Databricks Unveils DBRX: A High-Performance, Open-Source Large Language Model
Databricks has launched DBRX, a groundbreaking open-source large language model (LLM) built on a sophisticated mixture-of-experts (MoE) architecture. Unlike traditional LLMs that rely on a single neural network, DBRX employs multiple specialized "expert" networks, each optimized for specific tasks and data types. This innovative approach leads to superior performance and efficiency compared to models like GPT-3.5 and Llama 2. DBRX boasts a 73.7% score in language understanding benchmarks, surpassing Llama 2's 69.8%. This article delves into DBRX's capabilities, architecture, and usage.
Understanding Databricks DBRX
DBRX leverages a transformer-based decoder-only architecture, trained using next-token prediction. Its core innovation lies in its fine-grained MoE architecture. These "experts" are specialized LLM agents, enhanced with domain-specific knowledge and advanced reasoning capabilities. DBRX utilizes 16 smaller experts, selecting a subset of 4 for each input. This fine-grained approach, with 65 times more expert combinations than models like Mixtral and Grok-1, significantly improves model quality.
Key features of DBRX include:
- Parameter Size: A total of 132 billion parameters, with 36 billion active for any given input.
- Training Data: Pre-trained on a massive 12 trillion tokens of meticulously curated data, offering at least double the token-for-token effectiveness of datasets used for MPT models. A context length of 32,000 tokens is supported.
DBRX Training Methodology
DBRX's training involved a carefully designed curriculum and strategic data mix adjustments to optimize performance across diverse inputs. The process leveraged Databricks' powerful tools, including Apache Spark, Databricks notebooks, and Unity Catalog. Key technologies employed during pre-training include Rotary Position Encodings (RoPE), Gated Linear Units (GLU), Grouped Query Attention (GQA), and the GPT-4 tokenizer from the tiktoken repository.
Benchmarking DBRX Against Competitors
Databricks highlights DBRX's superior efficiency and performance compared to leading open-source LLMs:
Model Comparison | General Knowledge | Commonsense Reasoning | Databricks Gauntlet | Programming Reasoning | Mathematical Reasoning |
---|---|---|---|---|---|
DBRX vs LLaMA2-70B | 9.8% | 3.1% | 14% | 37.9% | 40.2% |
DBRX vs Mixtral Instruct | 2.3% | 1.4% | 6.1% | 15.3% | 5.8% |
DBRX vs Grok-1 | 0.7% | N/A | N/A | 6.9% | 4% |
DBRX vs Mixtral Base | 1.8% | 2.5% | 10% | 29.9% | N/A |
(A graph visualizing some of these results would be included here. Image URL: [])
Utilizing DBRX: A Practical Guide
Before using DBRX, ensure your system has at least 320GB of RAM. Follow these steps:
-
Installation: Install the
transformers
library:pip install "transformers>=4.40.0"
- Access Token: Obtain a Hugging Face access token with read permissions.
-
Model Loading: Use the following code (replace
hf_YOUR_TOKEN
with your token):
from transformers import AutoTokenizer, AutoModelForCausalLM import torch tokenizer = AutoTokenizer.from_pretrained("databricks/dbrx-base", token="hf_YOUR_TOKEN") model = AutoModelForCausalLM.from_pretrained("databricks/dbrx-base", device_map="auto", torch_dtype=torch.bfloat16, token="hf_YOUR_TOKEN") input_text = "Databricks was founded in " input_ids = tokenizer(input_text, return_tensors="pt").to("cuda") outputs = model.generate(**input_ids, max_new_tokens=100) print(tokenizer.decode(outputs[0]))
DBRX excels in various tasks, including text completion, language understanding, query optimization, code generation, explanation, debugging, and vulnerability identification.
(An image showcasing DBRX responding to a simple command would be included here. Image URL: [])
Fine-tuning DBRX
Fine-tuning DBRX is possible using Github's open-source LLM foundry. Training examples should be formatted as dictionaries: {'prompt': <prompt_text>, 'response': <response_text>}</response_text></prompt_text>
. The foundry supports fine-tuning with datasets from the Hugging Face Hub, local datasets, and StreamingDataset (.mds) format. Detailed instructions for each method are available in the original article. (Further details on the YAML configuration files for fine-tuning are omitted for brevity).
Conclusion
Databricks DBRX represents a significant advancement in LLM technology, leveraging its innovative MoE architecture for enhanced speed, cost-effectiveness, and performance. Its open-source nature fosters further development and community contributions.
The above is the detailed content of Databricks DBRX Tutorial: A Step-by-Step Guide. For more information, please follow other related articles on the PHP Chinese website!
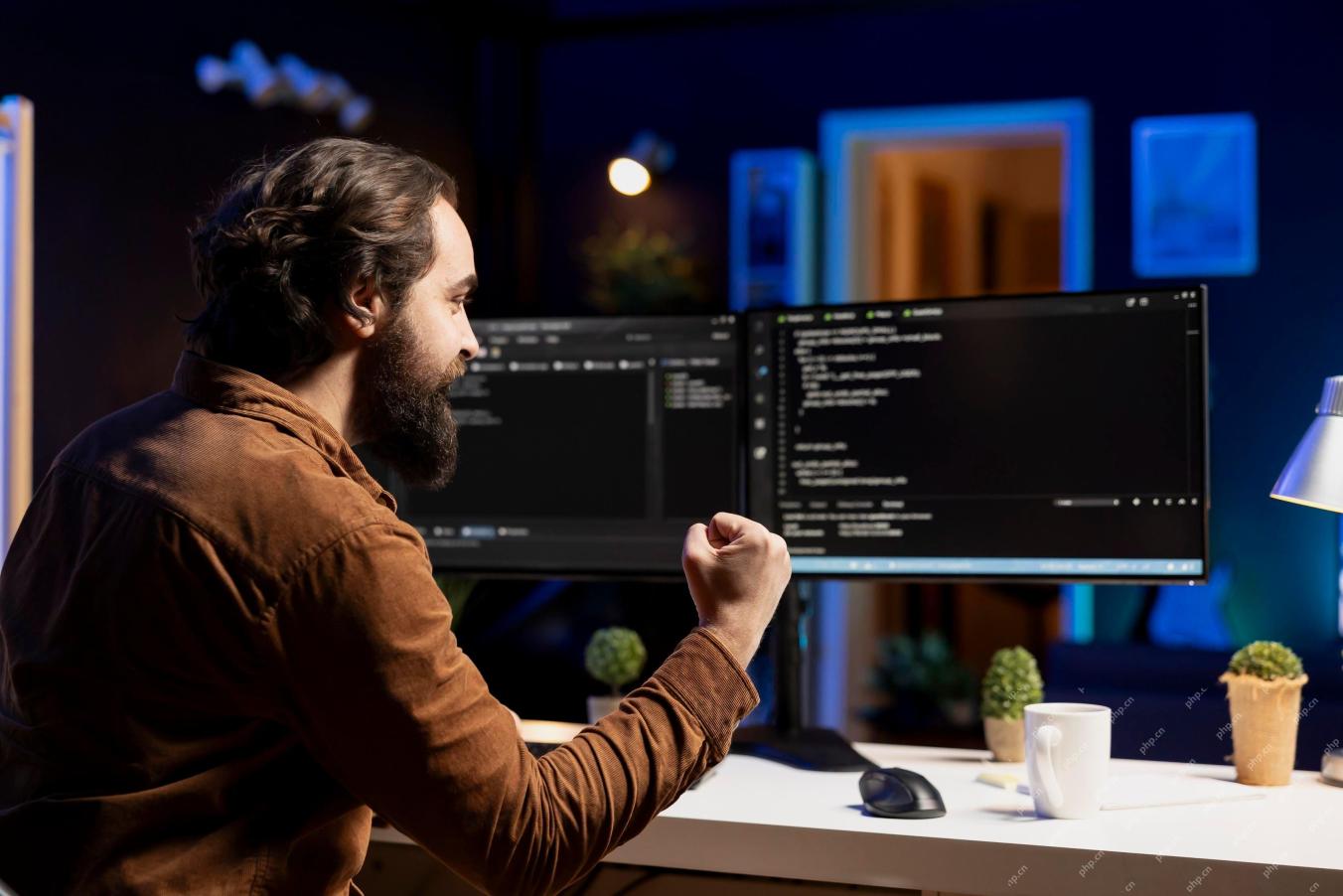
Let's discuss the rising use of "vibes" as an evaluation metric in the AI field. This analysis is part of my ongoing Forbes column on AI advancements, exploring complex aspects of AI development (see link here). Vibes in AI Assessment Tradi
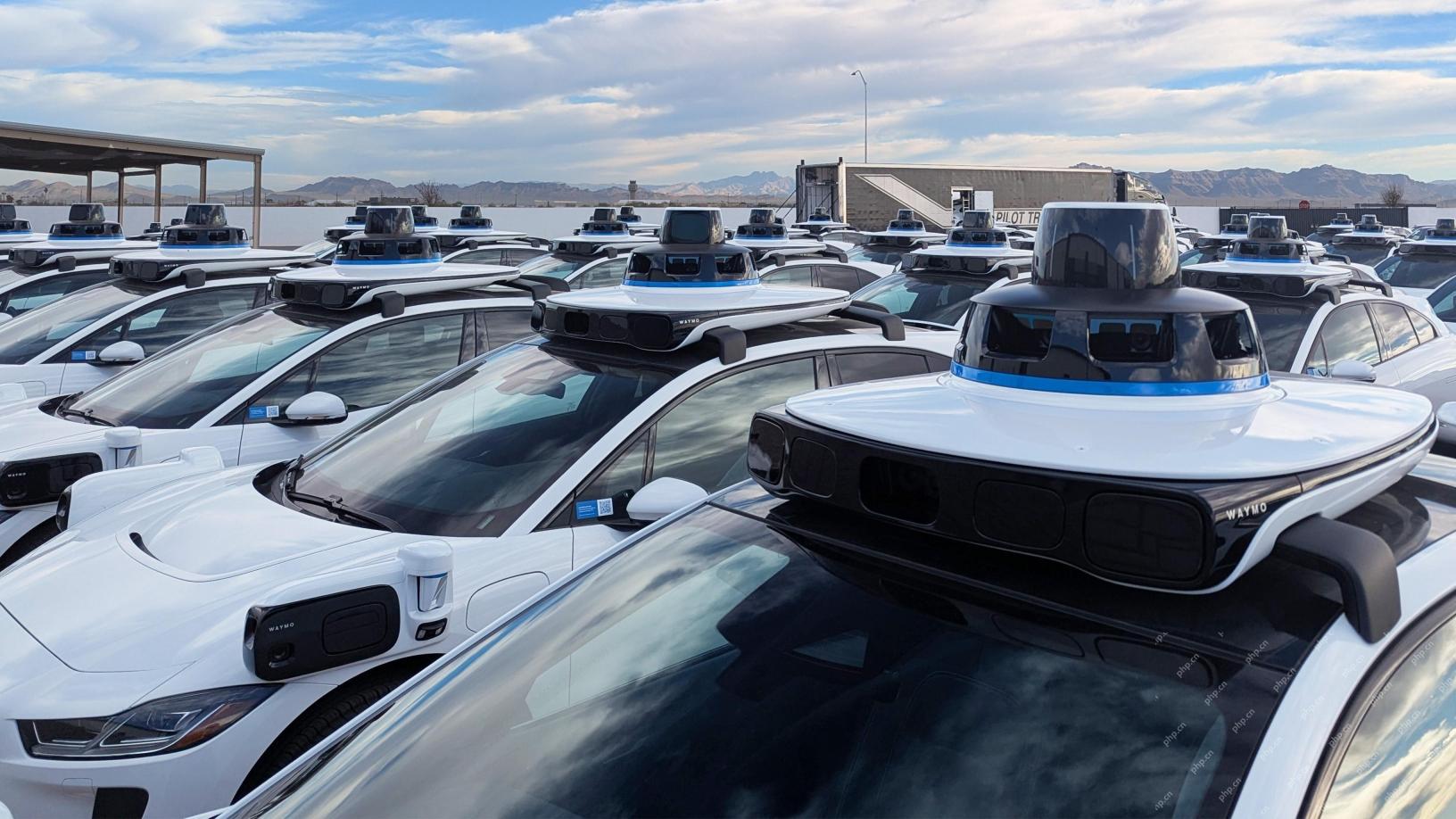
Waymo's Arizona Factory: Mass-Producing Self-Driving Jaguars and Beyond Located near Phoenix, Arizona, Waymo operates a state-of-the-art facility producing its fleet of autonomous Jaguar I-PACE electric SUVs. This 239,000-square-foot factory, opened
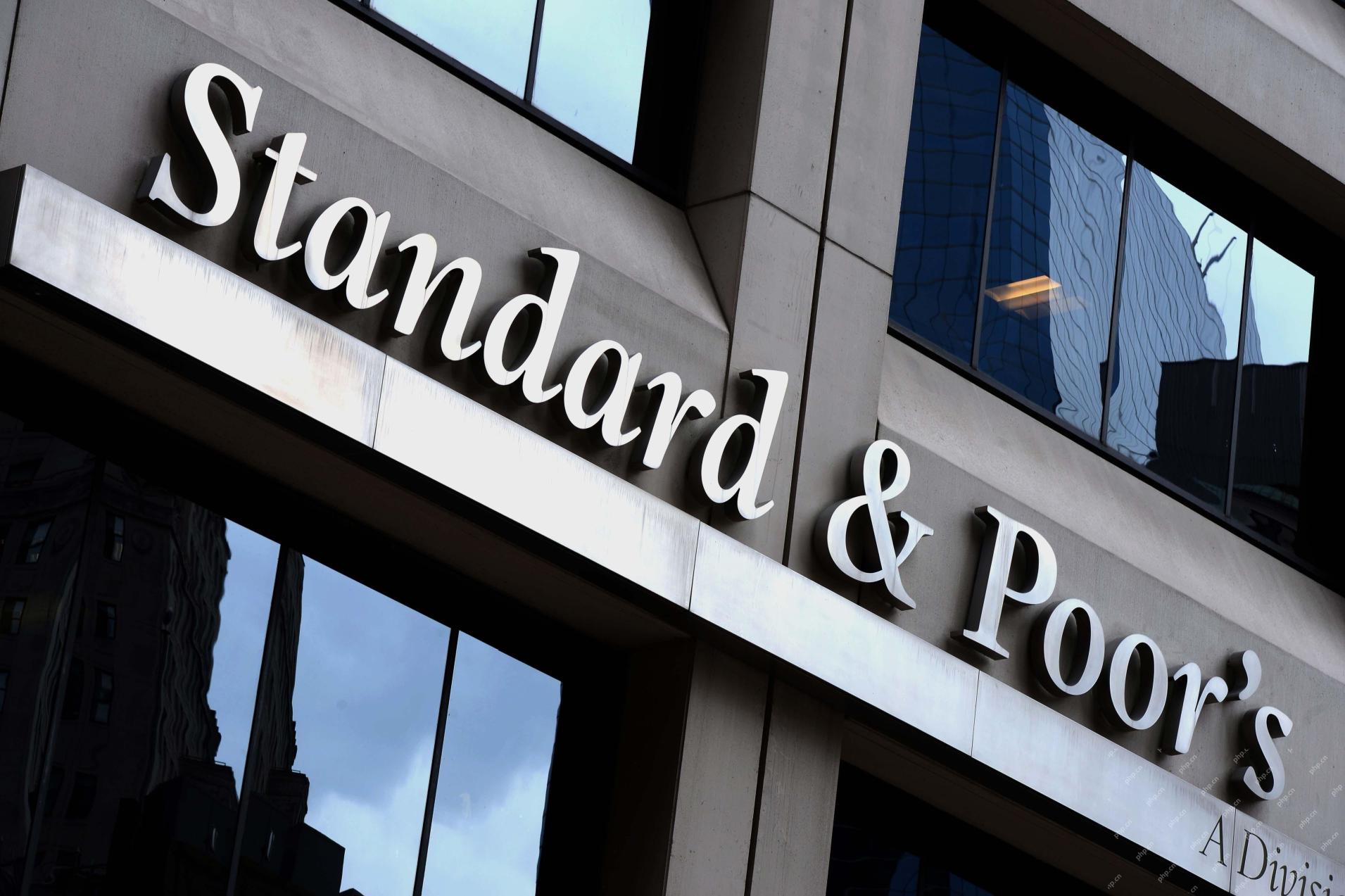
S&P Global's Chief Digital Solutions Officer, Jigar Kocherlakota, discusses the company's AI journey, strategic acquisitions, and future-focused digital transformation. A Transformative Leadership Role and a Future-Ready Team Kocherlakota's role
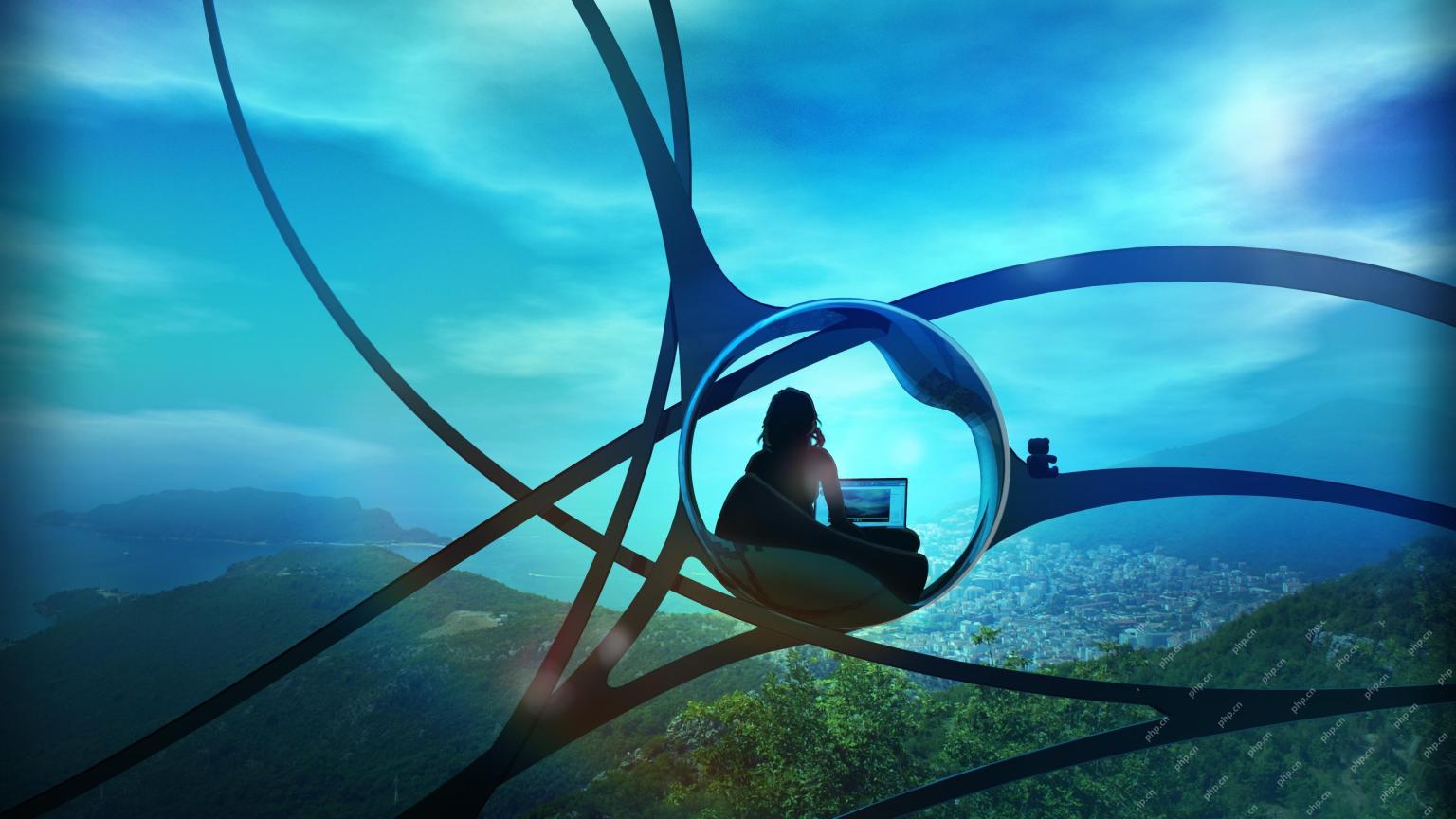
From Apps to Ecosystems: Navigating the Digital Landscape The digital revolution extends far beyond social media and AI. We're witnessing the rise of "everything apps"—comprehensive digital ecosystems integrating all aspects of life. Sam A
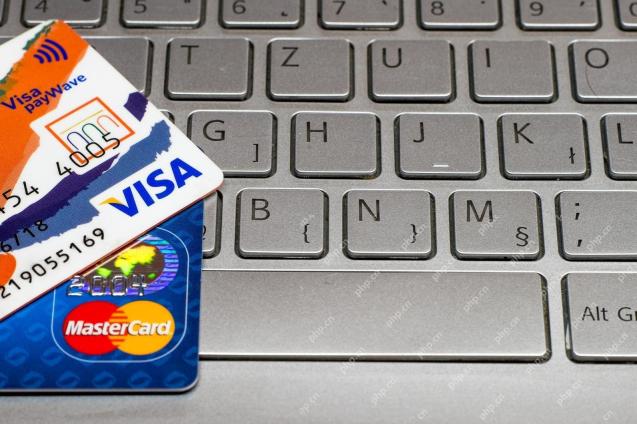
Mastercard's Agent Pay: AI-Powered Payments Revolutionize Commerce While Visa's AI-powered transaction capabilities made headlines, Mastercard has unveiled Agent Pay, a more advanced AI-native payment system built on tokenization, trust, and agentic
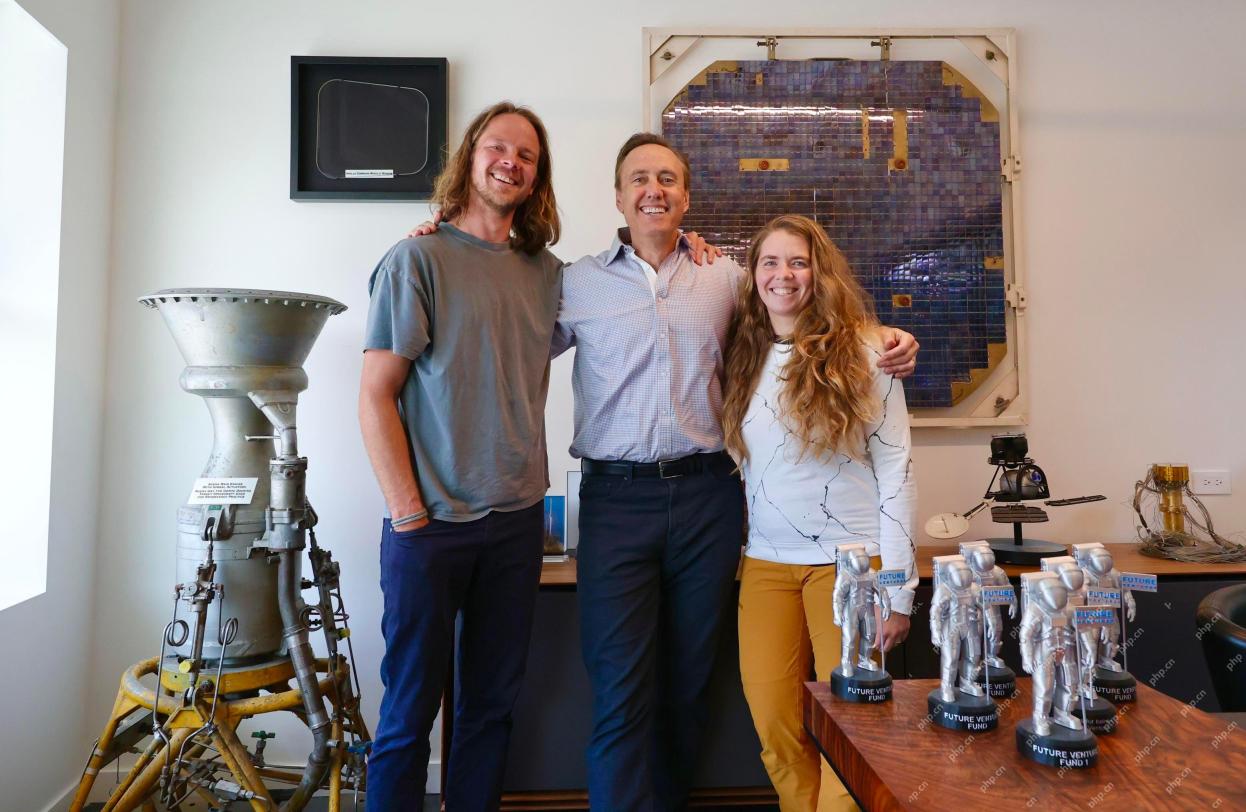
Future Ventures Fund IV: A $200M Bet on Novel Technologies Future Ventures recently closed its oversubscribed Fund IV, totaling $200 million. This new fund, managed by Steve Jurvetson, Maryanna Saenko, and Nico Enriquez, represents a significant inv
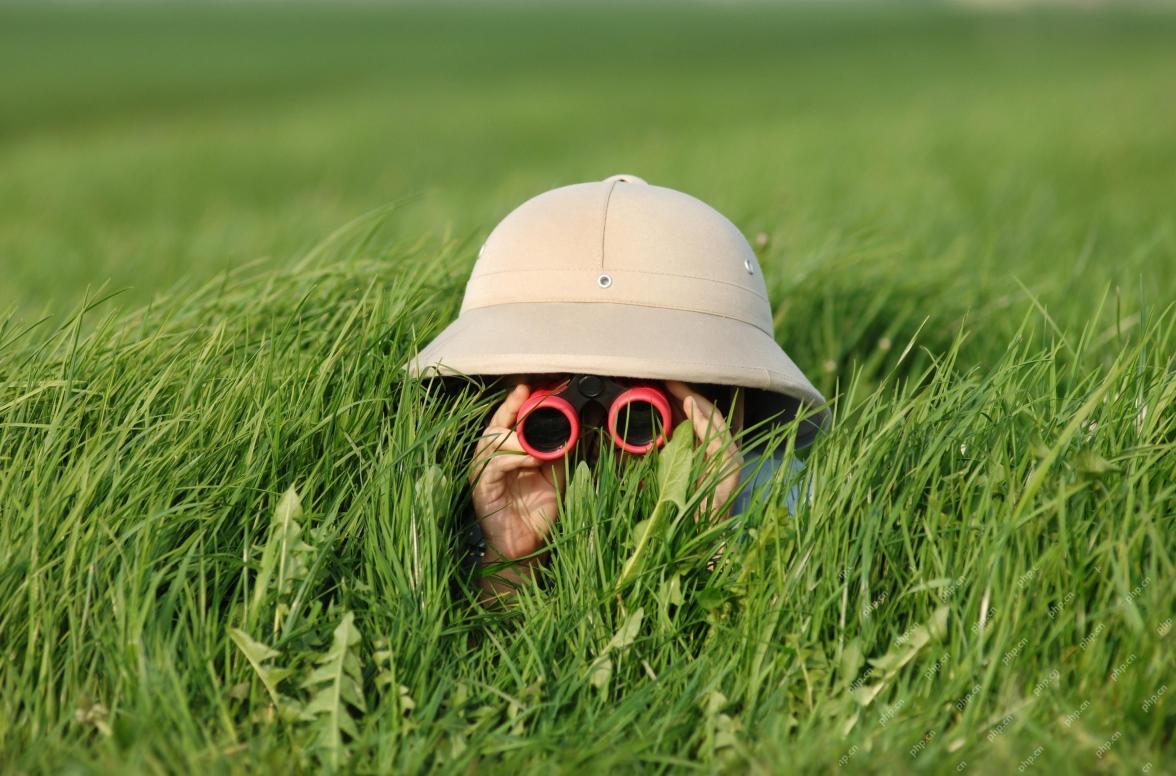
With the explosion of AI applications, enterprises are shifting from traditional search engine optimization (SEO) to generative engine optimization (GEO). Google is leading the shift. Its "AI Overview" feature has served over a billion users, providing full answers before users click on the link. [^2] Other participants are also rapidly rising. ChatGPT, Microsoft Copilot and Perplexity are creating a new “answer engine” category that completely bypasses traditional search results. If your business doesn't show up in these AI-generated answers, potential customers may never find you—even if you rank high in traditional search results. From SEO to GEO – What exactly does this mean? For decades
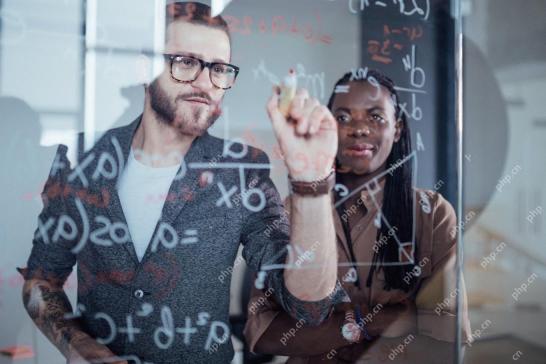
Let's explore the potential paths to Artificial General Intelligence (AGI). This analysis is part of my ongoing Forbes column on AI advancements, delving into the complexities of achieving AGI and Artificial Superintelligence (ASI). (See related art


Hot AI Tools

Undresser.AI Undress
AI-powered app for creating realistic nude photos

AI Clothes Remover
Online AI tool for removing clothes from photos.

Undress AI Tool
Undress images for free

Clothoff.io
AI clothes remover

Video Face Swap
Swap faces in any video effortlessly with our completely free AI face swap tool!

Hot Article

Hot Tools
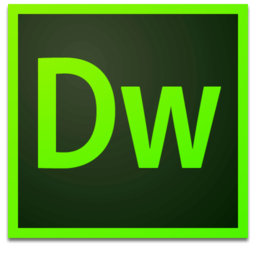
Dreamweaver Mac version
Visual web development tools
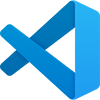
VSCode Windows 64-bit Download
A free and powerful IDE editor launched by Microsoft

SublimeText3 Chinese version
Chinese version, very easy to use

MinGW - Minimalist GNU for Windows
This project is in the process of being migrated to osdn.net/projects/mingw, you can continue to follow us there. MinGW: A native Windows port of the GNU Compiler Collection (GCC), freely distributable import libraries and header files for building native Windows applications; includes extensions to the MSVC runtime to support C99 functionality. All MinGW software can run on 64-bit Windows platforms.

SecLists
SecLists is the ultimate security tester's companion. It is a collection of various types of lists that are frequently used during security assessments, all in one place. SecLists helps make security testing more efficient and productive by conveniently providing all the lists a security tester might need. List types include usernames, passwords, URLs, fuzzing payloads, sensitive data patterns, web shells, and more. The tester can simply pull this repository onto a new test machine and he will have access to every type of list he needs.
