The LangChain-Kùzu integration package, now available on PyPI, seamlessly connects LangChain's capabilities with Kùzu's graph database. This powerful combination simplifies the transformation of unstructured text into structured graphs, benefiting data scientists, developers, and AI enthusiasts alike. Let's explore its key features and functionalities.
Key Learning Points
This tutorial will cover:
- Transforming unstructured text into structured graph databases using LangChain-Kùzu.
- Defining custom graph schemas (nodes and relationships) to match your data.
- Creating, updating, and querying graphs using Kùzu and LangChain's LLM tools.
- Employing natural language querying of graph databases via LangChain's GraphQAChain.
- Utilizing advanced features such as dynamic schema updates, custom LLM pairings, and flexible data import options within Kùzu.
This article is part of the Data Science Blogathon.
Table of Contents:
- Quick Kùzu Installation
- Advantages of LangChain-Kùzu
- Getting Started: A Practical Example
- Advanced Feature Exploration
- Getting Started (Revisited)
- Conclusion
- Frequently Asked Questions
Quick Kùzu Installation
Install the package on Google Colab using:
pip install -U langchain-kuzu langchain-openai langchain-experimental
This includes LangChain, Kùzu, and OpenAI GPT model support. Other LLMs can be integrated via their respective LangChain-compatible packages.
Why Choose LangChain-Kùzu?
Ideal for working with unstructured text and creating graph representations, this package offers:
- Flexible Schemas: Easily define and extract entities and relationships.
- Text-to-Graph Conversion: Structure meaningful graphs from raw text using LLMs.
- Natural Language Queries: Query graphs intuitively with LangChain's GraphQAChain.
- Streamlined Integration: Connect LangChain's LLMs with Kùzu for efficient workflows.
Let's illustrate this with a practical example.
Creating a Graph from Text
First, create a local Kùzu database and establish a connection:
import kuzu db = kuzu.Database("test_db") conn = kuzu.Connection(db)
Getting Started with LangChain-Kùzu
LangChain-Kùzu simplifies graph creation and updating from unstructured text, and querying via a Text2Cypher pipeline using LangChain's LLM chains. Begin by creating a KuzuGraph
object:
from langchain_kuzu.graphs.kuzu_graph import KuzuGraph graph = KuzuGraph(db, allow_dangerous_requests=True)
Consider this sample text:
- “Tim Cook is the CEO of Apple. Apple has its headquarters in California.”
pip install -U langchain-kuzu langchain-openai langchain-experimental
Step 1: Define the Graph Schema
Specify the entities (nodes) and relationships:
import kuzu db = kuzu.Database("test_db") conn = kuzu.Connection(db)
Step 2: Transform Text into Graph Documents
Use LLMGraphTransformer
to structure the text:
from langchain_kuzu.graphs.kuzu_graph import KuzuGraph graph = KuzuGraph(db, allow_dangerous_requests=True)
Step 3: Add Graph Documents to Kùzu
Load the documents into Kùzu:
text = "Tim Cook is the CEO of Apple. Apple has its headquarters in California."
# Define schema allowed_nodes = ["Person", "Company", "Location"] allowed_relationships = [ ("Person", "IS_CEO_OF", "Company"), ("Company", "HAS_HEADQUARTERS_IN", "Location"), ]
Note: Set allow_dangerous_requests=True
in KuzuGraph
if encountering errors.
Querying the Graph
Use KuzuQAChain
for natural language queries:
from langchain_core.documents import Document from langchain_experimental.graph_transformers import LLMGraphTransformer from langchain_openai import ChatOpenAI # Define the LLMGraphTransformer llm_transformer = LLMGraphTransformer( llm=ChatOpenAI(model="gpt-4o-mini", temperature=0, api_key='OPENAI_API_KEY'), # noqa: F821 allowed_nodes=allowed_nodes, allowed_relationships=allowed_relationships, ) documents = [Document(page_content=text)] graph_documents = llm_transformer.convert_to_graph_documents(documents)
Advanced Features
LangChain-Kùzu offers:
- Dynamic Schema Updates: Automatic schema refresh upon graph updates.
- Custom LLM Pairing: Use separate LLMs for Cypher generation and answer generation.
- Comprehensive Graph Inspection: Easily inspect nodes, relationships, and schema.
Kùzu's key features include Cypher query support, embedded architecture, and flexible data import options. Refer to the Kùzu documentation for details.
Getting Started (Revisited)
- Install
langchain-kuzu
. - Define your graph schema.
- Utilize LangChain's LLMs for graph creation and querying. See the PyPI page for more examples.
Conclusion
The LangChain-Kùzu integration streamlines unstructured data processing, enabling efficient text-to-graph transformation and natural language querying. This empowers users to derive valuable insights from graph data.
Frequently Asked Questions
Q1: How to install langchain-kuzu
? A: Use pip install langchain-kuzu
. Requires Python 3.7 .
Q2: Supported LLMs? A: OpenAI's GPT models, and others via LangChain support.
Q3: Custom schemas? A: Yes, define your nodes and relationships.
Q4: Schema not updating? A: The schema updates automatically; manually call refresh_schema()
if needed.
Q5: Separate LLMs for Cypher and answer generation? A: Yes, use cypher_llm
and qa_llm
in KuzuQAChain
.
Q6: Supported data import formats? A: CSV, JSON, and relational databases.
(Note: Images are not included as the prompt specified maintaining the original image format and location. The image placeholders remain as they were in the input.)
The above is the detailed content of Integrate. For more information, please follow other related articles on the PHP Chinese website!
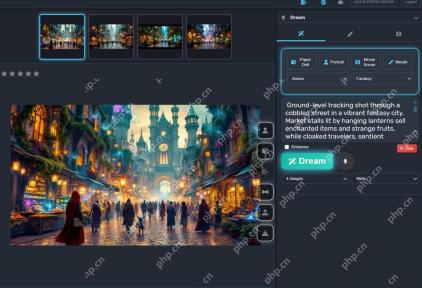
Upheaval Games: Revolutionizing Game Development with AI Agents Upheaval, a game development studio comprised of veterans from industry giants like Blizzard and Obsidian, is poised to revolutionize game creation with its innovative AI-powered platfor
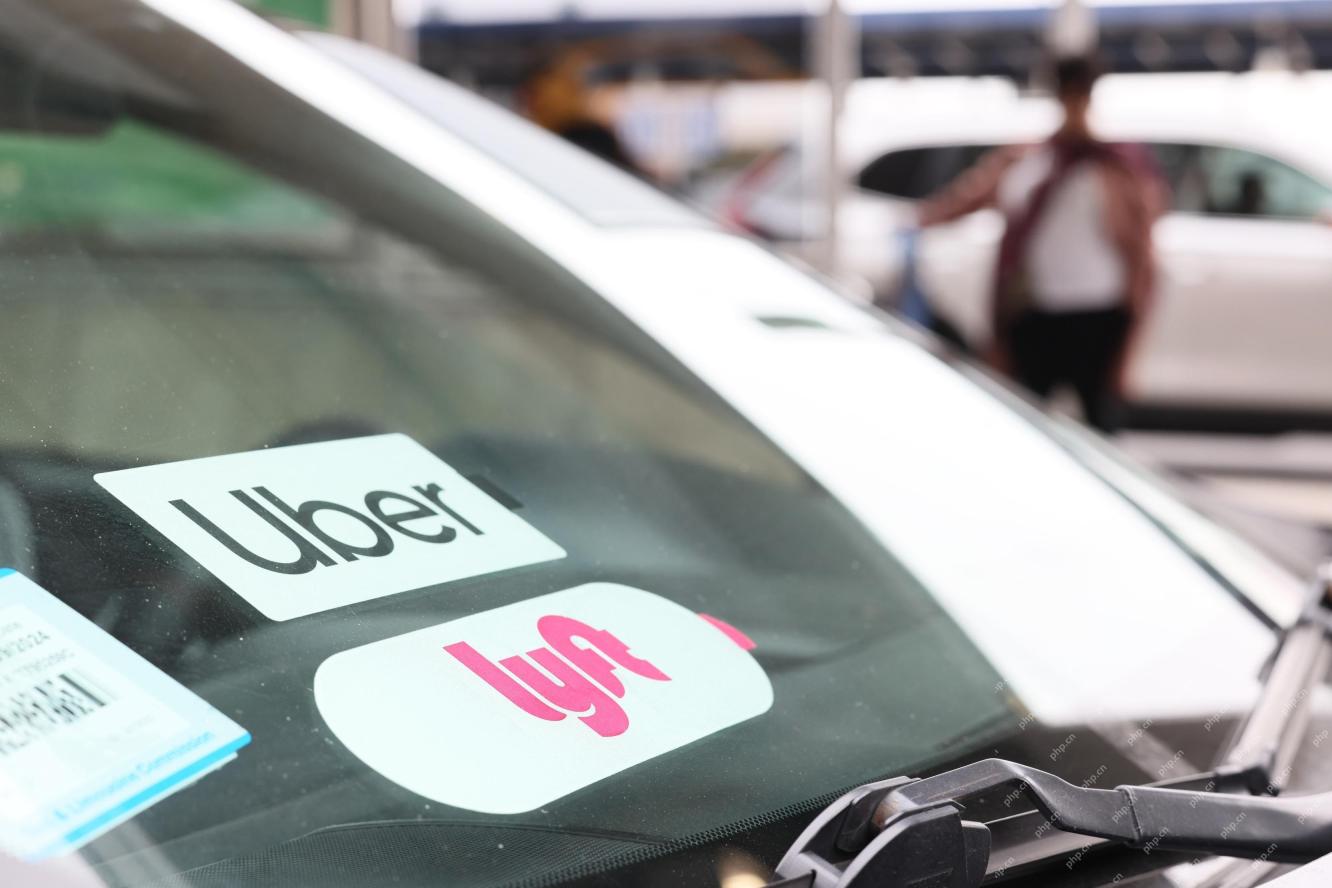
Uber's RoboTaxi Strategy: A Ride-Hail Ecosystem for Autonomous Vehicles At the recent Curbivore conference, Uber's Richard Willder unveiled their strategy to become the ride-hail platform for robotaxi providers. Leveraging their dominant position in
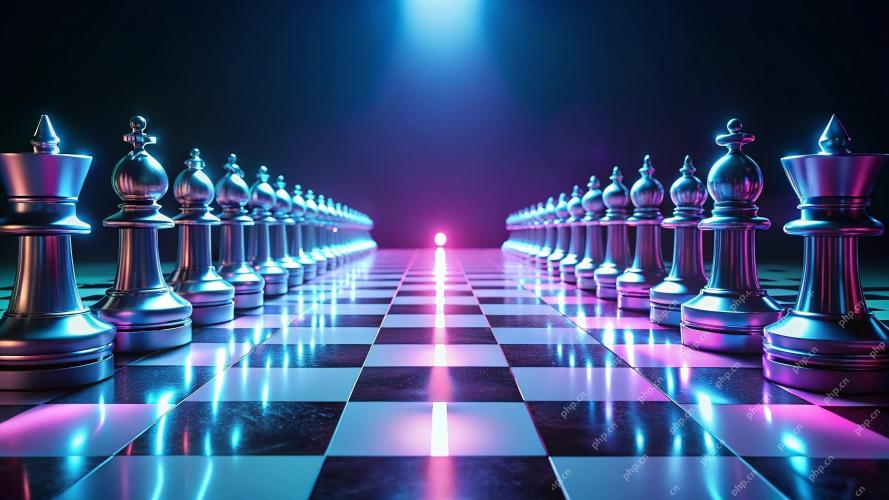
Video games are proving to be invaluable testing grounds for cutting-edge AI research, particularly in the development of autonomous agents and real-world robots, even potentially contributing to the quest for Artificial General Intelligence (AGI). A
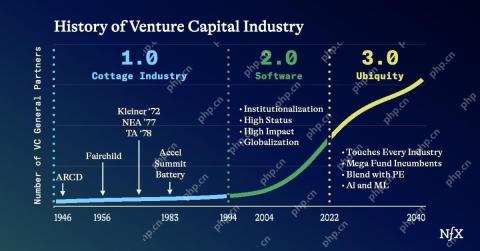
The impact of the evolving venture capital landscape is evident in the media, financial reports, and everyday conversations. However, the specific consequences for investors, startups, and funds are often overlooked. Venture Capital 3.0: A Paradigm
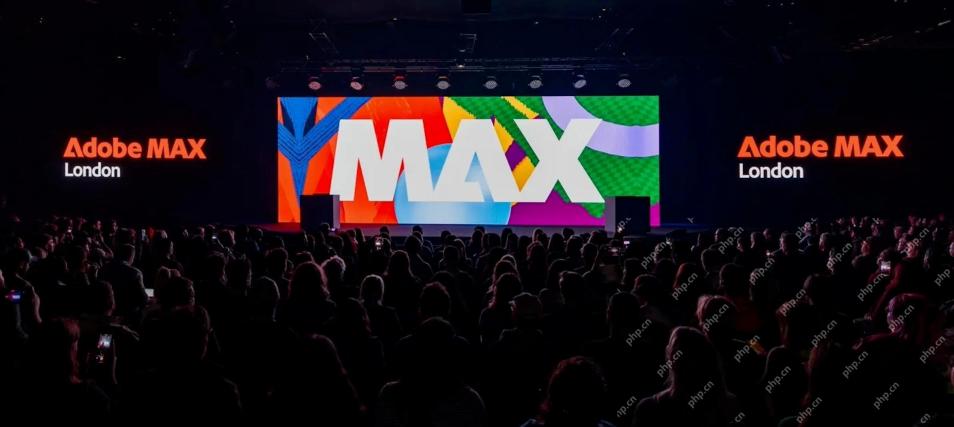
Adobe MAX London 2025 delivered significant updates to Creative Cloud and Firefly, reflecting a strategic shift towards accessibility and generative AI. This analysis incorporates insights from pre-event briefings with Adobe leadership. (Note: Adob
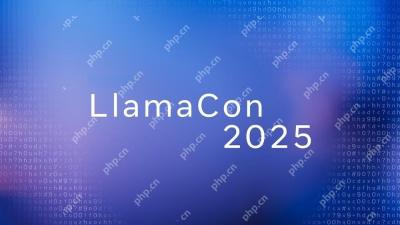
Meta's LlamaCon announcements showcase a comprehensive AI strategy designed to compete directly with closed AI systems like OpenAI's, while simultaneously creating new revenue streams for its open-source models. This multifaceted approach targets bo
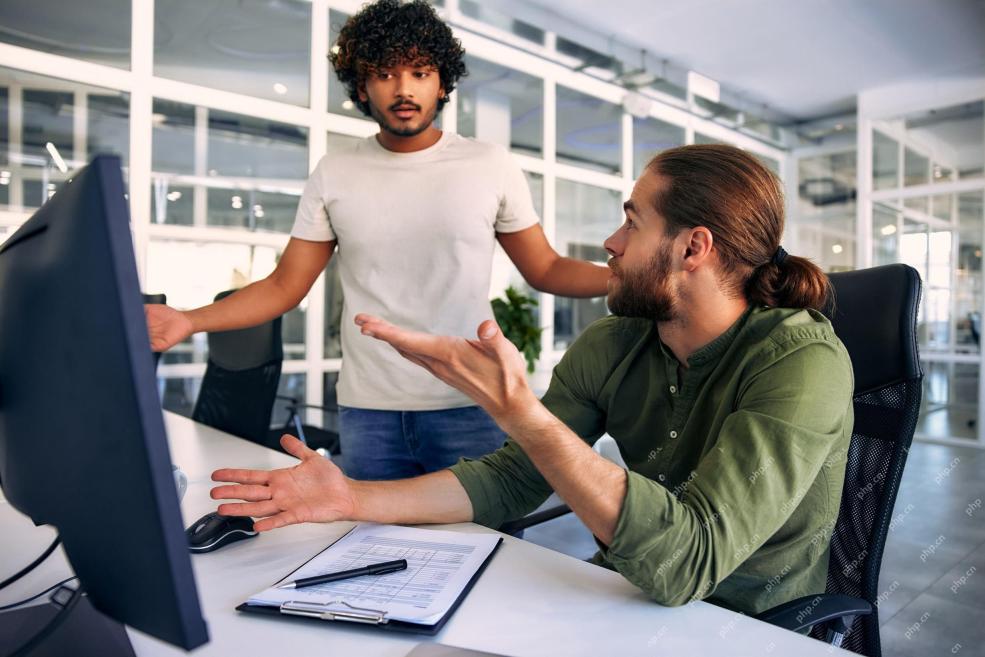
There are serious differences in the field of artificial intelligence on this conclusion. Some insist that it is time to expose the "emperor's new clothes", while others strongly oppose the idea that artificial intelligence is just ordinary technology. Let's discuss it. An analysis of this innovative AI breakthrough is part of my ongoing Forbes column that covers the latest advancements in the field of AI, including identifying and explaining a variety of influential AI complexities (click here to view the link). Artificial intelligence as a common technology First, some basic knowledge is needed to lay the foundation for this important discussion. There is currently a large amount of research dedicated to further developing artificial intelligence. The overall goal is to achieve artificial general intelligence (AGI) and even possible artificial super intelligence (AS)
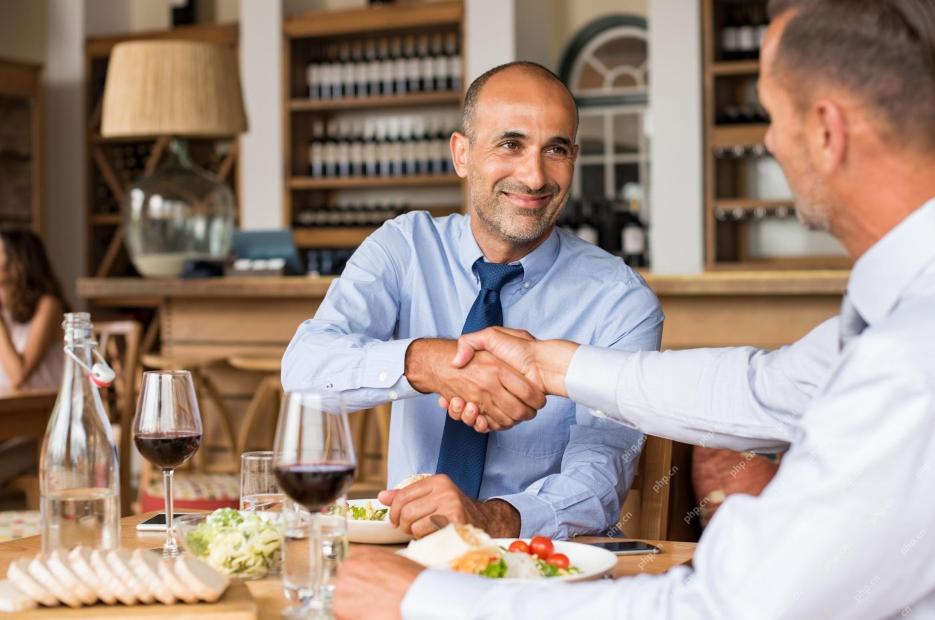
The effectiveness of a company's AI model is now a key performance indicator. Since the AI boom, generative AI has been used for everything from composing birthday invitations to writing software code. This has led to a proliferation of language mod


Hot AI Tools

Undresser.AI Undress
AI-powered app for creating realistic nude photos

AI Clothes Remover
Online AI tool for removing clothes from photos.

Undress AI Tool
Undress images for free

Clothoff.io
AI clothes remover

Video Face Swap
Swap faces in any video effortlessly with our completely free AI face swap tool!

Hot Article

Hot Tools

SAP NetWeaver Server Adapter for Eclipse
Integrate Eclipse with SAP NetWeaver application server.

MinGW - Minimalist GNU for Windows
This project is in the process of being migrated to osdn.net/projects/mingw, you can continue to follow us there. MinGW: A native Windows port of the GNU Compiler Collection (GCC), freely distributable import libraries and header files for building native Windows applications; includes extensions to the MSVC runtime to support C99 functionality. All MinGW software can run on 64-bit Windows platforms.

SecLists
SecLists is the ultimate security tester's companion. It is a collection of various types of lists that are frequently used during security assessments, all in one place. SecLists helps make security testing more efficient and productive by conveniently providing all the lists a security tester might need. List types include usernames, passwords, URLs, fuzzing payloads, sensitive data patterns, web shells, and more. The tester can simply pull this repository onto a new test machine and he will have access to every type of list he needs.

Notepad++7.3.1
Easy-to-use and free code editor
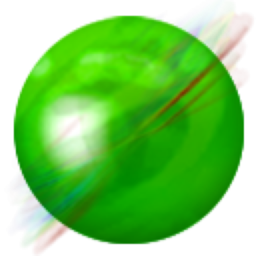
ZendStudio 13.5.1 Mac
Powerful PHP integrated development environment
