Optimizing Algorithms for Performance in Go
This question delves into the core of efficient Go programming. Optimizing algorithms for performance in Go involves a multi-faceted approach, focusing on both the algorithm's design and its implementation within the Go language's specific characteristics. The key is to minimize unnecessary computations and memory allocations. Here's a breakdown of strategies:
- Choose the right algorithm: The foundation of performance lies in selecting an algorithm with the optimal time and space complexity for your specific problem. For example, using a binary search on a sorted array is significantly faster than a linear search. Understanding Big O notation (O(n), O(log n), O(n^2), etc.) is crucial for making informed decisions.
- Data Structures: The choice of data structure significantly impacts performance. For instance, using a map (hash table) for fast lookups is preferable to iterating through a slice if you need to frequently access elements by key. Consider the trade-offs between different data structures in terms of insertion, deletion, and search times.
- Minimize allocations: Go's garbage collector is efficient, but frequent allocations can still cause performance issues. Reusing buffers and avoiding unnecessary allocations, particularly within loops, can dramatically improve performance. Techniques like object pooling can be helpful in scenarios with high object churn.
- Avoid unnecessary computations: Identify and eliminate redundant calculations. Memoization, caching frequently accessed results, and loop unrolling (in appropriate cases) can significantly reduce computational overhead.
- Concurrency: Go's concurrency features (goroutines and channels) can be leveraged to parallelize computations and improve performance, especially for I/O-bound or CPU-bound tasks. However, be mindful of the overhead introduced by concurrency and ensure that the gains outweigh the costs.
Common Go Performance Bottlenecks and How to Identify Them
Several common bottlenecks can hinder the performance of Go applications. Identifying them is crucial for targeted optimization.
- Garbage Collection: Excessive garbage collection pauses can significantly impact responsiveness. This often stems from frequent memory allocations. Profiling tools (discussed later) can highlight areas with high allocation rates.
-
I/O Operations: Slow I/O (disk, network) can be a major bottleneck. Asynchronous I/O operations, using techniques like
net/http
's non-blocking features, can mitigate this. - Inefficient Algorithms: Using algorithms with poor time complexity (e.g., O(n^2) for large datasets) is a primary source of performance issues. Profiling and algorithmic analysis are essential for identifying these.
- Context Switching: Excessive context switching between goroutines can introduce overhead. Careful design of concurrent programs, avoiding excessive goroutine creation and using appropriate synchronization primitives, is important.
- Unoptimized Data Structures: Using inappropriate data structures (e.g., using a slice for frequent lookups instead of a map) leads to performance degradation.
Identifying Bottlenecks: The pprof
tool (part of the Go standard library) is invaluable for profiling Go applications. It allows you to analyze CPU usage, memory allocation, and blocking profiles to pinpoint performance hotspots. Using benchmarks (testing
package) is also crucial for quantifying performance improvements after optimizations.
Profiling Go Code to Pinpoint Areas for Algorithmic Optimization
The pprof
tool is the key to profiling Go code for algorithmic optimization. It provides several profiling modes:
- CPU Profiling: This identifies functions consuming the most CPU time. High CPU usage in specific functions often points to inefficient algorithms or computations within those functions.
- Memory Profiling: This highlights areas with high memory allocation rates. Excessive allocations can lead to increased garbage collection pauses and decreased performance. It helps identify potential areas where memory reuse or more efficient data structures could be beneficial.
- Blocking Profiling: This reveals goroutines that are blocked waiting for resources (e.g., I/O, mutexes). It helps in identifying concurrency bottlenecks.
Using pprof: You can instrument your code to generate profile data, then use the pprof
command-line tool to analyze the data. Visualizing the profiles using tools like go tool pprof
(command-line) or web-based profilers provides a clear view of performance bottlenecks. Focus on functions consuming a disproportionate amount of CPU time or allocating excessive memory – these are prime candidates for algorithmic optimization.
Best Practices for Writing Efficient Algorithms in Go
Several best practices contribute to writing efficient algorithms in Go:
- Use appropriate data structures: Select data structures based on their time complexity for your specific operations (e.g., maps for fast lookups, slices for ordered sequences).
- Minimize allocations: Reuse buffers, avoid unnecessary allocations within loops, and consider object pooling for frequently created objects.
- Optimize for the common case: Focus on optimizing the most frequently executed parts of your code. Profiling can help identify these hotspots.
- Write clear and concise code: Clean code is easier to understand and optimize. Avoid unnecessary complexity.
- Use built-in functions: Go's standard library provides highly optimized functions for many common tasks. Leverage these whenever possible.
-
Benchmark your code: Use the
testing
package's benchmarking capabilities to measure the performance of your algorithms and track improvements after optimizations. - Profile regularly: Profiling is an iterative process. Regular profiling helps identify new bottlenecks as your code evolves.
By following these best practices and utilizing Go's profiling tools, you can write efficient and high-performing algorithms. Remember that optimization is an iterative process; continuous profiling and refinement are key to achieving optimal performance.
The above is the detailed content of How do I optimize algorithms for performance in Go?. For more information, please follow other related articles on the PHP Chinese website!
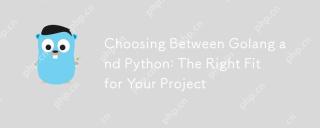
Golangisidealforperformance-criticalapplicationsandconcurrentprogramming,whilePythonexcelsindatascience,rapidprototyping,andversatility.1)Forhigh-performanceneeds,chooseGolangduetoitsefficiencyandconcurrencyfeatures.2)Fordata-drivenprojects,Pythonisp
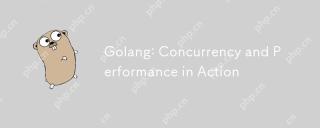
Golang achieves efficient concurrency through goroutine and channel: 1.goroutine is a lightweight thread, started with the go keyword; 2.channel is used for secure communication between goroutines to avoid race conditions; 3. The usage example shows basic and advanced usage; 4. Common errors include deadlocks and data competition, which can be detected by gorun-race; 5. Performance optimization suggests reducing the use of channel, reasonably setting the number of goroutines, and using sync.Pool to manage memory.
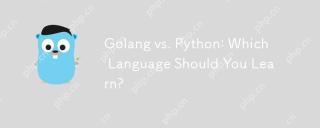
Golang is more suitable for system programming and high concurrency applications, while Python is more suitable for data science and rapid development. 1) Golang is developed by Google, statically typing, emphasizing simplicity and efficiency, and is suitable for high concurrency scenarios. 2) Python is created by Guidovan Rossum, dynamically typed, concise syntax, wide application, suitable for beginners and data processing.
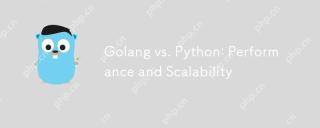
Golang is better than Python in terms of performance and scalability. 1) Golang's compilation-type characteristics and efficient concurrency model make it perform well in high concurrency scenarios. 2) Python, as an interpreted language, executes slowly, but can optimize performance through tools such as Cython.
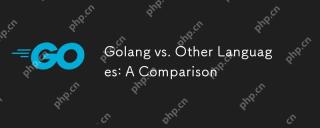
Go language has unique advantages in concurrent programming, performance, learning curve, etc.: 1. Concurrent programming is realized through goroutine and channel, which is lightweight and efficient. 2. The compilation speed is fast and the operation performance is close to that of C language. 3. The grammar is concise, the learning curve is smooth, and the ecosystem is rich.
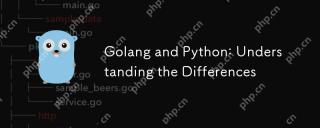
The main differences between Golang and Python are concurrency models, type systems, performance and execution speed. 1. Golang uses the CSP model, which is suitable for high concurrent tasks; Python relies on multi-threading and GIL, which is suitable for I/O-intensive tasks. 2. Golang is a static type, and Python is a dynamic type. 3. Golang compiled language execution speed is fast, and Python interpreted language development is fast.
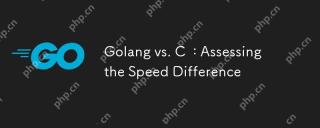
Golang is usually slower than C, but Golang has more advantages in concurrent programming and development efficiency: 1) Golang's garbage collection and concurrency model makes it perform well in high concurrency scenarios; 2) C obtains higher performance through manual memory management and hardware optimization, but has higher development complexity.
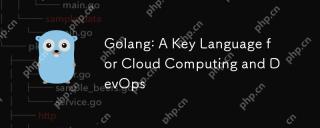
Golang is widely used in cloud computing and DevOps, and its advantages lie in simplicity, efficiency and concurrent programming capabilities. 1) In cloud computing, Golang efficiently handles concurrent requests through goroutine and channel mechanisms. 2) In DevOps, Golang's fast compilation and cross-platform features make it the first choice for automation tools.


Hot AI Tools

Undresser.AI Undress
AI-powered app for creating realistic nude photos

AI Clothes Remover
Online AI tool for removing clothes from photos.

Undress AI Tool
Undress images for free

Clothoff.io
AI clothes remover

Video Face Swap
Swap faces in any video effortlessly with our completely free AI face swap tool!

Hot Article

Hot Tools

SublimeText3 English version
Recommended: Win version, supports code prompts!

Safe Exam Browser
Safe Exam Browser is a secure browser environment for taking online exams securely. This software turns any computer into a secure workstation. It controls access to any utility and prevents students from using unauthorized resources.
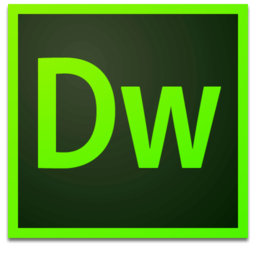
Dreamweaver Mac version
Visual web development tools
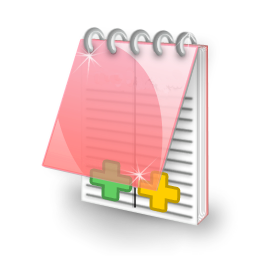
EditPlus Chinese cracked version
Small size, syntax highlighting, does not support code prompt function
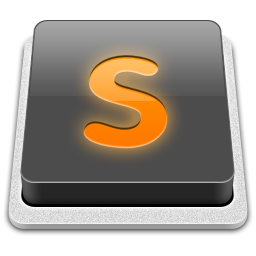
SublimeText3 Mac version
God-level code editing software (SublimeText3)