What are Python Lists and How Do I Use Them Effectively?
Python lists are ordered, mutable (changeable) sequences of items. This means that:
- Ordered: The items in a list maintain their order of insertion. The first element added will always be at index 0, the second at index 1, and so on.
- Mutable: You can change the contents of a list after it's created—you can add, remove, or modify elements. This contrasts with other sequence types like tuples (which are immutable).
- Sequences: Lists are a type of sequence, meaning you can access individual elements using their index (position).
How to use them effectively:
-
Creating Lists: Lists are created using square brackets
[]
, with items separated by commas:my_list = [1, 2, "hello", 3.14, True] empty_list = []
-
Accessing Elements: Use indexing to access elements. Remember that indexing starts at 0:
first_element = my_list[0] # 1 third_element = my_list[2] # "hello"
Negative indexing allows access from the end:
last_element = my_list[-1] # True
-
Slicing: Extract portions of a list:
sublist = my_list[1:4] # [2, "hello", 3.14] (elements from index 1 up to, but not including, 4)
-
List Methods: Python provides many built-in methods for list manipulation:
-
append(item)
: Adds an item to the end. -
insert(index, item)
: Inserts an item at a specific index. -
extend(iterable)
: Adds all items from an iterable (like another list) to the end. -
remove(item)
: Removes the first occurrence of an item. -
pop([index])
: Removes and returns the item at a specific index (default is the last element). -
del my_list[index]
: Deletes an item at a specific index. -
index(item)
: Returns the index of the first occurrence of an item. -
count(item)
: Counts the number of times an item appears. -
sort()
: Sorts the list in place. -
reverse()
: Reverses the order of elements in place. -
copy()
: Creates a shallow copy of the list.
-
What are the common pitfalls to avoid when working with Python lists?
-
Modifying a list while iterating: This can lead to unexpected behavior or errors. It's generally safer to iterate over a copy of the list or use list comprehensions.
my_list = [1, 2, "hello", 3.14, True] empty_list = []
-
Incorrect indexing: Accessing elements outside the list's bounds (e.g.,
my_list[10]
when the list only has 5 elements) will raise anIndexError
. -
Shallow copies vs. deep copies: When you create a copy using
my_list_copy = my_list
, you're creating a shallow copy. Changes to elements within the copied list will also affect the original list if those elements are mutable objects (like other lists). Use thecopy()
method or thecopy.deepcopy()
function from thecopy
module for deep copies to avoid this. -
Inefficient operations on large lists: Operations like
append()
are relatively efficient, but repeated insertions or deletions in the middle of a large list can be slow. Consider using more efficient data structures (likecollections.deque
) for certain tasks. -
Not checking for empty lists: Before performing operations that assume the list has elements (like accessing
my_list[0]
), always check if the list is empty usingif not my_list:
.
How do Python lists compare to other data structures like tuples and sets?
Feature | List | Tuple | Set |
---|---|---|---|
Mutability | Mutable | Immutable | Mutable |
Ordering | Ordered | Ordered | Unordered |
Duplicates | Allowed | Allowed | Not allowed |
Syntax | [item1, item2, ...] |
(item1, item2, ...) |
{item1, item2, ...} |
Use Cases | Collections of items that might change | Representing fixed collections of items | Unique items, membership testing |
In short:
- Lists: Use when you need an ordered collection that can be modified.
- Tuples: Use when you need an ordered collection that should not be changed (for data integrity). They are also slightly more memory-efficient than lists.
- Sets: Use when you need a collection of unique items and order doesn't matter. Set operations (union, intersection, etc.) are highly efficient.
What are some advanced techniques for manipulating and optimizing Python lists for large datasets?
-
List comprehensions: These provide a concise way to create new lists based on existing ones, often significantly faster than explicit loops.
my_list = [1, 2, "hello", 3.14, True] empty_list = []
-
Generator expressions: Similar to list comprehensions, but they generate values on demand instead of creating the entire list in memory at once. This is crucial for very large datasets that won't fit in memory.
first_element = my_list[0] # 1 third_element = my_list[2] # "hello"
- NumPy arrays: For numerical computations on large datasets, NumPy arrays are far more efficient than Python lists. They offer vectorized operations and optimized memory management.
- Memory mapping: For extremely large datasets that exceed available RAM, memory mapping allows you to work with parts of a file on disk as if they were in memory.
-
Specialized data structures: Consider using data structures from the
collections
module (likedeque
for efficient appends and pops from both ends) or other libraries depending on the specific operations you're performing. - Profiling: Use Python's profiling tools to identify bottlenecks in your code. This will help you target optimization efforts effectively.
By understanding these techniques and avoiding common pitfalls, you can work effectively with Python lists, even when dealing with substantial amounts of data.
The above is the detailed content of What are Python Lists and How Do I Use Them Effectively?. For more information, please follow other related articles on the PHP Chinese website!
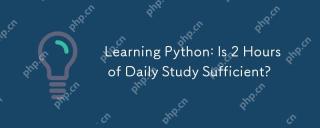
Is it enough to learn Python for two hours a day? It depends on your goals and learning methods. 1) Develop a clear learning plan, 2) Select appropriate learning resources and methods, 3) Practice and review and consolidate hands-on practice and review and consolidate, and you can gradually master the basic knowledge and advanced functions of Python during this period.
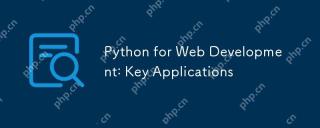
Key applications of Python in web development include the use of Django and Flask frameworks, API development, data analysis and visualization, machine learning and AI, and performance optimization. 1. Django and Flask framework: Django is suitable for rapid development of complex applications, and Flask is suitable for small or highly customized projects. 2. API development: Use Flask or DjangoRESTFramework to build RESTfulAPI. 3. Data analysis and visualization: Use Python to process data and display it through the web interface. 4. Machine Learning and AI: Python is used to build intelligent web applications. 5. Performance optimization: optimized through asynchronous programming, caching and code
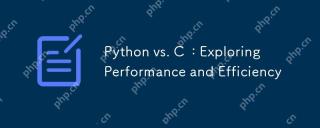
Python is better than C in development efficiency, but C is higher in execution performance. 1. Python's concise syntax and rich libraries improve development efficiency. 2.C's compilation-type characteristics and hardware control improve execution performance. When making a choice, you need to weigh the development speed and execution efficiency based on project needs.
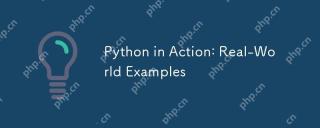
Python's real-world applications include data analytics, web development, artificial intelligence and automation. 1) In data analysis, Python uses Pandas and Matplotlib to process and visualize data. 2) In web development, Django and Flask frameworks simplify the creation of web applications. 3) In the field of artificial intelligence, TensorFlow and PyTorch are used to build and train models. 4) In terms of automation, Python scripts can be used for tasks such as copying files.
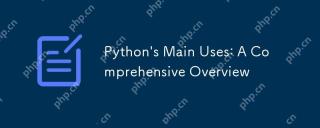
Python is widely used in data science, web development and automation scripting fields. 1) In data science, Python simplifies data processing and analysis through libraries such as NumPy and Pandas. 2) In web development, the Django and Flask frameworks enable developers to quickly build applications. 3) In automated scripts, Python's simplicity and standard library make it ideal.
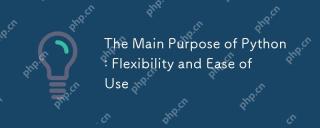
Python's flexibility is reflected in multi-paradigm support and dynamic type systems, while ease of use comes from a simple syntax and rich standard library. 1. Flexibility: Supports object-oriented, functional and procedural programming, and dynamic type systems improve development efficiency. 2. Ease of use: The grammar is close to natural language, the standard library covers a wide range of functions, and simplifies the development process.
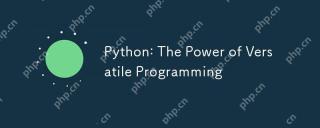
Python is highly favored for its simplicity and power, suitable for all needs from beginners to advanced developers. Its versatility is reflected in: 1) Easy to learn and use, simple syntax; 2) Rich libraries and frameworks, such as NumPy, Pandas, etc.; 3) Cross-platform support, which can be run on a variety of operating systems; 4) Suitable for scripting and automation tasks to improve work efficiency.
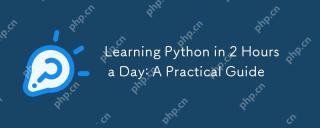
Yes, learn Python in two hours a day. 1. Develop a reasonable study plan, 2. Select the right learning resources, 3. Consolidate the knowledge learned through practice. These steps can help you master Python in a short time.


Hot AI Tools

Undresser.AI Undress
AI-powered app for creating realistic nude photos

AI Clothes Remover
Online AI tool for removing clothes from photos.

Undress AI Tool
Undress images for free

Clothoff.io
AI clothes remover

AI Hentai Generator
Generate AI Hentai for free.

Hot Article

Hot Tools

MinGW - Minimalist GNU for Windows
This project is in the process of being migrated to osdn.net/projects/mingw, you can continue to follow us there. MinGW: A native Windows port of the GNU Compiler Collection (GCC), freely distributable import libraries and header files for building native Windows applications; includes extensions to the MSVC runtime to support C99 functionality. All MinGW software can run on 64-bit Windows platforms.
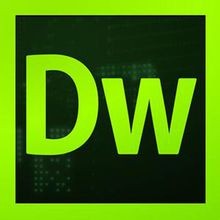
Dreamweaver CS6
Visual web development tools
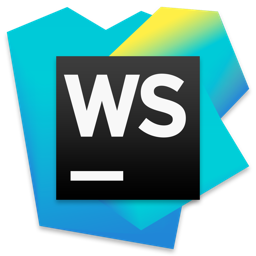
WebStorm Mac version
Useful JavaScript development tools
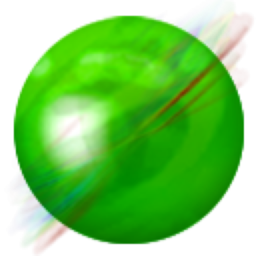
ZendStudio 13.5.1 Mac
Powerful PHP integrated development environment

Notepad++7.3.1
Easy-to-use and free code editor