This article explores MongoDB's embedded documents and arrays. It discusses creating, querying, and updating nested fields, comparing performance implications of embedding vs. referencing, and offering schema design best practices for optimal effic
Working with Embedded Documents and Arrays in MongoDB
MongoDB's flexibility shines through its support for embedded documents and arrays. Embedded documents are documents nested within another document, while arrays hold a list of documents or values. Let's explore how to use them.
Creating and Using Embedded Documents: Embedded documents are ideal when the related data is small and always accessed together. Consider a users
collection where each user has an address. Instead of having a separate addresses
collection and referencing it, you can embed the address directly within the user document:
{ "_id": ObjectId("..."), "name": "John Doe", "email": "john.doe@example.com", "address": { "street": "123 Main St", "city": "Anytown", "zip": "12345" } }
You can access the embedded document using dot notation in your queries: db.users.find({ "address.city": "Anytown" })
. You can also embed arrays of documents within documents. For example, a user might have multiple phone numbers:
{ "_id": ObjectId("..."), "name": "Jane Doe", "email": "jane.doe@example.com", "phones": [ { "type": "home", "number": "555-1212" }, { "type": "mobile", "number": "555-3434" } ] }
Creating and Using Arrays: Arrays are straightforward to use. You can add, remove, and update elements directly using update operators like $push
, $pull
, and $set
. For instance, adding a new phone number:
db.users.updateOne( { "_id": ObjectId("...") }, { $push: { "phones": { "type": "work", "number": "555-5656" } } } )
Performance Implications of Embedded vs. Referenced Documents
The choice between embedding and referencing significantly impacts performance. Embedding is generally faster for reads, especially when you frequently need the related data. It reduces the number of database queries needed because all the information is in a single document. However, embedding can lead to larger document sizes, potentially impacting write performance and storage costs, particularly if the embedded data is large or frequently updated.
Referencing, on the other hand, involves creating separate collections for related data and linking them using object IDs. This is better for large, frequently updated datasets. Reads become slightly slower as they require multiple queries, but writes are typically faster and more efficient because documents remain smaller. Referencing also helps avoid data duplication and promotes data normalization. The best approach depends on the specific use case and data characteristics. Consider the data size, update frequency, and query patterns when making this decision.
Efficiently Querying and Updating Nested Fields
Querying and updating nested fields requires using the dot notation we saw earlier. For example, to update a specific phone number:
db.users.updateOne( { "_id": ObjectId("..."), "phones.type": "mobile" }, { $set: { "phones.$.number": "555-9876" } } )
The $
operator targets the specific array element matching the query. For more complex queries or updates involving arrays, consider using aggregation pipelines. Aggregation provides powerful tools for processing and transforming data, including nested fields. For example, you could use $unwind
to deconstruct an array into individual documents, making it easier to filter and update specific elements. Remember to use indexes appropriately on nested fields to improve query performance. Indexes on nested fields are created using dot notation in the createIndex
command.
Best Practices for Schema Design
Designing a scalable and maintainable schema with embedded documents and arrays requires careful consideration.
- Data Locality: Embed documents only if they are small and always accessed with their parent document. Large or frequently updated data should be referenced.
- Data Duplication: Avoid excessive data duplication. Referencing helps minimize this.
- Data Size: Keep documents within a reasonable size (generally under 16MB). Large documents can negatively impact performance.
- Update Frequency: Frequently updated data is better suited for referencing to minimize write contention.
- Query Patterns: Analyze your application's query patterns to determine the optimal embedding/referencing strategy. If you frequently query for related data together, embedding is usually beneficial.
- Normalization: While MongoDB is flexible, consider some level of normalization to maintain data integrity and avoid redundancy.
- Indexing: Use indexes strategically on frequently queried nested fields to improve query performance.
- Schema Validation: Implement schema validation to ensure data consistency and quality. This can be done using tools like MongoDB's schema validation features or custom validation logic within your application.
By following these best practices, you can create a MongoDB schema that is efficient, scalable, and easy to maintain. Remember that the optimal approach depends heavily on the specific needs of your application.
The above is the detailed content of How do I work with embedded documents and arrays in MongoDB?. For more information, please follow other related articles on the PHP Chinese website!
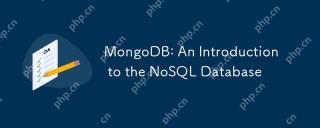
MongoDB is a document-based NoSQL database that uses BSON format to store data, suitable for processing complex and unstructured data. 1) Its document model is flexible and suitable for frequently changing data structures. 2) MongoDB uses WiredTiger storage engine and query optimizer to support efficient data operations and queries. 3) Basic operations include inserting, querying, updating and deleting documents. 4) Advanced usage includes using an aggregation framework for complex data analysis. 5) Common errors include connection problems, query performance problems, and data consistency problems. 6) Performance optimization and best practices include index optimization, data modeling, sharding, caching, monitoring and tuning.
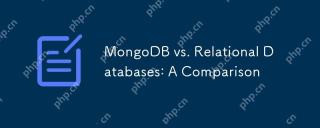
MongoDB is suitable for scenarios that require flexible data models and high scalability, while relational databases are more suitable for applications that complex queries and transaction processing. 1) MongoDB's document model adapts to the rapid iterative modern application development. 2) Relational databases support complex queries and financial systems through table structure and SQL. 3) MongoDB achieves horizontal scaling through sharding, which is suitable for large-scale data processing. 4) Relational databases rely on vertical expansion and are suitable for scenarios where queries and indexes need to be optimized.
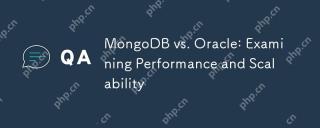
MongoDB performs excellent in performance and scalability, suitable for high scalability and flexibility requirements; Oracle performs excellent in requiring strict transaction control and complex queries. 1.MongoDB achieves high scalability through sharding technology, suitable for large-scale data and high concurrency scenarios. 2. Oracle relies on optimizers and parallel processing to improve performance, suitable for structured data and transaction control needs.
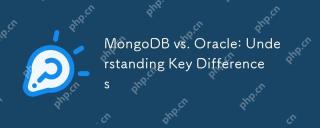
MongoDB is suitable for handling large-scale unstructured data, and Oracle is suitable for enterprise-level applications that require transaction consistency. 1.MongoDB provides flexibility and high performance, suitable for processing user behavior data. 2. Oracle is known for its stability and powerful functions and is suitable for financial systems. 3.MongoDB uses document models, and Oracle uses relational models. 4.MongoDB is suitable for social media applications, while Oracle is suitable for enterprise-level applications.
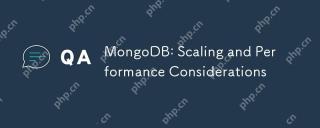
MongoDB's scalability and performance considerations include horizontal scaling, vertical scaling, and performance optimization. 1. Horizontal expansion is achieved through sharding technology to improve system capacity. 2. Vertical expansion improves performance by increasing hardware resources. 3. Performance optimization is achieved through rational design of indexes and optimized query strategies.
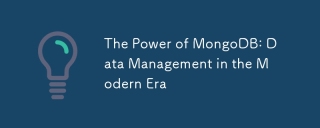
MongoDB is a NoSQL database because of its flexibility and scalability are very important in modern data management. It uses document storage, is suitable for processing large-scale, variable data, and provides powerful query and indexing capabilities.
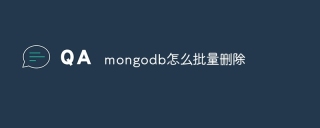
You can use the following methods to delete documents in MongoDB: 1. The $in operator specifies the list of documents to be deleted; 2. The regular expression matches documents that meet the criteria; 3. The $exists operator deletes documents with the specified fields; 4. The find() and remove() methods first get and then delete the document. Please note that these operations cannot use transactions and may delete all matching documents, so be careful when using them.
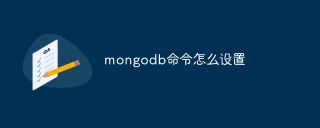
To set up a MongoDB database, you can use the command line (use and db.createCollection()) or the mongo shell (mongo, use and db.createCollection()). Other setting options include viewing database (show dbs), viewing collections (show collections), deleting database (db.dropDatabase()), deleting collections (db.<collection_name>.drop()), inserting documents (db.<collecti


Hot AI Tools

Undresser.AI Undress
AI-powered app for creating realistic nude photos

AI Clothes Remover
Online AI tool for removing clothes from photos.

Undress AI Tool
Undress images for free

Clothoff.io
AI clothes remover

AI Hentai Generator
Generate AI Hentai for free.

Hot Article

Hot Tools

Notepad++7.3.1
Easy-to-use and free code editor
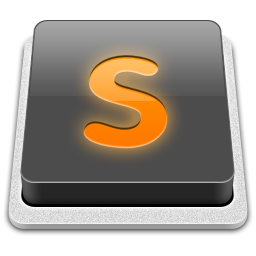
SublimeText3 Mac version
God-level code editing software (SublimeText3)
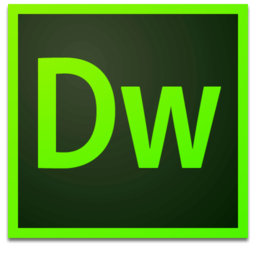
Dreamweaver Mac version
Visual web development tools
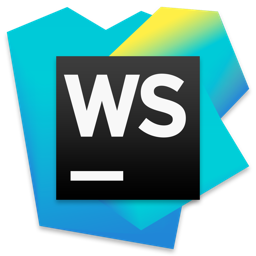
WebStorm Mac version
Useful JavaScript development tools

Zend Studio 13.0.1
Powerful PHP integrated development environment