Deep learning GPU benchmarks has revolutionized the way we solve complex problems, from image recognition to natural language processing. However, while training these models often relies on high-performance GPUs, deploying them effectively in resource-constrained environments such as edge devices or systems with limited hardware presents unique challenges. CPUs, being widely available and cost-efficient, often serve as the backbone for inference in such scenarios. But how do we ensure that models deployed on CPUs deliver optimal performance without compromising accuracy?
This article dives into the benchmarking of deep learning model inference on CPUs, focusing on three critical metrics: latency, CPU utilization and Memory Utilization. Using a spam classification example, We explore how popular frameworks like PyTorch, TensorFlow, JAX , and ONNX Runtime handle inference workloads. By the end, you’ll have a clear understanding of how to measure performance, optimize deployments, and select the right tools and frameworks for CPU-based inference in resource-constrained environments.
Impact: Optimal inference execution can save a significant amount of money and free up resources for other workloads.
Learning Objectives
- Understand the role of Deep Learning CPU benchmarks in assessing hardware performance for AI model training and inference.
- Evaluate PyTorch, TensorFlow, JAX, ONNX Runtime, and OpenVINO Runtime to choose the best for your needs.
- Master tools like psutil and time to collect accurate performance data and optimize inference.
- Prepare models, run inference, and measure performance, applying techniques to diverse tasks like image classification and NLP.
- Identify bottlenecks, optimize models, and enhance performance while managing resources efficiently.
This article was published as a part of theData Science Blogathon.
Table of contents
- Optimizing Inference with Runtime Acceleration
- Model Inference Performance Metrics
- Assumptions and Limitations
- Tools and Frameworks
- Install Dependencies
- Problem Statement and Input Specification
- Models Architecture and Formats
- Examples of Additional Networks for Benchmarking
- Benchmarking Workflow
- Benchmarking Function Definiton
- Model Inference and Perform Benchmarking for Each Framework
- Results and Discussion
- Conclusion
- Frequently Asked Questions
Optimizing Inference with Runtime Acceleration
Inference speed is essential for user experience and operational efficiency in machine learning applications. Runtime optimization plays a key role in enhancing this by streamlining execution. Using hardware-accelerated libraries like ONNX Runtime takes advantage of optimizations tailored to specific architectures, reducing latency (time per inference).
Additionally, lightweight model formats such as ONNX minimize overhead, enabling faster loading and execution. Optimized runtimes leverage parallel processing to distribute computation across available CPU cores and improve memory management, ensuring better performance especially on systems with limited resources. This approach makes models faster and more efficient while maintaining accuracy.
Model Inference Performance Metrics
To evaluate the performance of our models, we focus on three key metric:
Latency
- Definition : Latency refers to the time it takes for the model to make a prediction after receiving input. This is often measured as the time taken from sending the input data to receiving the output (prediction)
- Importance : In real-time or near-real-time applications, high latency leads to delays, which can result in slower responses.
- Measurement : Latency is typically measure in milliseconds (ms) or seconds (s). Shorter latency means the system is more responsive and efficient, crucial for applications requiring immediate decision-making or actions.
CPU Utilization
- Definition: CPU Utilization is the percentage of the CPU’s processing power that is consumed while performing inference tasks. It tells you how much of the system’s computational resources are being used during model inference.
- Importance : High CPU usage means that the machine might struggle to handle other tasks concurrently, leading to bottlenecks. Efficient use of CPU resources ensures that the model inference does not monopolize the system resources.
- Measurement : It is typically measured as a percentage (%) of the total available CPU resources. Lower utilization for the same workload generally indicates a more optimized model, utilizing CPU resources more effectively.
Memory Utilization
- Definition: Memory utilization refers to the amount of RAM used by the model during the inference process. It tracks the memory consumption by the model’s parameters, intermediate computations, and the input data.
- Importance : Optimizing memory usage is especially critical when deploying models to edge devices or systems whith limited memory. High memory consumption could lead to memory overfloe, slower processing, or system crashes.
- Measurement: Memory utilization is measure in megabytes (MB) or gigabytes (GB). Tracking the memory consumption at different stages of inference can help identify memory inefficiencies or memory leaks.
Assumptions and Limitations
To keep this benchmarking study focused and practical, we made the following assumptions and set a few boundaries:
- Hardware Constraints: The tests are designed to run on a single machine with limited CPU cores. While modern hardware is capable of handling parallel workloads, this setup mirrors the constraints often seen in edge devices or smaller-scale deployments.
- No Multi-System Parallelization: We didn’t incorporate distributed computing setups or cluster-based solutions. The benchmarks reflect performance standalone conditions, suitable for single-node environments with limited CPU cores and Memory.
- Scope:The primary focus is only on CPU inference performance. While GPU-based inference is an excellent option for resource-intensive tasks, this benchmarking aims to provide insights into CPU-only setups, which are more common in cost-sensitive or portable applications.
These assumptions ensure the benchmarks remain relevant for developers and teams working with resource-constrained hardware or who need predictable performance without the added complexity of distributed systems.
Tools and Frameworks
We’ll explore the essential tools and frameworks used to benchmark and optimize deep learning model inference on CPUs, providing insights into their capabilities for efficient execution in resource-constrained environments.
Profiling Tools
- Python Time (time library) : The time library in Python is a lightweight tool for measuring the execution time of code blocks. By recording the start and end time stamps, it helps calculate the time taken for operations like model inference or data processing.
- psutil (CPU, Memory Profiling) : psutil is a Python library for sustem monitoring and profiling. It provides real-time data on CPU usage, memory consumption, disk I/O and more, making it ideal for analyzing usage during model training or inference.
Frameworks for Inference
- TensorFlow : A robust framework for deep learning that is widely used for both training and inference tasks. It offers strong support for various models and deployment strategies.
- PyTorch: Known for its ease of use and dynamic computation graphs, PyTorch is a popular choice for research and production deployment.
- ONNX Runtime: An open-source , cross-platform engine for running ONXX(Open Neural Network Exchange) models, providing efficient inference across various hardware and frameworks.
- JAX : A functional framework focused on high-performance numerical computing and machine learning, offering automatic differentiation and GPU/TPU acceleration.
- OpenVINO: Optimized for Intel hardware, OpenVINO provides tools for model optimization and deployment on Intel CPUs, GPUs and VPUs.
Hardware Specification and Environment
We are utilizing github codespace (virtual machine) with below configuration:
- Specification of Virtual Machine: 2 cores, 8 GB RAM, and 32 GB storage
- Python Version: 3.12.1
Install Dependencies
The versions of the packages used are as follows and this primary include five deep learning inference libraries: Tensorflow, Pytorch, ONNX Runtime, JAX, and OpenVINO:
!pip install numpy==1.26.4 !pip install torch==2.2.2 !pip install tensorflow==2.16.2 !pip install onnx==1.17.0 !pip install onnxruntime==1.17.0!pip install jax==0.4.30 !pip install jaxlib==0.4.30 !pip install openvino==2024.6.0 !pip install matplotlib==3.9.3 !pip install Matplotlib: 3.4.3 !pip install Pillow: 8.3.2 !pip install psutil: 5.8.0
Problem Statement and Input Specification
Since model inference consists of performing a few matrix operations between network weights and input data, it doesn’t require model training or datasets. For our example the benchmarking process, we simulated a standard classification use case. This simulates common binary classification tasks like spam detection and loan application decisions(approval or denial). The binary nature of these problems makes them ideal for comparing model performance across different frameworks. This setup reflects real-world systems but allows us to focus on inference performance across frameworks without needing large datasets or pre-trained models.
Problem Statement
The sample task involves predicting whether a given sample is spam or not (loan approval or denial), based on a set of input features. This binary classification problem is computationally efficient, allowing for a focused analysis of inference performance without the complexity of multi-class classification tasks.
Input Specification
To simulate real-world email data, we generated randomly input. These embeddings mimic the type of data that might be processed by spam filters but avoid the need for external datasets. This simulated input data allows for benchmarking without relying on any specific external datasets, making it ideal for testing model inference times, memory usage, and CPU performance. Alternatively, you can use image classification, NLP task or any other deep learning tasks to perform this benchmarking process.
Models Architecture and Formats
Model selection is a critical step in benchmarking as it directly influences the inference performance and insights gained from the profiling process. As mentioned in the previous section, for this benchmarking study, we chose a standard Classification use case, which involves identifying whether a given email is spam or not. This task is a straightforward two-class classification problem that is computationally efficient yet provides meaningful results for comparison across frameworks.
Models Architecture for Benchmarking
The model for the Classification task is a Feedforward Neural Network (FNN) designed for binary classification (Spam vs. Not Spam). It consists of the following layers:
- Input Layer : Accepts a vector of size 200(embedding features). We have provided example of PyTorch, other frameworks follow the exact same network configuration
self.fc1 = torch.nn.Linear(200,128)
- Hidden Layers : The network has 5 hidden layers, with each successive layer containing fewer units than the previous one.
self.fc2 = torch.nn.Linear(128, 64) self.fc3 = torch.nn.Linear(64, 32) self.fc4 = torch.nn.Linear(32, 16) self.fc5 = torch.nn.Linear(16, 8) self.fc6 = torch.nn.Linear(8, 1)
- Output Layers : A single neuron with a Sigmoid activation function to output a probability (0 for Not Spam, 1 for Spam). We have utilized sigmoid layer as final output for binary classification.
self.sigmoid = torch.nn.Sigmoid()
The model is simple yet effective for classification task.
The model architecture diagram used for benchmarking in our use case is shown below:
Examples of Additional Networks for Benchmarking
- Image Classification : Models like ResNet-50 (medium complexity) and MobileNet (lightweight) can be added to the benchmark suite for tasks involving image recognition. ResNet-50 offers a balance between computational complexity and accuracy, while MobileNet is optimized for low-resource environments.
- NLP Tasks : DistilBERT: A smaller, faster variant of the BERT model, suited for natural language understanding tasks.
Model Formats
- Native Formats: Each framework supports its native model formats, such as .pt for PyTorch and .h5 for TensorFlow.
- Unified Format (ONNX): To ensure compatibility across frameworks, We exported the PyTorch model to the ONNX format (model.onnx). ONNX (Open Neural Network Exchange) acts as a bridge, enabling models to be used in other frameworks like PyTorch, TensorFlow, JAX, or OpenVINO without significant modifications. This is especially useful for multi-framework testing and real-world deployment scenarios, where interoperability is critical.
- These formats are optimized for their respective frameworks, making them easy to save, load, and deploy within those ecosystems.
Benchmarking Workflow
This workflow aims to compare the inference performance of multiple deep learning frameworks (TensorFlow, PyTorch, ONNX, JAX, and OpenVINO) using the classification task. The task involves using randomly generated input data and benchmarking each framework to measure the average time taken for a prediction.
- Import python packages
- Disable GPU usage and suppress Tensorflow Logging
- Input data preparation
- Model Implementations for each framework
- Benchmarking function definition
- Model Inference and Benchmarking execution for each framework
- Visualization and export of Benchmarking Results
Import Necessary Python Packages
To get started with benchmarking deep learning models, we first need to import the essential Python packages that enable seamless integration and performance evaluation.
import time import os import numpy as np import torch import tensorflow as tf from tensorflow.keras import Input import onnxruntime as ort import matplotlib.pyplot as plt from PIL import Image import psutil import jax import jax.numpy as jnp from openvino.runtime import Core import csv
Disable GPU Usage and Suppress TensorFlow Logging
os.environ["CUDA_VISIBLE_DEVICES"] = "-1" # Disable GPU os.environ["TF_CPP_MIN_LOG_LEVEL"] = "3" #Suppress Tensorflow Log
Input Data Preparation
In this step, we randomly generate input data for spam classification:
- Dimensionality of a sample (200-dimesnional features)
- The number of classes (2: Spam or Not Spam)
We generate randome data using NumPy to serve as input features for the models.
#Generate dummy data input_data = np.random.rand(1000, 200).astype(np.float32)
Model Definition
In this step, we define the netwrok architecture or setup the model from each deep learning framework( Tensorflow, PyTorch, ONNX, JAX and OpenVINO). Each framework requires a specific methods for loading models and setting them up for inference.
- PyTorch Model: In PyTorch, we define a simple neural neural network architecture with five fully connected layers.
- Tensorflow Model : The TensorFlow model is defined using the Keras API and consists of a simple feedforward neural network for the classification task.
- JAX Model: The model is initialized with parameters, and the prediction function is compiled using JAX’s Just-in-Time (JIT) compilation for efficient execution.
- ONNX Model: For ONNX, we export a model from PyTorch. After exporting to the ONNX format, we load the model using the onnxruntime. InferenceSession API. This allows us to run inference on the model across different hardware specification.
- OpenVINO Model: OpenVINO is used for running optimized and deploying models, particularly those trained using other frameworks (like PyTorch or TensorFlow). We load the ONNX model and compile it with OpenVINO’s runtime.
Pytorch
class PyTorchModel(torch.nn.Module): def __init__(self): super(PyTorchModel, self).__init__() self.fc1 = torch.nn.Linear(200, 128) self.fc2 = torch.nn.Linear(128, 64) self.fc3 = torch.nn.Linear(64, 32) self.fc4 = torch.nn.Linear(32, 16) self.fc5 = torch.nn.Linear(16, 8) self.fc6 = torch.nn.Linear(8, 1) self.sigmoid = torch.nn.Sigmoid() def forward(self, x): x = torch.relu(self.fc1(x)) x = torch.relu(self.fc2(x)) x = torch.relu(self.fc3(x)) x = torch.relu(self.fc4(x)) x = torch.relu(self.fc5(x)) x = self.sigmoid(self.fc6(x)) return x # Create PyTorch model pytorch_model = PyTorchModel()
TensorFlow
tensorflow_model = tf.keras.Sequential([ Input(shape=(200,)), tf.keras.layers.Dense(128, activation='relu'), tf.keras.layers.Dense(64, activation='relu'), tf.keras.layers.Dense(32, activation='relu'), tf.keras.layers.Dense(16, activation='relu'), tf.keras.layers.Dense(8, activation='relu'), tf.keras.layers.Dense(1, activation='sigmoid') ]) tensorflow_model.compile()
Jax
def jax_model(x): x = jax.nn.relu(jnp.dot(x, jnp.ones((200, 128)))) x = jax.nn.relu(jnp.dot(x, jnp.ones((128, 64)))) x = jax.nn.relu(jnp.dot(x, jnp.ones((64, 32)))) x = jax.nn.relu(jnp.dot(x, jnp.ones((32, 16)))) x = jax.nn.relu(jnp.dot(x, jnp.ones((16, 8)))) x = jax.nn.sigmoid(jnp.dot(x, jnp.ones((8, 1)))) return x
ONNX
# Convert PyTorch model to ONNX dummy_input = torch.randn(1, 200) onnx_model_path = "model.onnx" torch.onnx.export( pytorch_model, dummy_input, onnx_model_path, export_params=True, opset_version=11, input_names=['input'], output_names=['output'], dynamic_axes={'input': {0: 'batch_size'}, 'output': {0: 'batch_size'}} ) onnx_session = ort.InferenceSession(onnx_model_path)
OpenVINO
# OpenVINO Model Definition core = Core() openvino_model = core.read_model(model="model.onnx") compiled_model = core.compile_model(openvino_model, device_name="CPU")
Benchmarking Function Definiton
This function executes benchmarking tests across different frameworks by taking three arguments: predict_function, input_data, and num_runs. By default, it executes 1,000 times but It can be increased as per requirements.
def benchmark_model(predict_function, input_data, num_runs=1000): start_time = time.time() process = psutil.Process(os.getpid()) cpu_usage = [] memory_usage = [] for _ in range(num_runs): predict_function(input_data) cpu_usage.append(process.cpu_percent()) memory_usage.append(process.memory_info().rss) end_time = time.time() avg_latency = (end_time - start_time) / num_runs avg_cpu = np.mean(cpu_usage) avg_memory = np.mean(memory_usage) / (1024 * 1024) # Convert to MB return avg_latency, avg_cpu, avg_memory
Model Inference and Perform Benchmarking for Each Framework
Now that we have loaded the models, it’s time to benchmark the performance of each framework. The benchmarking process perform inference on the generated input data.
PyTorch
# Benchmark PyTorch model def pytorch_predict(input_data): pytorch_model(torch.tensor(input_data)) pytorch_latency, pytorch_cpu, pytorch_memory = benchmark_model(lambda x: pytorch_predict(x), input_data)
TensorFlow
# Benchmark TensorFlow model def tensorflow_predict(input_data): tensorflow_model(input_data) tensorflow_latency, tensorflow_cpu, tensorflow_memory = benchmark_model(lambda x: tensorflow_predict(x), input_data)
JAX
# Benchmark JAX model def jax_predict(input_data): jax_model(jnp.array(input_data)) jax_latency, jax_cpu, jax_memory = benchmark_model(lambda x: jax_predict(x), input_data)
ONNX
# Benchmark ONNX model def onnx_predict(input_data): # Process inputs in batches for i in range(input_data.shape[0]): single_input = input_data[i:i 1] # Extract single input onnx_session.run(None, {onnx_session.get_inputs()[0].name: single_input}) onnx_latency, onnx_cpu, onnx_memory = benchmark_model(lambda x: onnx_predict(x), input_data)
OpenVINO
# Benchmark OpenVINO model def openvino_predict(input_data): # Process inputs in batches for i in range(input_data.shape[0]): single_input = input_data[i:i 1] # Extract single input compiled_model.infer_new_request({0: single_input}) openvino_latency, openvino_cpu, openvino_memory = benchmark_model(lambda x: openvino_predict(x), input_data)
Results and Discussion
Here we discuss the results of performance benchmarking of previously mentioned deep learning frameworks. We compare them on – latency, CPU usage, and memory usage. We have included tabular data and plot for quick comparison.
Latency Comparison
Framework | Latency (ms) | Relative Latency (vs. PyTorch) |
PyTorch | 1.26 | 1.0 (baseline) |
TensorFlow | 6.61 | ~5.25× |
JAX | 3.15 | ~2.50× |
ONNX | 14.75 | ~11.72× |
OpenVINO | 144.84 | ~115× |
Insights:
- PyTorch leads as the fastest framework with ~1.26 ms latency.
- TensorFlow has ~6.61 ms latency, about 5.25× PyTorch’s time.
- JAX sits between PyTorch and TensorFlow in absolute latency.
- ONNX is relatively slow as well, at ~14.75 ms.
- OpenVINO is the slowest in this experiment, at ~145 ms (115× slower than PyTorch).
CPU Usage
Framework | CPU Usage (%) | Relative CPU Usage1 |
PyTorch | 99.79 | ~1.00 |
TensorFlow | 112.26 | ~1.13 |
JAX | 130.03 | ~1.31 |
ONNX | 99.58 | ~1.00 |
OpenVINO | 99.32 | 1.00 (baseline) |
Insights:
- JAX uses the most CPU (~130 %), ~31% higher than OpenVINO.
- TensorFlow is at ~112 %, more than PyTorch/ONNX/OpenVINO but still lower than JAX.
- PyTorch, ONNX, and OpenVINO, all have similar, ~99-100% CPU usage.
Memory Usage
Framework | Memory (MB) | Relative Memory Usage (vs. PyTorch) |
PyTorch | ~959.69 | 1.0 (baseline) |
TensorFlow | ~969.72 | ~1.01× |
JAX | ~1033.63 | ~1.08× |
ONNX | ~1033.82 | ~1.08× |
OpenVINO | ~1040.80 | ~1.08–1.09× |
Insights:
- PyTorch and TensorFlow have similar memory usage around ~960-970 MB
- JAX, ONNX, and OpenVINO use around ~1,030–1,040 MB of memory, approximately 8–9% more than PyTorch.
Here is the plot comparing the Performance of Deep Learning Frameworks:
Conclusion
In this article, we presented a comprehensive benchmarking workflow to evaluate the inference performance of prominent deep learning frameworks—TensorFlow, PyTorch, ONNX, JAX, and OpenVINO—using a spam classification task as a reference. By analyzing key metrics such as latency, CPU usage and memory consumption, the results highlighted the trade-offs between frameworks and their suitability for different deployment scenarios.
PyTorch demonstrated the most balanced performance, excelling in low latency and efficient memory usage, making it ideal for latency-sensitive applications like real-time predictions and recommendation systems. TensorFlow provided a middle-ground solution with moderately higher resource consumption. JAX showcased high computational throughput but at the cost of increased CPU utilization, which might be a limiting factor for resource-constrained environments. Meanwhile, ONNX and OpenVINO lagged in latency, with OpenVINO’s performance particularly hindered by the absence of hardware acceleration.
These findings underline the importance of aligning framework selection with deployment needs. Whether optimizing for speed, resource efficiency, or specific hardware, understanding the trade-offs is essential for effective model deployment in real-world environments.
Key Takeaways
- Deep Learning CPU Benchmarks provide critical insights into CPU performance, aiding in selecting optimal hardware for AI tasks.
- Leveraging Deep Learning CPU Benchmarks ensures efficient model training and inference by identifying high-performing CPUs.
- Achieved the best latency (1.26 ms) and maintained efficient memory usage, ideal for real-time and resource-limited applications.
- Balanced latency (6.61 ms) with slightly higher CPU usage, suitable for tasks requiring moderate performance compromises.
- Delivered competitive latency (3.15 ms) but at the cost of excessive CPU utilization (130%), limiting its utility in constrained setups.
- Showed higher latency (14.75 ms), but its cross-platform support makes it flexible for multi-framework deployments.
Frequently Asked Questions
Q1. Why is PyTorch preferred for real-time applications?A. PyTorch’s dynamic computation graph and efficient execution pipeline allow for low-latency inference (1.26 ms), making it well-suited for applications like recommendation systems and real-time predictions.
Q2. What affected OpenVINO’s performance in this study?A. OpenVINO’s optimizations are designed for Intel hardware. Without this acceleration, its latency (144.84 ms) and memory usage (1040.8 MB) were less competitive compared to other frameworks.
Q3. How do I choose a framework for resource-constrained environments?A. For CPU-only setups, PyTorch is the most efficient. TensorFlow is a strong alternative for moderate workloads. Avoid frameworks like JAX unless higher CPU utilization is acceptable.
Q4. What role does hardware play in framework performance?A. Framework performance depends heavily on hardware compatibility. For instance, OpenVINO excels on Intel CPUs with hardware-specific optimizations, while PyTorch and TensorFlow perform consistently across varied setups.
Q5. Can benchmarking results differ with complex models or tasks?A. Yes, these results reflect a simple binary classification task. Performance could vary with complex architectures like ResNet or tasks like NLP or others, where these frameworks might leverage specialized optimizations.
The media shown in this article is not owned by Analytics Vidhya and is used at the Author’s discretion.
The above is the detailed content of Deep Learning CPU Benchmarks. For more information, please follow other related articles on the PHP Chinese website!
![Can't use ChatGPT! Explaining the causes and solutions that can be tested immediately [Latest 2025]](https://img.php.cn/upload/article/001/242/473/174717025174979.jpg?x-oss-process=image/resize,p_40)
ChatGPT is not accessible? This article provides a variety of practical solutions! Many users may encounter problems such as inaccessibility or slow response when using ChatGPT on a daily basis. This article will guide you to solve these problems step by step based on different situations. Causes of ChatGPT's inaccessibility and preliminary troubleshooting First, we need to determine whether the problem lies in the OpenAI server side, or the user's own network or device problems. Please follow the steps below to troubleshoot: Step 1: Check the official status of OpenAI Visit the OpenAI Status page (status.openai.com) to see if the ChatGPT service is running normally. If a red or yellow alarm is displayed, it means Open
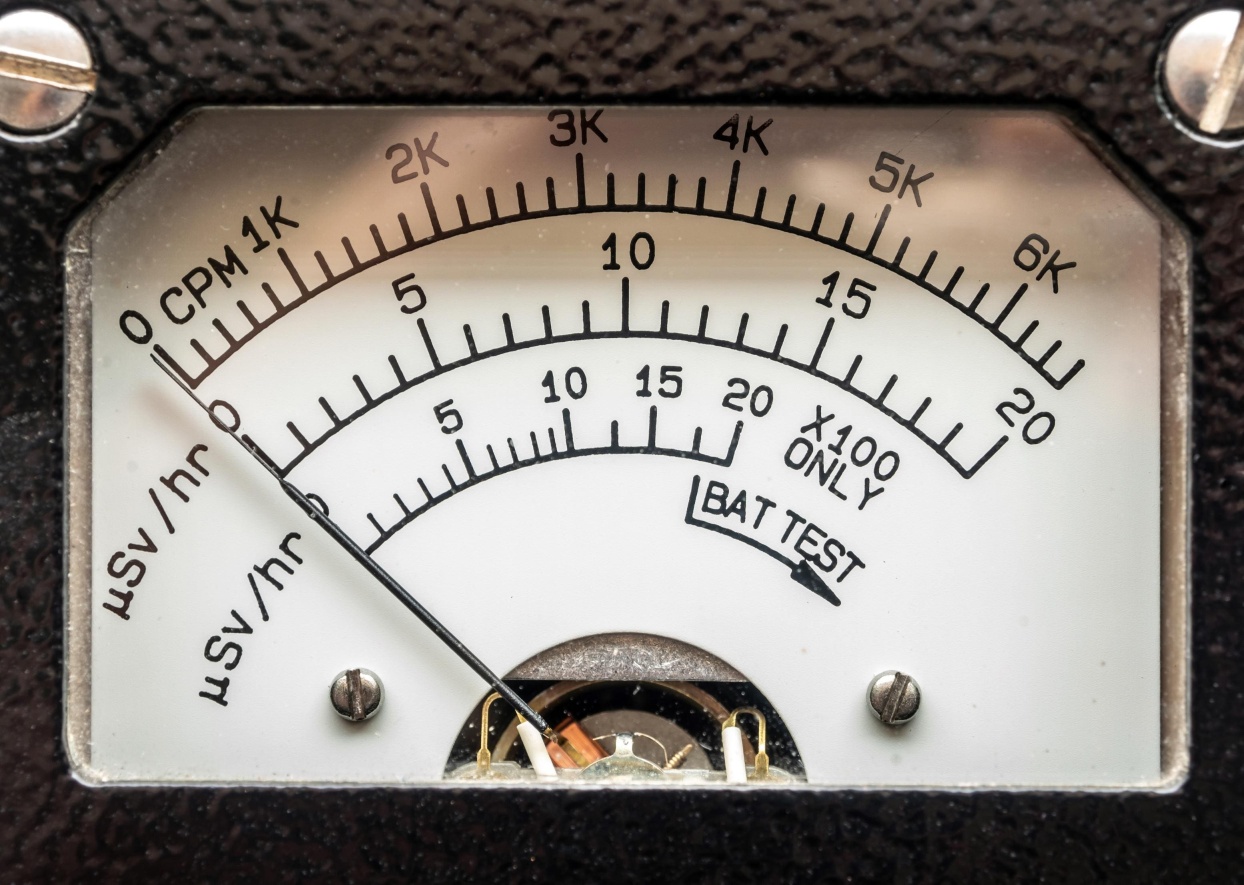
On 10 May 2025, MIT physicist Max Tegmark told The Guardian that AI labs should emulate Oppenheimer’s Trinity-test calculus before releasing Artificial Super-Intelligence. “My assessment is that the 'Compton constant', the probability that a race to
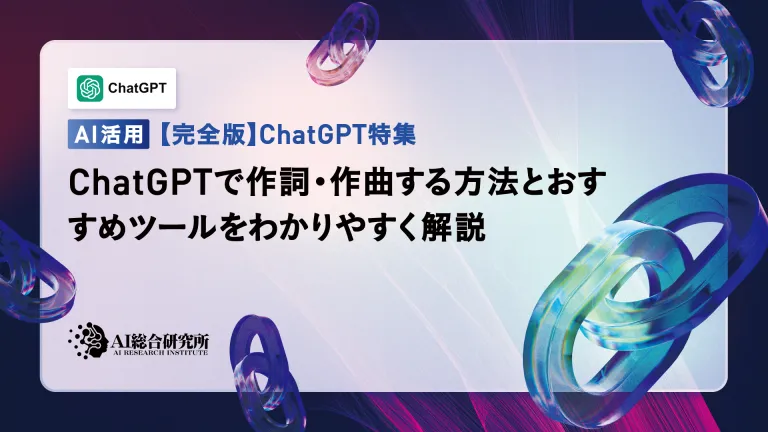
AI music creation technology is changing with each passing day. This article will use AI models such as ChatGPT as an example to explain in detail how to use AI to assist music creation, and explain it with actual cases. We will introduce how to create music through SunoAI, AI jukebox on Hugging Face, and Python's Music21 library. Through these technologies, everyone can easily create original music. However, it should be noted that the copyright issue of AI-generated content cannot be ignored, and you must be cautious when using it. Let’s explore the infinite possibilities of AI in the music field together! OpenAI's latest AI agent "OpenAI Deep Research" introduces: [ChatGPT]Ope
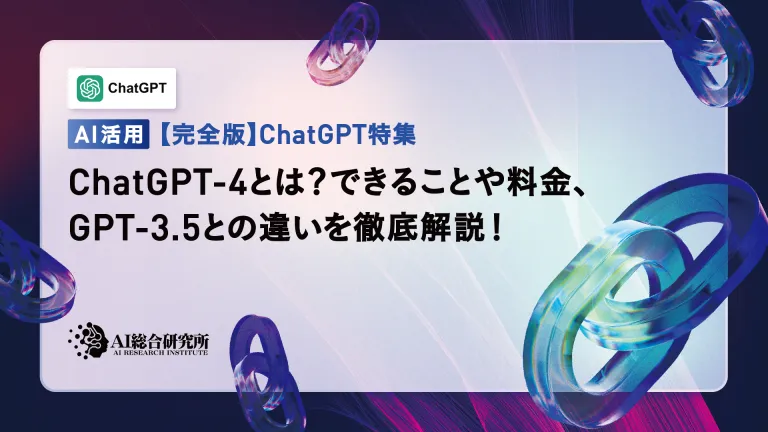
The emergence of ChatGPT-4 has greatly expanded the possibility of AI applications. Compared with GPT-3.5, ChatGPT-4 has significantly improved. It has powerful context comprehension capabilities and can also recognize and generate images. It is a universal AI assistant. It has shown great potential in many fields such as improving business efficiency and assisting creation. However, at the same time, we must also pay attention to the precautions in its use. This article will explain the characteristics of ChatGPT-4 in detail and introduce effective usage methods for different scenarios. The article contains skills to make full use of the latest AI technologies, please refer to it. OpenAI's latest AI agent, please click the link below for details of "OpenAI Deep Research"
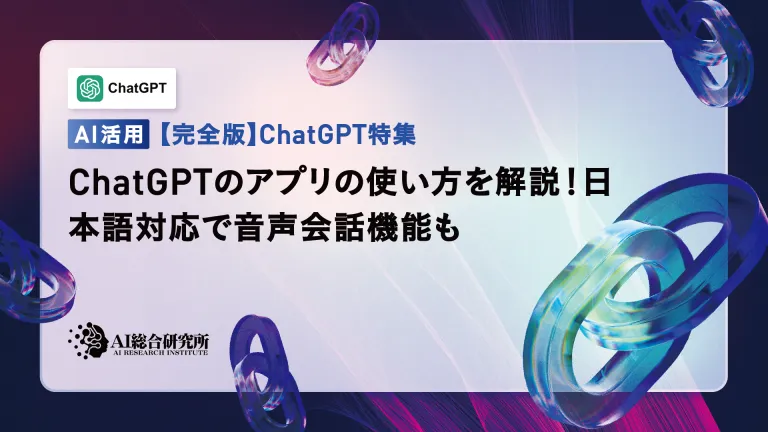
ChatGPT App: Unleash your creativity with the AI assistant! Beginner's Guide The ChatGPT app is an innovative AI assistant that handles a wide range of tasks, including writing, translation, and question answering. It is a tool with endless possibilities that is useful for creative activities and information gathering. In this article, we will explain in an easy-to-understand way for beginners, from how to install the ChatGPT smartphone app, to the features unique to apps such as voice input functions and plugins, as well as the points to keep in mind when using the app. We'll also be taking a closer look at plugin restrictions and device-to-device configuration synchronization
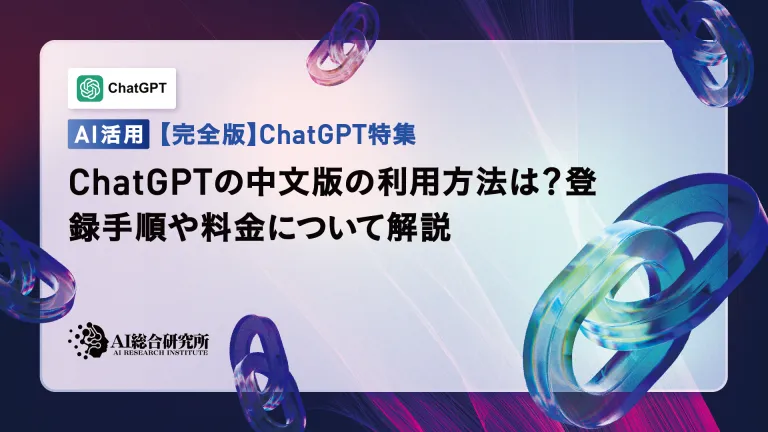
ChatGPT Chinese version: Unlock new experience of Chinese AI dialogue ChatGPT is popular all over the world, did you know it also offers a Chinese version? This powerful AI tool not only supports daily conversations, but also handles professional content and is compatible with Simplified and Traditional Chinese. Whether it is a user in China or a friend who is learning Chinese, you can benefit from it. This article will introduce in detail how to use ChatGPT Chinese version, including account settings, Chinese prompt word input, filter use, and selection of different packages, and analyze potential risks and response strategies. In addition, we will also compare ChatGPT Chinese version with other Chinese AI tools to help you better understand its advantages and application scenarios. OpenAI's latest AI intelligence
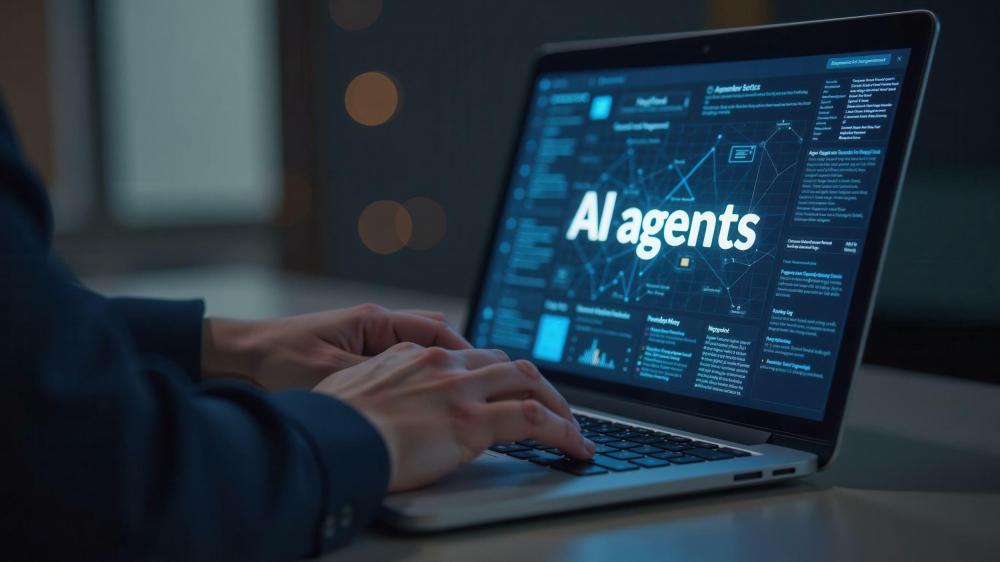
These can be thought of as the next leap forward in the field of generative AI, which gave us ChatGPT and other large-language-model chatbots. Rather than simply answering questions or generating information, they can take action on our behalf, inter
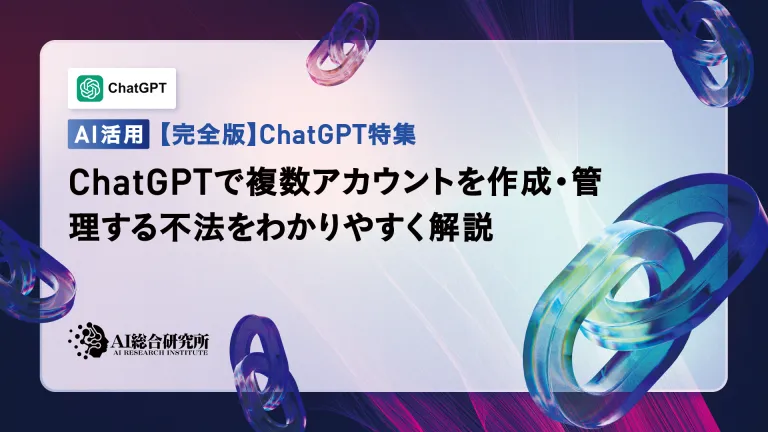
Efficient multiple account management techniques using ChatGPT | A thorough explanation of how to use business and private life! ChatGPT is used in a variety of situations, but some people may be worried about managing multiple accounts. This article will explain in detail how to create multiple accounts for ChatGPT, what to do when using it, and how to operate it safely and efficiently. We also cover important points such as the difference in business and private use, and complying with OpenAI's terms of use, and provide a guide to help you safely utilize multiple accounts. OpenAI


Hot AI Tools

Undresser.AI Undress
AI-powered app for creating realistic nude photos

AI Clothes Remover
Online AI tool for removing clothes from photos.

Undress AI Tool
Undress images for free

Clothoff.io
AI clothes remover

Video Face Swap
Swap faces in any video effortlessly with our completely free AI face swap tool!

Hot Article

Hot Tools

Safe Exam Browser
Safe Exam Browser is a secure browser environment for taking online exams securely. This software turns any computer into a secure workstation. It controls access to any utility and prevents students from using unauthorized resources.
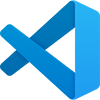
VSCode Windows 64-bit Download
A free and powerful IDE editor launched by Microsoft

MantisBT
Mantis is an easy-to-deploy web-based defect tracking tool designed to aid in product defect tracking. It requires PHP, MySQL and a web server. Check out our demo and hosting services.

SAP NetWeaver Server Adapter for Eclipse
Integrate Eclipse with SAP NetWeaver application server.

SecLists
SecLists is the ultimate security tester's companion. It is a collection of various types of lists that are frequently used during security assessments, all in one place. SecLists helps make security testing more efficient and productive by conveniently providing all the lists a security tester might need. List types include usernames, passwords, URLs, fuzzing payloads, sensitive data patterns, web shells, and more. The tester can simply pull this repository onto a new test machine and he will have access to every type of list he needs.
