What is an LLM Agent?
Understanding LLM Agents
An LLM (Large Language Model) agent is a software program that leverages the capabilities of a large language model to interact with its environment and achieve specific goals. Unlike a simple chatbot that only responds to prompts, an LLM agent actively plans, executes actions, and learns from its experiences. Think of it as a sophisticated AI assistant that can autonomously perform tasks, rather than simply providing information. This involves a crucial feedback loop: the agent takes action, observes the results, and uses that information to refine its future actions. This iterative process distinguishes it from simpler LLM applications. The agent's interaction with the environment can involve various modalities, such as accessing and manipulating databases, interacting with APIs, browsing the web, or even controlling physical robots. The key is its ability to autonomously decide what actions to take based on its understanding of its goals and the current state of the environment. The agent's "brain" is the LLM, providing the intelligence to understand, plan, and adapt.
What are the capabilities of an LLM agent?
Capabilities of LLM Agents
The capabilities of an LLM agent are largely determined by the underlying LLM and the design of the agent itself. However, some common capabilities include:
- Planning and Goal-Oriented Behavior: LLM agents can break down complex tasks into smaller, manageable steps, creating a plan to achieve a specific goal. This involves reasoning about the necessary actions and their order.
- Information Gathering and Retrieval: They can access and process information from various sources, such as databases, APIs, or the internet, to gather relevant data for decision-making. This ability allows them to stay informed and adapt to changing circumstances.
- Decision Making and Reasoning: Based on the information gathered and the established plan, LLM agents can make informed decisions and choose the most appropriate actions to take. This involves a degree of logical reasoning and problem-solving.
- Action Execution: This capability allows the agent to interact with its environment. This might involve sending emails, making API calls, modifying databases, or controlling physical robots depending on the agent's design and intended purpose.
- Learning and Adaptation: Through feedback loops, LLM agents can learn from their successes and failures, refining their strategies and improving their performance over time. This learning can be implicit, through the LLM's internal parameter adjustments, or explicit, through reinforcement learning techniques.
- Multi-modal Interaction: Advanced LLM agents can interact with the environment through various modalities, such as text, images, and audio. This expands their capabilities significantly, allowing for richer and more nuanced interactions.
How can I build an LLM agent?
Building an LLM Agent: A Step-by-Step Guide
Building an LLM agent involves several key steps:
- Define the Agent's Goal and Scope: Clearly define what the agent should achieve. This will guide the design and development process. A well-defined scope prevents the project from becoming overly ambitious.
- Choose an LLM: Select an appropriate LLM based on the agent's requirements. Consider factors like performance, cost, and API access. Popular choices include GPT-3, GPT-4, PaLM 2, and others.
- Design the Agent's Architecture: This involves determining how the agent will interact with its environment and process information. This might involve designing a state machine, a hierarchical planning system, or another suitable architecture.
- Develop the Agent's Code: Implement the agent's logic using a programming language like Python. This will involve integrating the chosen LLM, implementing the agent's decision-making process, and handling interactions with external systems.
- Integrate with External Systems: Connect the agent to necessary APIs, databases, or other resources it needs to interact with its environment. This might involve using libraries for web scraping, database access, or API communication.
- Test and Iterate: Thoroughly test the agent's performance and iterate on its design and implementation to improve its capabilities. This involves evaluating its success rate, identifying areas for improvement, and refining its decision-making processes.
- Implement a Feedback Loop: Create a mechanism to collect feedback on the agent's actions and use this feedback to improve its future performance. This is crucial for enabling learning and adaptation.
What are the limitations of using LLM agents?
Limitations of LLM Agents
While LLM agents offer significant potential, they also have several limitations:
- Hallucinations and Inaccurate Information: LLMs can sometimes generate incorrect or nonsensical information ("hallucinations"). This is a significant concern, especially when the agent makes decisions based on inaccurate data. Careful validation and verification mechanisms are crucial.
- Bias and Ethical Concerns: LLMs are trained on large datasets that may contain biases. These biases can be reflected in the agent's behavior, leading to unfair or discriminatory outcomes. Addressing bias in training data and agent design is essential.
- Computational Cost: Running LLMs can be computationally expensive, especially for complex tasks. This can limit the scalability and affordability of LLM agents.
- Lack of Common Sense and Real-World Understanding: While LLMs are powerful, they lack true common sense and an intuitive understanding of the physical world. This can lead to unexpected errors or failures in real-world scenarios.
- Security Risks: If an LLM agent interacts with sensitive data or external systems, it can pose security risks. Robust security measures are essential to prevent unauthorized access or manipulation.
- Interpretability and Explainability: Understanding why an LLM agent made a particular decision can be challenging. This lack of transparency can make it difficult to debug errors or ensure accountability.
These limitations highlight the need for careful design, thorough testing, and ongoing monitoring of LLM agents to ensure their safe and effective deployment.
The above is the detailed content of What is an LLM Agent. For more information, please follow other related articles on the PHP Chinese website!
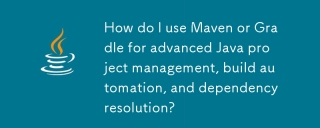
The article discusses using Maven and Gradle for Java project management, build automation, and dependency resolution, comparing their approaches and optimization strategies.
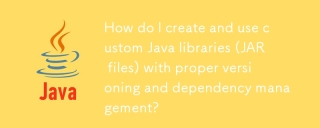
The article discusses creating and using custom Java libraries (JAR files) with proper versioning and dependency management, using tools like Maven and Gradle.
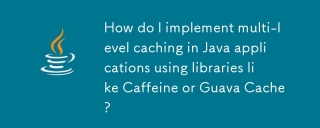
The article discusses implementing multi-level caching in Java using Caffeine and Guava Cache to enhance application performance. It covers setup, integration, and performance benefits, along with configuration and eviction policy management best pra
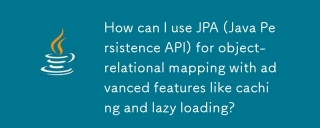
The article discusses using JPA for object-relational mapping with advanced features like caching and lazy loading. It covers setup, entity mapping, and best practices for optimizing performance while highlighting potential pitfalls.[159 characters]
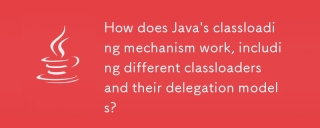
Java's classloading involves loading, linking, and initializing classes using a hierarchical system with Bootstrap, Extension, and Application classloaders. The parent delegation model ensures core classes are loaded first, affecting custom class loa


Hot AI Tools

Undresser.AI Undress
AI-powered app for creating realistic nude photos

AI Clothes Remover
Online AI tool for removing clothes from photos.

Undress AI Tool
Undress images for free

Clothoff.io
AI clothes remover

AI Hentai Generator
Generate AI Hentai for free.

Hot Article

Hot Tools

mPDF
mPDF is a PHP library that can generate PDF files from UTF-8 encoded HTML. The original author, Ian Back, wrote mPDF to output PDF files "on the fly" from his website and handle different languages. It is slower than original scripts like HTML2FPDF and produces larger files when using Unicode fonts, but supports CSS styles etc. and has a lot of enhancements. Supports almost all languages, including RTL (Arabic and Hebrew) and CJK (Chinese, Japanese and Korean). Supports nested block-level elements (such as P, DIV),

Safe Exam Browser
Safe Exam Browser is a secure browser environment for taking online exams securely. This software turns any computer into a secure workstation. It controls access to any utility and prevents students from using unauthorized resources.
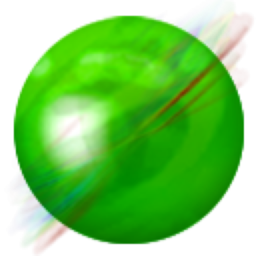
ZendStudio 13.5.1 Mac
Powerful PHP integrated development environment
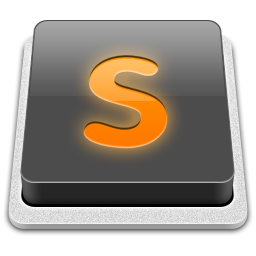
SublimeText3 Mac version
God-level code editing software (SublimeText3)
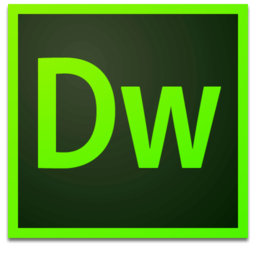
Dreamweaver Mac version
Visual web development tools