ByteDance's groundbreaking OmniHuman-1 framework revolutionizes human animation! This new model, detailed in a recent research paper, leverages a Diffusion Transformer architecture to generate incredibly realistic human videos from a single image and audio input. Forget complex setups – OmniHuman simplifies the process and delivers superior results. Let's dive into the details.
Table of Contents
- Limitations of Existing Animation Models
- The OmniHuman-1 Solution: A Multi-Modal Approach
- Sample OmniHuman-1 Videos
- Model Training and Architecture
- The Omni-Conditions Training Strategy
- Experimental Validation and Performance
- Ablation Study: Optimizing the Training Process
- Extended Visual Results: Demonstrating Versatility
- Conclusion
Limitations of Existing Human Animation Models
Current human animation models often suffer from limitations. They frequently rely on small, specialized datasets, resulting in low-quality, inflexible animations. Many struggle with generalization across diverse contexts, lacking realism and fluidity. The reliance on single input modalities (e.g., only text or image) severely restricts their ability to capture the nuances of human movement and expression.
The OmniHuman-1 Solution
OmniHuman-1 tackles these challenges head-on with a multi-modal approach. It integrates text, audio, and pose information as conditioning signals, creating contextually rich and realistic animations. The innovative Omni-Conditions design preserves subject identity and background details from the reference image, ensuring consistency. A unique training strategy maximizes data utilization, preventing overfitting and boosting performance.
Sample OmniHuman-1 Videos
OmniHuman-1 generates realistic videos from just an image and audio. It handles diverse visual and audio styles, producing videos in any aspect ratio and body proportion. The resulting animations boast detailed motion, lighting, and textures. (Note: Reference images are omitted for brevity but available upon request.)
Talking
Singing
Diversity
Halfbody Cases with Hands
Model Training and Architecture
OmniHuman-1's training leverages a multi-condition diffusion model. The core is a pre-trained Seaweed model (MMDiT architecture), initially trained on general text-video pairs. This is then adapted for human video generation by integrating text, audio, and pose signals. A causal 3D Variational Autoencoder (3DVAE) projects videos into a latent space for efficient denoising. The architecture cleverly reuses the denoising process to preserve subject identity and background from the reference image.
Model Architecture Diagram
The Omni-Conditions Training Strategy
This three-stage process progressively refines the diffusion model. It introduces conditioning modalities (text, audio, pose) sequentially, based on their motion correlation strength (weak to strong). This ensures a balanced contribution from each modality, optimizing animation quality. Audio conditioning uses wav2vec for feature extraction, and pose conditioning integrates pose heatmaps.
Experimental Validation and Performance
The paper presents rigorous experimental validation using a massive dataset (18.7K hours of human-related data). OmniHuman-1 outperforms existing methods across various metrics (IQA, ASE, Sync-C, FID, FVD), demonstrating its superior performance and versatility in handling different input configurations.
Ablation Study: Optimizing the Training Process
The ablation study explores the impact of different training data ratios for each modality. It reveals optimal ratios for audio and pose data, balancing realism and dynamic range. The study also highlights the importance of a sufficient reference image ratio for preserving identity and visual fidelity. Visualizations clearly demonstrate the effects of varying audio and pose condition ratios.
Extended Visual Results: Demonstrating Versatility
The extended visual results showcase OmniHuman-1's ability to generate diverse and high-quality animations, highlighting its capacity to handle various styles, object interactions, and pose-driven scenarios.
Conclusion
OmniHuman-1 represents a significant leap forward in human video generation. Its ability to create realistic animations from limited input and its multi-modal capabilities make it a truly remarkable achievement. This model is poised to revolutionize the field of digital animation.
The above is the detailed content of ByteDance Just Made AI Videos MIND BLOWING! - OmniHuman 1. For more information, please follow other related articles on the PHP Chinese website!
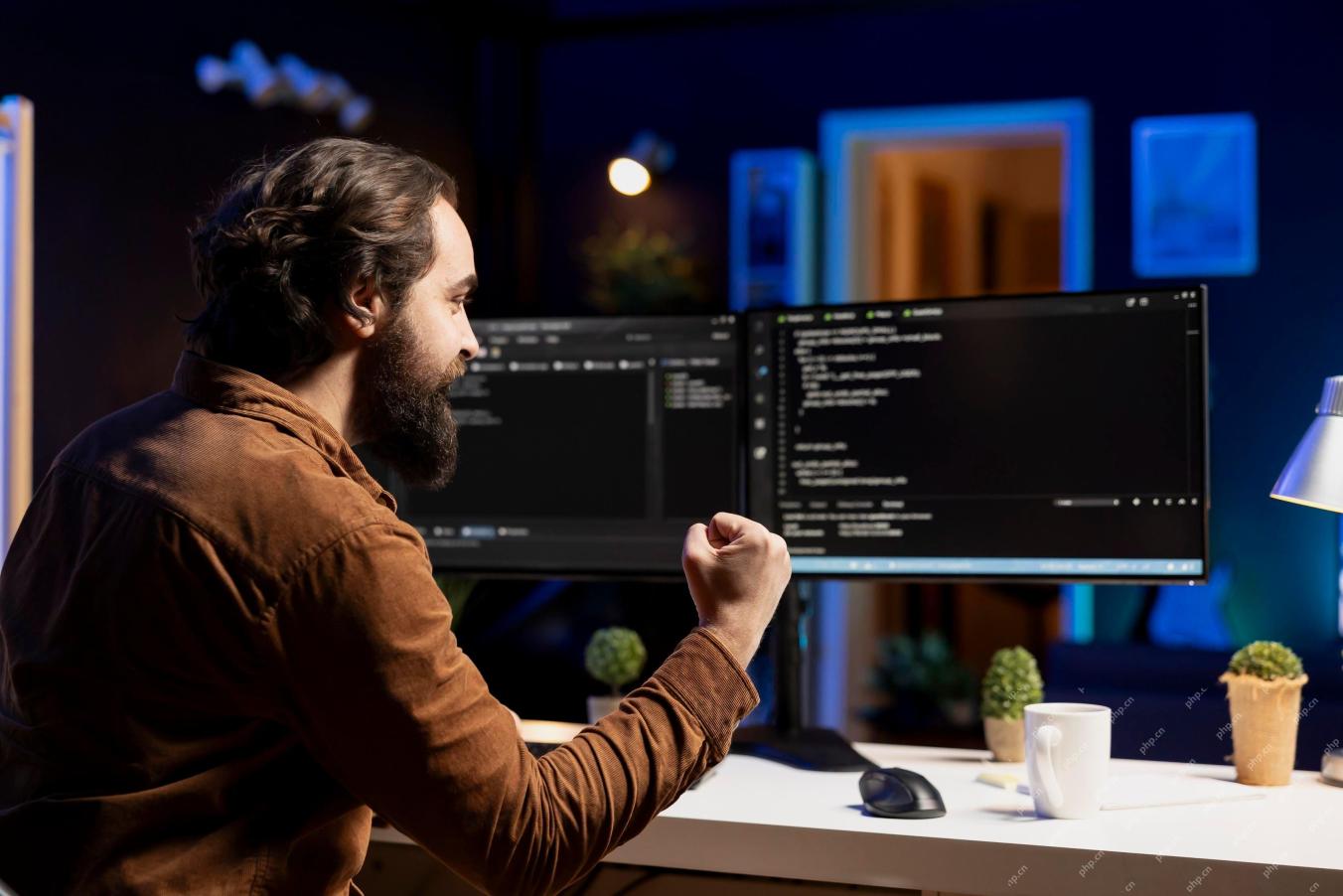
Let's discuss the rising use of "vibes" as an evaluation metric in the AI field. This analysis is part of my ongoing Forbes column on AI advancements, exploring complex aspects of AI development (see link here). Vibes in AI Assessment Tradi
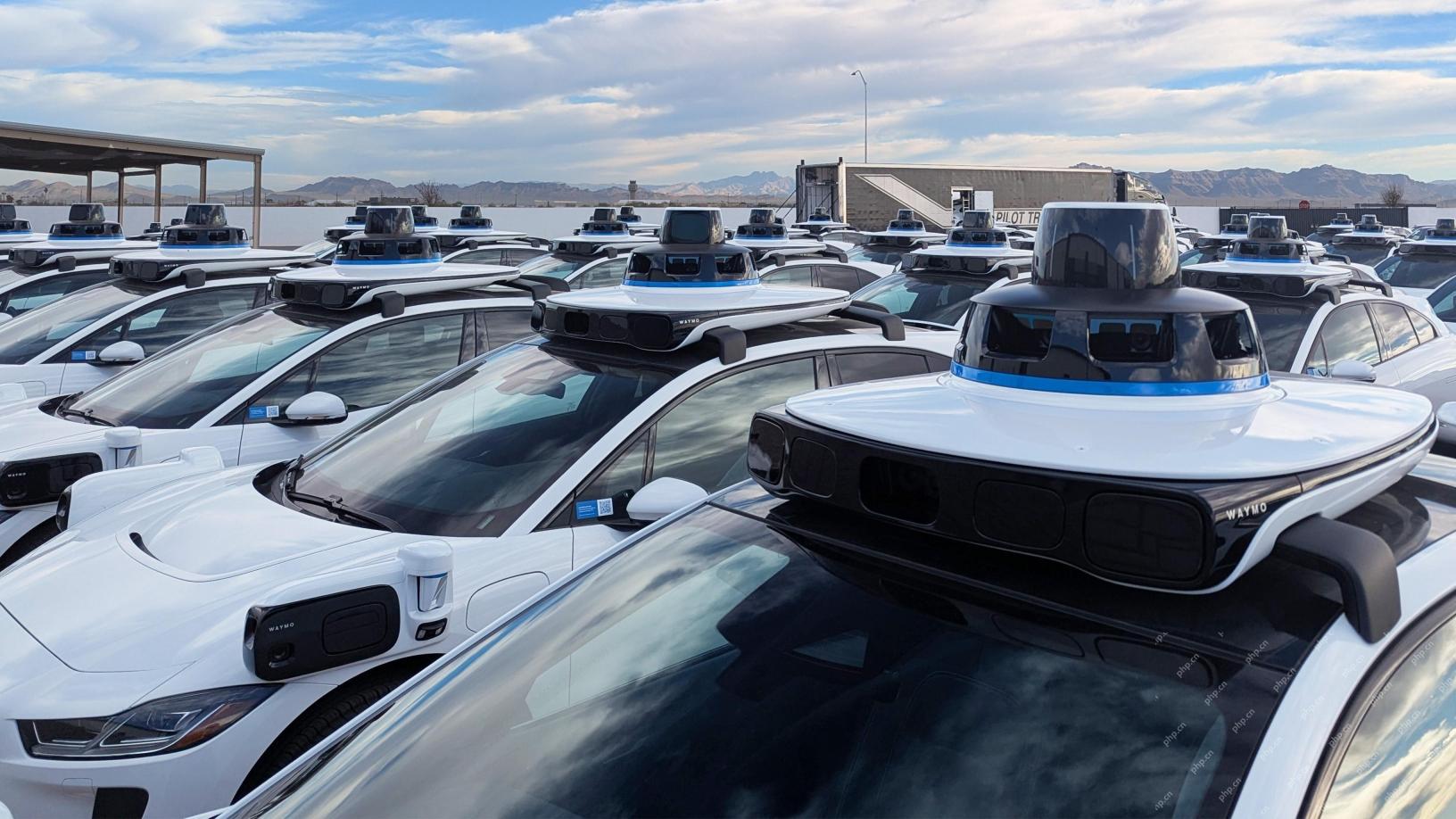
Waymo's Arizona Factory: Mass-Producing Self-Driving Jaguars and Beyond Located near Phoenix, Arizona, Waymo operates a state-of-the-art facility producing its fleet of autonomous Jaguar I-PACE electric SUVs. This 239,000-square-foot factory, opened
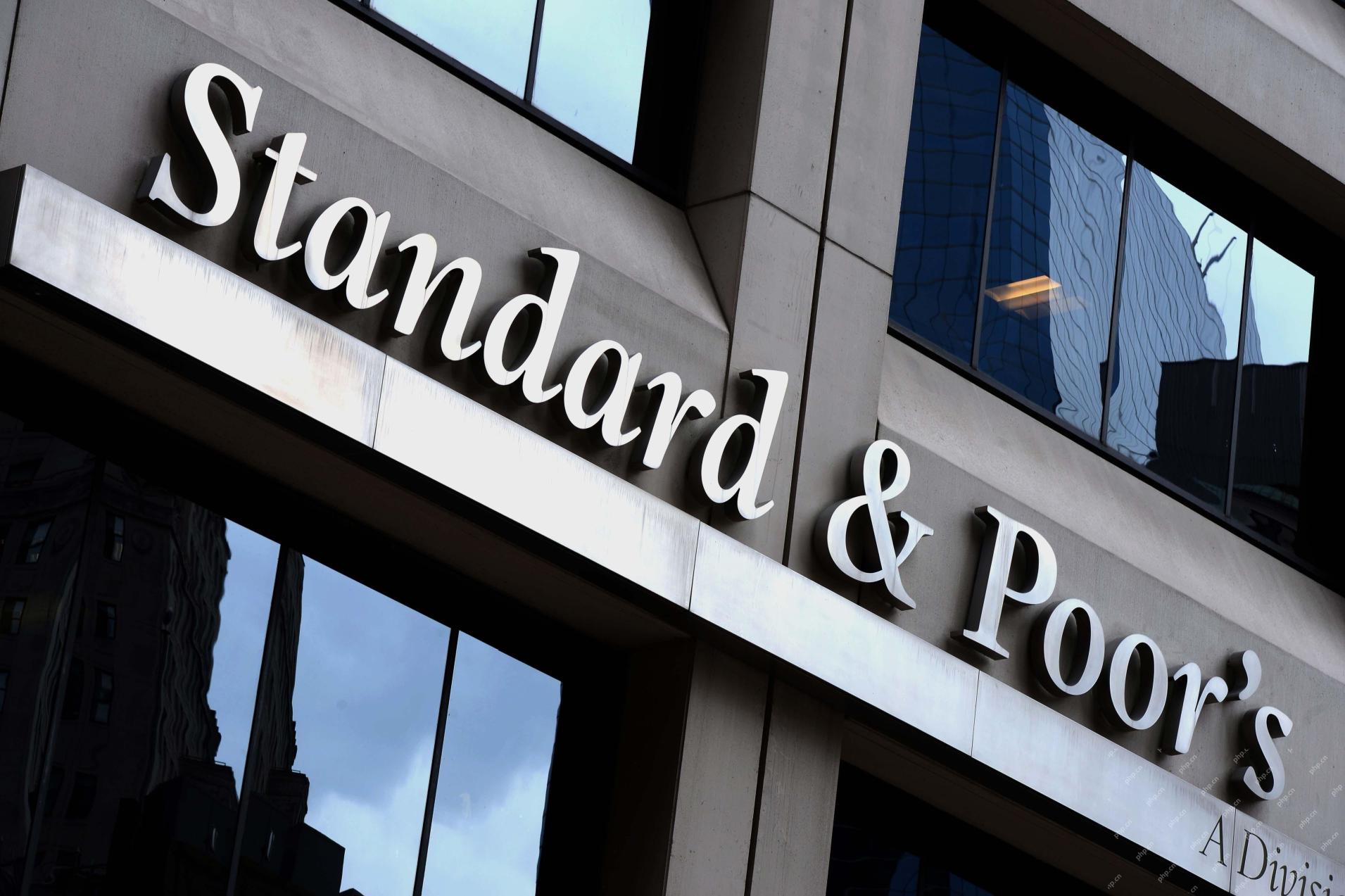
S&P Global's Chief Digital Solutions Officer, Jigar Kocherlakota, discusses the company's AI journey, strategic acquisitions, and future-focused digital transformation. A Transformative Leadership Role and a Future-Ready Team Kocherlakota's role
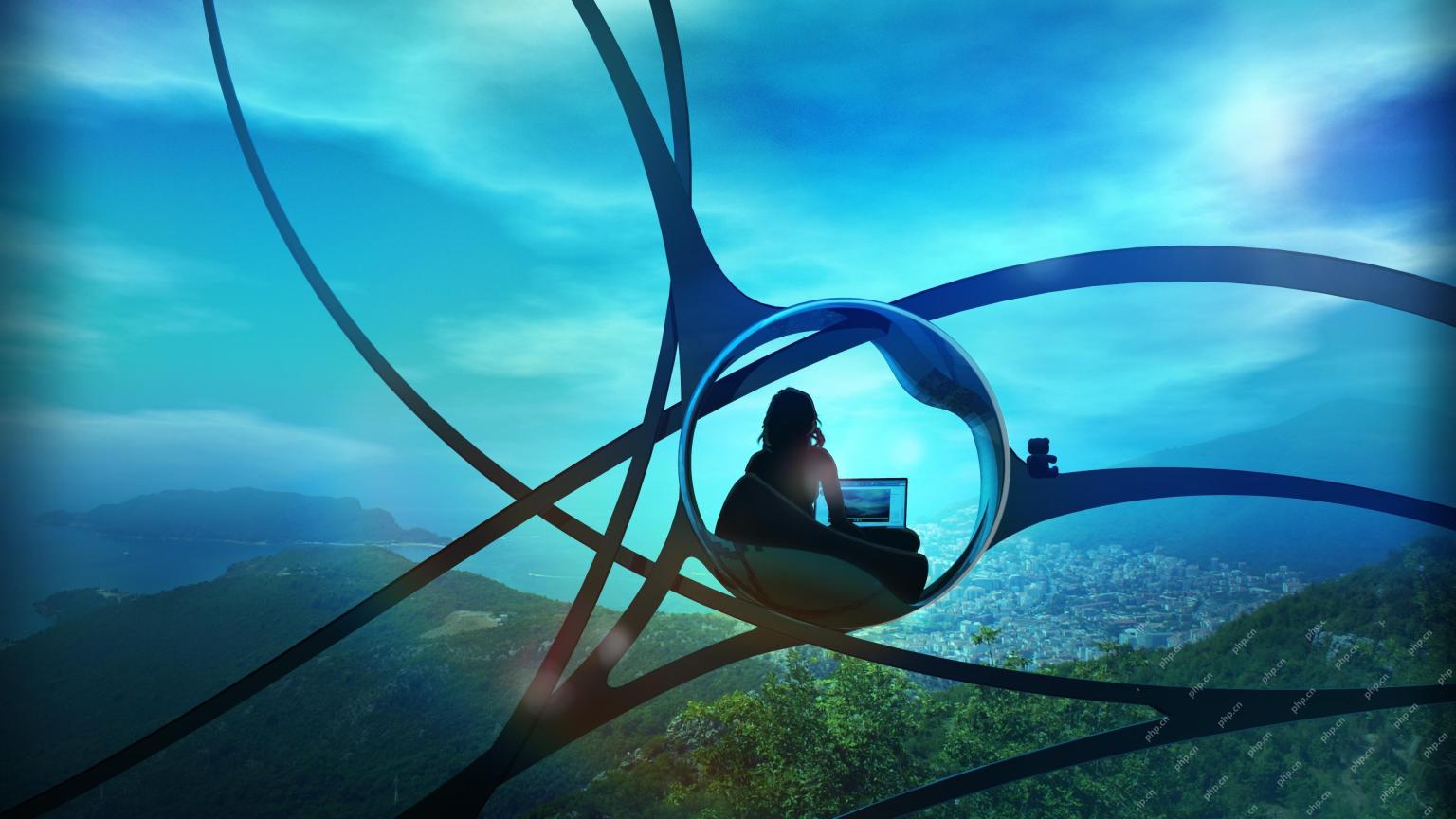
From Apps to Ecosystems: Navigating the Digital Landscape The digital revolution extends far beyond social media and AI. We're witnessing the rise of "everything apps"—comprehensive digital ecosystems integrating all aspects of life. Sam A
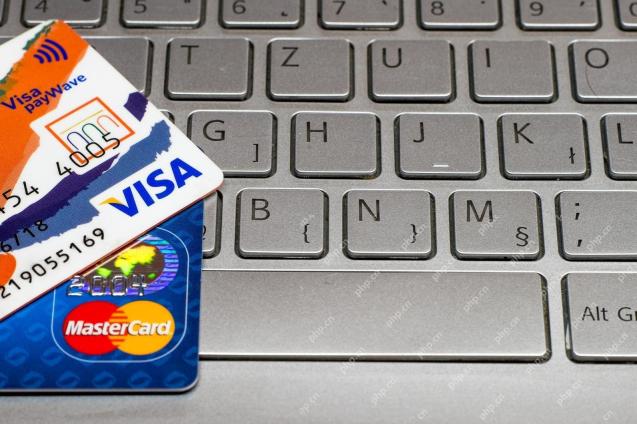
Mastercard's Agent Pay: AI-Powered Payments Revolutionize Commerce While Visa's AI-powered transaction capabilities made headlines, Mastercard has unveiled Agent Pay, a more advanced AI-native payment system built on tokenization, trust, and agentic
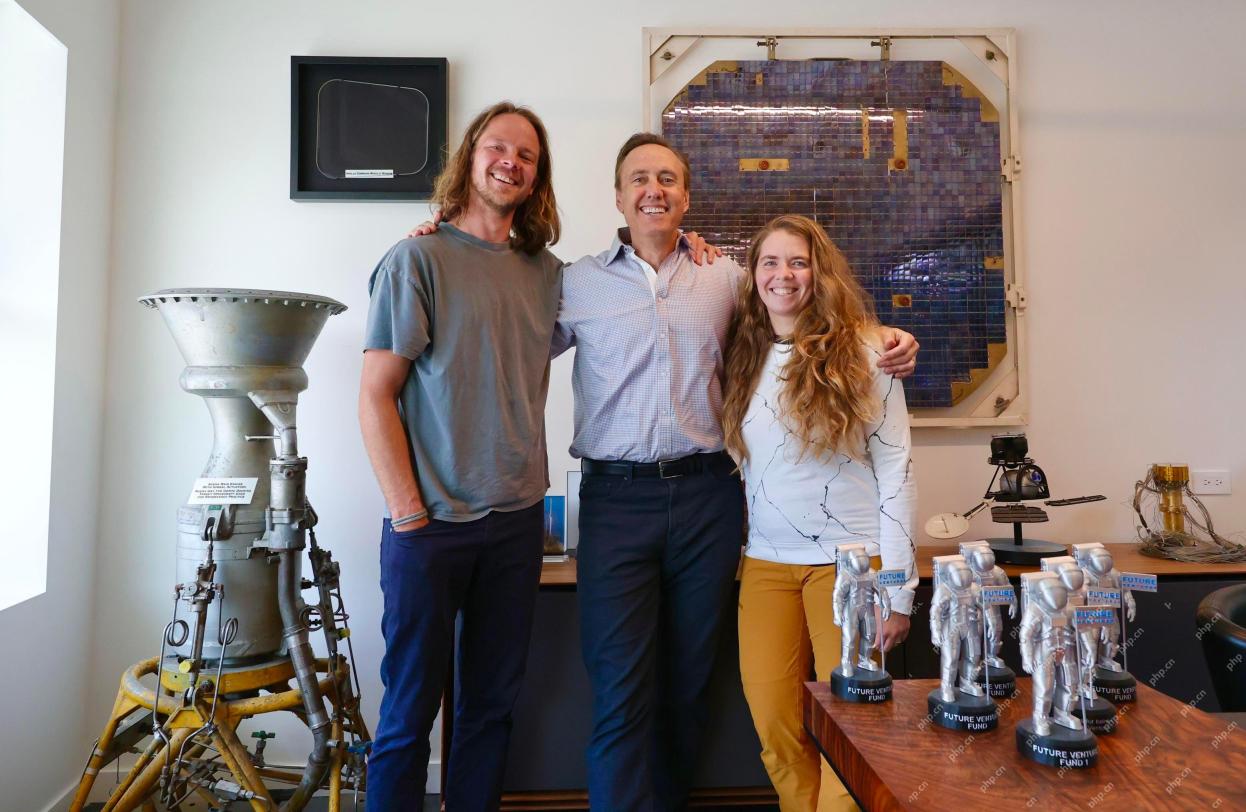
Future Ventures Fund IV: A $200M Bet on Novel Technologies Future Ventures recently closed its oversubscribed Fund IV, totaling $200 million. This new fund, managed by Steve Jurvetson, Maryanna Saenko, and Nico Enriquez, represents a significant inv
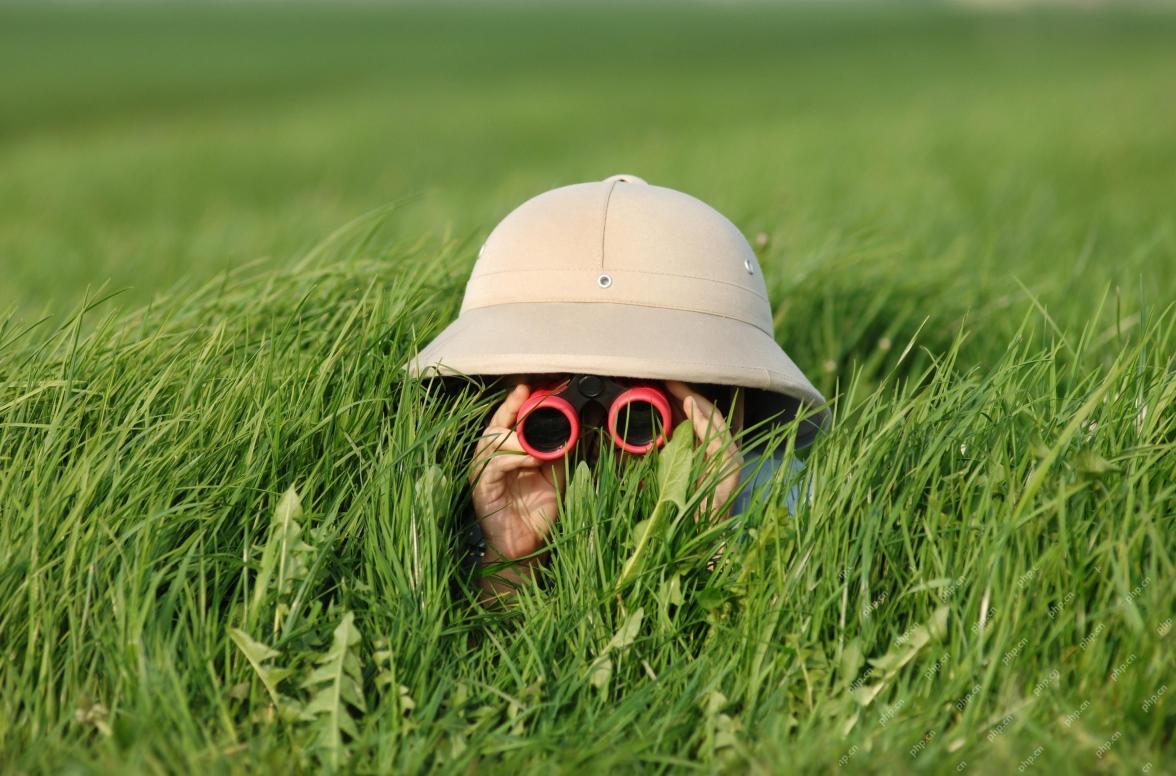
With the explosion of AI applications, enterprises are shifting from traditional search engine optimization (SEO) to generative engine optimization (GEO). Google is leading the shift. Its "AI Overview" feature has served over a billion users, providing full answers before users click on the link. [^2] Other participants are also rapidly rising. ChatGPT, Microsoft Copilot and Perplexity are creating a new “answer engine” category that completely bypasses traditional search results. If your business doesn't show up in these AI-generated answers, potential customers may never find you—even if you rank high in traditional search results. From SEO to GEO – What exactly does this mean? For decades
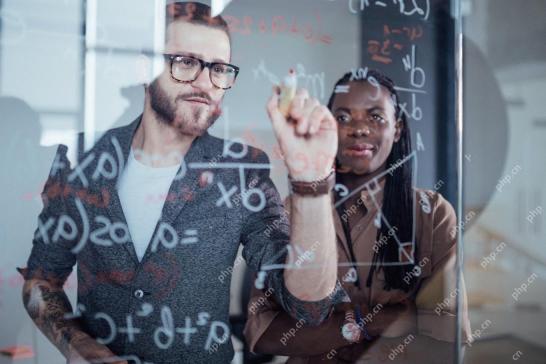
Let's explore the potential paths to Artificial General Intelligence (AGI). This analysis is part of my ongoing Forbes column on AI advancements, delving into the complexities of achieving AGI and Artificial Superintelligence (ASI). (See related art


Hot AI Tools

Undresser.AI Undress
AI-powered app for creating realistic nude photos

AI Clothes Remover
Online AI tool for removing clothes from photos.

Undress AI Tool
Undress images for free

Clothoff.io
AI clothes remover

Video Face Swap
Swap faces in any video effortlessly with our completely free AI face swap tool!

Hot Article

Hot Tools
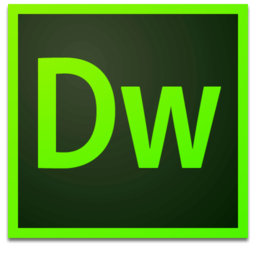
Dreamweaver Mac version
Visual web development tools
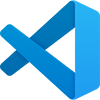
VSCode Windows 64-bit Download
A free and powerful IDE editor launched by Microsoft

SublimeText3 Chinese version
Chinese version, very easy to use

MinGW - Minimalist GNU for Windows
This project is in the process of being migrated to osdn.net/projects/mingw, you can continue to follow us there. MinGW: A native Windows port of the GNU Compiler Collection (GCC), freely distributable import libraries and header files for building native Windows applications; includes extensions to the MSVC runtime to support C99 functionality. All MinGW software can run on 64-bit Windows platforms.

SecLists
SecLists is the ultimate security tester's companion. It is a collection of various types of lists that are frequently used during security assessments, all in one place. SecLists helps make security testing more efficient and productive by conveniently providing all the lists a security tester might need. List types include usernames, passwords, URLs, fuzzing payloads, sensitive data patterns, web shells, and more. The tester can simply pull this repository onto a new test machine and he will have access to every type of list he needs.
