Machine learning (ML) is now a cornerstone of modern technology, empowering businesses and researchers to make more precise data-driven decisions. However, the sheer number of available ML models make choosing the right one for a specific task challenging. This article explores cruel factors for effective model selection, from data understanding and problem definition to model evaluation, trade-off analysis, and informed decision-making tailored to individual needs.
Table of contents
- Model selection definition
- The importance of model selection
- How to select the initial model set?
- How to select the best model from the selected model (model selection technique)?
- in conclusion
- Frequently Asked Questions
Model selection definition
Model selection refers to the process of identifying the most suitable machine learning model for a particular task by evaluating various options based on the performance of the model and consistency with problem requirements. It involves considering factors such as problem type (e.g., classification or regression), characteristics of the data, relevant performance metrics, and tradeoffs between underfitting and overfitting. Practical limitations, such as computing resources and the need for interpretability, can also affect choices. The goal is to select a model that provides the best performance and meets project goals and constraints.
The importance of model selection
Choosing the right machine learning (ML) model is a critical step in developing a successful AI solution. The importance of model selection lies in its impact on the performance, efficiency, and feasibility of ML applications. Here are the reasons for its importance:
1. Accuracy and performance
Different models are good at different task types. For example, a decision tree might be suitable for classified data, while a convolutional neural network (CNN) is good at image recognition. Choosing the wrong model may result in suboptimal predictions or high error rates, reducing the reliability of the solution.
2. Efficiency and scalability
The computational complexity of an ML model affects its training and inference time. For large-scale or real-time applications, lightweight models such as linear regression or random forests may be more appropriate than computationally intensive neural networks.
Models that cannot be effectively scaled as data increases can lead to bottlenecks.
3. Interpretability
Depending on the application, interpretability may be a priority. For example, in the healthcare or finance field, stakeholders often need to have clear reasons for predictions. Simple models (such as logistic regression) may be preferable to black box models (such as deep neural networks).
4. Field Applicability
Some models are designed for specific data types or fields. Time series prediction benefits from models such as ARIMA or LSTM, while natural language processing tasks often utilize converter-based architectures.
5. Resource limitations
Not all organizations have the computing power to run complex models. Simpler models that perform well within resource constraints can help balance performance and feasibility.
6. Overfitting and generalization
Complex models with many parameters are easily overfitted, capturing noise rather than latent patterns. Choosing a model that generalizes well to new data ensures better actual performance.
7. Adaptability
The ability of models to adapt to changing data distributions or requirements is crucial in dynamic environments. For example, online learning algorithms are more suitable for real-time evolution of data.
8. Cost and development time
Some models require a lot of hyperparameter adjustment, feature engineering, or labeling data, which increases development costs and time. Choosing the right model can simplify development and deployment.
How to select the initial model set?
First, you need to select a set of models based on the data you have and the tasks you want to perform. This will save you time compared to testing each ML model.
1. Based on task:
- Classification: If the goal is to predict categories (e.g., "spam" vs. "non-spam"), then the classification model should be used.
- Model examples: logistic regression, decision tree, random forest, support vector machine (SVM), k-nearest neighbor (K-NN), neural network.
- Regression: If the goal is to predict continuous values (e.g., house prices, stock prices), a regression model should be used.
- Model examples: linear regression, decision tree, random forest regression, support vector regression, neural network.
- Clustering: If the goal is to group data into a cluster without previous tags, a clustering model is used.
- Model examples: k-mean, DBSCAN, hierarchical clustering, Gaussian hybrid model.
- Anomaly detection: If the target is to identify rare events or outliers, use the anomaly detection algorithm.
- Model examples: Isolated Forest, Single Class SVM, and Autoencoder.
- Time series prediction: If the goal is to predict future values based on time data.
- Model examples: ARIMA, exponential smoothing, LSTM, Prophet.
2. Based on data
type
- Structured data (table data): Use models such as decision trees, random forests, XGBoost, or logistic regression.
- Unstructured data (text, images, audio, etc.): Use models such as CNN (for images), RNN or converter (for text) or audio processing models.
size
- Small datasets: Simple models (such as logistic regression or decision trees) tend to work well, because complex models may be overfitted.
- Large data sets: Deep learning models (such as neural networks, CNNs, RNNs) are more suitable for processing large amounts of data.
quality
- Missing values: Some models (such as random forests) can handle missing values, while others (such as SVM) need to be imputed.
- Noise and outliers: Robust models (such as random forests) or models with regularization (such as lasso) are good choices for processing noise data.
How to select the best model from the selected model (model selection technique)?
Model selection is an important aspect of machine learning, which helps identify the best performing models in a given dataset and problem. The two main techniques are resampling methods and probability measurements, each with its unique model evaluation method.
1. Resampling method
The resampling method involves rearranging and reusing subsets of data to test the performance of the model on unseen samples. This helps evaluate the model's ability to generalize new data. The two main resampling techniques are:
Cross-validation
Cross-validation is a systematic resampling procedure used to evaluate model performance. In this method:
- The data set is divided into groups or folds.
- One group is used as test data, and the rest are used for training.
- The model is trained and evaluated iteratively across all folds.
- Compute the average performance of all iterations to provide reliable accuracy metrics.
Cross-validation is especially useful when comparing models such as support vector machines (SVMs) and logistic regression to determine which model is better suited for a particular problem.
Bootstrap method
Bootstrap is a sampling technique in which data are randomly sampled in an alternative way to estimate the performance of the model.
Main features
- Mainly used in smaller data sets.
- The size of the sample and test data matches the original dataset.
- Samples that produce the highest score are usually used.
The process involves randomly selecting an observation value, recording it, putting it back into the dataset, and repeating the process n times. The generated boot samples provide insights into model robustness.
2. Probability Measurement
Probability metrics evaluate the performance of the model based on statistical metrics and complexity. These approaches focus on balancing performance and simplicity. Unlike resampling, they do not require separate test sets because performance is calculated using training data.
Akagi Information Guidelines (AIC)
AIC evaluates the model by balancing the goodness of fit and its complexity. It originates from information theory and penalizes the number of parameters in the model to avoid overfitting.
formula:
- Goodness of fit: Higher likelihood means better fitting of data.
- Complexity penalty: The term 2k penalizes models with more parameters to avoid overfitting.
- Explanation: The lower the AIC score, the better the model. However, AICs may sometimes skew towards overly complex models because they balance fit and complexity and are less stringent than other standards.
Bayesian Information Criteria (BIC)
BIC is similar to AIC, but the punishment for model complexity is stronger, making it more conservative. It is particularly useful in model selection for time series and regression models where overfitting is a problem.
formula:
- Goodness of fit: Like AIC, higher likelihoods improve scores.
- Complexity penalty: This term punishes models with more parameters, and the penalty increases as the sample size n increases.
- Explanation: BICs tend to be more simplistic models than AICs because it means stricter penalties for additional parameters.
Minimum Description Length (MDL)
MDL is a principle that selects the model that compresses data most efficiently. It is rooted in information theory and aims to minimize the total cost of describing models and data.
formula:
- Simplicity and efficiency: MDL tends to model that best balances between simplicity (shorter model description) and accuracy (the ability to represent the data).
- Compression: A good model provides a concise summary of the data, effectively reducing its description length.
- Explanation: The model with the lowest MDL is preferred.
in conclusion
Choosing the best machine learning model for a specific use case requires a systematic approach, balancing problem requirements, data characteristics, and practical limitations. By understanding the nature of the task, the structure of the data, and the tradeoffs involved in model complexity, accuracy, and interpretability, you can narrow down the candidate models. Technologies such as cross-validation and probability metrics (AIC, BIC, MDL) ensure that these candidates are rigorously evaluated, allowing you to choose a model that generalizes well and meets your goals.
Ultimately, the model selection process is iterative and context-driven. It is crucial to consider problem areas, resource constraints, and a balance between performance and feasibility. By carefully integrating domain expertise, experimentation, and evaluation metrics, you can choose an ML model that not only provides the best results, but also meets the practical and operational needs of your application.
If you are looking for online AI/ML courses, explore: Certified AI and ML Black Belt Plus Program
Frequently Asked Questions
Q1. How do I know which ML model is the best?
A: Choosing the best ML model depends on the type of problem (categorization, regression, clustering, etc.), the size and quality of the data, and the tradeoffs required between accuracy, interpretability, and computational efficiency. First determine your problem type (e.g., regression used to predict numbers or classifications used to classify data). For smaller data sets or when interpretability is critical, use simple models such as linear regression or decision trees, and for larger data sets that require higher accuracy, use more complex models such as random forests or neural networks. Always evaluate the model using metrics related to your goals (e.g., accuracy, accuracy, and RMSE) and test multiple algorithms to find the best fit.
Q2. How to compare 2 ML models?
A: To compare two ML models, evaluate their performance on the same dataset using consistent evaluation metrics. Split the data into training and test sets (or use cross validation) to ensure fairness and evaluate each model using metrics related to your question, such as accuracy, accuracy, or RMSE. The results are analyzed to determine which model performs better, but also consider tradeoffs such as interpretability, training time, and scalability. If the performance differences are small, use statistical tests to confirm the significance. Ultimately, a model that balances performance with the actual requirements of the use case is chosen.
Q3. Which ML model is best for predicting sales?
A: The best ML model for predicting sales depends on your dataset and requirements, but commonly used models include gradient boosting algorithms such as linear regression, decision trees, or XGBoost. Linear regression works well for simple data sets with clear linear trends. For more complex relationships or interactions, gradient boosts or random forests often provide higher accuracy. If the data involves time series patterns, models such as ARIMA, SARIMA, or long short-term memory (LSTM) networks are more suitable. Choose a model that balances predictive performance, interpretability, and scalability of sales forecast demand.
The above is the detailed content of How To Choose Best ML Model For Your Usecase?. For more information, please follow other related articles on the PHP Chinese website!
![Can't use ChatGPT! Explaining the causes and solutions that can be tested immediately [Latest 2025]](https://img.php.cn/upload/article/001/242/473/174717025174979.jpg?x-oss-process=image/resize,p_40)
ChatGPT is not accessible? This article provides a variety of practical solutions! Many users may encounter problems such as inaccessibility or slow response when using ChatGPT on a daily basis. This article will guide you to solve these problems step by step based on different situations. Causes of ChatGPT's inaccessibility and preliminary troubleshooting First, we need to determine whether the problem lies in the OpenAI server side, or the user's own network or device problems. Please follow the steps below to troubleshoot: Step 1: Check the official status of OpenAI Visit the OpenAI Status page (status.openai.com) to see if the ChatGPT service is running normally. If a red or yellow alarm is displayed, it means Open
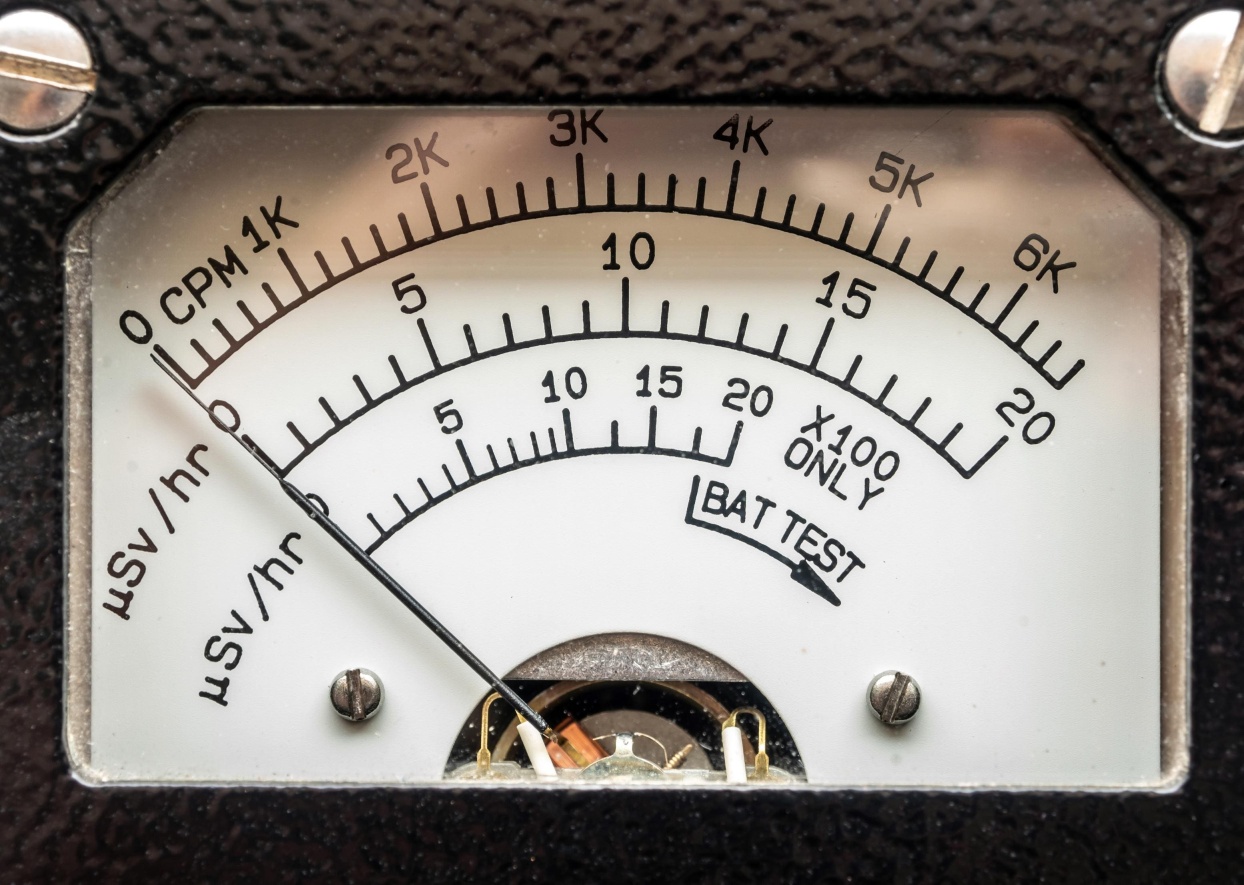
On 10 May 2025, MIT physicist Max Tegmark told The Guardian that AI labs should emulate Oppenheimer’s Trinity-test calculus before releasing Artificial Super-Intelligence. “My assessment is that the 'Compton constant', the probability that a race to
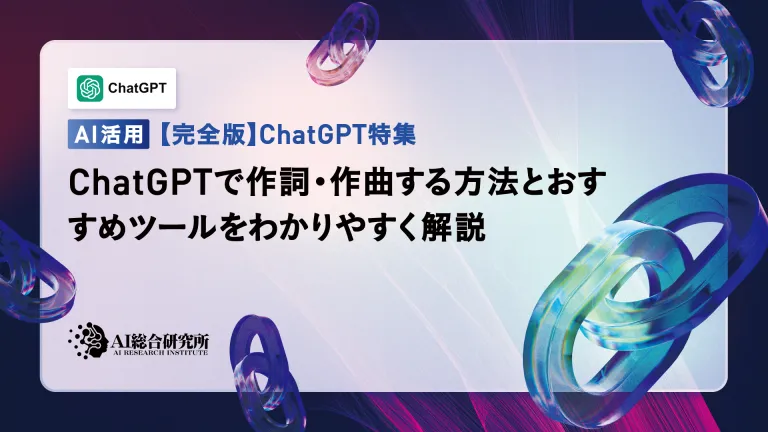
AI music creation technology is changing with each passing day. This article will use AI models such as ChatGPT as an example to explain in detail how to use AI to assist music creation, and explain it with actual cases. We will introduce how to create music through SunoAI, AI jukebox on Hugging Face, and Python's Music21 library. Through these technologies, everyone can easily create original music. However, it should be noted that the copyright issue of AI-generated content cannot be ignored, and you must be cautious when using it. Let’s explore the infinite possibilities of AI in the music field together! OpenAI's latest AI agent "OpenAI Deep Research" introduces: [ChatGPT]Ope
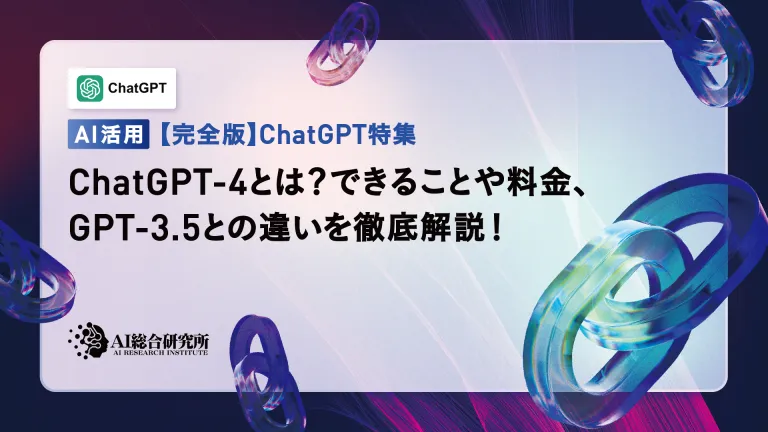
The emergence of ChatGPT-4 has greatly expanded the possibility of AI applications. Compared with GPT-3.5, ChatGPT-4 has significantly improved. It has powerful context comprehension capabilities and can also recognize and generate images. It is a universal AI assistant. It has shown great potential in many fields such as improving business efficiency and assisting creation. However, at the same time, we must also pay attention to the precautions in its use. This article will explain the characteristics of ChatGPT-4 in detail and introduce effective usage methods for different scenarios. The article contains skills to make full use of the latest AI technologies, please refer to it. OpenAI's latest AI agent, please click the link below for details of "OpenAI Deep Research"
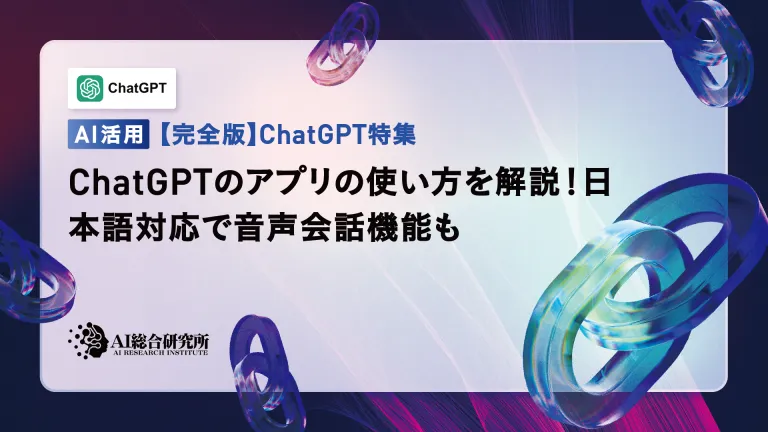
ChatGPT App: Unleash your creativity with the AI assistant! Beginner's Guide The ChatGPT app is an innovative AI assistant that handles a wide range of tasks, including writing, translation, and question answering. It is a tool with endless possibilities that is useful for creative activities and information gathering. In this article, we will explain in an easy-to-understand way for beginners, from how to install the ChatGPT smartphone app, to the features unique to apps such as voice input functions and plugins, as well as the points to keep in mind when using the app. We'll also be taking a closer look at plugin restrictions and device-to-device configuration synchronization
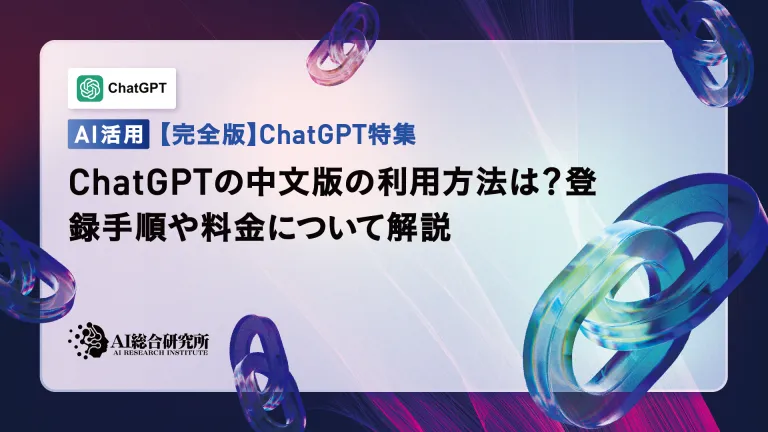
ChatGPT Chinese version: Unlock new experience of Chinese AI dialogue ChatGPT is popular all over the world, did you know it also offers a Chinese version? This powerful AI tool not only supports daily conversations, but also handles professional content and is compatible with Simplified and Traditional Chinese. Whether it is a user in China or a friend who is learning Chinese, you can benefit from it. This article will introduce in detail how to use ChatGPT Chinese version, including account settings, Chinese prompt word input, filter use, and selection of different packages, and analyze potential risks and response strategies. In addition, we will also compare ChatGPT Chinese version with other Chinese AI tools to help you better understand its advantages and application scenarios. OpenAI's latest AI intelligence
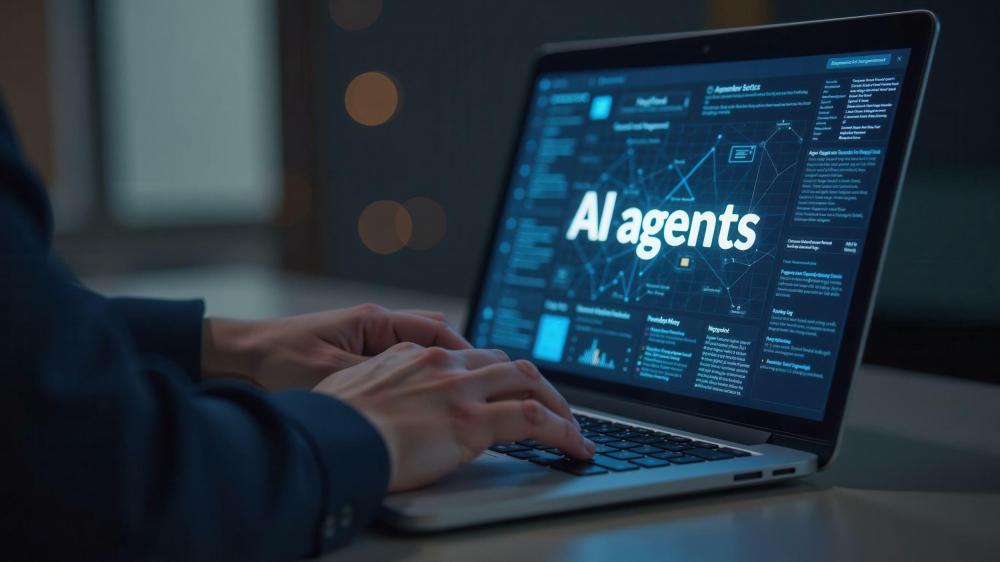
These can be thought of as the next leap forward in the field of generative AI, which gave us ChatGPT and other large-language-model chatbots. Rather than simply answering questions or generating information, they can take action on our behalf, inter
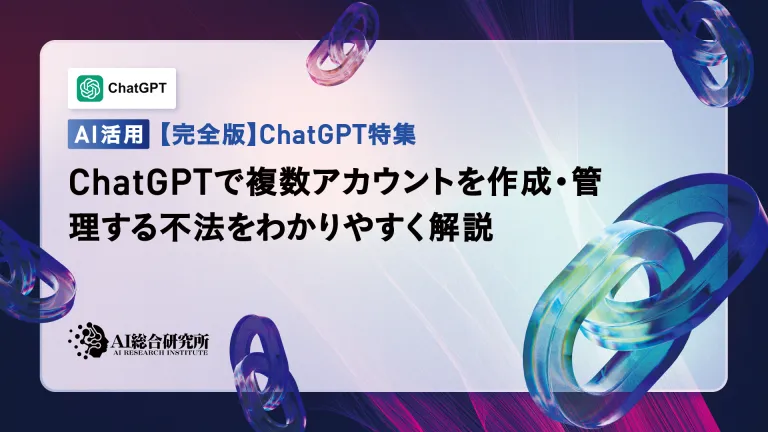
Efficient multiple account management techniques using ChatGPT | A thorough explanation of how to use business and private life! ChatGPT is used in a variety of situations, but some people may be worried about managing multiple accounts. This article will explain in detail how to create multiple accounts for ChatGPT, what to do when using it, and how to operate it safely and efficiently. We also cover important points such as the difference in business and private use, and complying with OpenAI's terms of use, and provide a guide to help you safely utilize multiple accounts. OpenAI


Hot AI Tools

Undresser.AI Undress
AI-powered app for creating realistic nude photos

AI Clothes Remover
Online AI tool for removing clothes from photos.

Undress AI Tool
Undress images for free

Clothoff.io
AI clothes remover

Video Face Swap
Swap faces in any video effortlessly with our completely free AI face swap tool!

Hot Article

Hot Tools
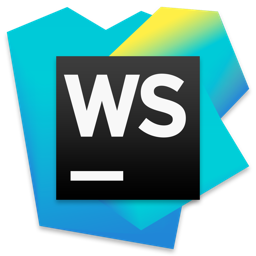
WebStorm Mac version
Useful JavaScript development tools

mPDF
mPDF is a PHP library that can generate PDF files from UTF-8 encoded HTML. The original author, Ian Back, wrote mPDF to output PDF files "on the fly" from his website and handle different languages. It is slower than original scripts like HTML2FPDF and produces larger files when using Unicode fonts, but supports CSS styles etc. and has a lot of enhancements. Supports almost all languages, including RTL (Arabic and Hebrew) and CJK (Chinese, Japanese and Korean). Supports nested block-level elements (such as P, DIV),

MantisBT
Mantis is an easy-to-deploy web-based defect tracking tool designed to aid in product defect tracking. It requires PHP, MySQL and a web server. Check out our demo and hosting services.

SublimeText3 Chinese version
Chinese version, very easy to use
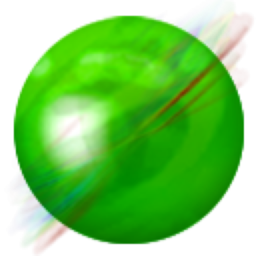
ZendStudio 13.5.1 Mac
Powerful PHP integrated development environment
