


SQL, the Structured Query Language, is a cornerstone of database management, enabling efficient data storage, retrieval, and manipulation. Its widespread adoption stems from its simplicity and effectiveness in handling vast datasets. However, the evolving data landscape introduces new challenges.
The rise of artificial intelligence and large language models (LLMs) presents powerful tools, but interacting with them can be cumbersome. This is where LMQL steps in.
Developed by the SRI Lab at ETH Zürich, LMQL acts as a bridge between developers and LLMs. It brings the structured querying power of SQL to the world of language models, streamlining interactions and enhancing efficiency.
This tutorial covers:
- What is LMQL?
- Why use LMQL?
- Setting up LMQL
- Practical LMQL applications
- LMQL limitations
- Best practices
What is LMQL?
LMQL, or Language Models Query Language, is a novel programming language designed for LLMs. It combines declarative SQL-like features with an imperative scripting syntax, offering a more structured approach to information extraction and response generation from LLMs.
Importantly, LMQL extends Python, adding new functionalities and expanding its capabilities. This allows developers to craft natural language prompts incorporating text and code, increasing query flexibility and expressiveness. As its creators state, LMQL seamlessly integrates LLM interaction into program code, moving beyond traditional templating. It was introduced in a research paper, "Prompting is Programming: A Query Language for Large Language Models," as a solution for "Language Model Prompting" (LMP).
LLMs excel at tasks like question answering and code generation, generating logical sequences based on input probabilities. LMP leverages this by using language instructions or examples to trigger tasks. Advanced techniques even allow interactions between users, the model, and external tools.
The challenge lies in achieving optimal performance or tailoring LLMs for specific tasks, often requiring complex, task-specific programs that may still depend on ad-hoc interactions. LMQL addresses this by providing an intuitive blend of text prompting and scripting, enabling users to define constraints on LLM output.
Why Use LMQL?
While modern LLMs can be prompted conceptually, maximizing their potential and adapting to new models requires deep understanding of their inner workings and vendor-specific tools. Tasks like limiting output to specific words or phrases can be complex due to tokenization. Furthermore, using LLMs, whether locally or via APIs, is expensive due to their size.
LMQL mitigates these issues. It reduces LLM calls by leveraging predefined behaviors and search constraints. It also simplifies prompting techniques that often involve iterative communication between user and model or specialized interfaces. LMQL's constraint capabilities are crucial for production environments, ensuring predictable and processable output. For instance, in sentiment analysis, LMQL ensures consistent output like "positive," "negative," or "neutral," rather than more verbose, less easily parsed responses. Human-readable constraints replace the need to work with model tokens directly.
Setting Up LMQL
LMQL can be installed locally or accessed via its online Playground IDE. Local installation is necessary for self-hosted models using Transformers or llama.cpp.
Installation and Environment Setup
Local installation is simple:
pip install lmql
For GPU support with PyTorch >= 1.11:
pip install lmql[hf]
Using a virtual environment is recommended.
Three ways to run LMQL programs exist:
-
Playground:
lmql playground
launches a browser-based IDE (requires Node.js). Access via https://www.php.cn/link/4a914e5c38172ae9b61780ffbd0b2f90 if not automatically launched. -
Command-line:
lmql run
executes local.lmql
files. -
Python Integration: Import
lmql
and uselmql.run
or the@lmql.query
decorator.
When using local Transformer models in the Playground or command line, launch the LMQL Inference API using lmql serve-model
.
Understanding LMQL Syntax
An LMQL program has five key parts:
-
Query: The primary communication method between user and LLM. Uses
[varname]
for generated text and{varname}
for variable retrieval. - Decoder: Specifies the decoding algorithm (e.g., beam search). Can be defined within the query or externally (in Python).
-
Model: LMQL supports various models (OpenAI, llama.cpp, HuggingFace Transformers). Models are loaded using
lmql.model(...)
, and passed to the query either externally or using afrom
clause. - Constraints: Control LLM output using various constraints (stopping phrases, data types, character/token length, regex, custom constraints).
- Distribution: Defines the output format and structure.
LMQL Limitations and Community Support
LMQL's relative newness leads to a small community and less comprehensive documentation. Limitations with the OpenAI API also restrict full utilization with certain models like ChatGPT. However, ongoing development promises improvements.
Conclusion
LMQL offers a powerful, SQL-inspired approach to interacting with LLMs. Its Python integration and constraint capabilities make it a valuable tool for various applications. For further learning, explore resources on LlamaIndex, ChatGPT alternatives, LLM training with PyTorch, LangChain, and the Cohere API.
The above is the detailed content of An Introduction to LMQL: The Bridge Between SQL and Large Language Models. For more information, please follow other related articles on the PHP Chinese website!
![[Ghibli-style images with AI] Introducing how to create free images with ChatGPT and copyright](https://img.php.cn/upload/article/001/242/473/174707263295098.jpg?x-oss-process=image/resize,p_40)
The latest model GPT-4o released by OpenAI not only can generate text, but also has image generation functions, which has attracted widespread attention. The most eye-catching feature is the generation of "Ghibli-style illustrations". Simply upload the photo to ChatGPT and give simple instructions to generate a dreamy image like a work in Studio Ghibli. This article will explain in detail the actual operation process, the effect experience, as well as the errors and copyright issues that need to be paid attention to. For details of the latest model "o3" released by OpenAI, please click here⬇️ Detailed explanation of OpenAI o3 (ChatGPT o3): Features, pricing system and o4-mini introduction Please click here for the English version of Ghibli-style article⬇️ Create Ji with ChatGPT
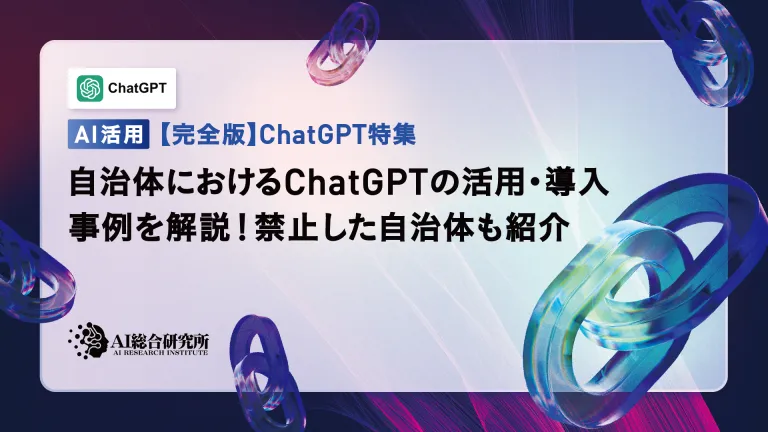
As a new communication method, the use and introduction of ChatGPT in local governments is attracting attention. While this trend is progressing in a wide range of areas, some local governments have declined to use ChatGPT. In this article, we will introduce examples of ChatGPT implementation in local governments. We will explore how we are achieving quality and efficiency improvements in local government services through a variety of reform examples, including supporting document creation and dialogue with citizens. Not only local government officials who aim to reduce staff workload and improve convenience for citizens, but also all interested in advanced use cases.
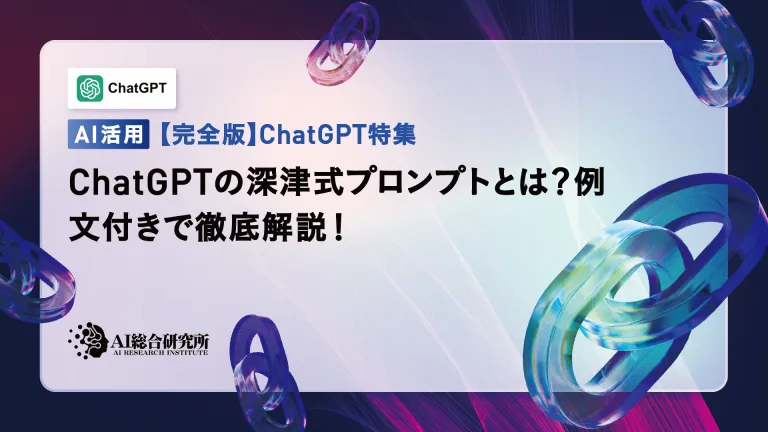
Have you heard of a framework called the "Fukatsu Prompt System"? Language models such as ChatGPT are extremely excellent, but appropriate prompts are essential to maximize their potential. Fukatsu prompts are one of the most popular prompt techniques designed to improve output accuracy. This article explains the principles and characteristics of Fukatsu-style prompts, including specific usage methods and examples. Furthermore, we have introduced other well-known prompt templates and useful techniques for prompt design, so based on these, we will introduce C.
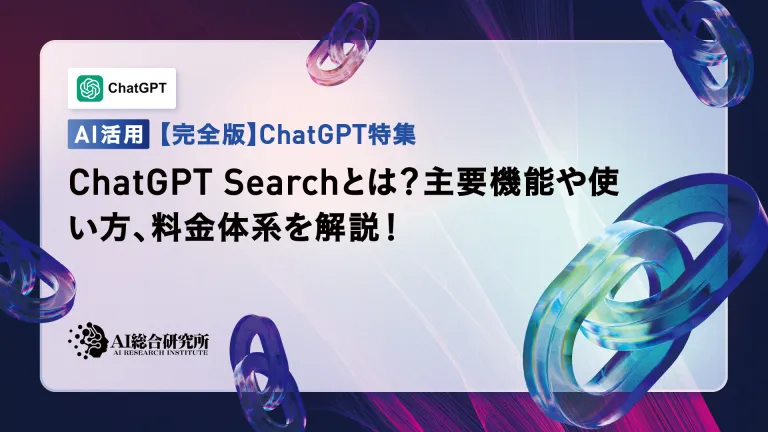
ChatGPT Search: Get the latest information efficiently with an innovative AI search engine! In this article, we will thoroughly explain the new ChatGPT feature "ChatGPT Search," provided by OpenAI. Let's take a closer look at the features, usage, and how this tool can help you improve your information collection efficiency with reliable answers based on real-time web information and intuitive ease of use. ChatGPT Search provides a conversational interactive search experience that answers user questions in a comfortable, hidden environment that hides advertisements
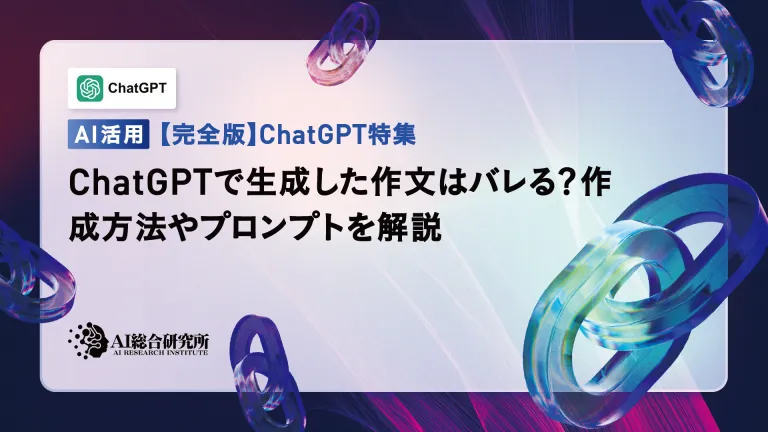
In a modern society with information explosion, it is not easy to create compelling articles. How to use creativity to write articles that attract readers within a limited time and energy requires superb skills and rich experience. At this time, as a revolutionary writing aid, ChatGPT attracted much attention. ChatGPT uses huge data to train language generation models to generate natural, smooth and refined articles. This article will introduce how to effectively use ChatGPT and efficiently create high-quality articles. We will gradually explain the writing process of using ChatGPT, and combine specific cases to elaborate on its advantages and disadvantages, applicable scenarios, and safe use precautions. ChatGPT will be a writer to overcome various obstacles,
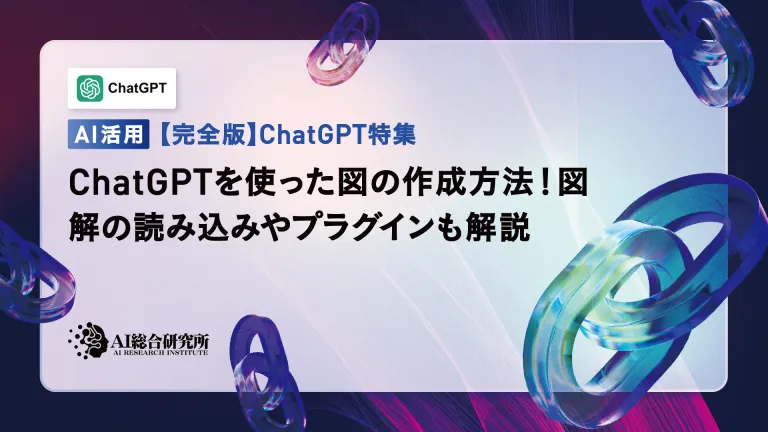
An efficient guide to creating charts using AI Visual materials are essential to effectively conveying information, but creating it takes a lot of time and effort. However, the chart creation process is changing dramatically due to the rise of AI technologies such as ChatGPT and DALL-E 3. This article provides detailed explanations on efficient and attractive diagram creation methods using these cutting-edge tools. It covers everything from ideas to completion, and includes a wealth of information useful for creating diagrams, from specific steps, tips, plugins and APIs that can be used, and how to use the image generation AI "DALL-E 3."
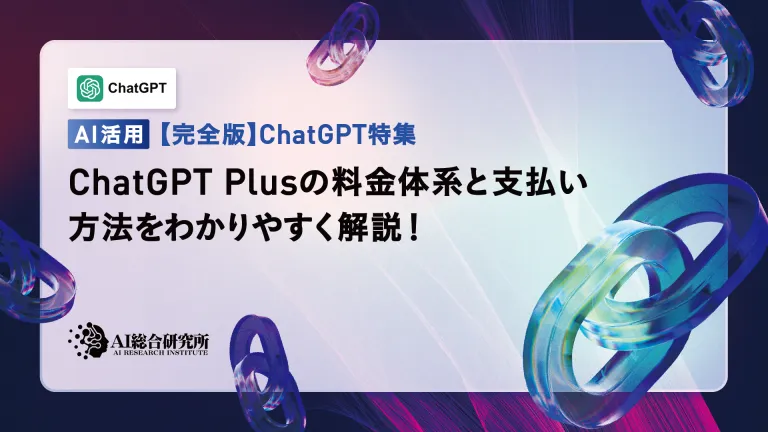
Unlock ChatGPT Plus: Fees, Payment Methods and Upgrade Guide ChatGPT, a world-renowned generative AI, has been widely used in daily life and business fields. Although ChatGPT is basically free, the paid version of ChatGPT Plus provides a variety of value-added services, such as plug-ins, image recognition, etc., which significantly improves work efficiency. This article will explain in detail the charging standards, payment methods and upgrade processes of ChatGPT Plus. For details of OpenAI's latest image generation technology "GPT-4o image generation" please click: Detailed explanation of GPT-4o image generation: usage methods, prompt word examples, commercial applications and differences from other AIs Table of contents ChatGPT Plus Fees Ch
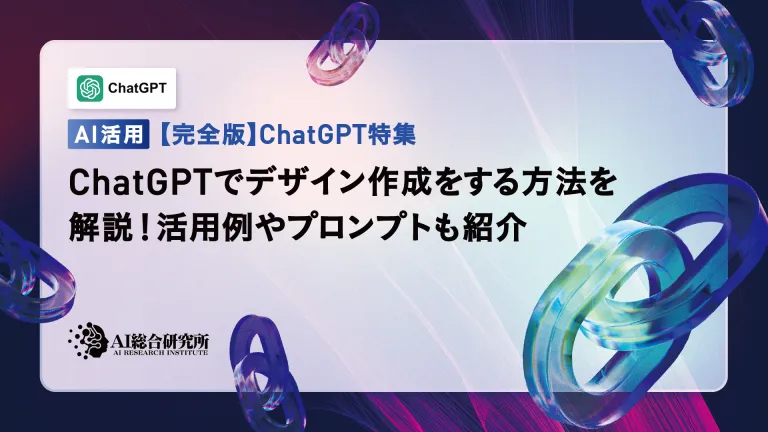
How to use ChatGPT to streamline your design work and increase creativity This article will explain in detail how to create a design using ChatGPT. We will introduce examples of using ChatGPT in various design fields, such as ideas, text generation, and web design. We will also introduce points that will help you improve the efficiency and quality of a variety of creative work, such as graphic design, illustration, and logo design. Please take a look at how AI can greatly expand your design possibilities. table of contents ChatGPT: A powerful tool for design creation


Hot AI Tools

Undresser.AI Undress
AI-powered app for creating realistic nude photos

AI Clothes Remover
Online AI tool for removing clothes from photos.

Undress AI Tool
Undress images for free

Clothoff.io
AI clothes remover

Video Face Swap
Swap faces in any video effortlessly with our completely free AI face swap tool!

Hot Article

Hot Tools
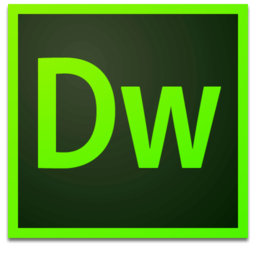
Dreamweaver Mac version
Visual web development tools
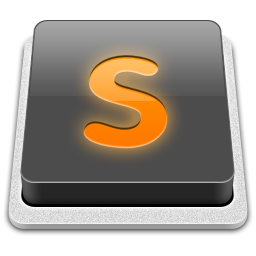
SublimeText3 Mac version
God-level code editing software (SublimeText3)
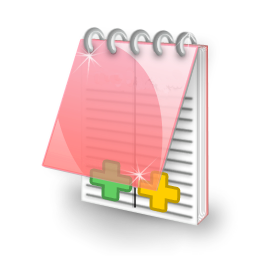
EditPlus Chinese cracked version
Small size, syntax highlighting, does not support code prompt function

MinGW - Minimalist GNU for Windows
This project is in the process of being migrated to osdn.net/projects/mingw, you can continue to follow us there. MinGW: A native Windows port of the GNU Compiler Collection (GCC), freely distributable import libraries and header files for building native Windows applications; includes extensions to the MSVC runtime to support C99 functionality. All MinGW software can run on 64-bit Windows platforms.

SecLists
SecLists is the ultimate security tester's companion. It is a collection of various types of lists that are frequently used during security assessments, all in one place. SecLists helps make security testing more efficient and productive by conveniently providing all the lists a security tester might need. List types include usernames, passwords, URLs, fuzzing payloads, sensitive data patterns, web shells, and more. The tester can simply pull this repository onto a new test machine and he will have access to every type of list he needs.
