LightRAG: A Lightweight Retrieval-Augmented Generation System
Large Language Models (LLMs) are rapidly evolving, but effectively integrating external knowledge remains a significant hurdle. Retrieval-Augmented Generation (RAG) techniques aim to improve LLM output by incorporating relevant information during generation. However, traditional RAG systems can be complex and resource-intensive. The HKU Data Science Lab addresses this with LightRAG, a more efficient alternative. LightRAG combines the power of knowledge graphs with vector retrieval, enabling efficient processing of textual information while maintaining the structured relationships within the data.
Key Learning Points:
- Limitations of traditional RAG and the need for LightRAG.
- LightRAG's architecture: dual-level retrieval and graph-based text indexing.
- Integration of graph structures and vector embeddings for efficient, context-rich retrieval.
- LightRAG's performance compared to GraphRAG across various domains.
Why LightRAG Outperforms Traditional RAG:
Traditional RAG systems often struggle with complex relationships between data points, resulting in fragmented responses. They use simple, flat data representations, lacking contextual understanding. For example, a query about the impact of electric vehicles on air quality and public transport might yield separate results on each topic, failing to connect them meaningfully. LightRAG addresses this limitation.
How LightRAG Functions:
LightRAG uses graph-based indexing and a dual-level retrieval mechanism for efficient and context-rich responses to complex queries.
Graph-Based Text Indexing:
This process involves:
- Chunking: Dividing documents into smaller segments.
- Entity Recognition: Using LLMs to identify and extract entities (names, dates, etc.) and their relationships.
- Knowledge Graph Construction: Building a knowledge graph representing the connections between entities. Redundancies are removed for optimization.
- Embedding Storage: Storing descriptions and relationships as vectors in a vector database.
Dual-Level Retrieval:
LightRAG employs two retrieval levels:
- Low-Level Retrieval: Focuses on specific entities and their attributes or connections. Retrieves detailed, specific data.
- High-Level Retrieval: Addresses broader concepts and themes. Gathers information spanning multiple entities, providing a comprehensive overview.
LightRAG vs. GraphRAG:
GraphRAG suffers from high token consumption and numerous LLM API calls due to its community-based traversal method. LightRAG, using vector-based search and retrieving entities/relationships instead of chunks, significantly reduces this overhead.
LightRAG Performance Benchmarks:
LightRAG was benchmarked against other RAG systems using GPT-4o-mini for evaluation across four domains (Agricultural, Computer Science, Legal, and Mixed). LightRAG consistently outperformed baselines, especially in diversity, particularly on the larger Legal dataset. This highlights its ability to generate varied and rich responses.
Hands-On Python Implementation (Google Colab):
The following steps outline a basic implementation using OpenAI models:
Step 1: Install Libraries
!pip install lightrag-hku aioboto3 tiktoken nano_vectordb !sudo apt update !sudo apt install -y pciutils !pip install langchain-ollama !curl -fsSL https://ollama.com/install.sh | sh !pip install ollama==0.4.2
Step 2: Import Libraries and Set API Key
from lightrag import LightRAG, QueryParam from lightrag.llm import gpt_4o_mini_complete import os os.environ['OPENAI_API_KEY'] = '' # Replace with your key import nest_asyncio nest_asyncio.apply()
Step 3: Initialize LightRAG and Load Data
WORKING_DIR = "./content" if not os.path.exists(WORKING_DIR): os.mkdir(WORKING_DIR) rag = LightRAG(working_dir=WORKING_DIR, llm_model_func=gpt_4o_mini_complete) with open("./Coffe.txt") as f: # Replace with your data file rag.insert(f.read())
Step 4 & 5: Querying (Hybrid and Naive Modes) (Examples provided in the original text)
Conclusion:
LightRAG significantly improves upon traditional RAG systems by addressing their limitations in handling complex relationships and contextual understanding. Its graph-based indexing and dual-level retrieval lead to more comprehensive and relevant responses, making it a valuable advancement in the field.
Key Takeaways:
- LightRAG overcomes traditional RAG's limitations in integrating interconnected information.
- Its dual-level retrieval system adapts to both specific and broad queries.
- Entity recognition and knowledge graph construction optimize information retrieval.
- The combination of graph structures and vector embeddings enhances contextual understanding.
Frequently Asked Questions: (Similar to the original text, but rephrased for conciseness) (This section would be included here, similar to the original.)
(Note: The image URLs remain unchanged.)
The above is the detailed content of LightRAG: Simple and Fast Alternative to GraphRAG. For more information, please follow other related articles on the PHP Chinese website!
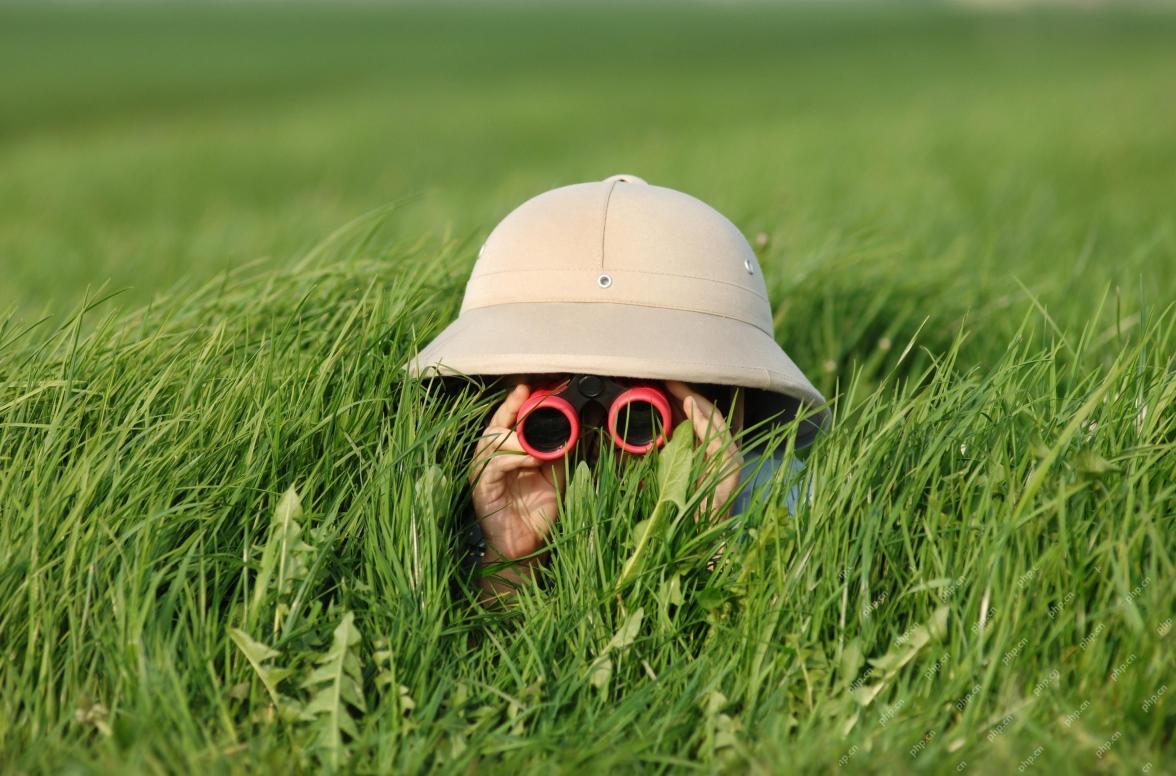
With the explosion of AI applications, enterprises are shifting from traditional search engine optimization (SEO) to generative engine optimization (GEO). Google is leading the shift. Its "AI Overview" feature has served over a billion users, providing full answers before users click on the link. [^2] Other participants are also rapidly rising. ChatGPT, Microsoft Copilot and Perplexity are creating a new “answer engine” category that completely bypasses traditional search results. If your business doesn't show up in these AI-generated answers, potential customers may never find you—even if you rank high in traditional search results. From SEO to GEO – What exactly does this mean? For decades
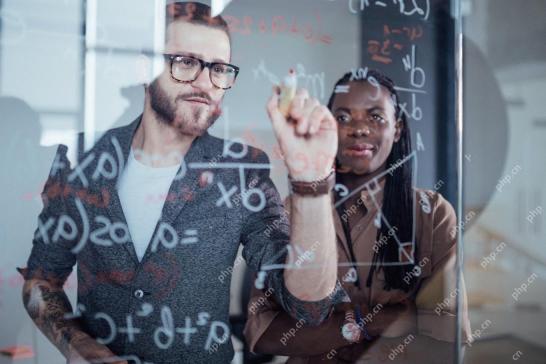
Let's explore the potential paths to Artificial General Intelligence (AGI). This analysis is part of my ongoing Forbes column on AI advancements, delving into the complexities of achieving AGI and Artificial Superintelligence (ASI). (See related art
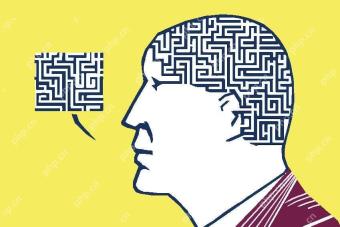
Human-computer interaction: a delicate dance of adaptation Interacting with an AI chatbot is like participating in a delicate dance of mutual influence. Your questions, responses, and preferences gradually shape the system to better meet your needs. Modern language models adapt to user preferences through explicit feedback mechanisms and implicit pattern recognition. They learn your communication style, remember your preferences, and gradually adjust their responses to fit your expectations. Yet, while we train our digital partners, something equally important is happening in the reverse direction. Our interactions with these systems are subtly reshaping our own communication patterns, thinking processes, and even expectations of interpersonal conversations. Our interactions with AI systems have begun to reshape our expectations of interpersonal interactions. We adapted to instant response,
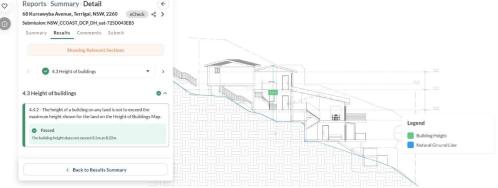
AI Streamlines Wildfire Recovery Permitting Australian tech firm Archistar's AI software, utilizing machine learning and computer vision, automates the assessment of building plans for compliance with local regulations. This pre-validation significan
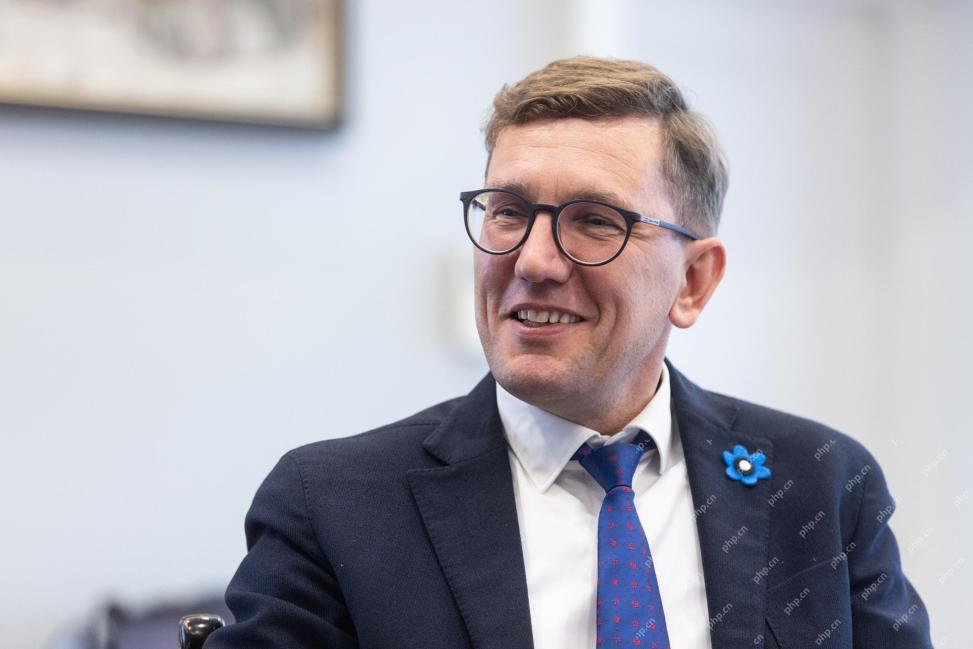
Estonia's Digital Government: A Model for the US? The US struggles with bureaucratic inefficiencies, but Estonia offers a compelling alternative. This small nation boasts a nearly 100% digitized, citizen-centric government powered by AI. This isn't
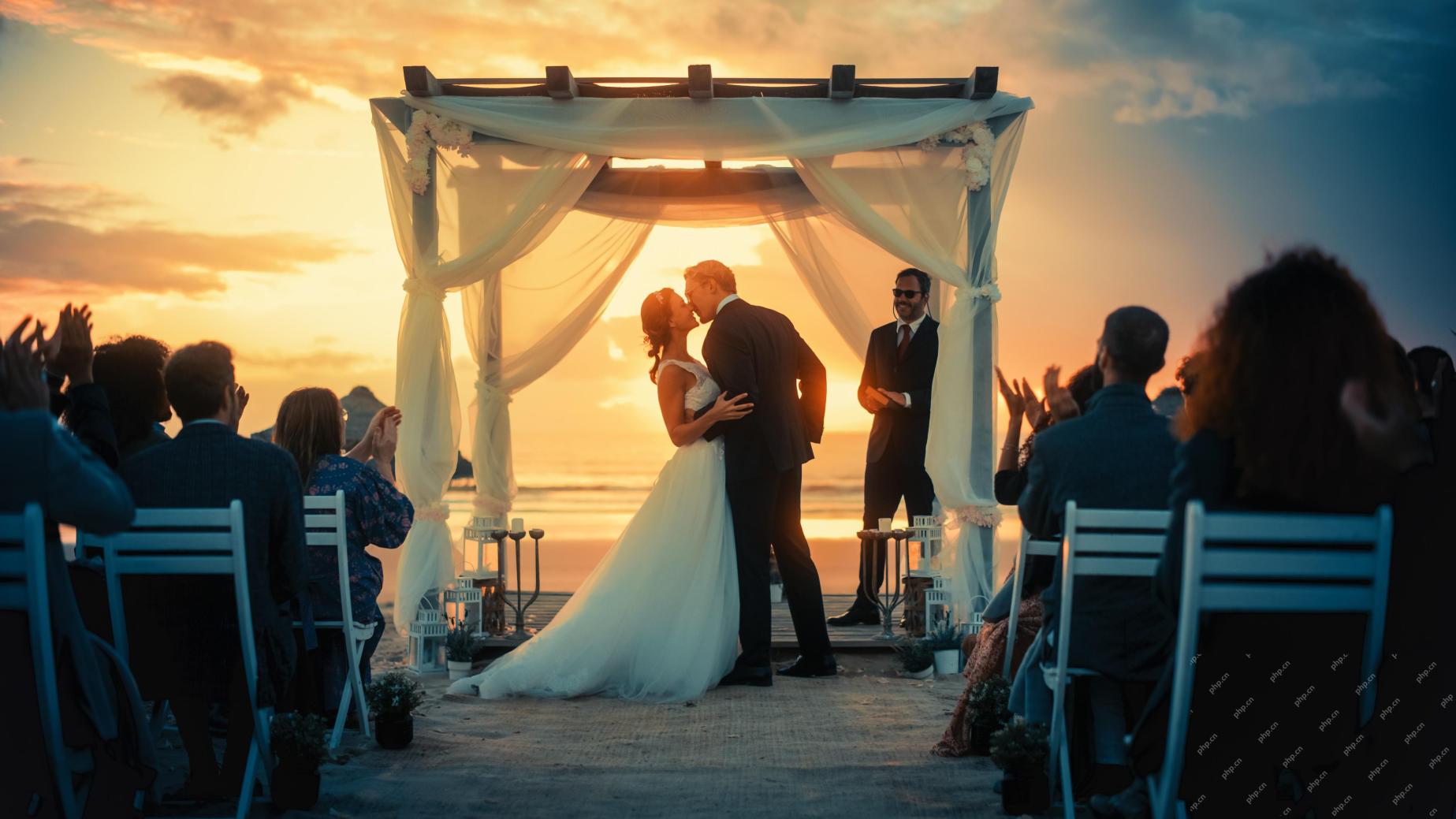
Planning a wedding is a monumental task, often overwhelming even the most organized couples. This article, part of an ongoing Forbes series on AI's impact (see link here), explores how generative AI can revolutionize wedding planning. The Wedding Pl
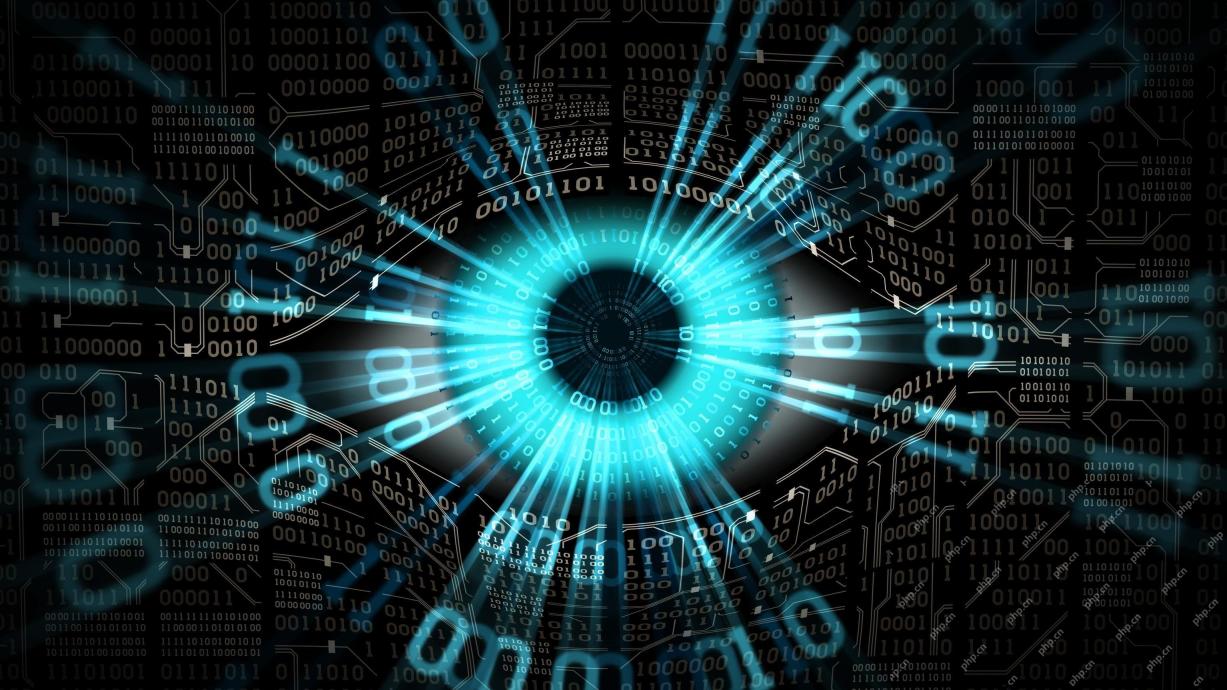
Businesses increasingly leverage AI agents for sales, while governments utilize them for various established tasks. However, consumer advocates highlight the need for individuals to possess their own AI agents as a defense against the often-targeted
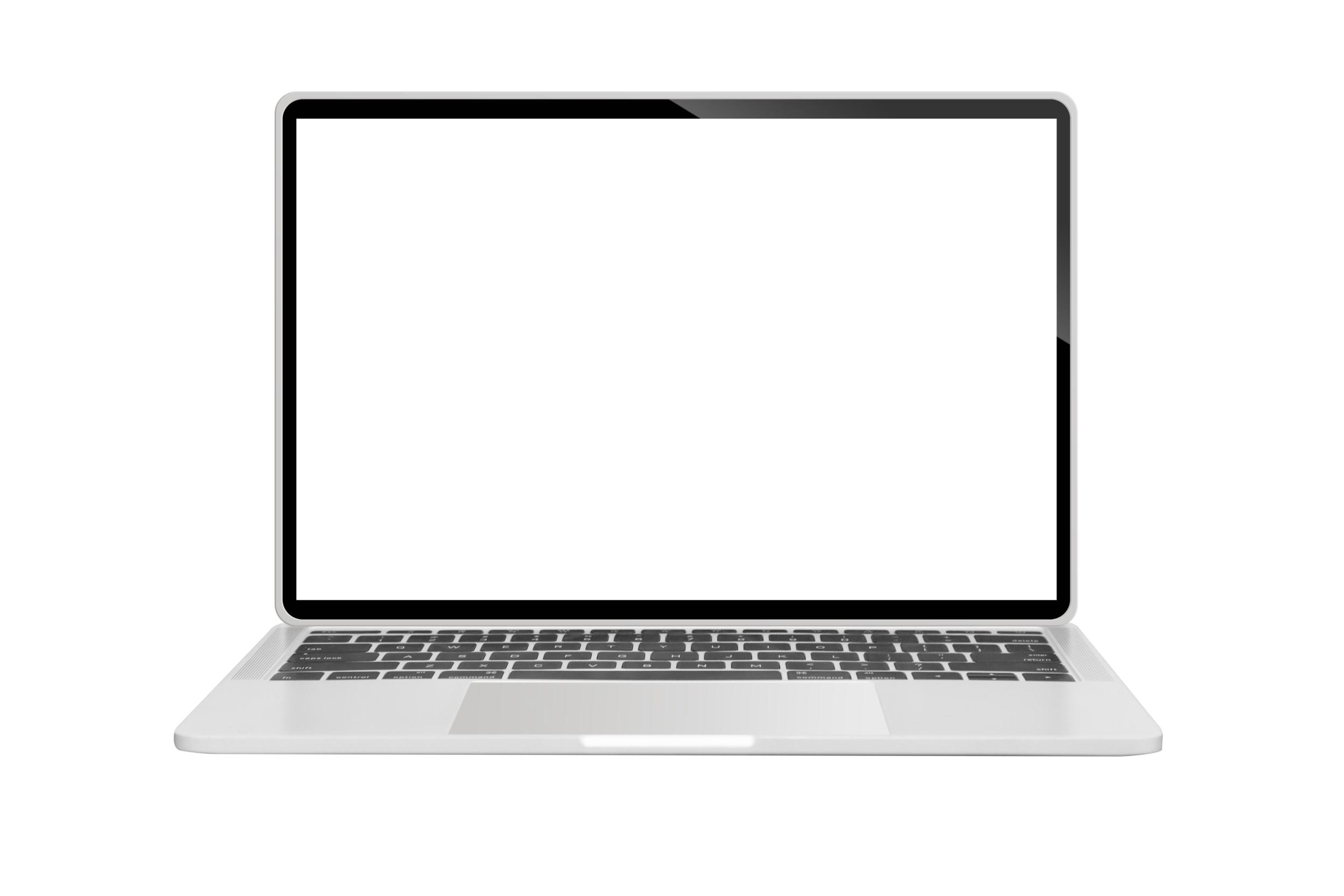
Google is leading this shift. Its "AI Overviews" feature already serves more than one billion users, providing complete answers before anyone clicks a link.[^2] Other players are also gaining ground fast. ChatGPT, Microsoft Copilot, and Pe


Hot AI Tools

Undresser.AI Undress
AI-powered app for creating realistic nude photos

AI Clothes Remover
Online AI tool for removing clothes from photos.

Undress AI Tool
Undress images for free

Clothoff.io
AI clothes remover

Video Face Swap
Swap faces in any video effortlessly with our completely free AI face swap tool!

Hot Article

Hot Tools

Atom editor mac version download
The most popular open source editor

DVWA
Damn Vulnerable Web App (DVWA) is a PHP/MySQL web application that is very vulnerable. Its main goals are to be an aid for security professionals to test their skills and tools in a legal environment, to help web developers better understand the process of securing web applications, and to help teachers/students teach/learn in a classroom environment Web application security. The goal of DVWA is to practice some of the most common web vulnerabilities through a simple and straightforward interface, with varying degrees of difficulty. Please note that this software

SublimeText3 Linux new version
SublimeText3 Linux latest version
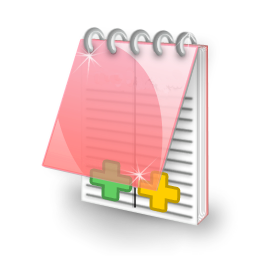
EditPlus Chinese cracked version
Small size, syntax highlighting, does not support code prompt function

Notepad++7.3.1
Easy-to-use and free code editor
