This guide demonstrates fine-tuning the Microsoft Phi-4 large language model (LLM) for specialized tasks using Low-Rank Adaptation (LoRA) adapters and Hugging Face. By focusing on specific domains, you can optimize Phi-4's performance for applications like customer support or medical advice. The efficiency of LoRA makes this process faster and less resource-intensive.
Key Learning Outcomes:
- Fine-tune Microsoft Phi-4 using LoRA adapters for targeted applications.
- Configure and load Phi-4 efficiently with 4-bit quantization.
- Prepare and transform datasets for fine-tuning with Hugging Face and the
unsloth
library. - Optimize model performance using Hugging Face's
SFTTrainer
. - Monitor GPU usage and save/upload fine-tuned models to Hugging Face for deployment.
Prerequisites:
Before starting, ensure you have:
- Python 3.8
- PyTorch (with CUDA support for GPU acceleration)
-
unsloth
library - Hugging Face
transformers
anddatasets
libraries
Install necessary libraries using:
pip install unsloth pip install --force-reinstall --no-cache-dir --no-deps git+https://github.com/unslothai/unsloth.git
Fine-Tuning Phi-4: A Step-by-Step Approach
This section details the fine-tuning process, from setup to deployment on Hugging Face.
Step 1: Model Setup
This involves loading the model and importing essential libraries:
from unsloth import FastLanguageModel import torch max_seq_length = 2048 load_in_4bit = True model, tokenizer = FastLanguageModel.from_pretrained( model_name="unsloth/Phi-4", max_seq_length=max_seq_length, load_in_4bit=load_in_4bit, ) model = FastLanguageModel.get_peft_model( model, r=16, target_modules=["q_proj", "k_proj", "v_proj", "o_proj", "gate_proj", "up_proj", "down_proj"], lora_alpha=16, lora_dropout=0, bias="none", use_gradient_checkpointing="unsloth", random_state=3407, )
Step 2: Dataset Preparation
We'll use the FineTome-100k dataset in ShareGPT format. unsloth
helps convert this to Hugging Face's format:
from datasets import load_dataset from unsloth.chat_templates import standardize_sharegpt, get_chat_template dataset = load_dataset("mlabonne/FineTome-100k", split="train") dataset = standardize_sharegpt(dataset) tokenizer = get_chat_template(tokenizer, chat_template="phi-4") def formatting_prompts_func(examples): texts = [ tokenizer.apply_chat_template(convo, tokenize=False, add_generation_prompt=False) for convo in examples["conversations"] ] return {"text": texts} dataset = dataset.map(formatting_prompts_func, batched=True)
Step 3: Model Fine-tuning
Fine-tune using Hugging Face's SFTTrainer
:
from trl import SFTTrainer from transformers import TrainingArguments, DataCollatorForSeq2Seq from unsloth import is_bfloat16_supported from unsloth.chat_templates import train_on_responses_only trainer = SFTTrainer( # ... (Trainer configuration as in the original response) ... ) trainer = train_on_responses_only( trainer, instruction_part="user", response_part="assistant", )
Step 4: GPU Usage Monitoring
Monitor GPU memory usage:
import torch # ... (GPU monitoring code as in the original response) ...
Step 5: Inference
Generate responses:
pip install unsloth pip install --force-reinstall --no-cache-dir --no-deps git+https://github.com/unslothai/unsloth.git
Step 6: Saving and Uploading
Save locally or push to Hugging Face:
from unsloth import FastLanguageModel import torch max_seq_length = 2048 load_in_4bit = True model, tokenizer = FastLanguageModel.from_pretrained( model_name="unsloth/Phi-4", max_seq_length=max_seq_length, load_in_4bit=load_in_4bit, ) model = FastLanguageModel.get_peft_model( model, r=16, target_modules=["q_proj", "k_proj", "v_proj", "o_proj", "gate_proj", "up_proj", "down_proj"], lora_alpha=16, lora_dropout=0, bias="none", use_gradient_checkpointing="unsloth", random_state=3407, )
Remember to replace <your_hf_token></your_hf_token>
with your actual Hugging Face token.
Conclusion:
This streamlined guide empowers developers to efficiently fine-tune Phi-4 for specific needs, leveraging the power of LoRA and Hugging Face for optimized performance and easy deployment. Remember to consult the original response for complete code snippets and detailed explanations.
The above is the detailed content of How to Fine-Tune Phi-4 Locally?. For more information, please follow other related articles on the PHP Chinese website!
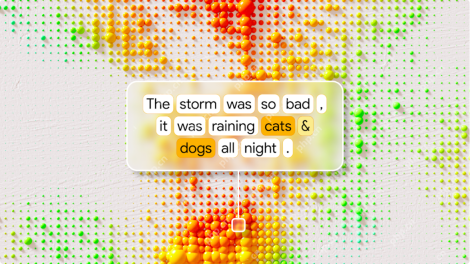
Exploring the Inner Workings of Language Models with Gemma Scope Understanding the complexities of AI language models is a significant challenge. Google's release of Gemma Scope, a comprehensive toolkit, offers researchers a powerful way to delve in
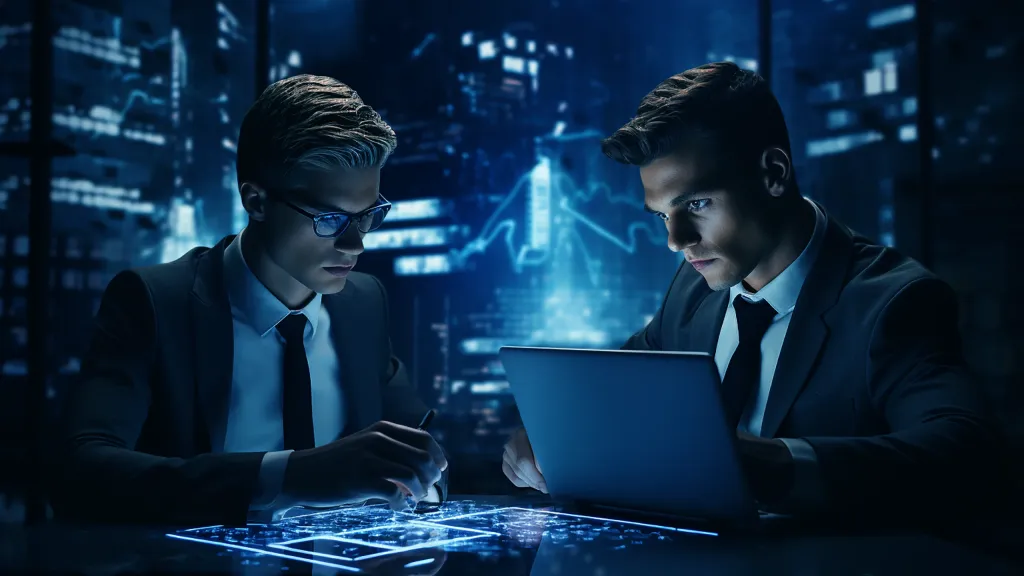
Unlocking Business Success: A Guide to Becoming a Business Intelligence Analyst Imagine transforming raw data into actionable insights that drive organizational growth. This is the power of a Business Intelligence (BI) Analyst – a crucial role in gu

SQL's ALTER TABLE Statement: Dynamically Adding Columns to Your Database In data management, SQL's adaptability is crucial. Need to adjust your database structure on the fly? The ALTER TABLE statement is your solution. This guide details adding colu
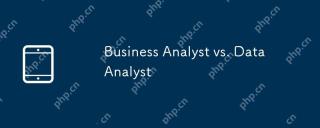
Introduction Imagine a bustling office where two professionals collaborate on a critical project. The business analyst focuses on the company's objectives, identifying areas for improvement, and ensuring strategic alignment with market trends. Simu
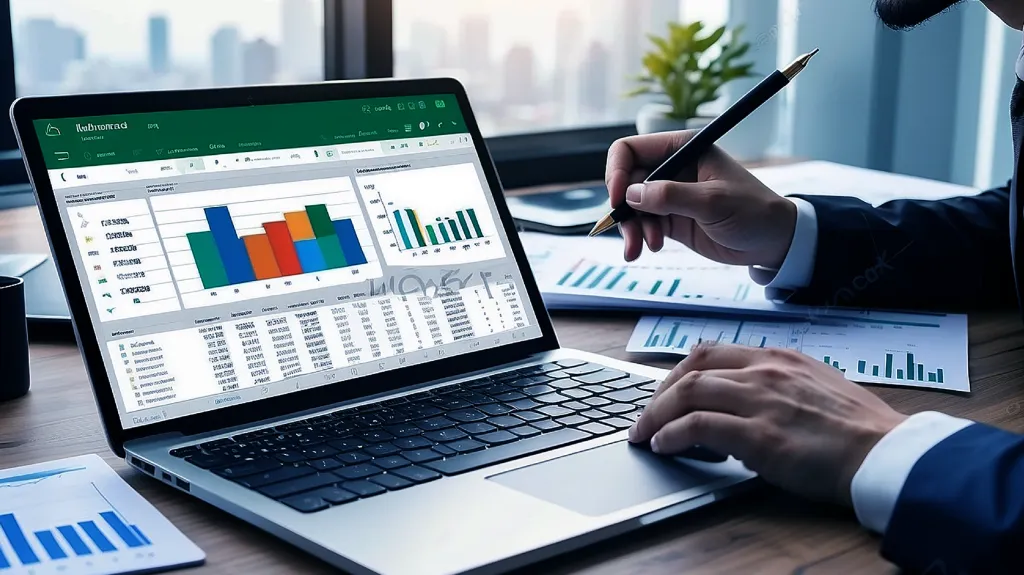
Excel data counting and analysis: detailed explanation of COUNT and COUNTA functions Accurate data counting and analysis are critical in Excel, especially when working with large data sets. Excel provides a variety of functions to achieve this, with the COUNT and COUNTA functions being key tools for counting the number of cells under different conditions. Although both functions are used to count cells, their design targets are targeted at different data types. Let's dig into the specific details of COUNT and COUNTA functions, highlight their unique features and differences, and learn how to apply them in data analysis. Overview of key points Understand COUNT and COU
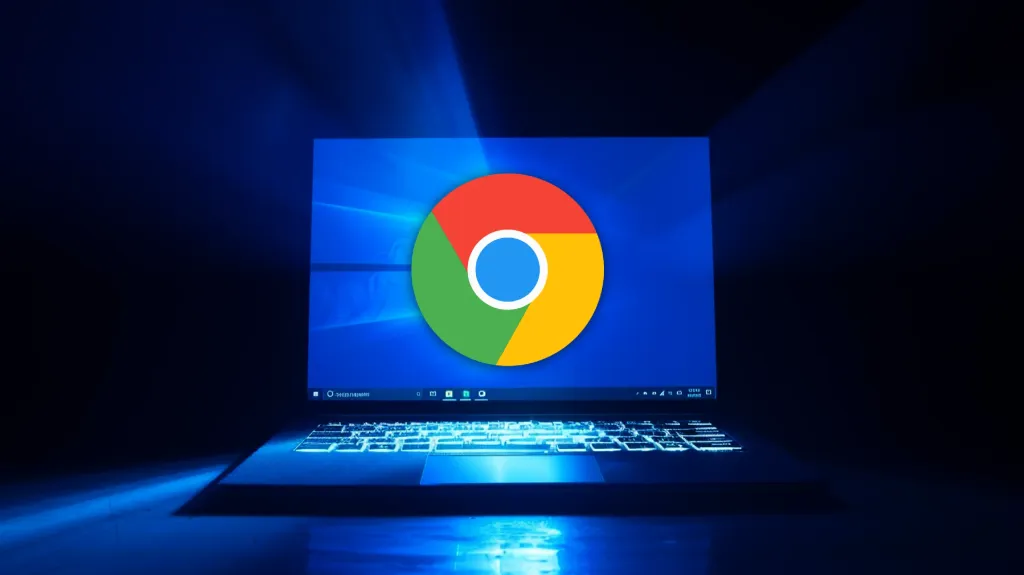
Google Chrome's AI Revolution: A Personalized and Efficient Browsing Experience Artificial Intelligence (AI) is rapidly transforming our daily lives, and Google Chrome is leading the charge in the web browsing arena. This article explores the exciti
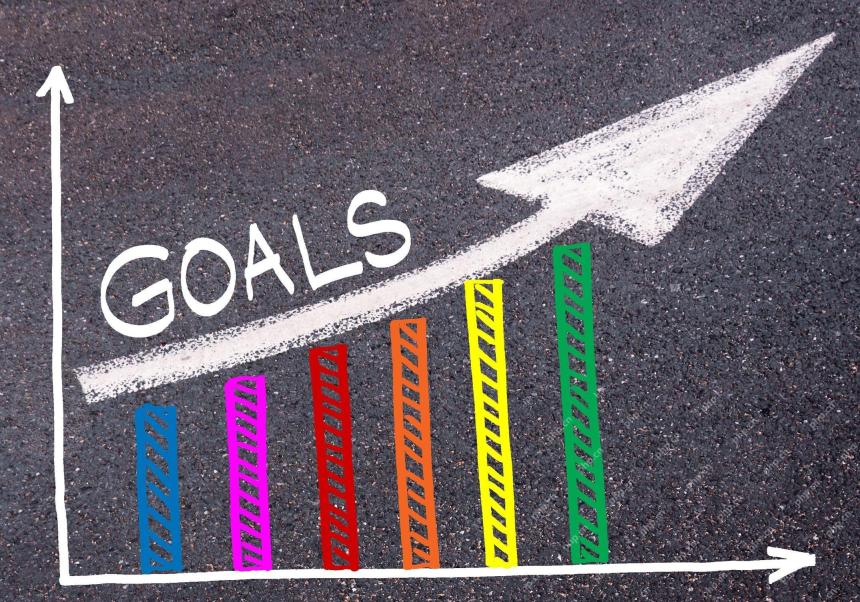
Reimagining Impact: The Quadruple Bottom Line For too long, the conversation has been dominated by a narrow view of AI’s impact, primarily focused on the bottom line of profit. However, a more holistic approach recognizes the interconnectedness of bu
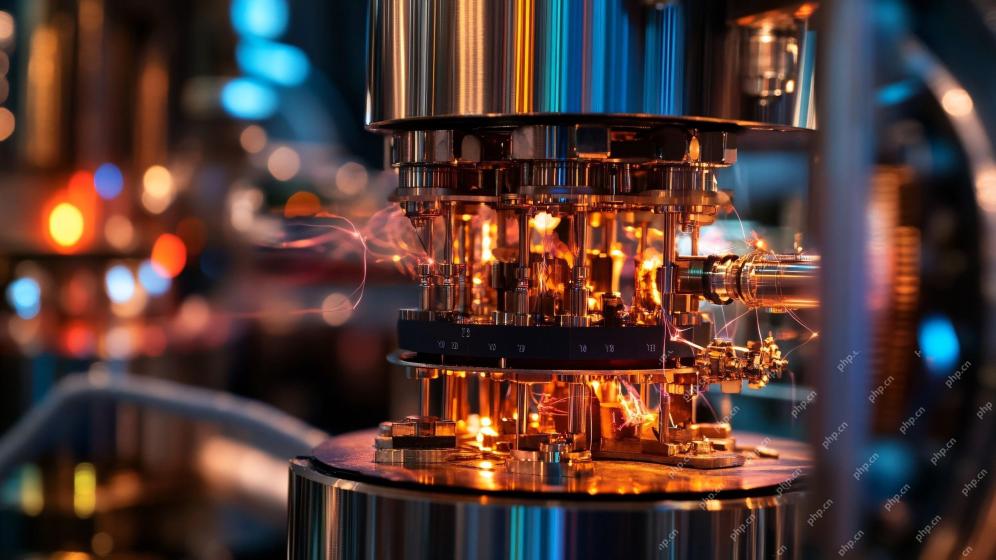
Things are moving steadily towards that point. The investment pouring into quantum service providers and startups shows that industry understands its significance. And a growing number of real-world use cases are emerging to demonstrate its value out


Hot AI Tools

Undresser.AI Undress
AI-powered app for creating realistic nude photos

AI Clothes Remover
Online AI tool for removing clothes from photos.

Undress AI Tool
Undress images for free

Clothoff.io
AI clothes remover

AI Hentai Generator
Generate AI Hentai for free.

Hot Article

Hot Tools

MinGW - Minimalist GNU for Windows
This project is in the process of being migrated to osdn.net/projects/mingw, you can continue to follow us there. MinGW: A native Windows port of the GNU Compiler Collection (GCC), freely distributable import libraries and header files for building native Windows applications; includes extensions to the MSVC runtime to support C99 functionality. All MinGW software can run on 64-bit Windows platforms.

Notepad++7.3.1
Easy-to-use and free code editor
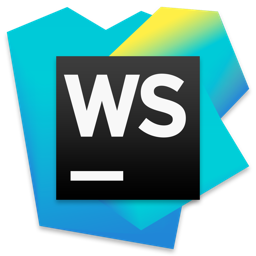
WebStorm Mac version
Useful JavaScript development tools
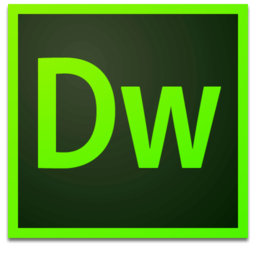
Dreamweaver Mac version
Visual web development tools
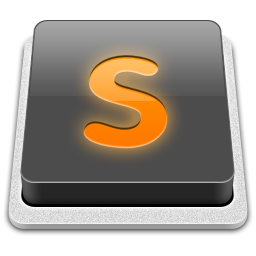
SublimeText3 Mac version
God-level code editing software (SublimeText3)