DeepSeek-R1’s advanced reasoning capabilities have made it the new leader in the generative LLM field. It has caused a stir in the AI industry, with reports of Nvidia’s $600 billion loss post-launch. But what makes DeepSeek-R1 so famous overnight? In this article, we’ll explore why DeepSeek-R1 is gaining so much attention, delve into its groundbreaking capabilities, and analyze how its reasoning powers are reshaping real-world applications. Stay tuned as we break down the model’s performance through a detailed, structured analysis.
Learning Objectives
- Understand DeepSeek-R1’s advanced reasoning capabilities and its impact on the LLM landscape.
- Learn how Group Relative Policy Optimization (GRPO) enhances reinforcement learning without a Critic model.
- Explore the differences between DeepSeek-R1-Zero and DeepSeek-R1 in terms of training and performance.
- Analyze the evaluation metrics and benchmarks that showcase DeepSeek-R1’s superiority in reasoning tasks.
- Discover how DeepSeek-R1 optimizes STEM and coding tasks with scalable, high-throughput AI models.
This article was published as a part of theData Science Blogathon.
Table of contents
- What is Deepseek-R1?
- What is Group Relative Policy Optimization (GRPO)?
- Training Process and Optimization in DeepSeek-R1-Zero
- How does the GRPO Work?
- Enhancing Reasoning and General Capabilities in DeepSeek-R1
- Evaluation of DeepSeek-R1
- Evaluating Reasoning Capabilities of DeepSeek-R1-7B
- Advanced Reasoning and Problem-Solving Scenario
- Conclusion
- Frequently Asked Questions
What is Deepseek-R1?
In simple words, DeepSeek-R1 is a cutting-edge language model series developed by DeepSeek, established in 2023 by Liang Wenfeng. It achieved advanced reasoning capabilities in LLMs through reinforcement learning(RL). There are two variants:
DeepSeek-R1-Zero
It is trained purely via RL on the base model without supervised fine-tuned (SFT), and it autonomously develops advanced reasoning behavior like self-verification and multi-step reflection, achieving 71% accuracy on the AIME 2024 benchmark
DeepSeek-R1
It was enhanced with cold-start data and multi-stage training (RL SFT), it addresses readability issues and outperforms OpenAI’s o1 on tasks like MATH-500 (97.3% accuracy) and coding challenges (Codeforces rating 2029)
DeepSeek uses Group Relative Policy Optimization(GRPO), an RL technique that does not use the Critic model and saves RL’s training costs. GRPO optimizes policies by grouping outputs and normalizing rewards, eliminating the need for the Critic models.
The project also distills its reasoning patterns into smaller models (1.5B-70B), enabling efficient deployment. According to the benchmark It’s 7B model surpasses GPT-4o.
DeepSeek-R1 Paper here.
Comparison Chart
Model | GPQA | LiveCode | Diamond Bench | CodeForces pass@1 cons@64 | CodeForces pass@1 | Rating |
---|---|---|---|---|---|---|
OpenAI-01-mini | 63.6 | 80.0 | 90.0 | 60.0 | 53.8 | 1820 |
OpenAI-01-0912 | 74.4 | 83.3 | 94.8 | 77.3 | 63.4 | 1843 |
DeepSeek-R1-Zero | 71.0 | 86.7 | 95.9 | 73.3 | 50.0 | 1444 |
Accuracy Plot of Deepseek-R1-Zero on AIME Dataset
DeepSeek open-sourced the models, training pipelines, and benchmarks aim to democratize RL-driven reasoning research, offering scalable solutions for STEM, coding, and knowledge-intensive tasks. DeepSeek-R1 directs a path to the new era of low-cost, high-throughput SLMs and LLMs.
What is Group Relative Policy Optimization (GRPO)?
Before going into the cutting-edge GRPO, let’s surf on some basics of Reinforcement Learning(RL).
Reinforcement Learning is the interaction between the Agent and Environment. During training, the agent takes actions so that it maximizes the cumulative rewards. Think about a bot playing Chess or a Robot on a factory floor trying to do tasks with actual items.
The agent is learning by doing. It gets a reward when it does things right; otherwise, it gets negative. By doing these repetitive trials, it will be on a journey to find the optimal strategy to adapt to the unknown environment.
Here is the simple diagram of Reinforcement Learning, It has 3 components:
Core RL Loop
- Agent which takes actions based on the learned policy.
- Action is the decision made by the agent at a given state.
- The environment is the external system (game, workshop floor, flying drone, etc) where the agent operates and learns by interacting.
- The environment provides feedback to the agent in the form of new state and rewards.
Agent Components
- Value function estimates how good a particular state or action is in terms of long-term rewards
- Policy is a strategy that defines the agent’s action selection.
- The value function informs the policy by helping it improve decision-making
- The policy guides (Guides Relationship) the agent in choosing actions in the RL Loops
Learning Elements
- Experience, here the agent collects transactions while interacting with the environment.
- Optimization or Policy updates use the experience to refine the policy and important decision-making.
Training Process and Optimization in DeepSeek-R1-Zero
The experience gathered is used to update the policy through optimization. The value function provides insights to refine the policy. The policy guides the agent, which interacts with the environment to collect new experiences and the cycle goes on until the agent learns the optimum strategy or improves to adapt to the environment.
In the training of DeepSeek-R1-Zero, they use Group Relative Policy optimization or GRPO, it eliminate the Critic Model and lowers the training cost.
As for my understanding of the DeepSeek-R1 Research Paper, here is the schematic training process of the DeepSeek-R1-Zero and DeepSeek-R1 models.
Tentative DeepSeek-R1-Zero and R1 Training Diagram
How does the GRPO Work?
For each question q, GRPO samples a group of output {o1, o2, o2..} from the old policy and optimizes the policy model by maximizing the below objective:
Here epsilon and beta are hyper-parameters, and A_i is the advantage computed using a group of rewards {r1, r2, r3…rG} corresponding to the output within each group.
Advantage Calculation
In the Advantage calculation, Normalize rewards within group outputs, r_i is the reward for output I and r_group is the rewards of all output in the group.
To maximize the clipped policy updates with KL penalty,
Kullback-Leibler Divergence
The KL Divergence also known as Relative Entropy is a statistical distance function, that measures the difference between the models’s probability distribution (Q) and true probability distribution (P).
For more KL-Divergence
The below equation is the mathematical form of KL-Divergence:
Relative entropy or KL distance is always a non-negative real number. It has the lowest value of 0 if and only if the Q and P are identical. That means both the Model Probability distribution(Q) and True Probability distribution (P) overlap or a perfect system.
Example of KL Divergence
Here are simple examples to showcase KL divergence,
We will use the entropy function from the Scipy Statistical package, It will calculate the relative entropy between two distributions.
import numpy as np import matplotlib.pyplot as plt from scipy.stats import entropy
# Define two probability distributions P and Q x = np.linspace(-3, 3, 100) P = np.exp(-(x**2)) # Gaussian-like distribution Q = np.exp(-((x - 1) ** 2)) # Shifted Gaussian # Normalize to ensure they sum to 1 P /= P.sum() Q /= Q.sum() # Compute KL divergence kl_div = entropy(P, Q)
Our P and Q as Gaussian-like and shifted Gaussian distribution respectively.
plt.style.use("ggplot") plt.figure(figsize=(12, 8)) plt.plot(x, P, label="P (Original)", line, color="blue") plt.plot(x, Q, label="Q (Shifted)", line, color="red") plt.fill_between(x, P, Q, color="yellow", alpha=0.3, label="Difference") plt.title(f"KL Divergence: {kl_div:.4f}") plt.xlabel("x") plt.ylabel("Probability Density") plt.legend() plt.show()
The yellow portion is the KL difference between P and Q.
In the GRPO equation, GRPO samples a group of outputs for each query and computes advantages relative to the group’s mean and standard deviation. This avoids training a separate critic model. The objective includes a clipped ratio and KL penalty to stay close to the reference policy.
The ratio part is the probability ratio of the new and old policy.Clip(ratio) is bound between 1-epsilon and 1 epsilon.
The conversation process between User and Assistant
The user asks a question, and the model or assistant solves it by first thinking about the reasoning process and then responding to the user.
The reasoning and answer are enclosed in the below diagram.
import numpy as np import matplotlib.pyplot as plt from scipy.stats import entropy
The Self-Evolution Process of DeepSeek-R1-Zero demonstrates how Reinforcement Learning can improve the model’s reasoning capabilities autonomously. The chart shows how the model’s reasoning capabilities for handling complex reasoning tasks evolve.
Enhancing Reasoning and General Capabilities in DeepSeek-R1
DeepSeek-R1, answers two significant questions that arise after promising results of the Zero model.
- Can reasoning performance be further improved?
- How can we train a user-friendly model that not only produces a clear and coherent Chain Of Thought (CoT) but also demonstrates strong general capabilities?
The DeepSeek-R1 uses Cold-Start Data in a format where the developer collects thousands of cold-start data to fine-tune the DeepSeek-V3-Base as a starting point of RL.
These data have two important advantages compared to DeepSeek-R1-zero.
- Readability: A key limitation of the Zero model is that its content is not suitable for reading. The responses are mixed with many languages, and not well formatted to highlight answers for users.
- Potential: Expert lead designing the pattern for cold-start data to help better performance against DeepSeek-R1-Zero.
Evaluation of DeepSeek-R1
According to the DeepSeek-R1 paper, They (the developer)set the maximum generation length to 32768 tokens for the models. They found long output reasoning model result in higher repetition rates with greedy decoding and significant variability. Therefore, they use pass@k evaluation, It use a sampling temperature of 0.6 and a top-p value of 0.95 to generate k numbers response for each question.
Pass@1 is then calculated as:
Here, P_i denotes the correctness of the i-th response, according to the research paper this method ensures more reliable performance estimates.
We can see that the education-oriented knowledge benchmarks such as MMLU, MMLU-Pro, GPQA Diamond, and DeepSeek-R1 perform better compared to DeepSeek-V3. It has primarily enhanced accuracy in STEM-related questions. DeepSeek-R1 also delivers great results on IF-Eval, a benchmark data designed to assess the model’s ability to follow format instructions.
Enough maths and theoretical understanding has been done, which I wish significantly boost your overall knowledge of Reinforcement Learning and its cutting-edge application on DeepSeek-R1 model development. Now we will get our hands on DeepSeek-R1 using Ollama and taste the newly minted LLM.
Evaluating Reasoning Capabilities of DeepSeek-R1-7B
The evaluation of DeepSeek-R1-7B focuses on its enhanced reasoning capabilities, particularly its performance in complex problem-solving scenarios. By analyzing key benchmarks, this assessment provides insights into how effectively the model handles intricate reasoning tasks compared to its predecessors.
What We Want to Achieve
- Evaluate DeepSeek-R1’s reasoning capabilities across different cognitive domains
- Identify strengths and limitations in specific reasoning tasks
- Understand the model’s potential real-world applications
Setup the Environment
- Install Ollama fromhere
- After installing it to your system open your terminal and type the below command, it will download and start the DeepSeek-R1 7B model.
import numpy as np import matplotlib.pyplot as plt from scipy.stats import entropy
Now I put a Linear inequality question from NCERT
Q.Solve 4x 3
and the response is:
Which is accurate according to the book.
Amazing!!
Now will set up a testing environment using Llamaindex which will be a more prominent way to do this.
Setup Testing Environment
# Define two probability distributions P and Q x = np.linspace(-3, 3, 100) P = np.exp(-(x**2)) # Gaussian-like distribution Q = np.exp(-((x - 1) ** 2)) # Shifted Gaussian # Normalize to ensure they sum to 1 P /= P.sum() Q /= Q.sum() # Compute KL divergence kl_div = entropy(P, Q)
Now we install the necessary packages
Install Packages
plt.style.use("ggplot") plt.figure(figsize=(12, 8)) plt.plot(x, P, label="P (Original)", line, color="blue") plt.plot(x, Q, label="Q (Shifted)", line, color="red") plt.fill_between(x, P, Q, color="yellow", alpha=0.3, label="Difference") plt.title(f"KL Divergence: {kl_div:.4f}") plt.xlabel("x") plt.ylabel("Probability Density") plt.legend() plt.show()
Now Open VScode and create a Jupyter Notebook name prompt_analysis.ipynb root of the project folder.
Import Libraries
<think> reasoning process</think> <answer> answer here </answer> USER: Prompt Assistant: Answer
You must stay running ollama deepseek-r1:7b on your terminal.
Now, start with the mathematical problem
Imporant: OUTPUT will be very long so the output in this blog will be abridged, For full output you must see the blog’s code repository here.
Advanced Reasoning and Problem-Solving Scenario
This section explores complex problem-solving tasks that require a deep understanding of various reasoning techniques, from mathematical calculations to ethical dilemmas. By engaging with these scenarios, you will enhance your ability to think critically, analyze data, and draw logical conclusions across diverse contexts.
Mathematical Problem: Discount and Loyalty Card Calculation
A store offers a 20% discount on all items. After applying the discount, there’s an additional 10% off for loyalty card members. If an item originally costs $150, what is the final price for a loyalty card member? Show your step-by-step calculation and explain your reasoning.
import numpy as np import matplotlib.pyplot as plt from scipy.stats import entropy
Output:
The key aspect of this prompt is:
- Sequential calculation ability
- Understanding of percentage concepts
- Step-by-step reasoning
- Clarity of explanation.
Logical Reasoning: Identifying Contradictions in Statements
Consider these statements: All birds can flyPenguins are birdsPenguins cannot flyIdentify any contradictions in these statements. If there are contradictions, explain how to resolve them using logical reasoning.
# Define two probability distributions P and Q x = np.linspace(-3, 3, 100) P = np.exp(-(x**2)) # Gaussian-like distribution Q = np.exp(-((x - 1) ** 2)) # Shifted Gaussian # Normalize to ensure they sum to 1 P /= P.sum() Q /= Q.sum() # Compute KL divergence kl_div = entropy(P, Q)
Output:
This will show Logical consistency, Propose logical solutions, understand class relationships, and syllogistic reasoning.
Causal Chain Analysis: Ecosystem Impact of a Disease on Wolves
In a forest ecosystem, a disease kills 80% of the wolf population. Describe the potential chain of effects this might have on the ecosystem over the next 5 years. Include at least three levels of cause and effect, and explain your reasoning for each step.
plt.style.use("ggplot") plt.figure(figsize=(12, 8)) plt.plot(x, P, label="P (Original)", line, color="blue") plt.plot(x, Q, label="Q (Shifted)", line, color="red") plt.fill_between(x, P, Q, color="yellow", alpha=0.3, label="Difference") plt.title(f"KL Divergence: {kl_div:.4f}") plt.xlabel("x") plt.ylabel("Probability Density") plt.legend() plt.show()
Output:
This prompt model shows the understanding of complex systems, tracks multiple casual chains, considers indirect effects, and applies domain knowledge.
Pattern Recognition: Identifying and Explaining Number Sequences
Consider this sequence: 2, 6, 12, 20, 30, __What’s the next number?
- Explain the pattern
- Create a formula for the nth term.
- Verify your formula works for all given numbers
<think> reasoning process</think> <answer> answer here </answer> USER: Prompt Assistant: Answer
Output:
Model excels at identifying numerical patterns, generating mathematical formulas, explaining the reasoning process, and verifying the solution.
Probability Problem: Calculating Probabilities with Marbles
A bag contains 3 red marbles, 4 blue marbles, and 5 green marbles. If you draw two marbles without replacement:
- What’s the probability of drawing two blue marbles?
- What’s the probability of drawing marbles of different colors?
Show all calculations and explain your approach.
import numpy as np import matplotlib.pyplot as plt from scipy.stats import entropy
Output:
The model can calculate probabilities, handle conditional problems, and explain probabilistic reasoning.
Debugging: Logical Errors in Code and Their Solutions
This code has logical errors that prevent it from running correctly.
# Define two probability distributions P and Q x = np.linspace(-3, 3, 100) P = np.exp(-(x**2)) # Gaussian-like distribution Q = np.exp(-((x - 1) ** 2)) # Shifted Gaussian # Normalize to ensure they sum to 1 P /= P.sum() Q /= Q.sum() # Compute KL divergence kl_div = entropy(P, Q)
- Identify all potential problems
- Explain why each is a problem
- Provide a corrected version
- Explain why your solution is better
plt.style.use("ggplot") plt.figure(figsize=(12, 8)) plt.plot(x, P, label="P (Original)", line, color="blue") plt.plot(x, Q, label="Q (Shifted)", line, color="red") plt.fill_between(x, P, Q, color="yellow", alpha=0.3, label="Difference") plt.title(f"KL Divergence: {kl_div:.4f}") plt.xlabel("x") plt.ylabel("Probability Density") plt.legend() plt.show()
Output:
DeepSeek-R1 finds edge cases, understands error conditions, applies correction, and explains the technical solution.
Comparative Analysis: Electric vs. Gasoline Cars
Compare electric cars and traditional gasoline cars in terms of:
- Environmental impact
- Long-term cost
- Convenience
- Performance
For each factor, provide specific examples and data points. Then, explain which type of car would be better for:
- A city dweller with a short commute
- A traveling salesperson who drives 30,000 miles annually
Justify your recommendations.
<think> reasoning process</think> <answer> answer here </answer> USER: Prompt Assistant: Answer
Output:
It is a huge response, I loved the reasoning process. It analyzes multiple factors, considers context, makes nice recommendations, and balances competing priorities.
Ethical Dilemma: Decision-Making in Self-Driving Cars
A self-driving car must make a split-second decision:
- Swerve left: Hit two pedestrians
- Swerve right: Hit a wall, seriously injuring the passenger
- Swerve right: Hit a wall, seriously injuring the passenger
What should the car do? Provide your reasoning, considering:
- Ethical frameworks used
- Assumptions made
- Priority hierarchy
- Long-term implications
$ollama run deepseek-r1:7b
Output:
These types of problems are most problematic for the generative AI models. It tests ethical reasoning, multiple perspectives, moral dilemmas, and value judgments. Overall, it was one well. I think more ethical domain-specific fine-tuning will produce a more profound response.
Statistical Analysis: Evaluating Study Claims on Coffee Consumption
A study claims that coffee drinkers live longer than non-coffee drinkers. The study observed 1000 people aged 40-50 for 5 years.
Identify:
- Potential confounding variables
- Sampling biases
- Alternative explanations
- What additional data would strengthen or weaken the conclusion?
import numpy as np import matplotlib.pyplot as plt from scipy.stats import entropy
Output:
It understands the statistical concepts well enough, identifies research limitations, and critical thinking on data, and proposes methodological improvements.
Time Series Analysis
# Define two probability distributions P and Q x = np.linspace(-3, 3, 100) P = np.exp(-(x**2)) # Gaussian-like distribution Q = np.exp(-((x - 1) ** 2)) # Shifted Gaussian # Normalize to ensure they sum to 1 P /= P.sum() Q /= Q.sum() # Compute KL divergence kl_div = entropy(P, Q)
Output:
DeepSeek loves Mathematical problems, handles exponential decay, provides good mathematical models, and provides calculations.
Scheduling Task
plt.style.use("ggplot") plt.figure(figsize=(12, 8)) plt.plot(x, P, label="P (Original)", line, color="blue") plt.plot(x, Q, label="Q (Shifted)", line, color="red") plt.fill_between(x, P, Q, color="yellow", alpha=0.3, label="Difference") plt.title(f"KL Divergence: {kl_div:.4f}") plt.xlabel("x") plt.ylabel("Probability Density") plt.legend() plt.show()
Output:
It can handle multiple constraints, produce optimized schedules, and provide the problem-solving process.
Cross-Domain Analysis
<think> reasoning process</think> <answer> answer here </answer> USER: Prompt Assistant: Answer
Output:
It nicely done the job of comparing different types of domains together which is very impressive. This type of reasoning helps different types of domains entangle together so one domain’s problems can be solved by the solutions from other domains. It helps research on the cross-domain understanding.
Although, there are plenty of example prompts you can experiment with the model on your local systems without spending any penny. I will use DeepSeek-R1 for more research, and learning about different areas. All you need is a Laptop, your time, and a nice place.
All the code used in this article here.
Conclusion
DeepSeek-R1 shows promising capabilities across various reasoning tasks, showcasing its advanced reasoning capabilities in structured logical analysis, step-by-step problem solving, multi-context understanding, and knowledge accumulation from different subjects. However, there are areas for improvement, such as complex temporal reasoning, handling deep ambiguity, and generating creative solutions. Most importantly, it demonstrates how a model like DeepSeek-R1 can be developed without the burden of huge training costs of GPUs.
Its open-sourced model pushes AI toward more democratic realms. New research will soon be conducted on this training method, leading to more potent and powerful AI models with even better reasoning capabilities. While AGI may still be in the distant future, DeepSeek-R1’s advancements point toward a future where AGI will emerge hand in hand with people. DeepSeek-R1 is undoubtedly a key step forward in realizing more advanced AI reasoning systems.
Key Takeaways
- DeepSeek R1’s Advanced Reasoning Capabilities shine through its ability to perform structured logical analysis, solve problems step-by-step, and understand complex contexts across different domains.
- The model pushes the boundaries of reasoning by accumulating knowledge from diverse subjects, demonstrating an impressive multi-contextual understanding that sets it apart from other generative LLMs.
- Despite its strengths, DeepSeek R1’s Advanced Reasoning Capabilities still face challenges in areas such as complex temporal reasoning and handling ambiguity, which opens the door for future improvements.
- By making the model open-source, DeepSeek R1 not only advances reasoning but also makes cutting-edge AI more accessible, offering a more democratic approach to AI development.
- DeepSeek R1’s Advanced Reasoning Capabilities pave the way for future breakthroughs in AI models, with the potential for AGI to emerge through continuous research and innovation.
Frequently Asked Questions
Q1. How does DeepSeek-R1-7B compare to large models in reasoning tasks?A. While it may not match the power of larger 32B or 70B models, it shows comparable performance in structure reasoning tasks, particularly in mathematical and logical analysis.
Q2. What are the best practices for prompt design when testing reasoning?A. Write step-by-step requirements, focus on clear instructions, and explicit evaluation criteria. Multipart questions often yield better insight than single questions.
Q3. How reliable are these evaluation methods?A. We are human, we must use our brains to evaluate the response. It should be used as part of a broader evaluation strategy that includes quantitative metrics and real-world testing. Following this principle will help better evaluation.
Human->Prompt->AI->Response-> Human -> Actual Response
The media shown in this article is not owned by Analytics Vidhya and is used at the Author’s discretion.
The above is the detailed content of Decoding DeepSeek R1's Advanced Reasoning Capabilities. For more information, please follow other related articles on the PHP Chinese website!
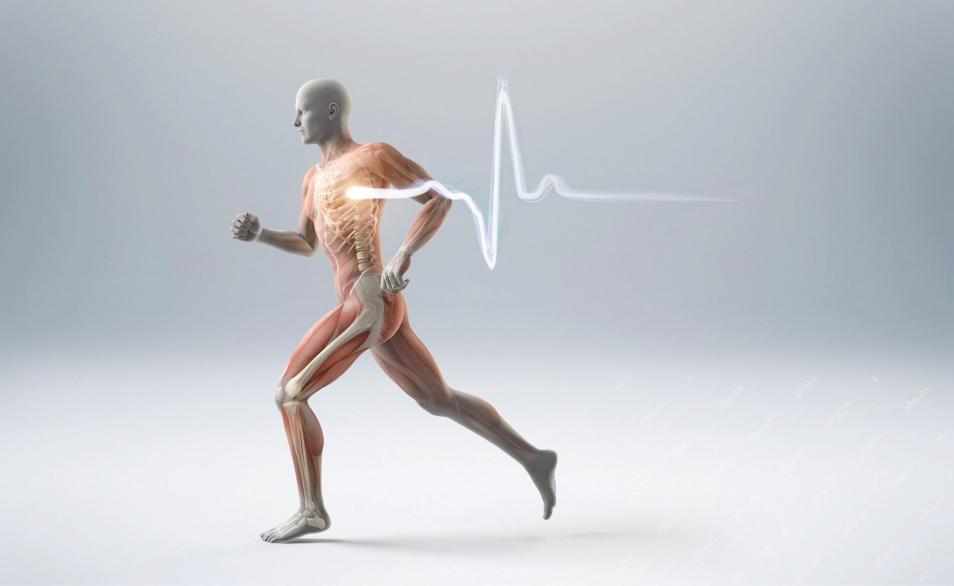
Scientists have extensively studied human and simpler neural networks (like those in C. elegans) to understand their functionality. However, a crucial question arises: how do we adapt our own neural networks to work effectively alongside novel AI s
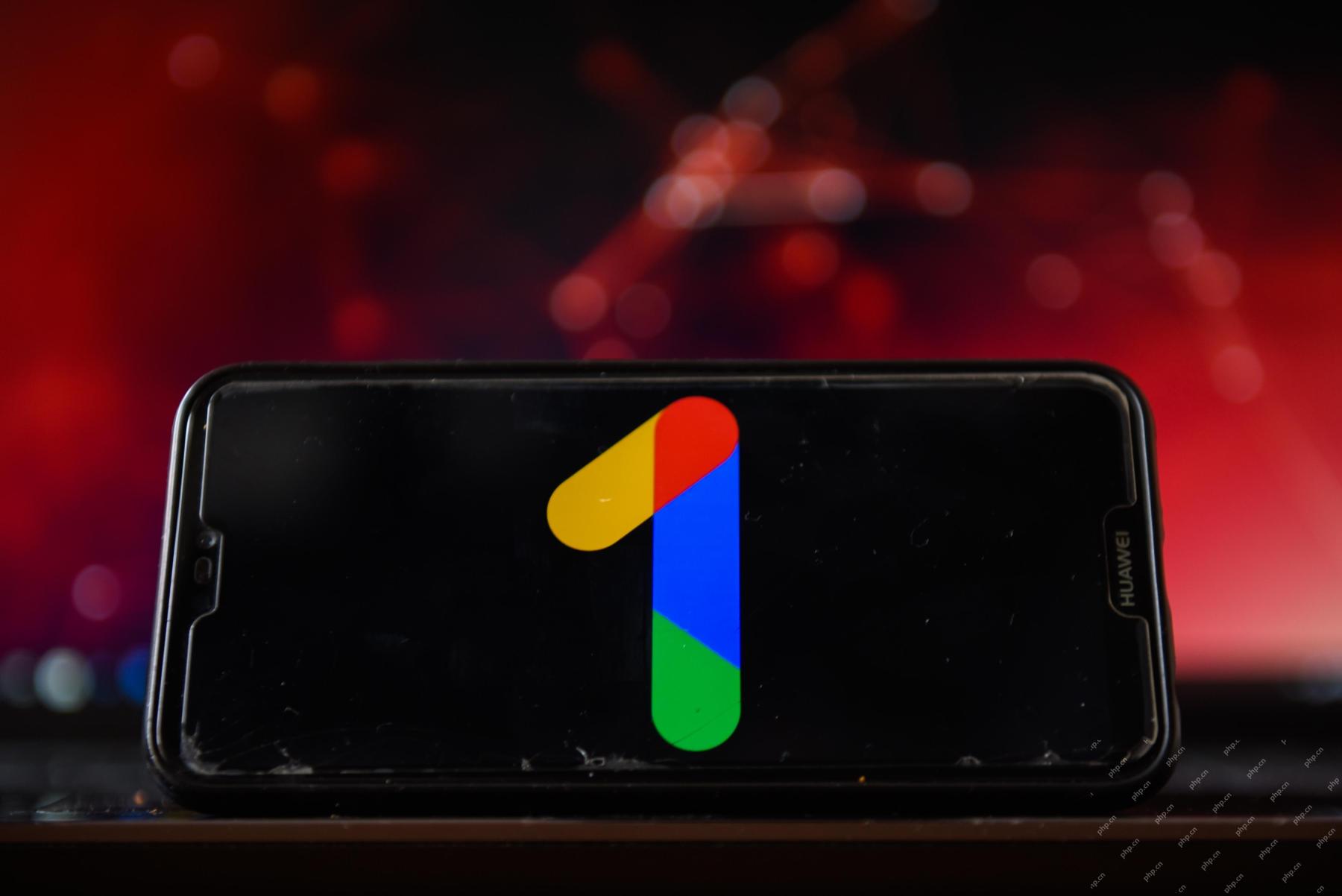
Google's Gemini Advanced: New Subscription Tiers on the Horizon Currently, accessing Gemini Advanced requires a $19.99/month Google One AI Premium plan. However, an Android Authority report hints at upcoming changes. Code within the latest Google P
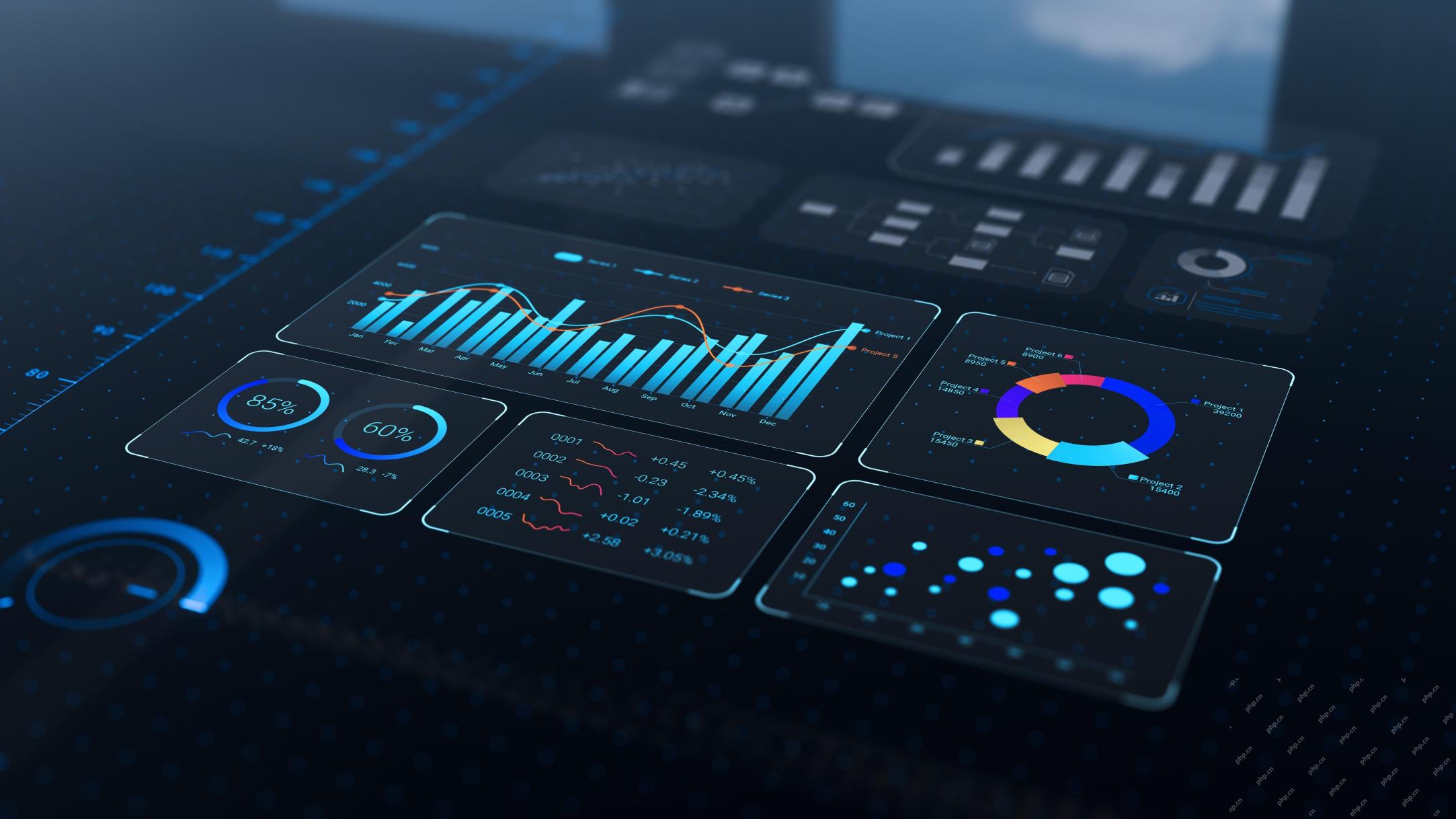
Despite the hype surrounding advanced AI capabilities, a significant challenge lurks within enterprise AI deployments: data processing bottlenecks. While CEOs celebrate AI advancements, engineers grapple with slow query times, overloaded pipelines, a

Handling documents is no longer just about opening files in your AI projects, it’s about transforming chaos into clarity. Docs such as PDFs, PowerPoints, and Word flood our workflows in every shape and size. Retrieving structured
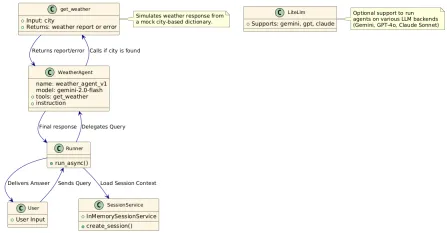
Harness the power of Google's Agent Development Kit (ADK) to create intelligent agents with real-world capabilities! This tutorial guides you through building conversational agents using ADK, supporting various language models like Gemini and GPT. W
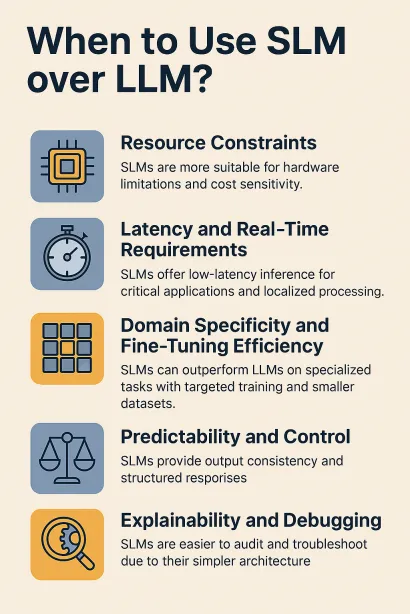
summary: Small Language Model (SLM) is designed for efficiency. They are better than the Large Language Model (LLM) in resource-deficient, real-time and privacy-sensitive environments. Best for focus-based tasks, especially where domain specificity, controllability, and interpretability are more important than general knowledge or creativity. SLMs are not a replacement for LLMs, but they are ideal when precision, speed and cost-effectiveness are critical. Technology helps us achieve more with fewer resources. It has always been a promoter, not a driver. From the steam engine era to the Internet bubble era, the power of technology lies in the extent to which it helps us solve problems. Artificial intelligence (AI) and more recently generative AI are no exception
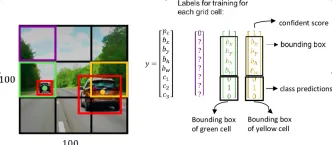
Harness the Power of Google Gemini for Computer Vision: A Comprehensive Guide Google Gemini, a leading AI chatbot, extends its capabilities beyond conversation to encompass powerful computer vision functionalities. This guide details how to utilize
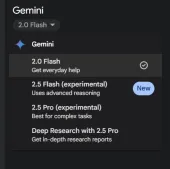
The AI landscape of 2025 is electrifying with the arrival of Google's Gemini 2.0 Flash and OpenAI's o4-mini. These cutting-edge models, launched weeks apart, boast comparable advanced features and impressive benchmark scores. This in-depth compariso


Hot AI Tools

Undresser.AI Undress
AI-powered app for creating realistic nude photos

AI Clothes Remover
Online AI tool for removing clothes from photos.

Undress AI Tool
Undress images for free

Clothoff.io
AI clothes remover

Video Face Swap
Swap faces in any video effortlessly with our completely free AI face swap tool!

Hot Article

Hot Tools

Notepad++7.3.1
Easy-to-use and free code editor

Safe Exam Browser
Safe Exam Browser is a secure browser environment for taking online exams securely. This software turns any computer into a secure workstation. It controls access to any utility and prevents students from using unauthorized resources.
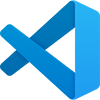
VSCode Windows 64-bit Download
A free and powerful IDE editor launched by Microsoft
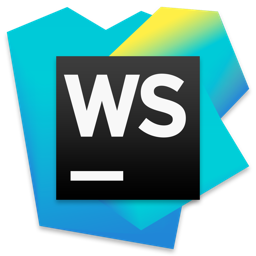
WebStorm Mac version
Useful JavaScript development tools

PhpStorm Mac version
The latest (2018.2.1) professional PHP integrated development tool
