc.collect()
torch.cuda.empty_cache()
torch.cuda.reset_peak_memory_stats()
flush()
There are various quantization techniques, which we won’t discuss in detail here, but in general, all quantization techniques work as follows:
- Quantize all weights to the target precision.
- Load the quantized weights, and pass the input sequence of vectors in bfloat16 precision.
- Dynamically dequantize weights to bfloat16 to perform the computation with their input vectors in bfloat16 precision.
- Quantize the weights again to the target precision after computation with their inputs.
In a nutshell, this means thatinputs-weight matrixmultiplications, withXbeing theinputs,Wbeing a weight matrix andYbeing the output:
Y=X∗W are changed to Y=X∗dequantize(W);quantize(W) for every matrix multiplication. Dequantization and re-quantization is performed sequentially for all weight matrices as the inputs run through the network graph.
# !pip install bitsandbytes
model = AutoModelForCausalLM.from_pretrained("bigcode/octocoder", load_in_8bit=True, low_cpu_mem_usage=True, pad_token_id=0)
pipe = pipeline("text-generation", model=model, tokenizer=tokenizer)
result = pipe(prompt, max_new_tokens=60)[0]["generated_text"][len(prompt):]
result
bytes_to_giga_bytes(torch.cuda.max_memory_allocated())
model.cpu()
del model
del pipe
flush()
model = AutoModelForCausalLM.from_pretrained("bigcode/octocoder", load_in_4bit=True, low_cpu_mem_usage=True, pad_token_id=0)
pipe = pipeline("text-generation", model=model, tokenizer=tokenizer)
result = pipe(prompt, max_new_tokens=60)[0]["generated_text"][len(prompt):]
result
bytes_to_giga_bytes(torch.cuda.max_memory_allocated())
model.cpu()
del model
del pipe
model.cpu()
del model
del pipe
flush()
model = AutoModelForCausalLM.from_pretrained("bigcode/octocoder", load_in_4bit=True, low_cpu_mem_usage=True, pad_token_id=0)
pipe = pipeline("text-generation", model=model, tokenizer=tokenizer)
result = pipe(prompt, max_new_tokens=60)[0]["generated_text"][len(prompt):]
result
bytes_to_giga_bytes(torch.cuda.max_memory_allocated())
model.cpu()
del model
del pipe
flush()
Flash Attention Mechanism
Flash Attention optimizes the attention mechanism by enhancing memory efficiency and leveraging better GPU memory utilization. This approach allows for:
-
Reduced memory footprint: Drastically minimizes memory overhead by handling attention computation more efficiently.
-
Higher performance: Significant improvements in speed during inference.
system_prompt = """Below are a series of dialogues between various people and an AI technical assistant.
The assistant tries to be helpful, polite, honest, sophisticated, emotionally aware, and humble but knowledgeable.
The assistant is happy to help with code questions and will do their best to understand exactly what is needed.
It also tries to avoid giving false or misleading information, and it caveats when it isn't entirely sure about the right answer.
That said, the assistant is practical really does its best, and doesn't let caution get too much in the way of being useful.
The Starcoder models are a series of 15.5B parameter models trained on 80 programming languages from The Stack (v1.2) (excluding opt-out requests).
The model uses Multi Query Attention, was trained using the Fill-in-the-Middle objective, and with 8,192 tokens context window for a trillion tokens of heavily deduplicated data.
-----
Question: Write a function that takes two lists and returns a list that has alternating elements from each input list.
Answer: Sure. Here is a function that does that.
def alternating(list1, list2):
results = []
for i in range(len(list1)):
results.append(list1[i])
results.append(list2[i])
return results
Question: Can you write some test cases for this function?
Answer: Sure, here are some tests.
assert alternating([10, 20, 30], [1, 2, 3]) == [10, 1, 20, 2, 30, 3]
assert alternating([True, False], [4, 5]) == [True, 4, False, 5]
assert alternating([], []) == []
Question: Modify the function so that it returns all input elements when the lists have uneven length. The elements from the longer list should be at the end.
Answer: Here is the modified function.
def alternating(list1, list2):
results = []
for i in range(min(len(list1), len(list2))):
results.append(list1[i])
results.append(list2[i])
if len(list1) > len(list2):
results.extend(list1[i 1:])
else:
results.extend(list2[i 1:])
return results
-----
"""
long_prompt = 10 * system_prompt prompt
model = AutoModelForCausalLM.from_pretrained("bigcode/octocoder", torch_dtype=torch.bfloat16, device_map="auto")
tokenizer = AutoTokenizer.from_pretrained("bigcode/octocoder")
pipe = pipeline("text-generation", model=model, tokenizer=tokenizer)
import time
start_time = time.time()
result = pipe(long_prompt, max_new_tokens=60)[0]["generated_text"][len(long_prompt):]
print(f"Generated in {time.time() - start_time} seconds.")
result
bytes_to_giga_bytes(torch.cuda.max_memory_allocated())
Output:
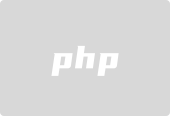
flush()
model = model.to_bettertransformer()
start_time = time.time()
result = pipe(long_prompt, max_new_tokens=60)[0]["generated_text"][len(long_prompt):]
print(f"Generated in {time.time() - start_time} seconds.")
result
bytes_to_giga_bytes(torch.cuda.max_memory_allocated())
flush()
Output:
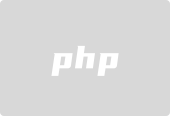
Science Behind LLM Architectures
So far, we have explored strategies to improve computational and memory efficiency, including:
- Casting weights to a lower precision format.
- Implementing a more efficient version of the self-attention algorithm.
Now, we turn our attention to how we can modify the architecture of large language models (LLMs) to optimize them for tasks requiring long text inputs, such as:
- Retrieval-augmented question answering,
- Summarization,
- Chat applications.
Notably, chat interactions necessitate that LLMs not only process long text inputs but also efficiently handle dynamic, back-and-forth dialogue between the user and the model, similar to what ChatGPT accomplishes.
Since modifying the fundamental architecture of an LLM post-training is challenging, making well-considered design decisions upfront is essential. Two primary components in LLM architectures that often become performance bottlenecks for large input sequences are:
- Positional embeddings
- Key-value cache
Let’s delve deeper into these components.
Improving Positional Embeddings in LLMs
The self-attention mechanism relates each token to others within a text sequence. For instance, the Softmax(QKT) matrix for the input sequence “Hello”, “I”, “love”, “you” could appear as follows:
|
Hello |
I |
Love |
You |
Hello |
0.2 |
0.4 |
0.3 |
0.1 |
I |
0.1 |
0.5 |
0.2 |
0.2 |
Love |
0.05 |
0.3 |
0.65 |
0.0 |
You |
0.15 |
0.25 |
0.35 |
0.25 |
Each word token has a probability distribution indicating how much it attends to other tokens. For example, the word “love” attends to “Hello” with 0.05 probability, “I” with 0.3, and itself with 0.65.
However, without positional embeddings, an LLM struggles to understand the relative positions of tokens, making it hard to distinguish sequences like “Hello I love you” from “You love I hello”. QKT computation relates tokens without considering the positional distance, treating each as equidistant.
To resolve this, positional encodings are introduced, providing numerical cues that help the model understand the order of tokens.
Traditional Positional Embeddings
In the original Attention Is All You Need paper, sinusoidal positional embeddings were proposed, where each vector is defined as a sinusoidal function of its position. These embeddings are added to input sequence vectors as:
Some models, such as BERT, introduced learned positional embeddings, which are learned during training.
Challenges with Absolute Positional Embeddings
Sinusoidal and learned positional embeddings are absolute, encoding unique positions. However, as noted by Huang et al. and Su et al., absolute embeddings can hinder performance for long sequences. Key issues include:
-
Long Input Limitation: Absolute embeddings perform poorly when handling long sequences since they focus on fixed positions instead of relative distances.
-
Fixed Training Length: Learned embeddings tie the model to a maximum training length, limiting its ability to generalize to longer inputs.
Advancements: Relative Positional Embeddings
To address these challenges, relative positional embeddings have gained traction. Two notable methods include:
- Rotary Position Embedding (RoPE)
- ALiBi (Attention with Linear Biases)
Both methods modify the QKT computation to incorporate sentence order directly into the self-attention mechanism, improving how models handle long text inputs.
Rotary Position Embedding (RoPE) encodes positional information by rotating query and key vectors by angles and, respectively, where denote positions:
Here, is a rotational matrix, and is predefined based on the training’s maximum input length.
These approaches enable LLMs to focus on relative distances, improving generalization for longer sequences and facilitating efficient task-specific optimizations.
input_ids = tokenizer(prompt, return_tensors="pt")["input_ids"].to("cuda")
for _ in range(5):
next_logits = model(input_ids)["logits"][:, -1:]
next_token_id = torch.argmax(next_logits,dim=-1)
input_ids = torch.cat([input_ids, next_token_id], dim=-1)
print("shape of input_ids", input_ids.shape)
generated_text = tokenizer.batch_decode(input_ids[:, -5:])
generated_text
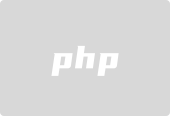
past_key_values = None # past_key_values is the key-value cache
generated_tokens = []
next_token_id = tokenizer(prompt, return_tensors="pt")["input_ids"].to("cuda")
for _ in range(5):
next_logits, past_key_values = model(next_token_id, past_key_values=past_key_values, use_cache=True).to_tuple()
next_logits = next_logits[:, -1:]
next_token_id = torch.argmax(next_logits, dim=-1)
print("shape of input_ids", next_token_id.shape)
print("length of key-value cache", len(past_key_values[0][0])) # past_key_values are of shape [num_layers, 0 for k, 1 for v, batch_size, length, hidden_dim]
generated_tokens.append(next_token_id.item())
generated_text = tokenizer.batch_decode(generated_tokens)
generated_text
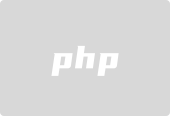
config = model.config
2 * 16_000 * config.n_layer * config.n_head * config.n_embd // config.n_head
Output
7864320000
Conclusion
Optimizing LLM architectures for long text inputs and dynamic chat applications is pivotal in advancing their real-world applicability. The challenges of managing extensive input contexts, maintaining computational efficiency, and delivering meaningful conversational interactions necessitate innovative solutions at the architectural level. Techniques like Rotary Position Embedding (RoPE), ALiBi, and Flash Attention illustrate the transformative potential of fine-tuning center components like positional embeddings and self-attention.
As the field proceeds to advance, a center on mixing computational effectiveness with engineering inventiveness will drive the following wave of breakthroughs. By understanding and actualizing these procedures, designers can tackle the total control of LLMs, guaranteeing they are not fair brilliantly but too adaptable, responsive, and common for different real-world applications.
Key Takeaways
- Techniques like RoPE and ALiBi improve LLMs’ ability to process longer texts without sacrificing performance.
- Innovations like Flash Attention and sliding window attention reduce memory usage, making large models practical for real-world applications.
- Optimizing LLMs for long text inputs enhances their ability to maintain context and coherence in extended conversations and complex tasks.
- LLMs are evolving to support tasks such as summarization, retrieval, and multi-turn dialogues with better scalability and responsiveness.
- Reducing model precision improves computational efficiency while maintaining accuracy, enabling broader adoption.
- Balancing architecture design and resource optimization ensures LLMs remain effective for diverse and growing use cases.
Frequently Asked Questions
Q1. 1. What are LLMs, and why are they significant? A. Large Language Models (LLMs) are AI models outlined to get it and create human-like content. They are critical due to their capacity to perform a wide extend of assignments, from replying questions to imaginative composing, making them flexible apparatuses for different businesses.
Q2. How do RoPE and ALiBi improve LLMs? A. RoPE (Rotary Positional Encoding) and ALiBi (Attention with Linear Biases) enhance LLMs by improving their capability to handle long contexts, ensuring efficient processing of extended text without losing coherence.
Q3. What is Flash Attention, and how does it optimize memory usage? A. Flash Attention is an algorithm that computes attention more efficiently, significantly reducing memory consumption and speeding up processing for large-scale models.
Q4. Why is quantization important for LLMs? A. Quantization decreases the accuracy of demonstrate weights (e.g., from 32-bit to 8-bit), which brings down computational necessities and memory utilization whereas keeping up shows execution, empowering arrangement on smaller gadgets.
Q5. What challenges remain for scaling LLMs further? A. Major challenges include managing computational and memory costs, addressing ethical concerns like bias and misuse, and ensuring models can generalize effectively across diverse tasks and languages.
Q6. How can LLMs be optimized for processing long text inputs effectively? A. Optimizing LLMs for long text inputs involves techniques like context window expansion, memory mechanisms, and efficient token processing to ensure they maintain coherence and performance during extended conversations or document analysis.