Analyzing customer feedback and identifying key themes in textual data is traditionally a laborious process. It involves data gathering, manual labeling, and the fine-tuning of specialized models. Zero-shot text classification, however, offers a streamlined approach, leveraging the power of Large Language Models (LLMs) to bypass the need for extensive model training. This article explores how zero-shot classification simplifies sentiment analysis using the SKLLM library (combining scikit-learn and LLMs), demonstrating its application on the Kaggle Women’s E-Commerce Clothing Reviews dataset.
Key Learning Outcomes
This tutorial will cover:
- The conventional sentiment analysis workflow and its limitations.
- The principles and benefits of zero-shot text classification with LLMs.
- An introduction to the SKLLM library and its integration with scikit-learn.
- Practical application of zero-shot classification to the Women’s E-Commerce Clothing Reviews dataset.
- Hands-on experience with zero-shot classification for real-world scenarios.
*This article is part of the***Data Science Blogathon.
Table of contents
- What is Zero-Shot Text Classification?
- Why is Zero-Shot So Efficient?
- Dataset Overview
- Step-by-Step Guide
- Potential Drawbacks
- Few-Shot Text Classification
- Chain-of-Thought Text Classification
- Summary
- Frequently Asked Questions
What is Zero-Shot Text Classification?
Analyzing the large volume of customer reviews received by online retailers presents a significant challenge for efficient sentiment analysis and theme identification. Traditional methods involve:
- Gathering and cleaning review data.
- Manually labeling thousands of samples (e.g., "positive," "negative," "neutral").
- Fine-tuning a specialized classification model using this labeled data.
This process is time-consuming and resource-intensive. Zero-shot text classification offers a solution: using LLMs directly to classify text without the need for custom training. By providing descriptive labels (e.g., "positive," "negative," "neutral"), the model infers the correct class.
Why is Zero-Shot So Efficient?
The efficiency of zero-shot classification stems from:
- Elimination of Fine-Tuning: The costly process of fine-tuning LLMs like GPT-4 is avoided. Pre-trained LLMs are used directly, providing immediate high-quality classification.
- Easy Label Adaptation: Changing the label set (e.g., from general sentiments to more specific ones) only requires updating the label list; no model retraining is needed.
- Reduced Data Requirements: Unlike supervised learning, zero-shot classification requires only descriptive labels, making it suitable for situations with limited or unlabeled data.
- Faster Deployment: By skipping data annotation and model training, deployment is significantly accelerated.
Dataset Overview
The Women’s E-Commerce Clothing Reviews dataset from Kaggle is used in this tutorial.
[Link to Dataset]
Key dataset characteristics:
- Contains thousands of customer reviews on women's clothing.
- The "Review Text" column contains the main text data.
- Additional metadata ("Title," "Rating," "Recommended IND," etc.) is available but not essential for zero-shot classification.
Step-by-Step Guide
This section details how to perform sentiment analysis and theme detection using zero-shot classification with LLMs and the SKLLM library.
Step 1: Installation and Setup
Ensure Python 3.7 is installed and install SKLLM:
pip install scikit-llm
Obtain a valid API key for an LLM provider (e.g., OpenAI) and set it in your environment:
from skllm.config import SKLLMConfig # Replace with your OpenAI API key SKLLMConfig.set_openai_key("your_openai_api_key")
Step 2: Import Libraries and Load Data
import pandas as pd from skllm.models.gpt.classification.zero_shot import ZeroShotGPTClassifier # Load dataset df = pd.read_csv("Womens Clothing E-Commerce Reviews.csv") # Handle missing review texts df = df.dropna(subset=["Review Text"]).reset_index(drop=True) X = df["Review Text"].tolist()
Step 3: Define Labels
For sentiment classification, use: ["positive", "negative", "neutral"]
. This can be customized as needed.
Step 4: Zero-Shot Classification
Instantiate ZeroShotGPTClassifier
(using gpt-4o
or another suitable model):
clf = ZeroShotGPTClassifier(model="gpt-4o") clf.fit(None, ["positive", "negative", "neutral"])
fit(None, labels)
indicates that no training data is required; the classifier is initialized with the label set.
Step 5: Classify Reviews
predictions = clf.predict(X) for review_text, sentiment in zip(X[:5], predictions[:5]): print(f"Review: {review_text}") print(f"Predicted Sentiment: {sentiment}") print("-" * 50)
This displays the first five reviews and their predicted sentiments.
Results Comparison
Traditional ML approaches require labeling, model training, validation, and continuous updates. Zero-shot significantly reduces this overhead, offering immediate results without labeled data and easy label refinement.
Potential Drawbacks
- Accuracy Fluctuations: Accuracy can vary depending on the complexity of the text and the model's ability to interpret domain-specific jargon.
- Cost Considerations: Using powerful models like GPT-4 incurs API costs.
- Data Privacy: Ensure compliance with data privacy regulations when sending data to external APIs.
Few-Shot Text Classification
Few-shot classification uses a small number of labeled examples per class to guide the model. The SKLLM estimators use the entire training set to create few-shot examples. For large datasets, consider splitting the data and using a small training subset (e.g., no more than 10 examples per class) and shuffling the examples.
pip install scikit-llm
Chain-of-Thought Text Classification
Chain-of-thought classification generates intermediate reasoning steps, potentially improving accuracy but increasing token usage and cost.
from skllm.config import SKLLMConfig # Replace with your OpenAI API key SKLLMConfig.set_openai_key("your_openai_api_key")
Experimenting with few-shot and chain-of-thought approaches may yield better results than the baseline zero-shot method.
Summary
The SKLLM library provides a fast and efficient alternative to building custom sentiment analysis pipelines. Zero-shot classification enables rapid analysis of customer feedback without the need for manual labeling or model training. This is particularly valuable for iterative tasks and label expansion.
Key Points
- Zero-shot classification simplifies sentiment analysis without manual labeling or model training.
- SKLLM integrates scikit-learn with LLMs for efficient text classification.
- LLMs like GPT-4 provide high-quality classification results immediately.
- Zero-shot classification is fast, adaptable, and requires minimal data.
Frequently Asked Questions
Q1. Choosing between zero-shot, few-shot, and chain-of-thought: Zero-shot is ideal for quick prototyping and limited data; few-shot improves accuracy with a small labeled dataset; chain-of-thought enhances performance but increases cost.
Q2. Number of examples for few-shot: Up to 10 examples per class are recommended; shuffle examples to avoid bias.
Q3. Chain-of-thought impact on accuracy: Not guaranteed to improve accuracy; effectiveness depends on task complexity and prompt clarity.
Q4. Cost at scale: Cost depends on token usage, model choice, prompt length, and dataset size. Chain-of-thought increases cost due to longer prompts.
Note: The image used in this article is not owned by the author and is used with permission.
The above is the detailed content of Zero-Shot and Few-Shot Text Classification with SCIKIT-LLM. For more information, please follow other related articles on the PHP Chinese website!
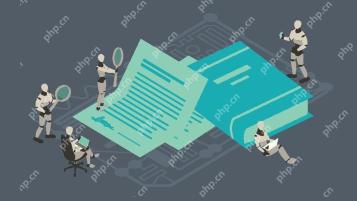
The legal tech revolution is gaining momentum, pushing legal professionals to actively embrace AI solutions. Passive resistance is no longer a viable option for those aiming to stay competitive. Why is Technology Adoption Crucial? Legal professional
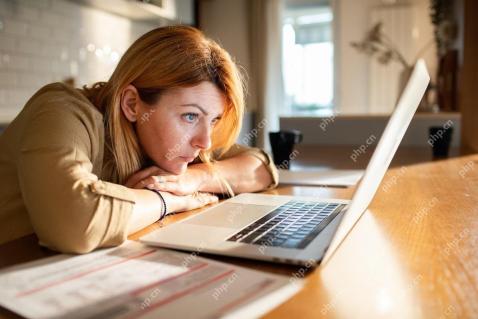
Many assume interactions with AI are anonymous, a stark contrast to human communication. However, AI actively profiles users during every chat. Every prompt, every word, is analyzed and categorized. Let's explore this critical aspect of the AI revo
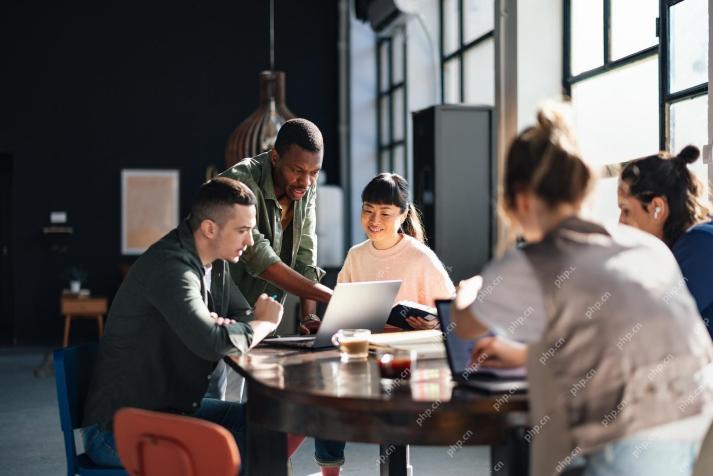
A successful artificial intelligence strategy cannot be separated from strong corporate culture support. As Peter Drucker said, business operations depend on people, and so does the success of artificial intelligence. For organizations that actively embrace artificial intelligence, building a corporate culture that adapts to AI is crucial, and it even determines the success or failure of AI strategies. West Monroe recently released a practical guide to building a thriving AI-friendly corporate culture, and here are some key points: 1. Clarify the success model of AI: First of all, we must have a clear vision of how AI can empower business. An ideal AI operation culture can achieve a natural integration of work processes between humans and AI systems. AI is good at certain tasks, while humans are good at creativity and judgment
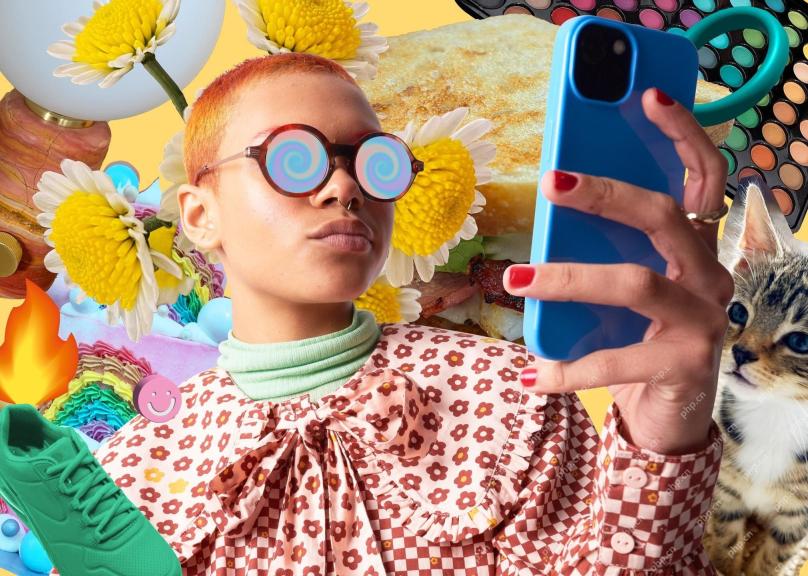
Meta upgrades AI assistant application, and the era of wearable AI is coming! The app, designed to compete with ChatGPT, offers standard AI features such as text, voice interaction, image generation and web search, but has now added geolocation capabilities for the first time. This means that Meta AI knows where you are and what you are viewing when answering your question. It uses your interests, location, profile and activity information to provide the latest situational information that was not possible before. The app also supports real-time translation, which completely changed the AI experience on Ray-Ban glasses and greatly improved its usefulness. The imposition of tariffs on foreign films is a naked exercise of power over the media and culture. If implemented, this will accelerate toward AI and virtual production
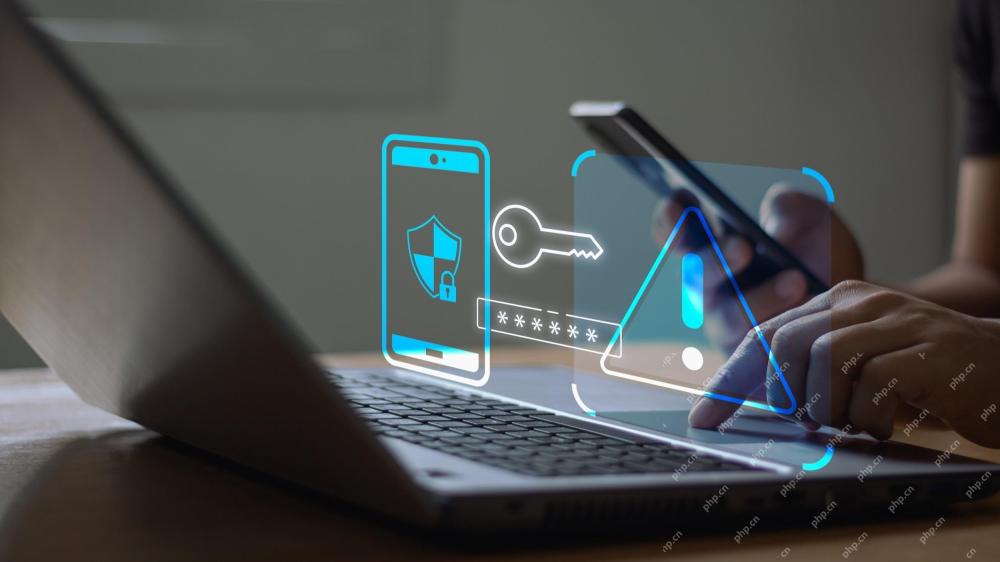
Artificial intelligence is revolutionizing the field of cybercrime, which forces us to learn new defensive skills. Cyber criminals are increasingly using powerful artificial intelligence technologies such as deep forgery and intelligent cyberattacks to fraud and destruction at an unprecedented scale. It is reported that 87% of global businesses have been targeted for AI cybercrime over the past year. So, how can we avoid becoming victims of this wave of smart crimes? Let’s explore how to identify risks and take protective measures at the individual and organizational level. How cybercriminals use artificial intelligence As technology advances, criminals are constantly looking for new ways to attack individuals, businesses and governments. The widespread use of artificial intelligence may be the latest aspect, but its potential harm is unprecedented. In particular, artificial intelligence
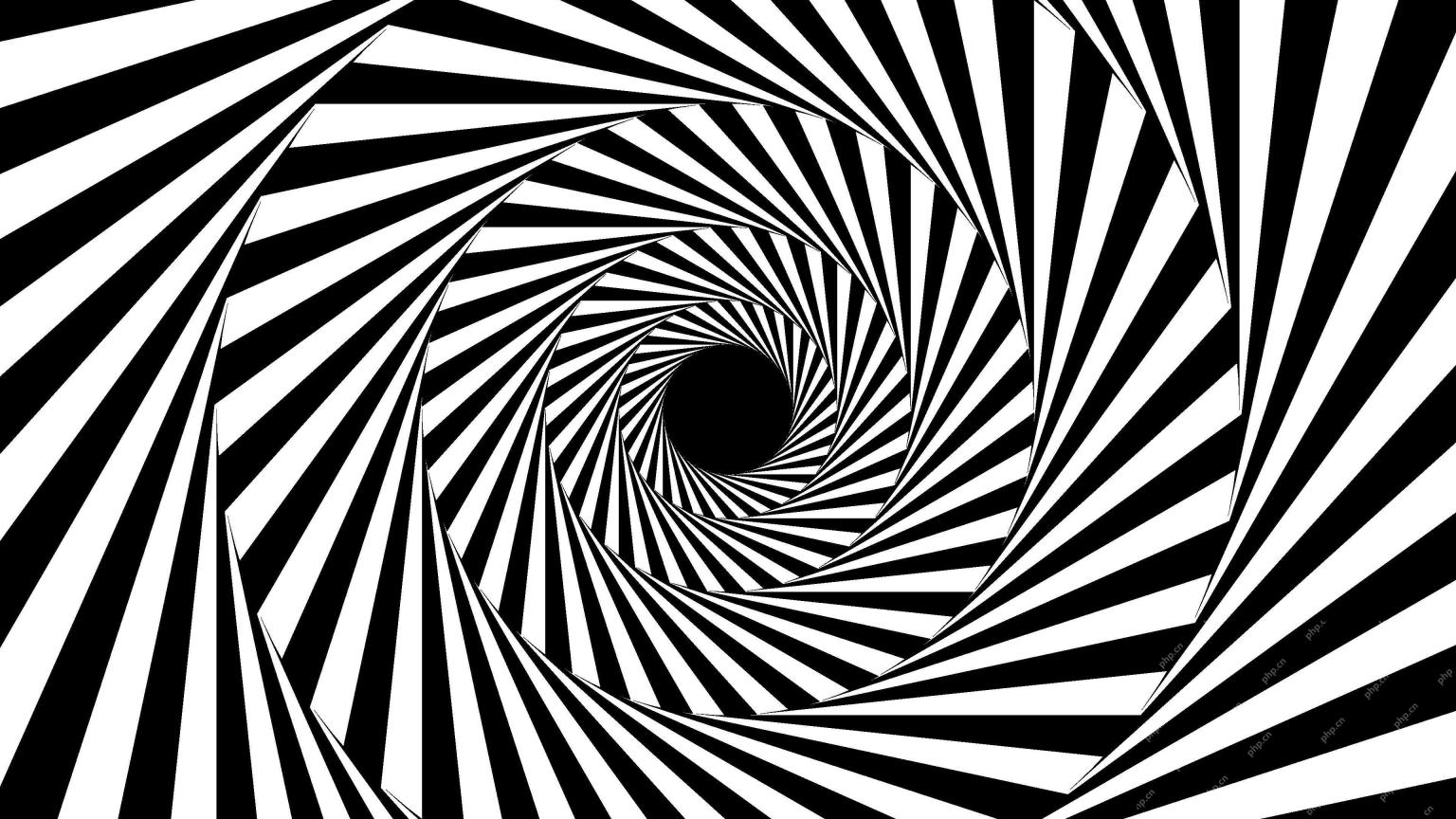
The intricate relationship between artificial intelligence (AI) and human intelligence (NI) is best understood as a feedback loop. Humans create AI, training it on data generated by human activity to enhance or replicate human capabilities. This AI
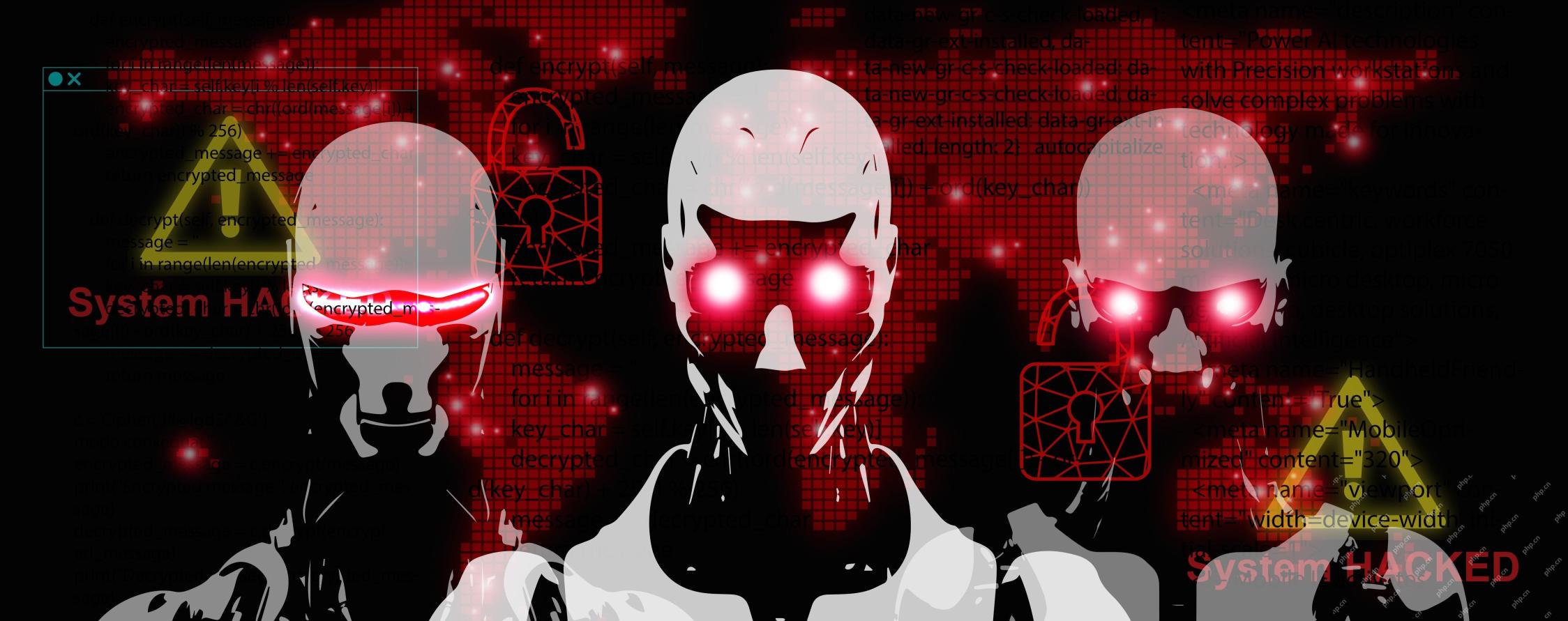
Anthropic's recent statement, highlighting the lack of understanding surrounding cutting-edge AI models, has sparked a heated debate among experts. Is this opacity a genuine technological crisis, or simply a temporary hurdle on the path to more soph
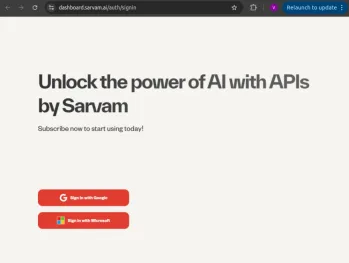
India is a diverse country with a rich tapestry of languages, making seamless communication across regions a persistent challenge. However, Sarvam’s Bulbul-V2 is helping to bridge this gap with its advanced text-to-speech (TTS) t


Hot AI Tools

Undresser.AI Undress
AI-powered app for creating realistic nude photos

AI Clothes Remover
Online AI tool for removing clothes from photos.

Undress AI Tool
Undress images for free

Clothoff.io
AI clothes remover

Video Face Swap
Swap faces in any video effortlessly with our completely free AI face swap tool!

Hot Article

Hot Tools

PhpStorm Mac version
The latest (2018.2.1) professional PHP integrated development tool

SublimeText3 English version
Recommended: Win version, supports code prompts!

mPDF
mPDF is a PHP library that can generate PDF files from UTF-8 encoded HTML. The original author, Ian Back, wrote mPDF to output PDF files "on the fly" from his website and handle different languages. It is slower than original scripts like HTML2FPDF and produces larger files when using Unicode fonts, but supports CSS styles etc. and has a lot of enhancements. Supports almost all languages, including RTL (Arabic and Hebrew) and CJK (Chinese, Japanese and Korean). Supports nested block-level elements (such as P, DIV),

MinGW - Minimalist GNU for Windows
This project is in the process of being migrated to osdn.net/projects/mingw, you can continue to follow us there. MinGW: A native Windows port of the GNU Compiler Collection (GCC), freely distributable import libraries and header files for building native Windows applications; includes extensions to the MSVC runtime to support C99 functionality. All MinGW software can run on 64-bit Windows platforms.
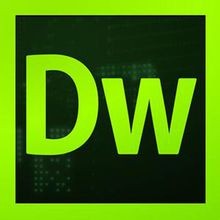
Dreamweaver CS6
Visual web development tools
