


LlamaIndex: A Data Framework for the Large Language Models (LLMs) based applications
LlamaIndex: Data framework that empowers large language models
LlamaIndex is an application data framework based on large language models (LLM). LLMs like GPT-4 pre-train a massive amount of public data sets to provide powerful natural language processing capabilities out of the box. However, their utility will be limited without access to your own private data.
LlamaIndex allows you to ingest data from APIs, databases, PDFs and other sources through flexible data connectors. These data are indexed into intermediate representations optimized for LLM. LlamaIndex then allows natural language query and conversation with your data through a query engine, chat interface, and LLM-driven agent. It enables your LLM to access and interpret private data at scale without retraining the model.
Whether you are a beginner looking for a simple natural language method for querying data, or an advanced user who needs deep customization, LlamaIndex has the corresponding tools. The advanced API allows you to get started with just five-element code, while the low-level API allows you to fully control data ingestion, indexing, retrieval and more.
How does LlamaIndex work
LlamaIndex uses a Retrieval Enhanced Generation (RAG) system that combines large language models with a private knowledge base. It usually consists of two phases: the indexing phase and the query phase.
Pictures are from advanced concepts
Index Phase
During the indexing phase, LlamaIndex will efficiently index private data into vector indexes. This step helps create a searchable knowledge base specific to your field. You can enter text documents, database records, knowledge graphs, and other data types.
Essentially, the index converts the data into a numeric vector or embedding to capture its semantic meaning. It enables quick searches of similarity across content.
Query stage
In the query stage, the RAG pipeline searches for the most relevant information based on the user's query. This information is then provided to the LLM with the query to create an accurate response.
This procedure allows LLM to access current and updated information that may not be included in its initial training.
The main challenge at this stage is to retrieve, organize, and reason about information from multiple knowledge bases that may exist.
Learn more about RAG in our PineCone Retrieval Enhanced Generation Code Sample.
Settings of LlamaIndex
Before we dive into the LlamaIndex tutorials and projects, we have to install the Python package and set up the API.
We can simply install LlamaIndex using pip.
<code>pip install llama-index</code>
By default, LlamaIndex uses the OpenAI GPT-3 text-davinci-003 model. To use this model, you must set OPENAI_API_KEY. You can create a free account and get the API key by logging into OpenAI's new API token.
<code>pip install llama-index</code>
Also, make sure you have installed the openai package.
<code>import os os.environ["OPENAI_API_KEY"] = "INSERT OPENAI KEY"</code>
Add personal data to LLM using LlamaIndex
In this section, we will learn how to create a resume reader using LlamaIndex. You can download your resume by visiting the LinkedIn profile page, clicking "More", and then "Save as PDF".
Please note that we use DataLab to run Python code. You can access all relevant code and output in the LlamaIndex: Add personal data to LLM workbook; you can easily create your own copy to run all your code without installing anything on your computer!
We must install llama-index, openai and pypdf before running anything. We install pypdf so that we can read and convert PDF files.
<code>pip install openai</code>
Load data and create index
We have a directory called "Private-Data" which contains only one PDF file. We will read it using SimpleDirectoryReader and then convert it to index using TreeIndex.
<code>%pip install llama-index openai pypdf</code>
Run query
Once the data is indexed, you can start asking questions by using as_query_engine(). This function allows you to ask questions about specific information in the document and get the corresponding response with the help of the OpenAI GPT-3 text-davinci-003 model.
Note: You can set up the OpenAI API in DataLab following the instructions for using GPT-3.5 and GPT-4 through the OpenAI API in Python tutorial.
As we can see, the LLM model answers the query accurately. It searched for the index and found relevant information.
<code>from llama_index import TreeIndex, SimpleDirectoryReader resume = SimpleDirectoryReader("Private-Data").load_data() new_index = TreeIndex.from_documents(resume)</code>
<code>query_engine = new_index.as_query_engine() response = query_engine.query("When did Abid graduated?") print(response)</code>
We can further ask for certification information. It seems that LlamaIndex has fully understood the candidates, which may be beneficial for companies looking for specific talents.
<code>Abid graduated in February 2014.</code>
<code>response = query_engine.query("What is the name of certification that Abid received?") print(response)</code>
Save and load context
Creating an index is a time-consuming process. We can avoid recreating the index by saving the context. By default, the following command will save the index store stored in the ./storage directory.
<code>Data Scientist Professional</code>
When we are done, we can quickly load the storage context and create an index.
<code>new_index.storage_context.persist()</code>
To verify that it works properly, we will ask the query engine questions in the resume. It seems we have successfully loaded the context.
<code>from llama_index import StorageContext, load_index_from_storage storage_context = StorageContext.from_defaults(persist_) index = load_index_from_storage(storage_context)</code>
<code>query_engine = index.as_query_engine() response = query_engine.query("What is Abid's job title?") print(response)</code>
Chatbot
In addition to Q&A, we can also create personal chatbots using LlamaIndex. We just need to use the as_chat_engine() function to initialize the index.
We will ask a simple question.
<code>Abid's job title is Technical Writer.</code>
<code>query_engine = index.as_chat_engine() response = query_engine.chat("What is the job title of Abid in 2021?") print(response)</code>
and without providing additional context, we will ask follow-up questions.
<code>Abid's job title in 2021 is Data Science Consultant.</code>
<code>response = query_engine.chat("What else did he do during that time?") print(response)</code>
It's obvious that the chat engine runs perfectly.
After building a language application, the next step on your timeline is to read about the pros and cons of using large language models (LLMs) in the cloud versus running them locally. This will help you determine which approach is best for your needs.
Build Wikitext to Speech with LlamaIndex
Our next project involves developing an application that can respond to questions from Wikipedia and convert them into voice.
Code source and additional information can be found in the DataLab workbook.
Website crawling Wikipedia page
First, we will crawl the data from the Italian-Wikipedia webpage and save it as an italy_text.txt file in the data folder.
<code>pip install llama-index</code>
Loading data and building index
Next, we need to install the necessary packages. The elevenlabs package allows us to easily convert text to speech using the API.
<code>import os os.environ["OPENAI_API_KEY"] = "INSERT OPENAI KEY"</code>
By using SimpleDirectoryReader, we will load the data and convert the TXT file to a vector store using VectorStoreIndex.
<code>pip install openai</code>
Query
Our plan is to ask general questions about the country and get a response from LLM query_engine.
<code>%pip install llama-index openai pypdf</code>
Text to voice
After, we will use the llama_index.tts module to access the ElevenLabsTTS api. You need to provide the ElevenLabs API key to enable the audio generation feature. You can get API keys for free on the ElevenLabs website.
<code>from llama_index import TreeIndex, SimpleDirectoryReader resume = SimpleDirectoryReader("Private-Data").load_data() new_index = TreeIndex.from_documents(resume)</code>
We add the response to the generate_audio function to generate natural speech. To listen to the audio, we will use the Audio function of IPython.display.
<code>query_engine = new_index.as_query_engine() response = query_engine.query("When did Abid graduated?") print(response)</code>
This is a simple example. You can use multiple modules to create your assistant, such as Siri, which answers your questions by interpreting your private data. For more information, see the LlamaIndex documentation.
In addition to LlamaIndex, LangChain also allows you to build LLM-based applications. Additionally, you can read the LangChain Getting Started with Data Engineering and Data Applications to learn an overview of what you can do with LangChain, including the issues and data use case examples that LangChain solves.
LlamaIndex Use Cases
LlamaIndex provides a complete toolkit for building language-based applications. Most importantly, you can use the various data loaders and agent tools in Llama Hub to develop complex applications with multiple capabilities.
You can use one or more plugin data loaders to connect a custom data source to your LLM.
Data loader from Llama Hub
You can also use the agent tool to integrate third-party tools and APIs.
Agistrator Tool from Llama Hub
In short, you can build with LlamaIndex:
- Document-based Q&A
- Chatbot
- Agencies
- Structured Data
- Full stack web application
- Private Settings
To learn more about these use cases, visit the LlamaIndex documentation.
Conclusion
LlamaIndex provides a powerful toolkit for building retrieval enhancement generation systems that combine the benefits of large language models and custom knowledge bases. It is able to create an index store of domain-specific data and utilize it during inference to provide relevant context for LLM to generate high-quality responses.
In this tutorial, we learned about LlamaIndex and its working principles. Additionally, we built a resume reader and text-to-speech project using just a few lines of Python code. Creating an LLM application with LlamaIndex is very simple, and it provides a huge library of plugins, data loaders and agents.
To become an expert LLM developer, the next step is to take the large language model concept master course. This course will give you a comprehensive understanding of LLMs, including their applications, training methods, ethical considerations and latest research.
The above is the detailed content of LlamaIndex: A Data Framework for the Large Language Models (LLMs) based applications. For more information, please follow other related articles on the PHP Chinese website!
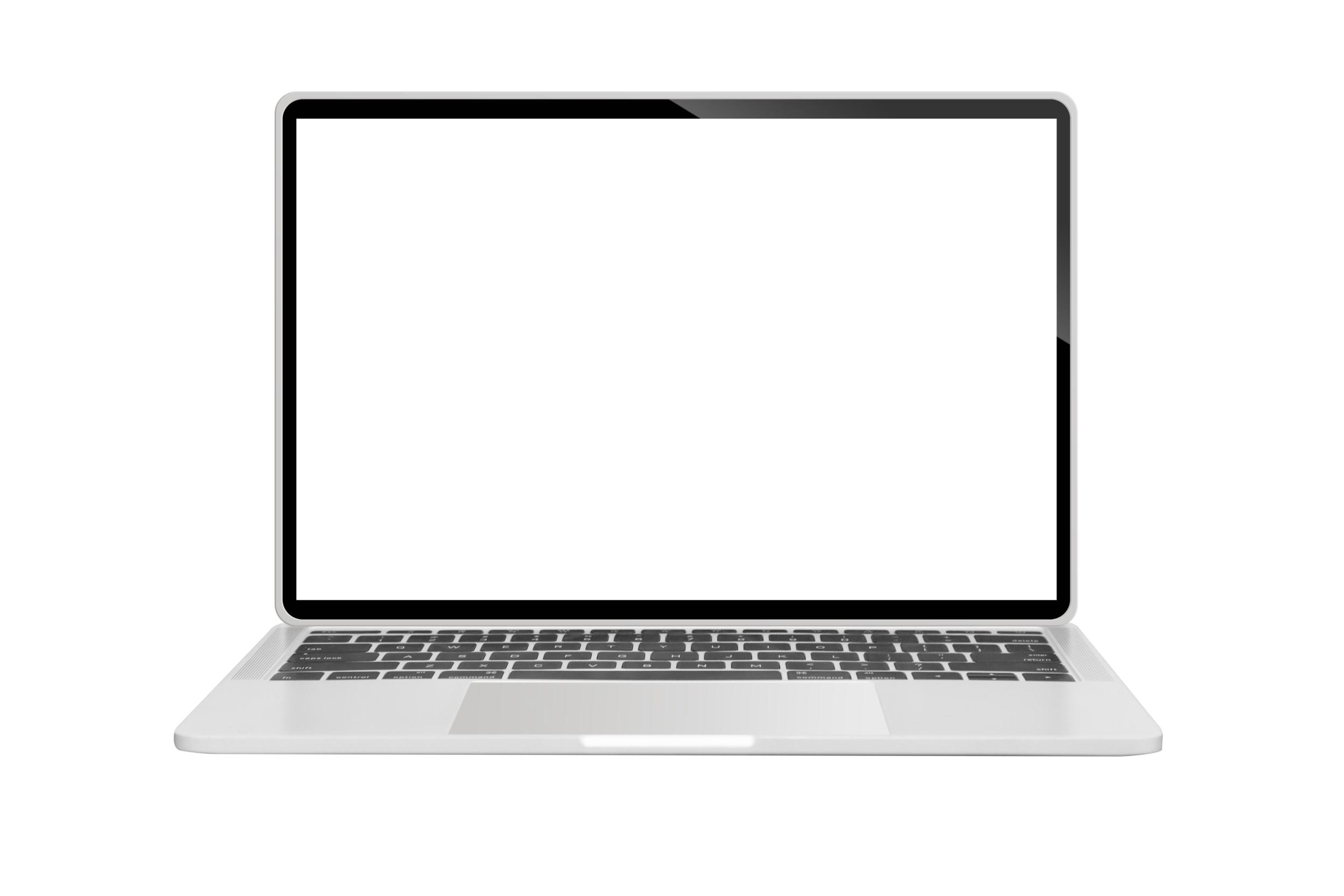
Google is leading this shift. Its "AI Overviews" feature already serves more than one billion users, providing complete answers before anyone clicks a link.[^2] Other players are also gaining ground fast. ChatGPT, Microsoft Copilot, and Pe
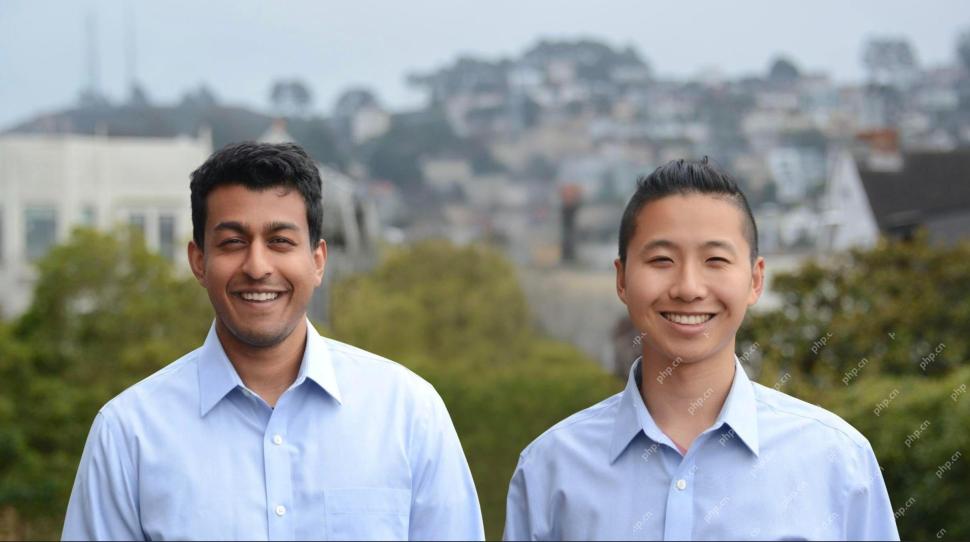
In 2022, he founded social engineering defense startup Doppel to do just that. And as cybercriminals harness ever more advanced AI models to turbocharge their attacks, Doppel’s AI systems have helped businesses combat them at scale— more quickly and
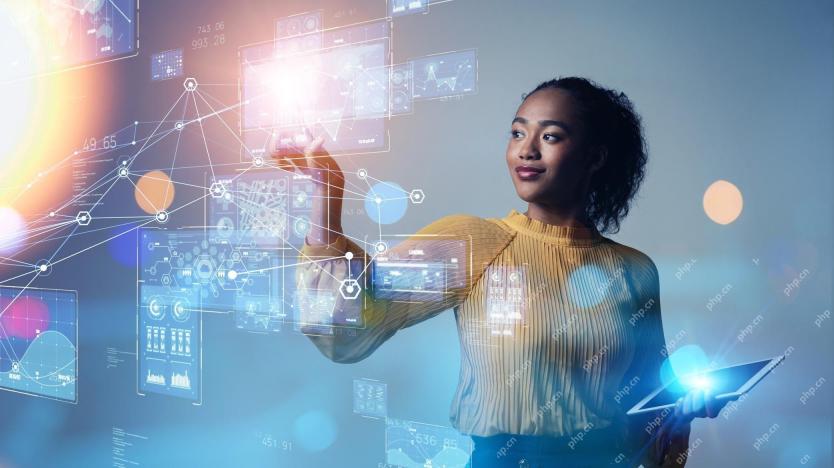
Voila, via interacting with suitable world models, generative AI and LLMs can be substantively boosted. Let’s talk about it. This analysis of an innovative AI breakthrough is part of my ongoing Forbes column coverage on the latest in AI, including
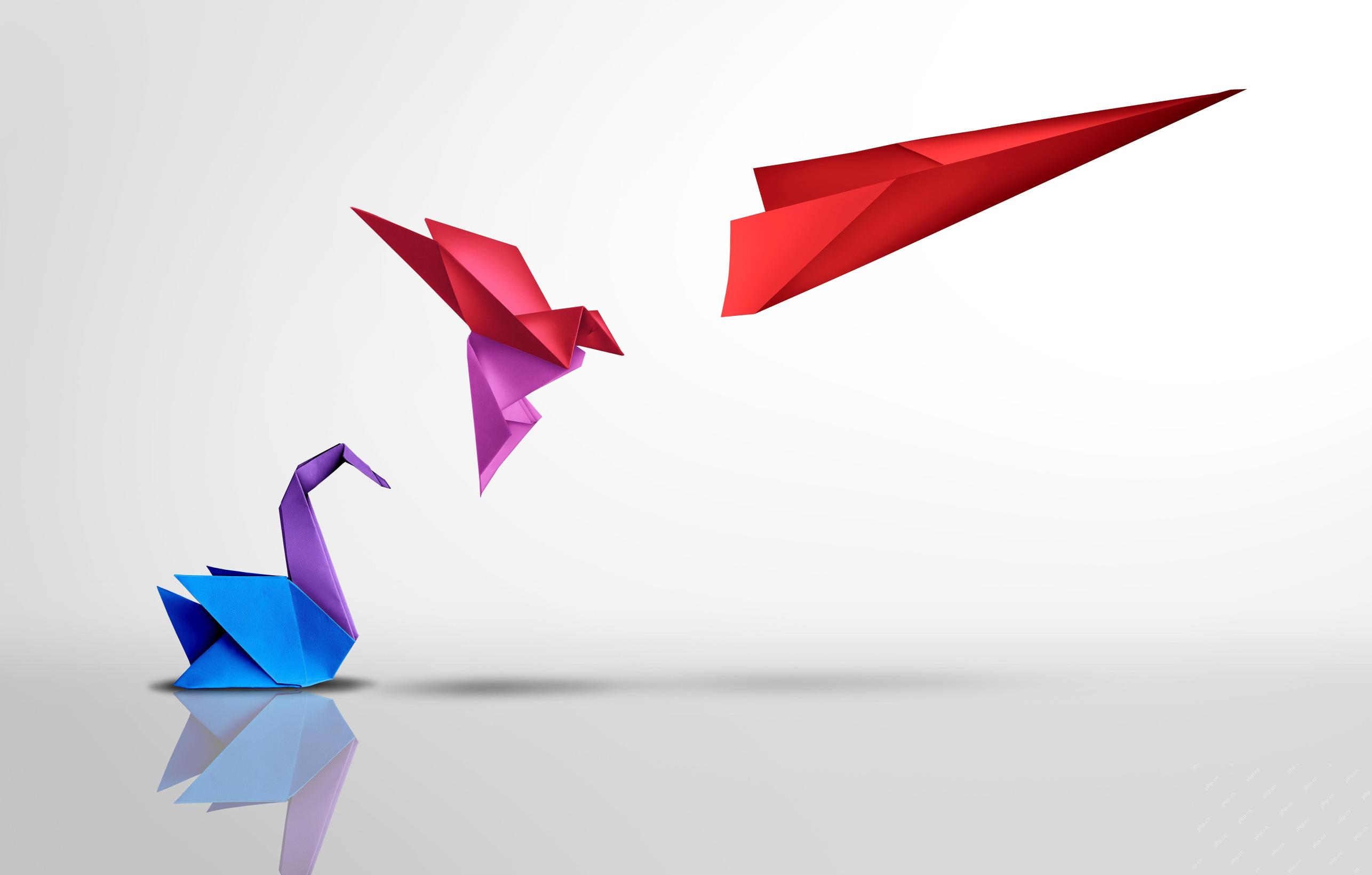
Labor Day 2050. Parks across the nation fill with families enjoying traditional barbecues while nostalgic parades wind through city streets. Yet the celebration now carries a museum-like quality — historical reenactment rather than commemoration of c
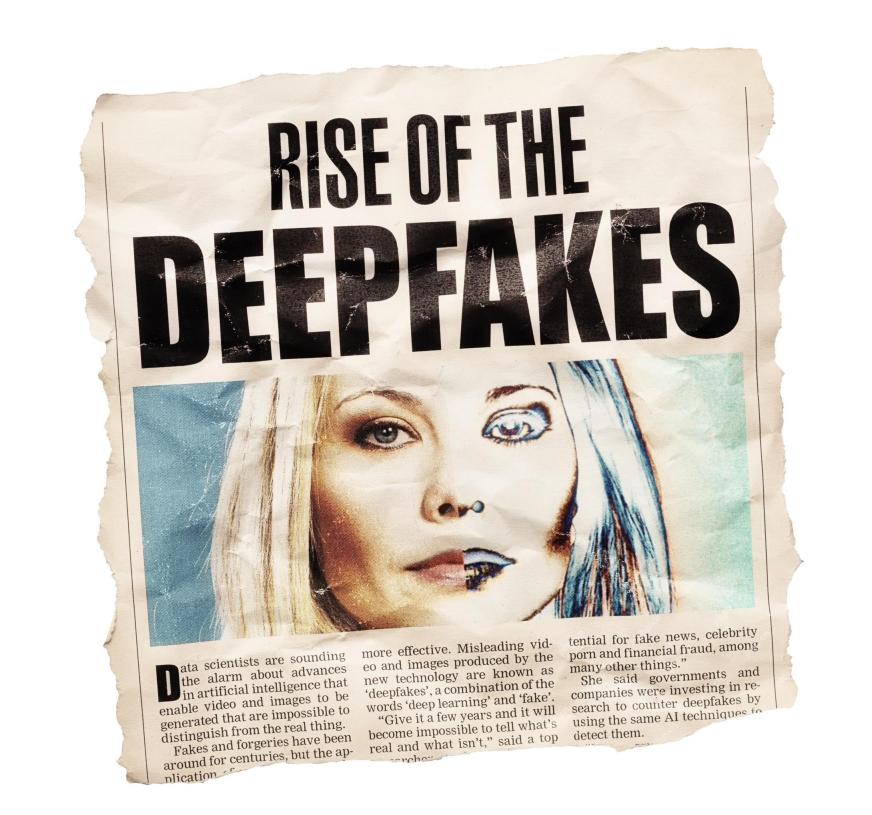
To help address this urgent and unsettling trend, a peer-reviewed article in the February 2025 edition of TEM Journal provides one of the clearest, data-driven assessments as to where that technological deepfake face off currently stands. Researcher
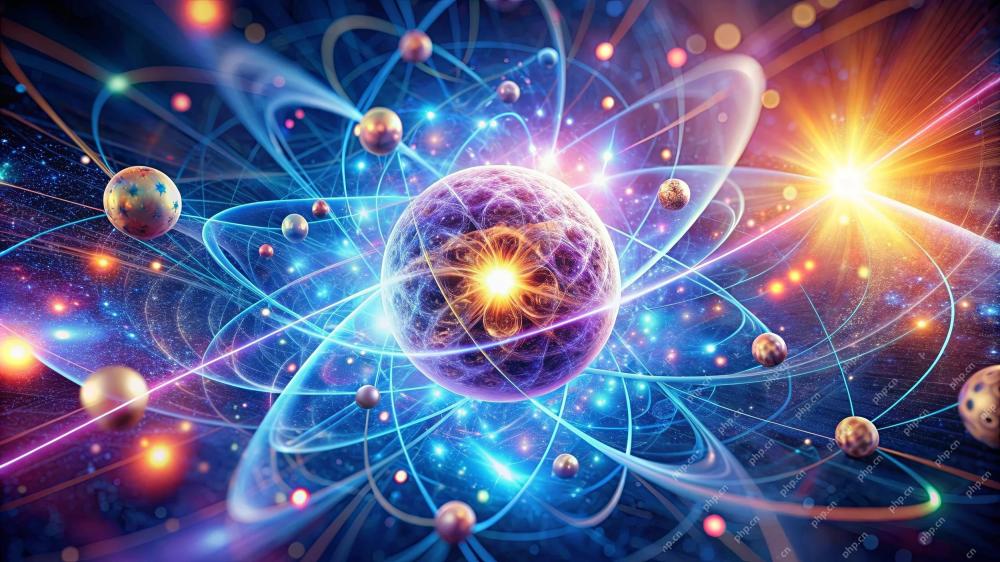
From vastly decreasing the time it takes to formulate new drugs to creating greener energy, there will be huge opportunities for businesses to break new ground. There’s a big problem, though: there’s a severe shortage of people with the skills busi
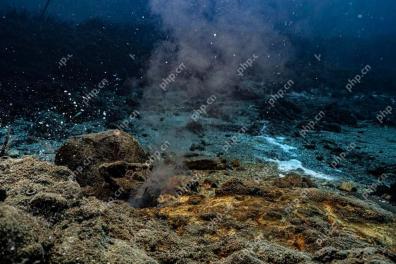
Years ago, scientists found that certain kinds of bacteria appear to breathe by generating electricity, rather than taking in oxygen, but how they did so was a mystery. A new study published in the journal Cell identifies how this happens: the microb
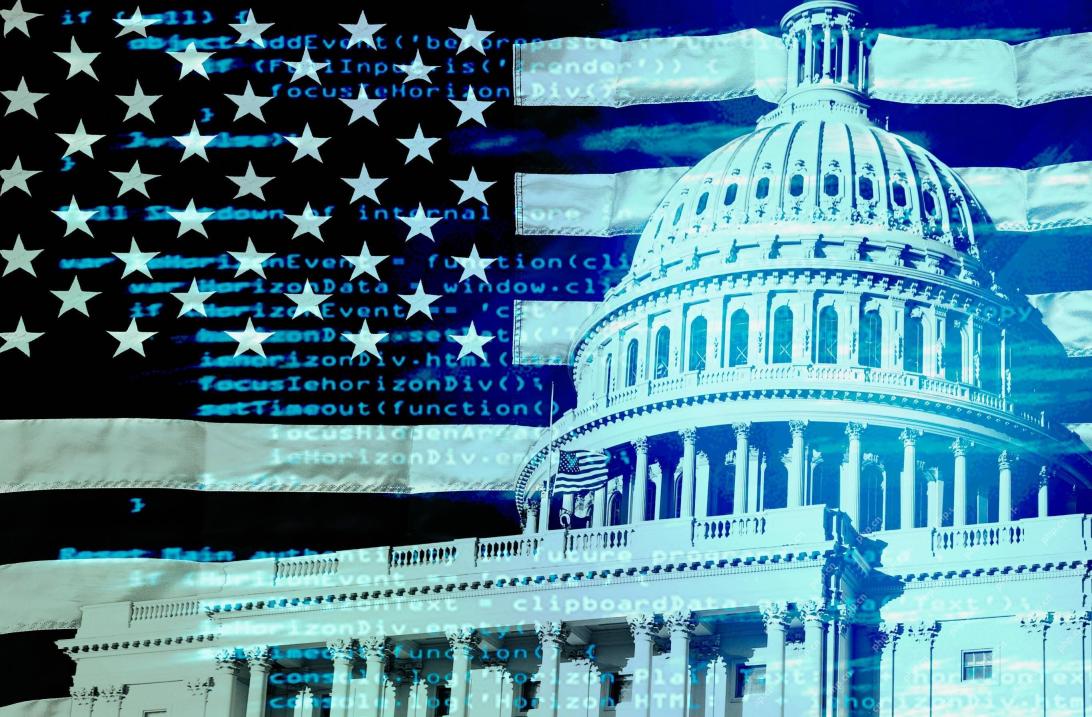
At the RSAC 2025 conference this week, Snyk hosted a timely panel titled “The First 100 Days: How AI, Policy & Cybersecurity Collide,” featuring an all-star lineup: Jen Easterly, former CISA Director; Nicole Perlroth, former journalist and partne


Hot AI Tools

Undresser.AI Undress
AI-powered app for creating realistic nude photos

AI Clothes Remover
Online AI tool for removing clothes from photos.

Undress AI Tool
Undress images for free

Clothoff.io
AI clothes remover

Video Face Swap
Swap faces in any video effortlessly with our completely free AI face swap tool!

Hot Article

Hot Tools

Atom editor mac version download
The most popular open source editor

Notepad++7.3.1
Easy-to-use and free code editor

DVWA
Damn Vulnerable Web App (DVWA) is a PHP/MySQL web application that is very vulnerable. Its main goals are to be an aid for security professionals to test their skills and tools in a legal environment, to help web developers better understand the process of securing web applications, and to help teachers/students teach/learn in a classroom environment Web application security. The goal of DVWA is to practice some of the most common web vulnerabilities through a simple and straightforward interface, with varying degrees of difficulty. Please note that this software

mPDF
mPDF is a PHP library that can generate PDF files from UTF-8 encoded HTML. The original author, Ian Back, wrote mPDF to output PDF files "on the fly" from his website and handle different languages. It is slower than original scripts like HTML2FPDF and produces larger files when using Unicode fonts, but supports CSS styles etc. and has a lot of enhancements. Supports almost all languages, including RTL (Arabic and Hebrew) and CJK (Chinese, Japanese and Korean). Supports nested block-level elements (such as P, DIV),

PhpStorm Mac version
The latest (2018.2.1) professional PHP integrated development tool
