This blog post explores the rapidly evolving field of semantic search, driven by advancements in Generative AI. It details how to build a semantic search application using Python, Pinecone (a vector database), and OpenAI's GPT embedding model.
Search and retrieval, traditionally keyword-based, is revolutionized by semantic search, which understands query intent and context. This requires understanding concepts like embeddings and vector databases.
Embeddings: Bridging the Gap Between Language and Numbers
Embeddings convert unstructured data (text, images, audio, video) into multi-dimensional numerical vectors. Similar items have vectors close together in this high-dimensional space. This allows machines to understand semantic relationships, such as synonyms and analogies. OpenAI's text-embedding-ada-002
model is used in this example, producing 1536-dimensional vectors. Creating embeddings involves training a large neural network model; using pre-trained models like OpenAI's is more practical.
Embeddings are crucial for various applications, enabling contextually relevant search results. They are not limited to text; image embeddings are used in computer vision.
Vector Databases: Efficiently Storing and Querying Vectors
Vector databases are specialized for storing and querying high-dimensional vector data, unlike traditional relational databases. They excel at similarity searches, crucial for applications like recommendation systems and semantic search. Pinecone is a fully managed, scalable vector database used in this tutorial.
Semantic Search and Its Applications
Semantic search goes beyond keyword matching, understanding the meaning and context of queries. Factors driving its rise include voice search and the emergence of multimodal large language models (LLMs). Semantic search improves search relevance across various domains: e-commerce, content discovery, customer support, knowledge management, and voice search optimization.
Pinecone and OpenAI: The Tools of the Trade
Pinecone provides a managed, scalable vector database, simplifying deployment. OpenAI offers powerful embedding models via its API, easily accessible through Python.
Python Implementation: A Step-by-Step Guide
The tutorial provides a detailed walkthrough of building a semantic search application in Python:
- Sign up for OpenAI and Pinecone: Obtain API keys.
-
Install Python libraries:
pinecone-client
,pinecone-datasets
,openai
. -
Sample Dataset: Use the
wikipedia-simple-text-embedding-ada-002-100K
dataset. - Create Pinecone Index: Create an index to store the vectors.
- Insert Data: Upsert the embedded data into the Pinecone index.
-
Embed New Data using OpenAI API: Create a function to embed new queries using
text-embedding-ada-002
. - Query the vector database: Query the index with the new embedding and retrieve top results.
Conclusion
This tutorial provides a practical guide to building semantic search applications, highlighting the importance of embeddings and vector databases. The combination of Pinecone and OpenAI's API empowers developers to create powerful and relevant search experiences. The blog concludes with links to further learning resources. The future of search is semantic.
The above is the detailed content of Semantic Search with Pinecone and OpenAI. For more information, please follow other related articles on the PHP Chinese website!
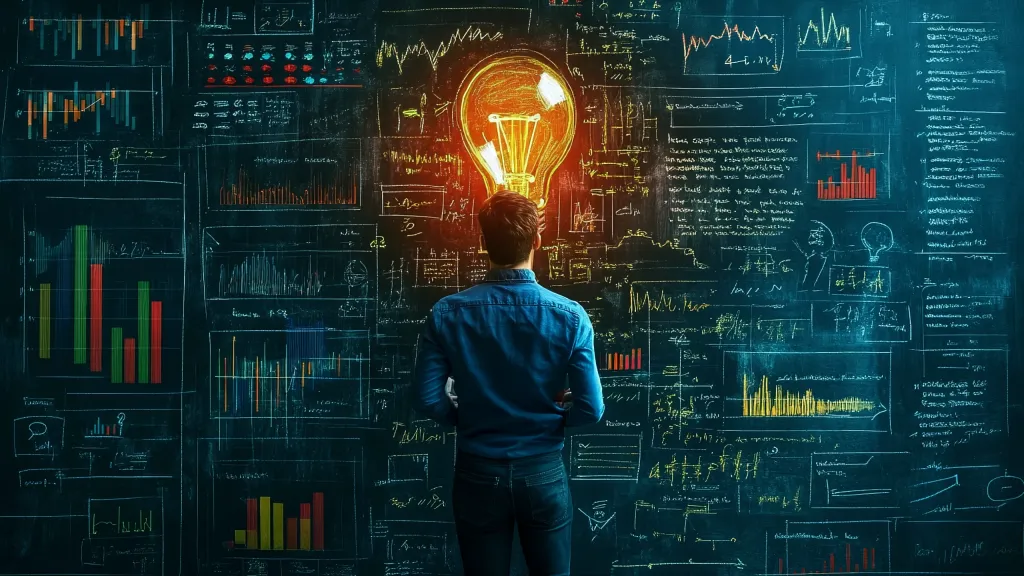
Introduction In prompt engineering, “Graph of Thought” refers to a novel approach that uses graph theory to structure and guide AI’s reasoning process. Unlike traditional methods, which often involve linear s
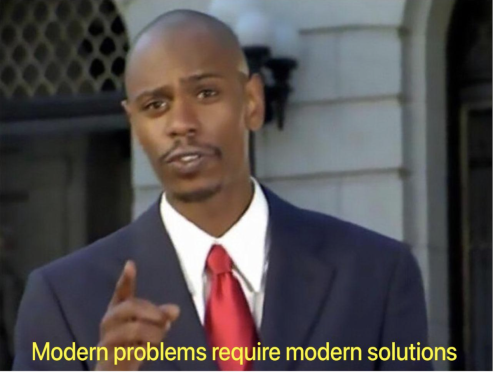
Introduction Congratulations! You run a successful business. Through your web pages, social media campaigns, webinars, conferences, free resources, and other sources, you collect 5000 email IDs daily. The next obvious step is
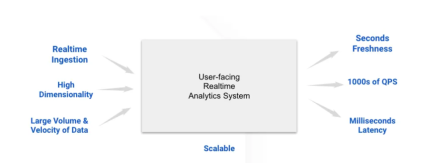
Introduction In today’s fast-paced software development environment, ensuring optimal application performance is crucial. Monitoring real-time metrics such as response times, error rates, and resource utilization can help main
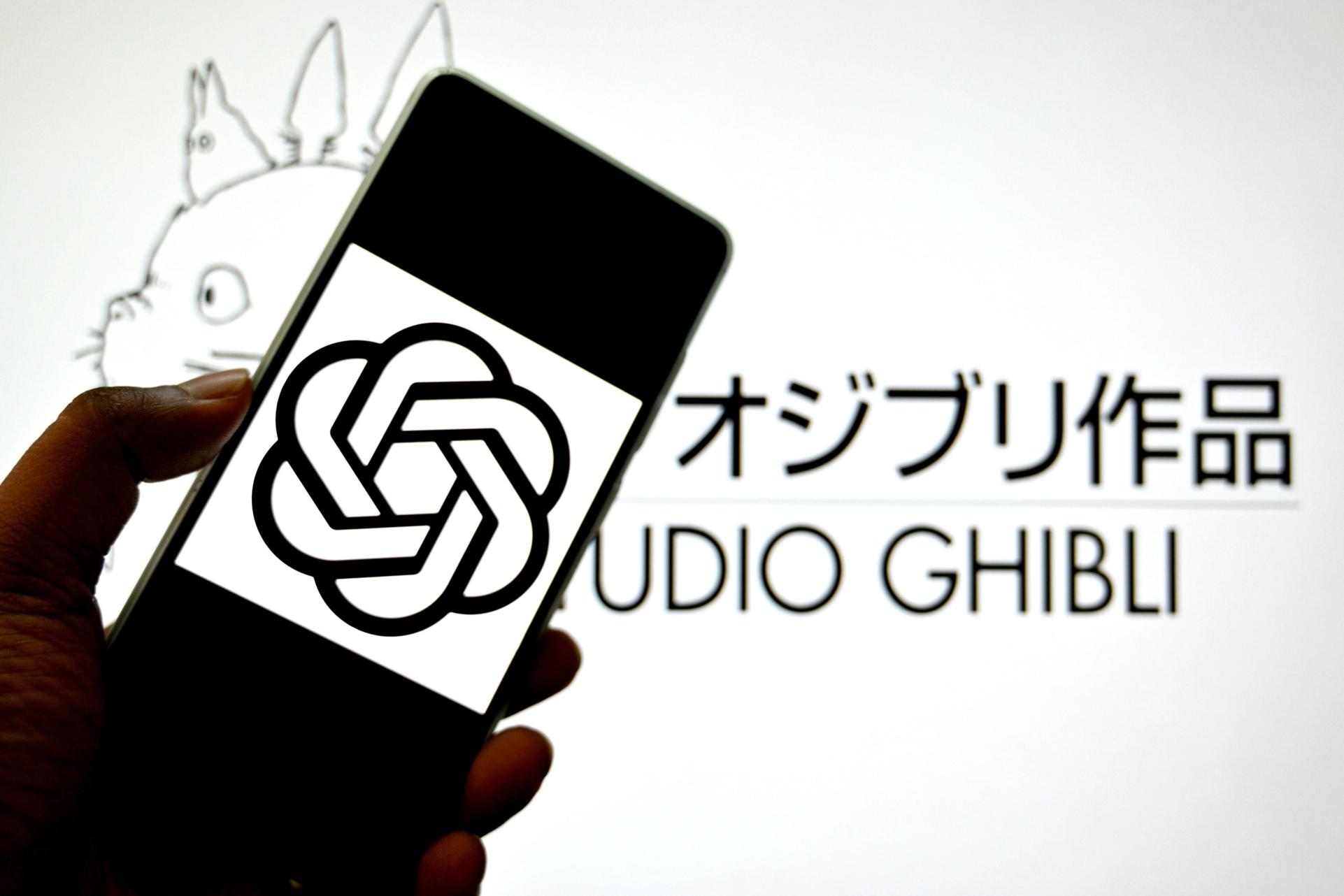
“How many users do you have?” he prodded. “I think the last time we said was 500 million weekly actives, and it is growing very rapidly,” replied Altman. “You told me that it like doubled in just a few weeks,” Anderson continued. “I said that priv
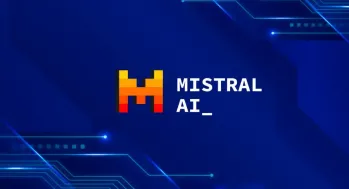
Introduction Mistral has released its very first multimodal model, namely the Pixtral-12B-2409. This model is built upon Mistral’s 12 Billion parameter, Nemo 12B. What sets this model apart? It can now take both images and tex
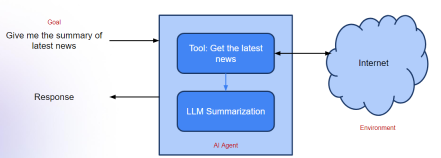
Imagine having an AI-powered assistant that not only responds to your queries but also autonomously gathers information, executes tasks, and even handles multiple types of data—text, images, and code. Sounds futuristic? In this a
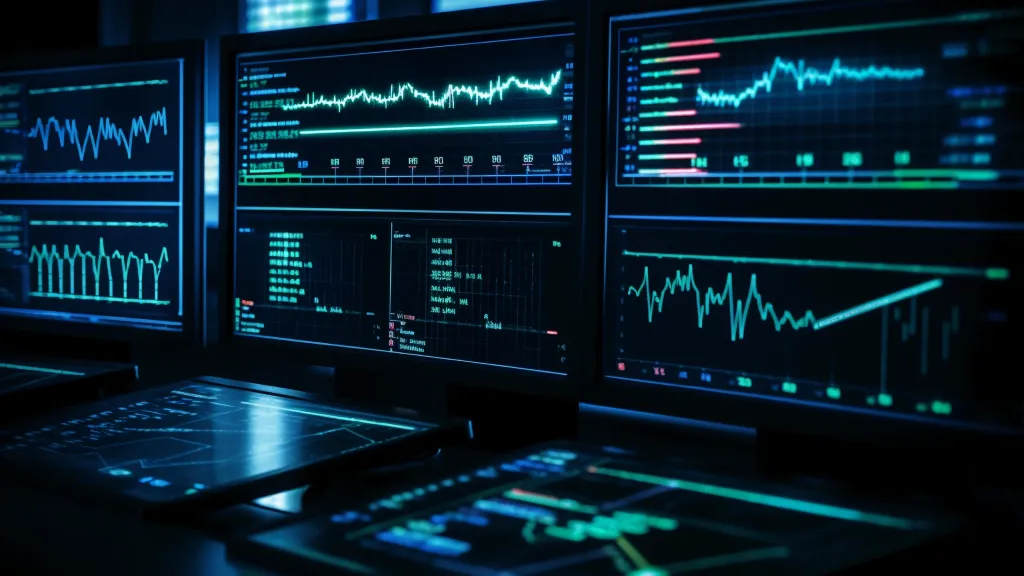
Introduction The finance industry is the cornerstone of any country’s development, as it drives economic growth by facilitating efficient transactions and credit availability. The ease with which transactions occur and credit

Introduction Data is being generated at an unprecedented rate from sources such as social media, financial transactions, and e-commerce platforms. Handling this continuous stream of information is a challenge, but it offers an


Hot AI Tools

Undresser.AI Undress
AI-powered app for creating realistic nude photos

AI Clothes Remover
Online AI tool for removing clothes from photos.

Undress AI Tool
Undress images for free

Clothoff.io
AI clothes remover

AI Hentai Generator
Generate AI Hentai for free.

Hot Article

Hot Tools

Atom editor mac version download
The most popular open source editor
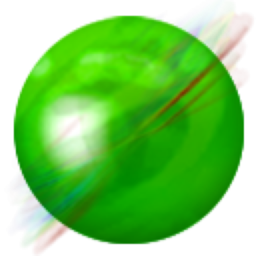
ZendStudio 13.5.1 Mac
Powerful PHP integrated development environment

SublimeText3 Chinese version
Chinese version, very easy to use
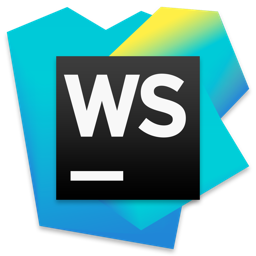
WebStorm Mac version
Useful JavaScript development tools
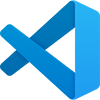
VSCode Windows 64-bit Download
A free and powerful IDE editor launched by Microsoft