Harnessing Hugging Face for Image Classification: A Comprehensive Guide
Image classification, a cornerstone of AI and machine learning, finds applications across diverse fields, from facial recognition to medical imaging. Hugging Face emerges as a powerful platform for this task, particularly for those familiar with natural language processing (NLP) and increasingly, computer vision. This guide details using Hugging Face for image classification, catering to both beginners and experienced practitioners.
Understanding Image Classification and Hugging Face's Advantages
Image classification involves categorizing images into predefined classes using algorithms that analyze visual content and predict categories based on learned patterns. Convolutional Neural Networks (CNNs) are the standard approach due to their pattern-recognition capabilities. For a deeper dive into CNNs, refer to our article "An Introduction to Convolutional Neural Networks (CNNs)." Our "Classification in Machine Learning: An Introduction" article provides a broader understanding of classification algorithms.
Hugging Face offers several advantages:
Key benefits of using Hugging Face for image classification
- Accessibility: Intuitive APIs and comprehensive documentation cater to all skill levels.
- Pre-trained Models: A vast repository of pre-trained models allows for efficient fine-tuning on custom datasets, minimizing training time and computational resources. Users can train and deploy their own models.
- Community & Support: A vibrant community provides invaluable support and troubleshooting assistance.
Hugging Face also simplifies model deployment across major cloud platforms (AWS, Azure, Google Cloud Platform) with various inference options.
Model deployment options across cloud platforms
Data Preparation and Preprocessing
This guide uses the Hugging Face "beans" dataset for demonstration. After loading, we'll visualize the data before preprocessing. The accompanying Google Colab notebook provides the code. The code is inspired by Hugging Face's official documentation.
Library Requirements:
Install necessary libraries using pip:
pip -q install datasets pip -q install transformers=='4.29.0' pip -q install tensorflow=='2.15' pip -q install evaluate pip -q install --upgrade accelerate
Restart the kernel after installation. Import required libraries:
import torch import torchvision import numpy as np import evaluate from datasets import load_dataset from huggingface_hub import notebook_login from torchvision import datasets, transforms from torch.utils.data import DataLoader from transformers import DefaultDataCollator from transformers import AutoImageProcessor from torchvision.transforms import RandomResizedCrop, Compose, Normalize, ToTensor from transformers import AutoModelForImageClassification, TrainingArguments, Trainer import matplotlib.pyplot as plt
Data Loading and Organization:
Load the dataset:
pip -q install datasets pip -q install transformers=='4.29.0' pip -q install tensorflow=='2.15' pip -q install evaluate pip -q install --upgrade accelerate
The dataset contains 1034 images, each with 'image_file_path', 'image' (PIL object), and 'labels' (0: angular_leaf_spot, 1: bean_rust, 2: healthy).
A helper function visualizes random images:
import torch import torchvision import numpy as np import evaluate from datasets import load_dataset from huggingface_hub import notebook_login from torchvision import datasets, transforms from torch.utils.data import DataLoader from transformers import DefaultDataCollator from transformers import AutoImageProcessor from torchvision.transforms import RandomResizedCrop, Compose, Normalize, ToTensor from transformers import AutoModelForImageClassification, TrainingArguments, Trainer import matplotlib.pyplot as plt
Visualize six random images:
beans_train = load_dataset("beans", split="train")
Sample images from the beans dataset
Data Preprocessing:
Split the dataset (80% train, 20% validation):
labels_names = {0: "angular_leaf_spot", 1: "bean_rust", 2: "healthy"} def display_random_images(dataset, num_images=4): # ... (function code as in original input) ...
Create label mappings:
display_random_images(beans_train, num_images=6)
Model Loading and Fine-tuning
Load the pre-trained ViT model:
beans_train = beans_train.train_test_split(test_size=0.2)
The code loads the pre-trained model, defines transformations (resizing, normalization), and prepares the dataset for training. The accuracy metric is defined for evaluation.
Log in to Hugging Face:
labels = beans_train["train"].features["labels"].names label2id, id2label = dict(), dict() for i, label in enumerate(labels): label2id[label] = str(i) id2label[str(i)] = label
(Follow on-screen instructions)
Configure and initiate training:
checkpoint = "google/vit-base-patch16-224-in21k" image_processor = AutoImageProcessor.from_pretrained(checkpoint) # ... (rest of the preprocessing code as in original input) ...
(Training results as shown in the original input)
Model Deployment and Integration
Push the trained model to the Hugging Face Hub:
notebook_login()
The model can then be accessed and used via:
- Hugging Face Portal: Directly upload images for prediction.
- Transformers Library: Use the model within your Python code.
- REST API: Utilize the provided API endpoint for predictions. Example using the API:
training_args = TrainingArguments( # ... (training arguments as in original input) ... ) trainer = Trainer( # ... (trainer configuration as in original input) ... ) trainer.train()
Conclusion and Further Resources
This guide provides a comprehensive walkthrough of image classification using Hugging Face. Further learning resources include:
- "An Introduction to Using Transformers and Hugging Face"
- "Image Processing with Python" skill track
- "What is Image Recognition?" article
This guide empowers users of all levels to leverage Hugging Face for their image classification projects.
The above is the detailed content of Hugging Face Image Classification: A Comprehensive Guide With Examples. For more information, please follow other related articles on the PHP Chinese website!
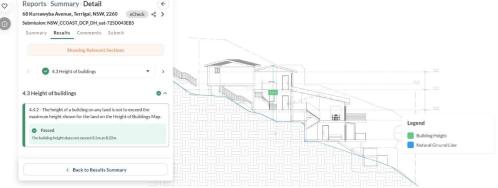
AI Streamlines Wildfire Recovery Permitting Australian tech firm Archistar's AI software, utilizing machine learning and computer vision, automates the assessment of building plans for compliance with local regulations. This pre-validation significan
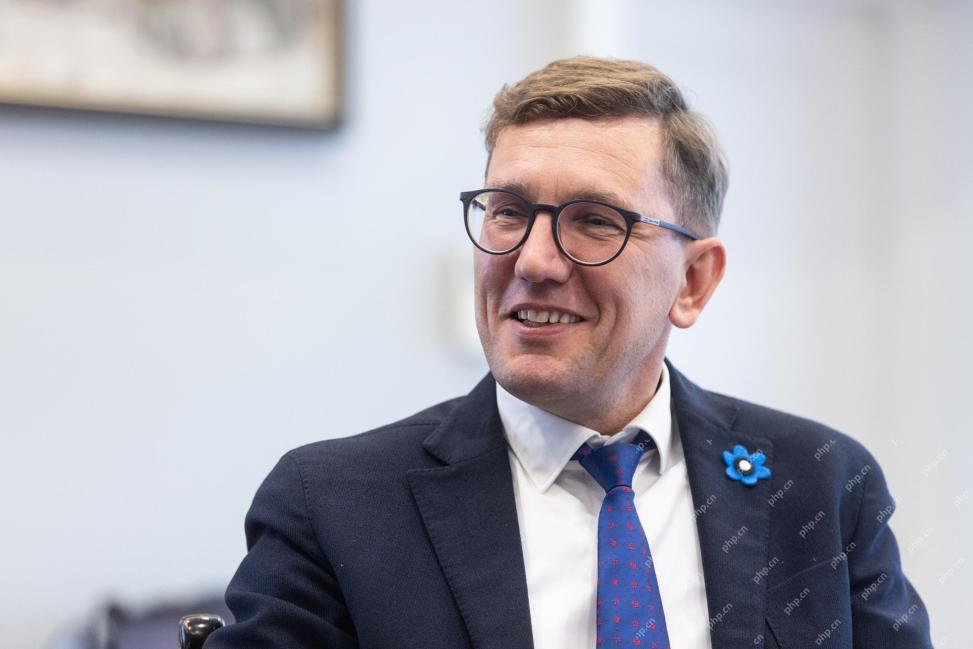
Estonia's Digital Government: A Model for the US? The US struggles with bureaucratic inefficiencies, but Estonia offers a compelling alternative. This small nation boasts a nearly 100% digitized, citizen-centric government powered by AI. This isn't
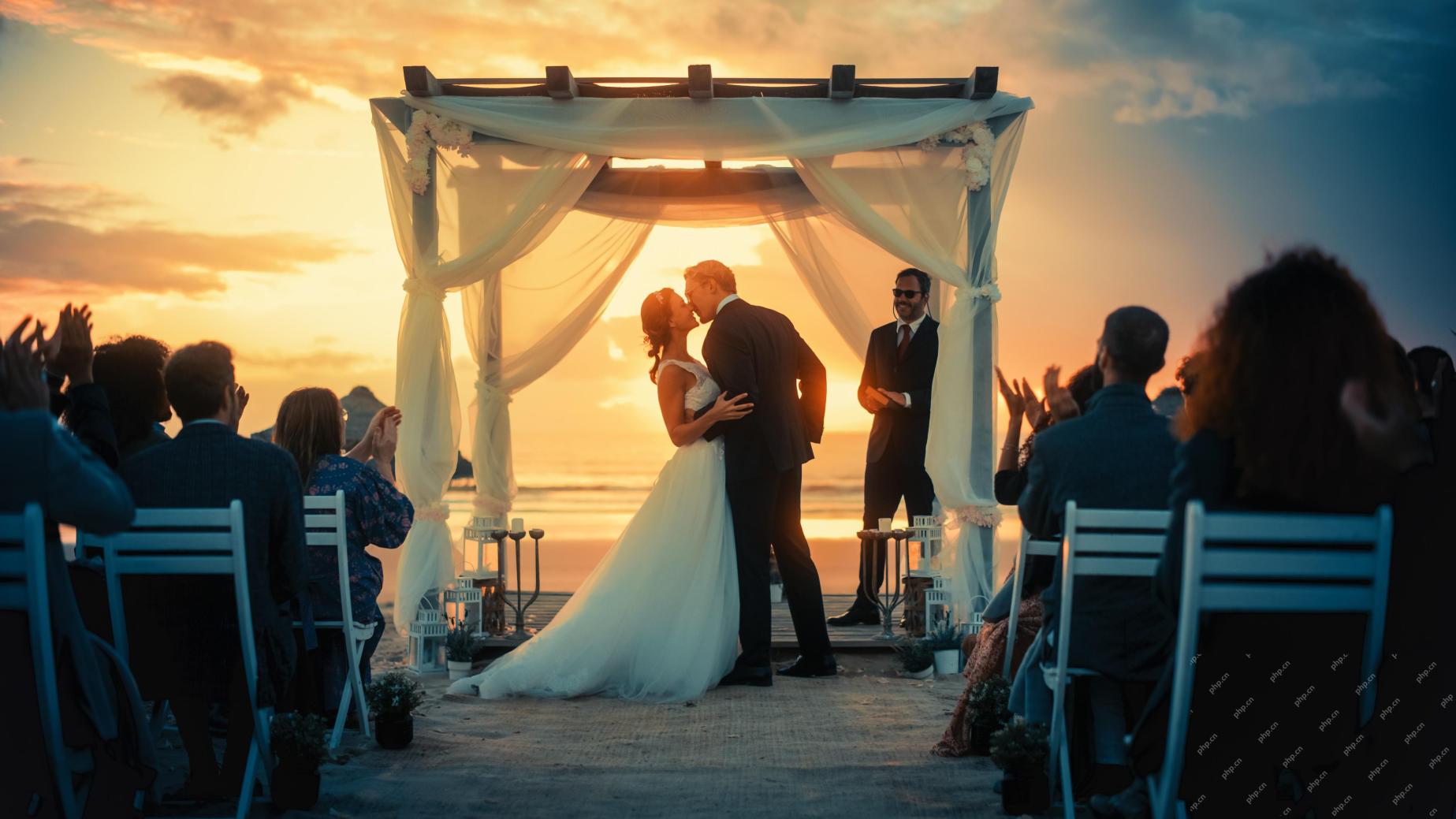
Planning a wedding is a monumental task, often overwhelming even the most organized couples. This article, part of an ongoing Forbes series on AI's impact (see link here), explores how generative AI can revolutionize wedding planning. The Wedding Pl
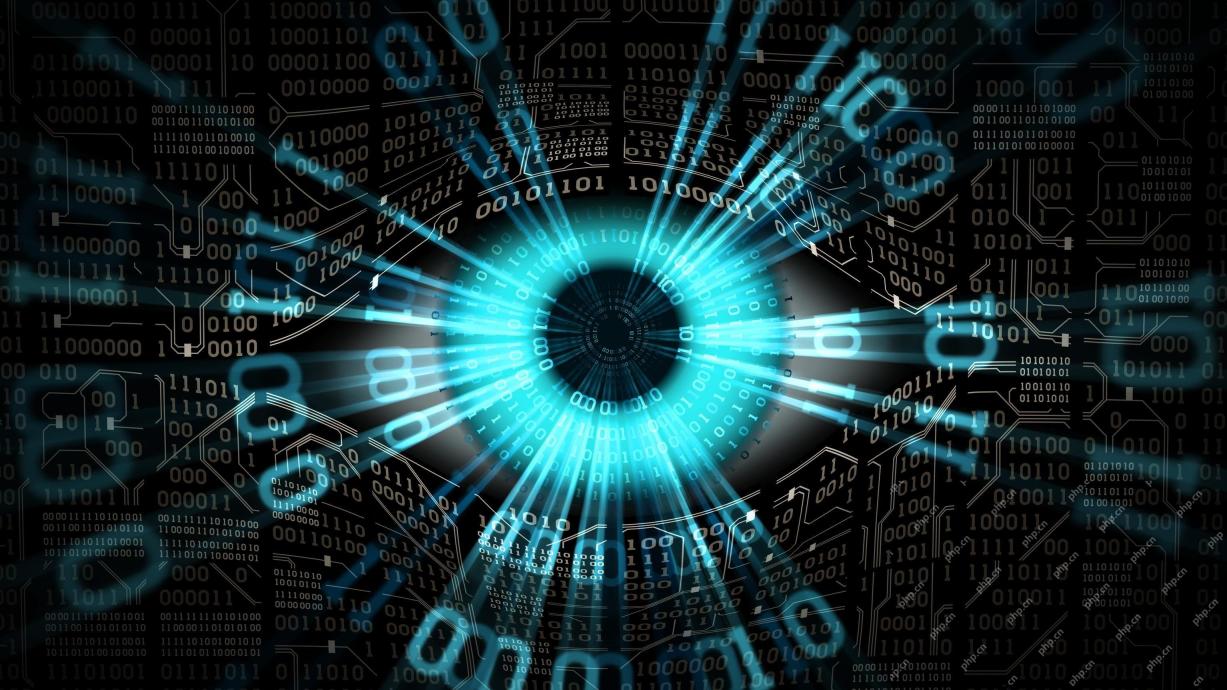
Businesses increasingly leverage AI agents for sales, while governments utilize them for various established tasks. However, consumer advocates highlight the need for individuals to possess their own AI agents as a defense against the often-targeted
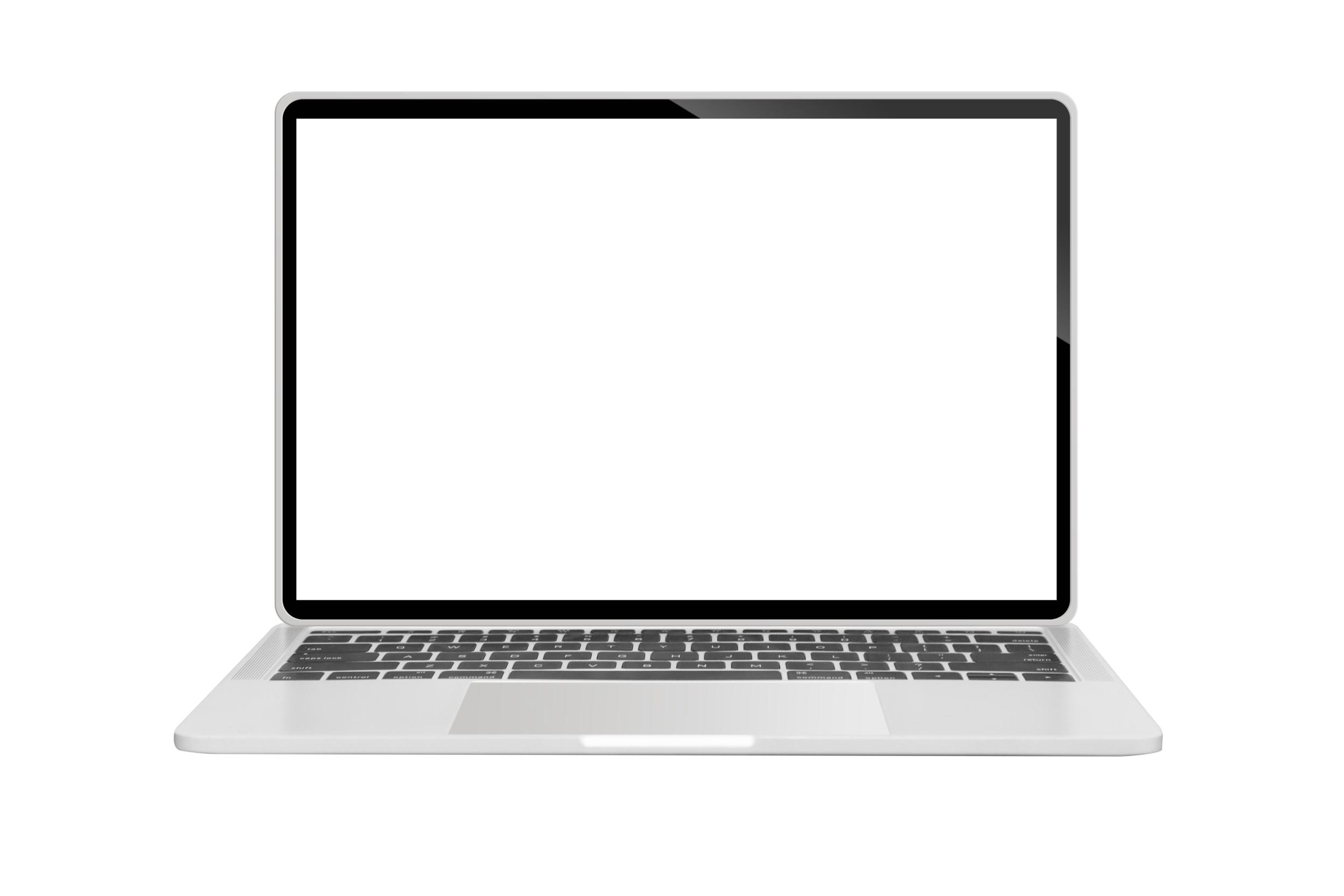
Google is leading this shift. Its "AI Overviews" feature already serves more than one billion users, providing complete answers before anyone clicks a link.[^2] Other players are also gaining ground fast. ChatGPT, Microsoft Copilot, and Pe
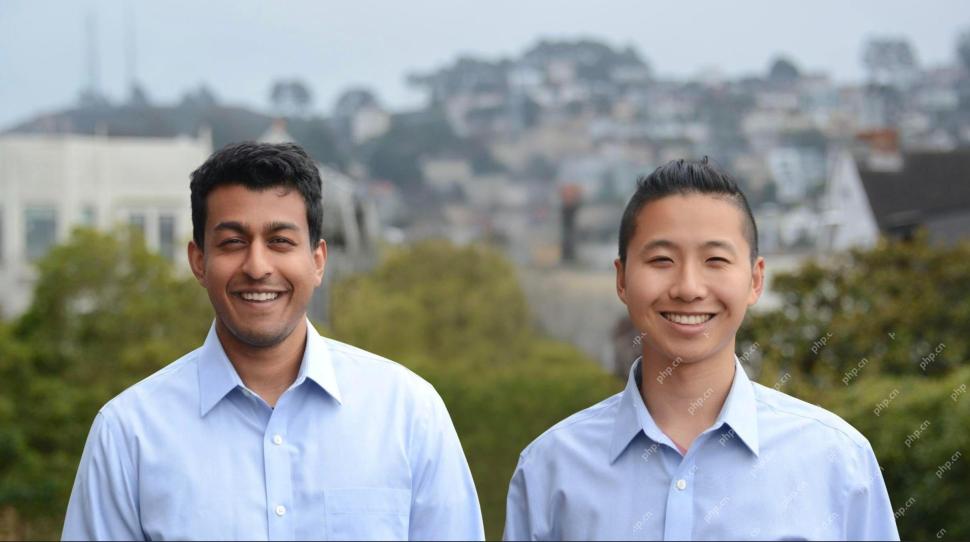
In 2022, he founded social engineering defense startup Doppel to do just that. And as cybercriminals harness ever more advanced AI models to turbocharge their attacks, Doppel’s AI systems have helped businesses combat them at scale— more quickly and
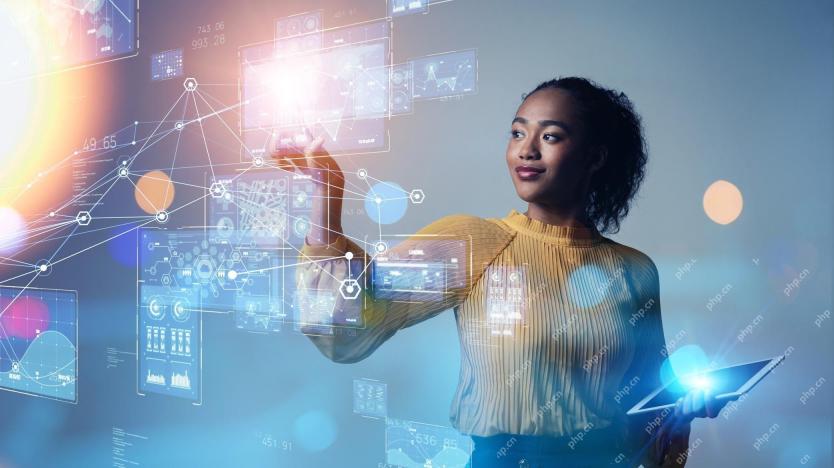
Voila, via interacting with suitable world models, generative AI and LLMs can be substantively boosted. Let’s talk about it. This analysis of an innovative AI breakthrough is part of my ongoing Forbes column coverage on the latest in AI, including
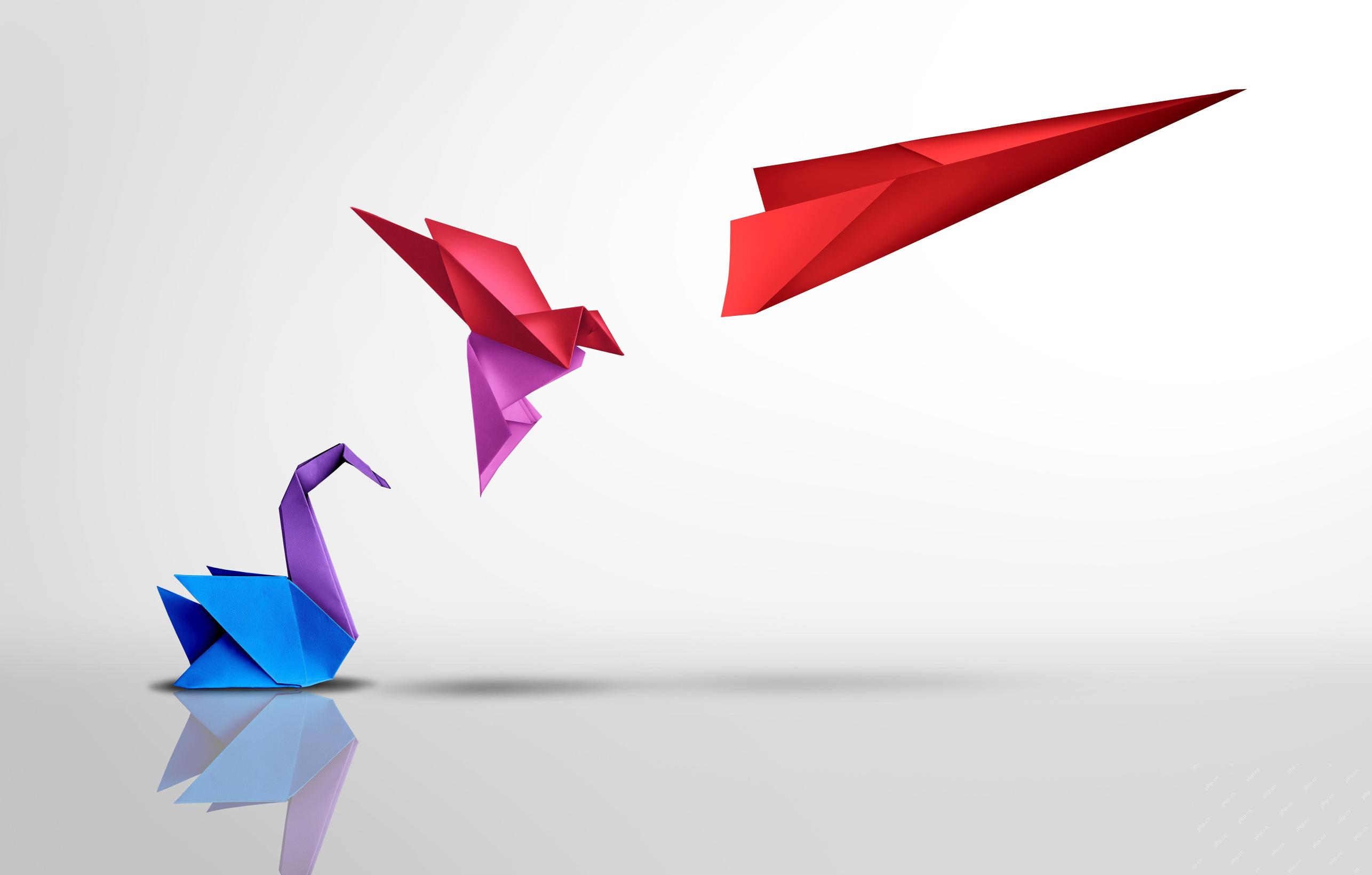
Labor Day 2050. Parks across the nation fill with families enjoying traditional barbecues while nostalgic parades wind through city streets. Yet the celebration now carries a museum-like quality — historical reenactment rather than commemoration of c


Hot AI Tools

Undresser.AI Undress
AI-powered app for creating realistic nude photos

AI Clothes Remover
Online AI tool for removing clothes from photos.

Undress AI Tool
Undress images for free

Clothoff.io
AI clothes remover

Video Face Swap
Swap faces in any video effortlessly with our completely free AI face swap tool!

Hot Article

Hot Tools

SecLists
SecLists is the ultimate security tester's companion. It is a collection of various types of lists that are frequently used during security assessments, all in one place. SecLists helps make security testing more efficient and productive by conveniently providing all the lists a security tester might need. List types include usernames, passwords, URLs, fuzzing payloads, sensitive data patterns, web shells, and more. The tester can simply pull this repository onto a new test machine and he will have access to every type of list he needs.

Safe Exam Browser
Safe Exam Browser is a secure browser environment for taking online exams securely. This software turns any computer into a secure workstation. It controls access to any utility and prevents students from using unauthorized resources.

Atom editor mac version download
The most popular open source editor
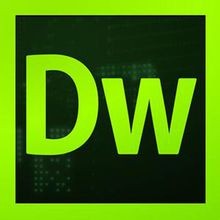
Dreamweaver CS6
Visual web development tools

MantisBT
Mantis is an easy-to-deploy web-based defect tracking tool designed to aid in product defect tracking. It requires PHP, MySQL and a web server. Check out our demo and hosting services.
