


Salesforce XGen-7B: A Step-by-Step Tutorial on Using And Fine-Tuning XGen-7B
Salesforce's XGen-7B: A Powerful, Compact Open-Source LLM with 8k Context Length
Several leading open-source Large Language Models (LLMs) suffer from a significant limitation: short context windows, typically capped at 2048 tokens. This contrasts sharply with proprietary models like GPT-3.5 and GPT-4, boasting context lengths up to 32,000 tokens. This constraint severely impacts performance on tasks demanding extensive contextual understanding, such as summarization, translation, and code generation.
Enter Salesforce's XGen-7B. This model tackles the context length bottleneck head-on, offering an impressive 8,000-token context window—four times greater than comparable open-source alternatives. This article explores XGen-7B's key features, usage, and fine-tuning on a sample dataset.
Why Choose XGen-7B?
XGen-7B's advantages extend beyond its extended context length. Its key features include:
Exceptional Efficiency: Despite its relatively modest 7 billion parameters, XGen-7B delivers performance rivaling or surpassing much larger models. This efficiency allows deployment on high-end local machines, eliminating the need for extensive cloud computing resources. This makes it accessible to a broader range of users, from individual researchers to small businesses.
Versatile Model Variants: Salesforce provides three XGen-7B variants to cater to diverse needs:
- XGen-7B-4K-base: A 4,000-token model suitable for tasks requiring moderate context. Licensed under the Apache 2.0 license.
- XGen-7B-8K-base: The flagship 8,000-token model, ideal for complex tasks needing extensive contextual analysis. Also licensed under Apache 2.0.
- XGen-7B-{4K,8K}-inst: Fine-tuned for interactive and instructional applications (non-commercial use). Perfect for educational tools and chatbots.
Superior Benchmark Performance: XGen-7B consistently outperforms similarly sized models on various benchmarks, including MMLU and HumanEval. Refer to the official announcement for detailed benchmark results.
Optimized for Long Sequences: XGen-7B's architecture is specifically optimized for long-sequence tasks. This is crucial for applications like detailed document summarization and comprehensive question-answering, where understanding the entire input is essential for accurate and coherent outputs.
Salesforce XGen-7B Training Methodology
XGen-7B's impressive capabilities stem from its sophisticated training process:
-
Stage 1: Training on 1.37 trillion tokens of mixed natural language and code data.
-
Stage 2: Further training on 55 billion tokens of code data to enhance code generation capabilities.
The training leveraged Salesforce's JaxFormer library, designed for efficient LLM training on TPU-v4 hardware.
Setting Up and Running XGen-7B
Running XGen-7B locally requires a powerful machine (32GB RAM, high-end GPU). Alternatively, services like Google Colab Pro offer sufficient resources.
Installation:
After setting up your environment, install necessary libraries:
pip install torch torchvision torchaudio transformers[torch] accelerate peft bitsandbytes trl datasets --upgrade
Initial Run:
This code snippet demonstrates a basic run using the 8k-token model:
import torch from transformers import AutoTokenizer, AutoModelForCausalLM tokenizer = AutoTokenizer.from_pretrained("Salesforce/xgen-7b-8k-base", trust_remote_code=True) model = AutoModelForCausalLM.from_pretrained("Salesforce/xgen-7b-8k-base", torch_dtype=torch.bfloat16) inputs = tokenizer("DataCamp is one he ...", return_tensors="pt") sample = model.generate(**inputs, max_length=128) print(tokenizer.decode(sample[0]))
Fine-Tuning XGen-7B
Fine-tuning XGen-7B involves several steps (detailed instructions are omitted for brevity, but the original text provides a comprehensive guide):
- Installation (already covered above).
- Import necessary modules (from
datasets
,transformers
,peft
,trl
). - Define configurations for base and fine-tuned models.
- Load the dataset (e.g., Guanaco LLaMA2 dataset).
- Define quantization parameters using
BitsAndBytesConfig
. - Load the model and tokenizer.
- Define PEFT parameters using
LoraConfig
. - Set training arguments using
TrainingArguments
. - Fine-tune the model using
SFTTrainer
. - Evaluate the fine-tuned model.
- Save the fine-tuned model and tokenizer.
Conclusion
While straightforward to use, adapting XGen-7B to specific tasks requires careful consideration of datasets and computational resources. The fine-tuning process, as outlined above, provides a robust framework for tailoring this powerful LLM to your specific needs. Remember to consult the provided links for more detailed explanations and resources on LLMs and fine-tuning techniques.
The above is the detailed content of Salesforce XGen-7B: A Step-by-Step Tutorial on Using And Fine-Tuning XGen-7B. For more information, please follow other related articles on the PHP Chinese website!
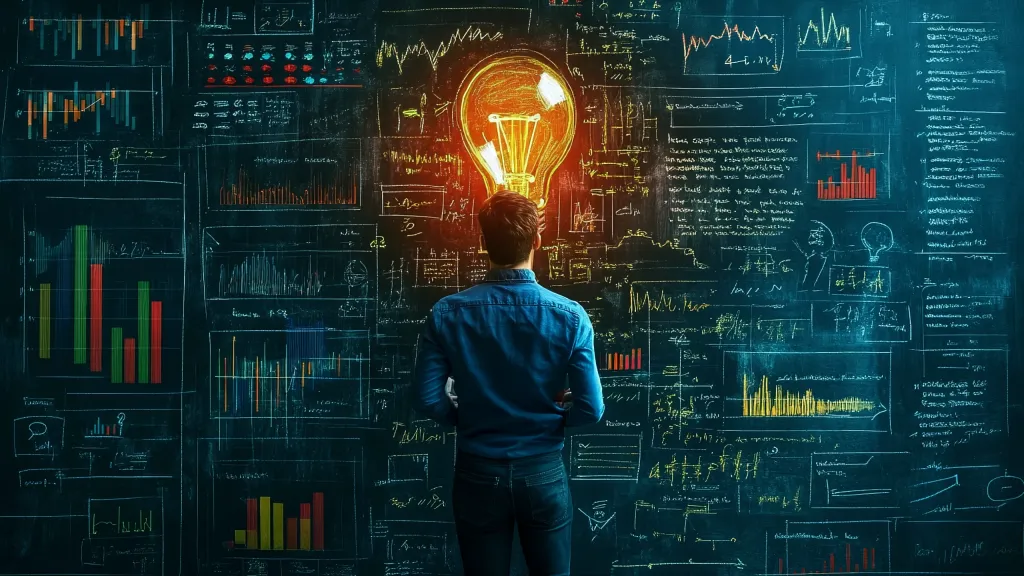
Introduction In prompt engineering, “Graph of Thought” refers to a novel approach that uses graph theory to structure and guide AI’s reasoning process. Unlike traditional methods, which often involve linear s
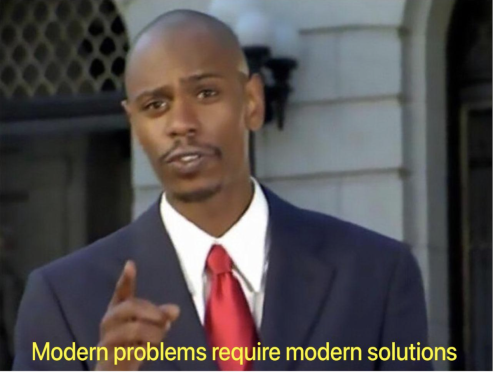
Introduction Congratulations! You run a successful business. Through your web pages, social media campaigns, webinars, conferences, free resources, and other sources, you collect 5000 email IDs daily. The next obvious step is
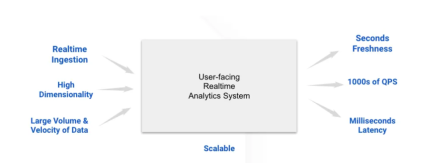
Introduction In today’s fast-paced software development environment, ensuring optimal application performance is crucial. Monitoring real-time metrics such as response times, error rates, and resource utilization can help main
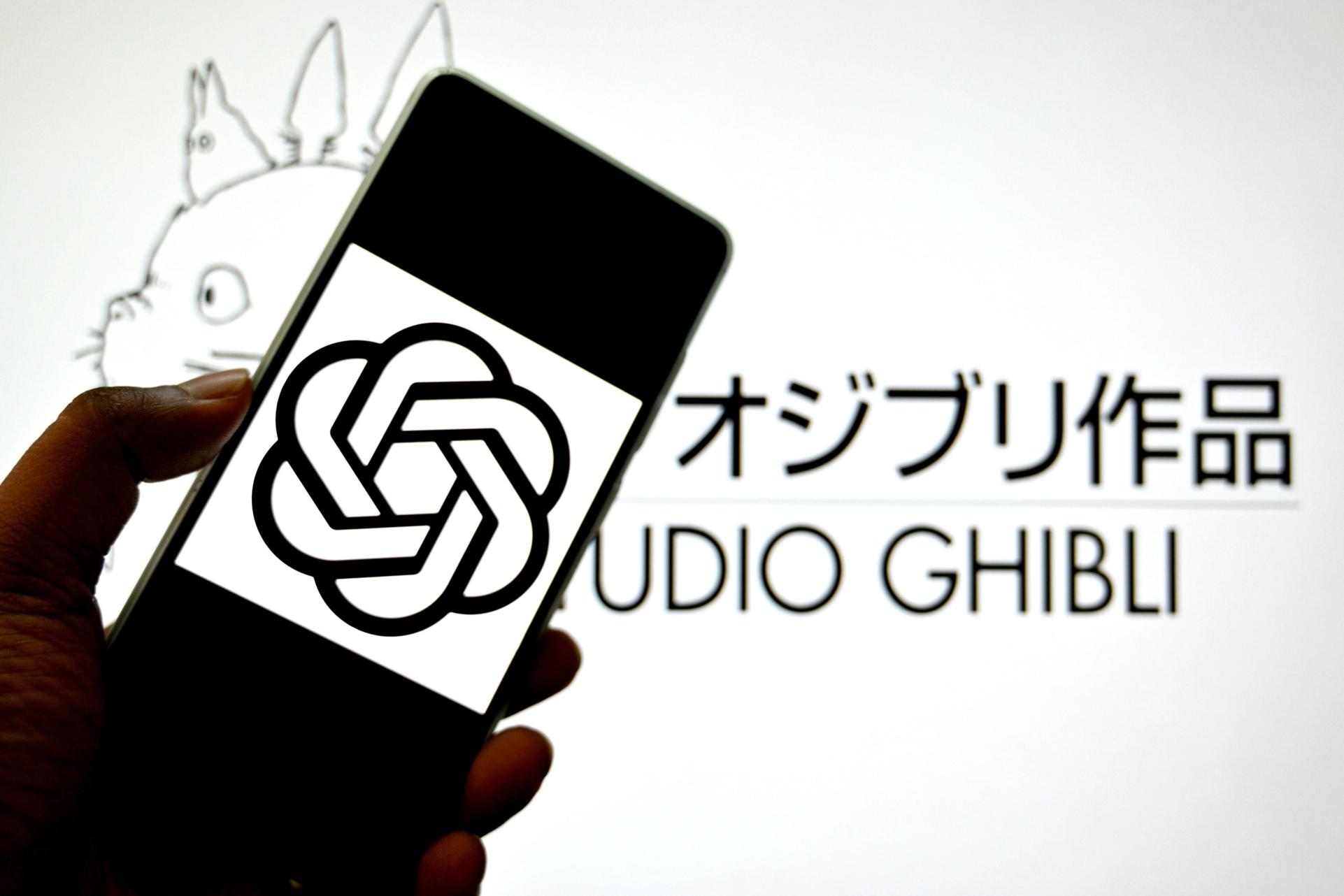
“How many users do you have?” he prodded. “I think the last time we said was 500 million weekly actives, and it is growing very rapidly,” replied Altman. “You told me that it like doubled in just a few weeks,” Anderson continued. “I said that priv
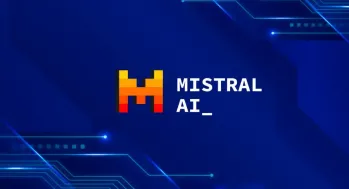
Introduction Mistral has released its very first multimodal model, namely the Pixtral-12B-2409. This model is built upon Mistral’s 12 Billion parameter, Nemo 12B. What sets this model apart? It can now take both images and tex
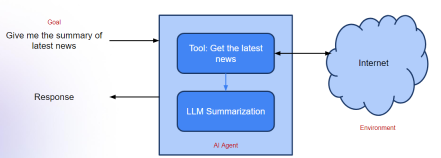
Imagine having an AI-powered assistant that not only responds to your queries but also autonomously gathers information, executes tasks, and even handles multiple types of data—text, images, and code. Sounds futuristic? In this a
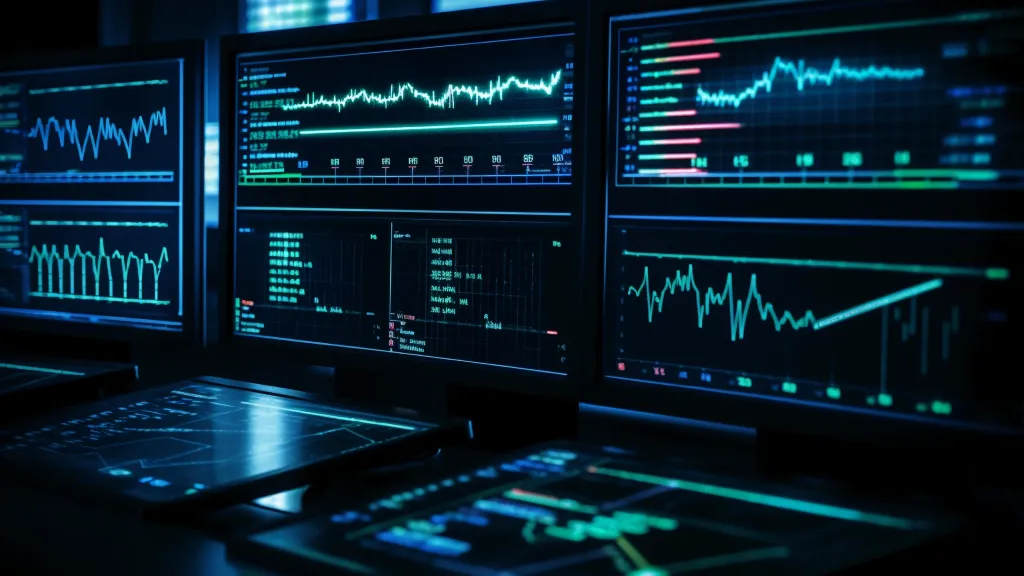
Introduction The finance industry is the cornerstone of any country’s development, as it drives economic growth by facilitating efficient transactions and credit availability. The ease with which transactions occur and credit

Introduction Data is being generated at an unprecedented rate from sources such as social media, financial transactions, and e-commerce platforms. Handling this continuous stream of information is a challenge, but it offers an


Hot AI Tools

Undresser.AI Undress
AI-powered app for creating realistic nude photos

AI Clothes Remover
Online AI tool for removing clothes from photos.

Undress AI Tool
Undress images for free

Clothoff.io
AI clothes remover

AI Hentai Generator
Generate AI Hentai for free.

Hot Article

Hot Tools

MinGW - Minimalist GNU for Windows
This project is in the process of being migrated to osdn.net/projects/mingw, you can continue to follow us there. MinGW: A native Windows port of the GNU Compiler Collection (GCC), freely distributable import libraries and header files for building native Windows applications; includes extensions to the MSVC runtime to support C99 functionality. All MinGW software can run on 64-bit Windows platforms.

MantisBT
Mantis is an easy-to-deploy web-based defect tracking tool designed to aid in product defect tracking. It requires PHP, MySQL and a web server. Check out our demo and hosting services.
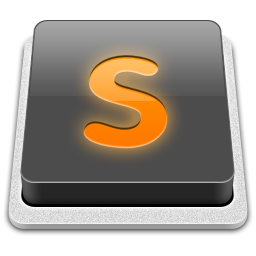
SublimeText3 Mac version
God-level code editing software (SublimeText3)

Notepad++7.3.1
Easy-to-use and free code editor

SublimeText3 Chinese version
Chinese version, very easy to use