Machine learning often encounters the challenge of handling categorical variables (like colors, product types, or locations) due to algorithms' preference for numerical input. One-hot encoding offers a robust solution.
One-hot encoding transforms categorical data into numerical vectors. Each unique category gets its own binary column; a '1' signifies its presence, and '0' its absence. This article explores one-hot encoding, its advantages, and practical Python implementation using Pandas and Scikit-learn. Interested in a structured machine learning curriculum? Explore this four-course Machine Learning Fundamentals With Python track.
Understanding One-Hot Encoding
One-hot encoding converts categorical variables into a machine-learning-friendly format, boosting prediction accuracy. It creates new binary columns for each unique category within a feature. A '1' or '0' indicates the category's presence or absence.
Consider a dataset with a 'Color' feature (Red, Green, Blue). One-hot encoding transforms it as follows:
The original 'Color' column is replaced by three binary columns, one for each color. A '1' shows the color's presence in that row.
Benefits of One-Hot Encoding
One-hot encoding is crucial in data preprocessing because it:
- Enhances Machine Learning Compatibility: Transforms categorical data into a format easily understood and utilized by machine learning models. Each category is treated independently, preventing false relationships.
- Avoids Ordinality Issues: Unlike label encoding (assigning numbers to categories), one-hot encoding prevents the model from misinterpreting an order or ranking where none exists. Label encoding, assigning 1 to Red, 2 to Green, and 3 to Blue, might falsely suggest Green > Red. One-hot encoding avoids this. Label encoding is appropriate for inherently ordinal data (e.g., education levels: High School, Bachelor's, Master's, PhD).
Implementing One-Hot Encoding in Python
Pandas and Scikit-learn simplify one-hot encoding in Python.
Pandas get_dummies()
: A simple method for straightforward encoding.
import pandas as pd data = {'Color': ['Red', 'Green', 'Blue', 'Red']} df = pd.DataFrame(data) df_encoded = pd.get_dummies(df, dtype=int) print(df_encoded)
Scikit-learn's OneHotEncoder
: Offers more control, especially for complex scenarios.
from sklearn.preprocessing import OneHotEncoder import numpy as np enc = OneHotEncoder(handle_unknown='ignore') X = [['Red'], ['Green'], ['Blue']] enc.fit(X) result = enc.transform([['Red']]).toarray() print(result)
<code>[[1. 0. 0.]]</code>
Handling High-Cardinality Features
High-cardinality categorical features (many unique values) present a challenge ("curse of dimensionality"). Solutions include:
- Feature Hashing: Hashes categories into a fixed number of columns, managing dimensionality efficiently.
- Dimensionality Reduction (PCA): Reduces dimensions after one-hot encoding, preserving essential information.
Best Practices
-
Handling Unknown Categories: Scikit-learn's
OneHotEncoder
handles unseen categories during model deployment usinghandle_unknown='ignore'
. - Dropping the Original Column: Avoid multicollinearity by removing the original categorical column after one-hot encoding.
-
OneHotEncoder
vs.get_dummies()
: Choose based on complexity;get_dummies()
for simplicity,OneHotEncoder
for more control.
Conclusion
One-hot encoding is a vital technique for preparing categorical data for machine learning. It improves model accuracy and efficiency. Python libraries like Pandas and Scikit-learn provide efficient implementation. Remember to consider dimensionality and unknown categories. For further learning, explore this Preprocessing for Machine Learning in Python course.
FAQs
- Missing Values: One-hot encoding doesn't handle missing values directly; address them beforehand.
- Suitability: Ideal for nominal data, less so for ordinal data.
- Large Datasets: Increased dimensionality can impact performance; use feature hashing or dimensionality reduction.
- Text Data: Word embeddings or TF-IDF are often preferred over one-hot encoding for text.
- Choosing Encoding Techniques: Consider the data's nature, model requirements, and dimensionality impact.
The above is the detailed content of What Is One Hot Encoding and How to Implement It in Python. For more information, please follow other related articles on the PHP Chinese website!
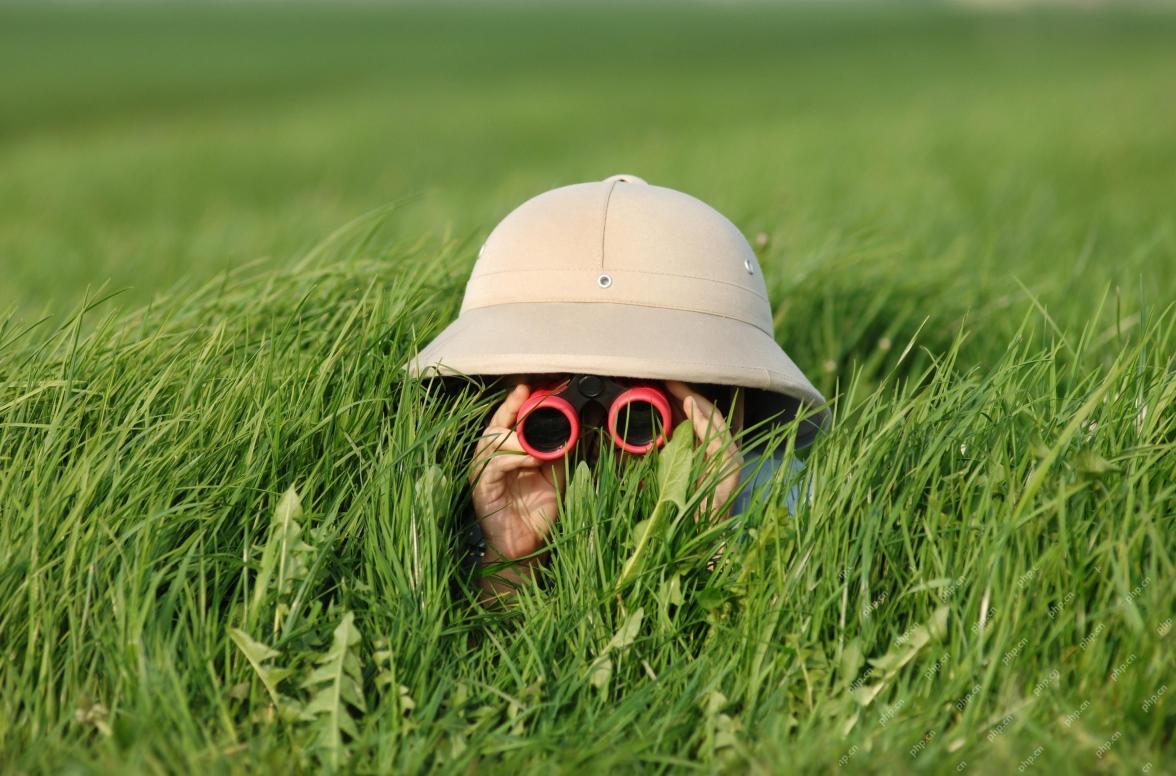
With the explosion of AI applications, enterprises are shifting from traditional search engine optimization (SEO) to generative engine optimization (GEO). Google is leading the shift. Its "AI Overview" feature has served over a billion users, providing full answers before users click on the link. [^2] Other participants are also rapidly rising. ChatGPT, Microsoft Copilot and Perplexity are creating a new “answer engine” category that completely bypasses traditional search results. If your business doesn't show up in these AI-generated answers, potential customers may never find you—even if you rank high in traditional search results. From SEO to GEO – What exactly does this mean? For decades
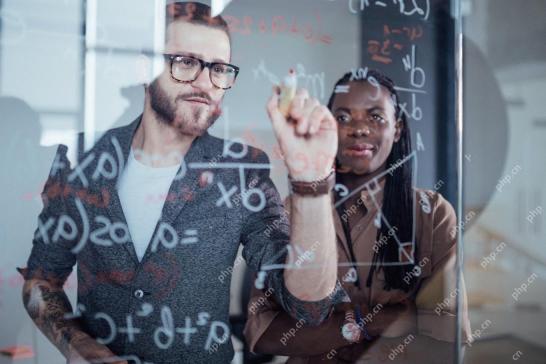
Let's explore the potential paths to Artificial General Intelligence (AGI). This analysis is part of my ongoing Forbes column on AI advancements, delving into the complexities of achieving AGI and Artificial Superintelligence (ASI). (See related art
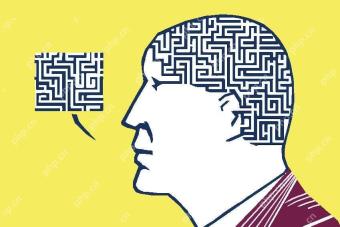
Human-computer interaction: a delicate dance of adaptation Interacting with an AI chatbot is like participating in a delicate dance of mutual influence. Your questions, responses, and preferences gradually shape the system to better meet your needs. Modern language models adapt to user preferences through explicit feedback mechanisms and implicit pattern recognition. They learn your communication style, remember your preferences, and gradually adjust their responses to fit your expectations. Yet, while we train our digital partners, something equally important is happening in the reverse direction. Our interactions with these systems are subtly reshaping our own communication patterns, thinking processes, and even expectations of interpersonal conversations. Our interactions with AI systems have begun to reshape our expectations of interpersonal interactions. We adapted to instant response,
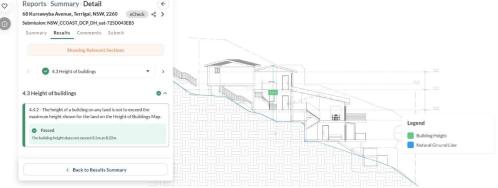
AI Streamlines Wildfire Recovery Permitting Australian tech firm Archistar's AI software, utilizing machine learning and computer vision, automates the assessment of building plans for compliance with local regulations. This pre-validation significan
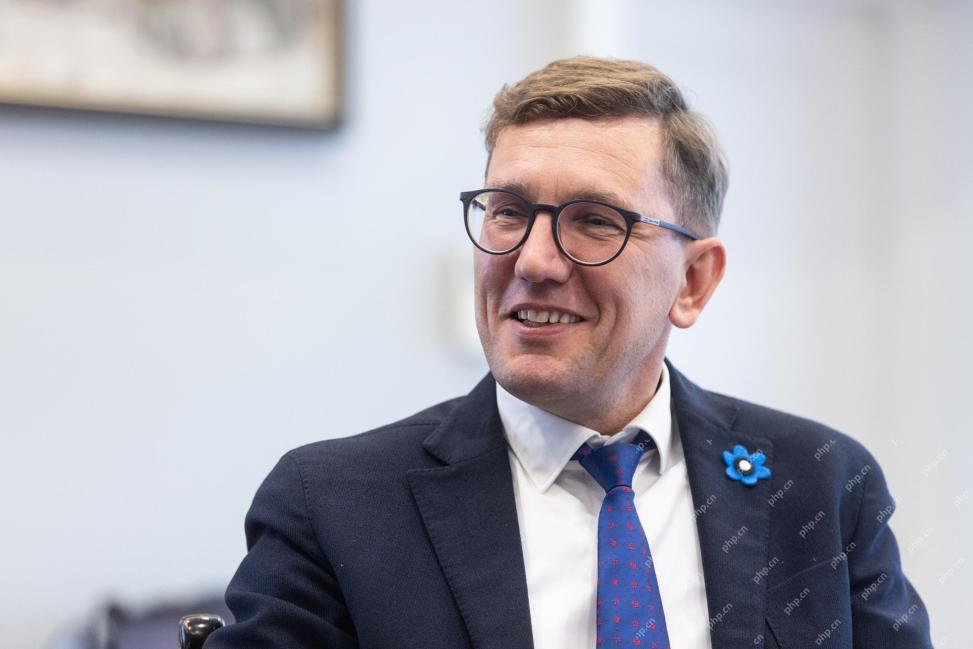
Estonia's Digital Government: A Model for the US? The US struggles with bureaucratic inefficiencies, but Estonia offers a compelling alternative. This small nation boasts a nearly 100% digitized, citizen-centric government powered by AI. This isn't
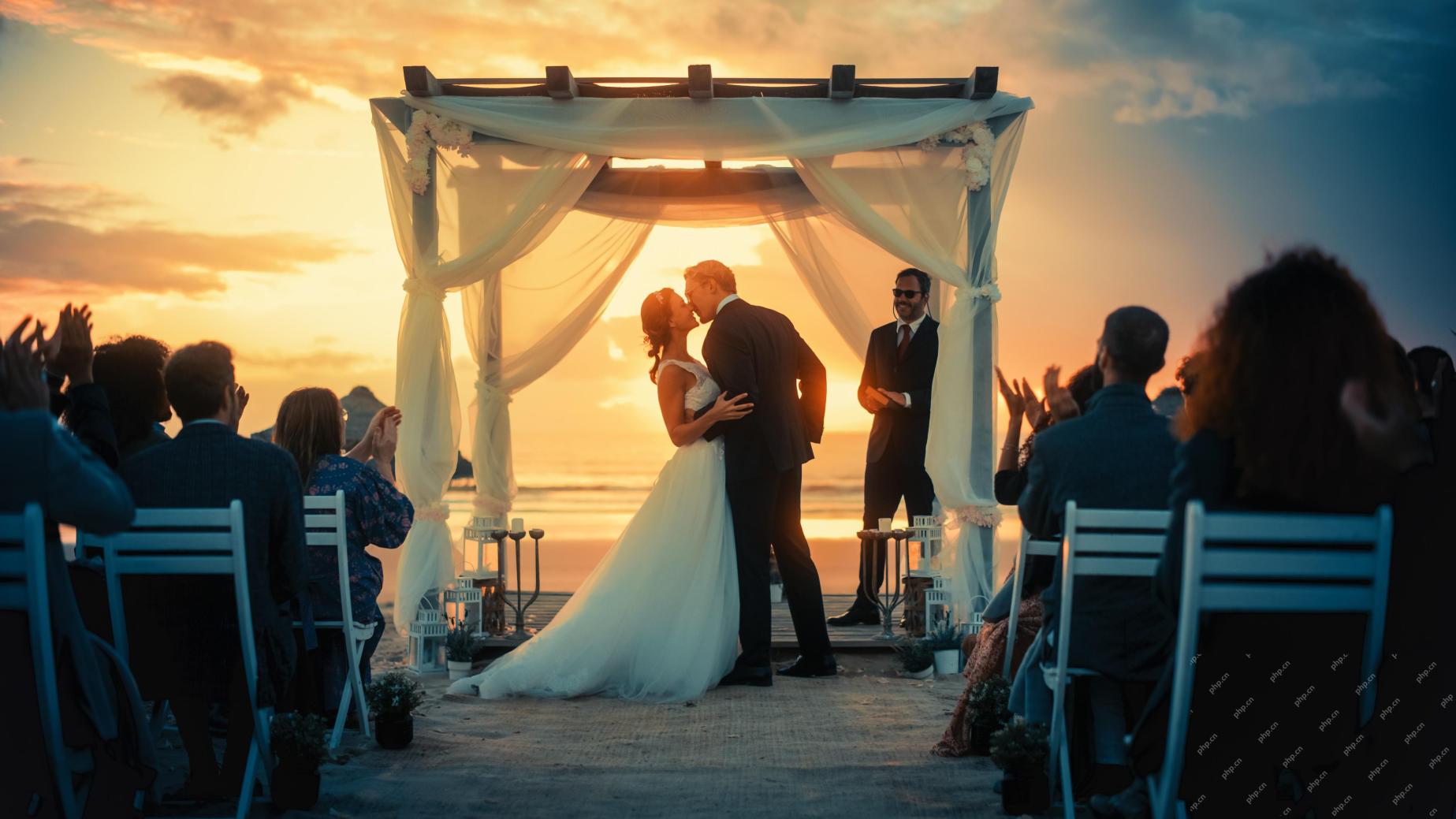
Planning a wedding is a monumental task, often overwhelming even the most organized couples. This article, part of an ongoing Forbes series on AI's impact (see link here), explores how generative AI can revolutionize wedding planning. The Wedding Pl
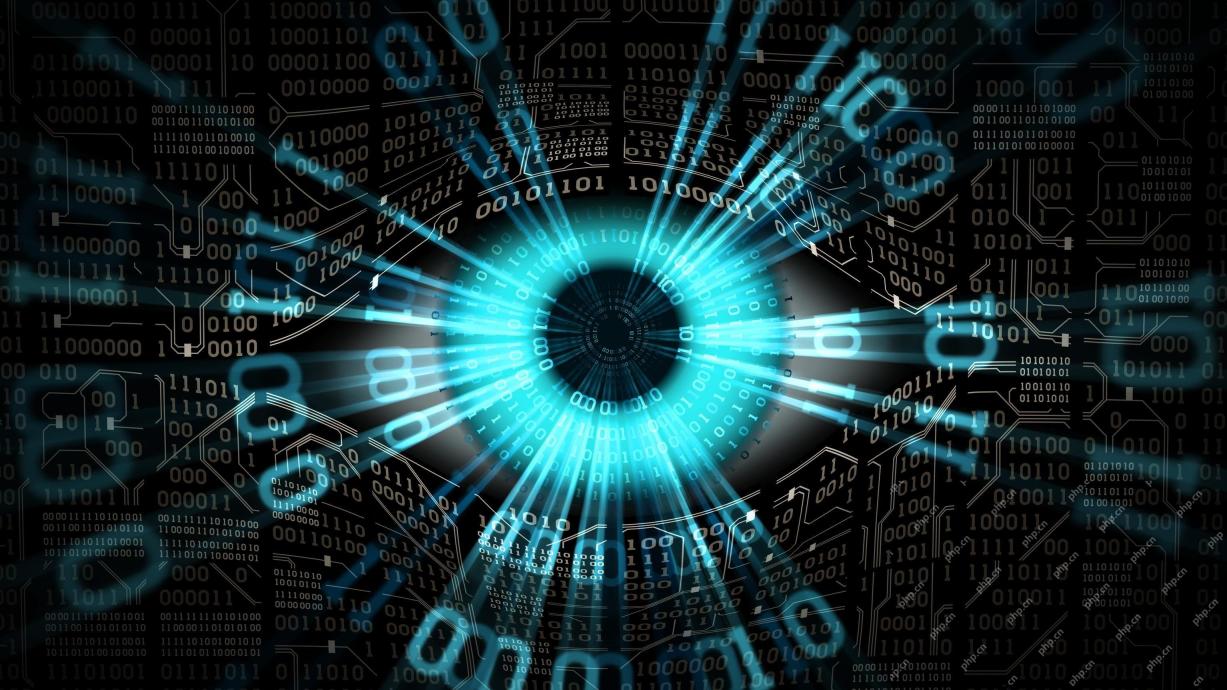
Businesses increasingly leverage AI agents for sales, while governments utilize them for various established tasks. However, consumer advocates highlight the need for individuals to possess their own AI agents as a defense against the often-targeted
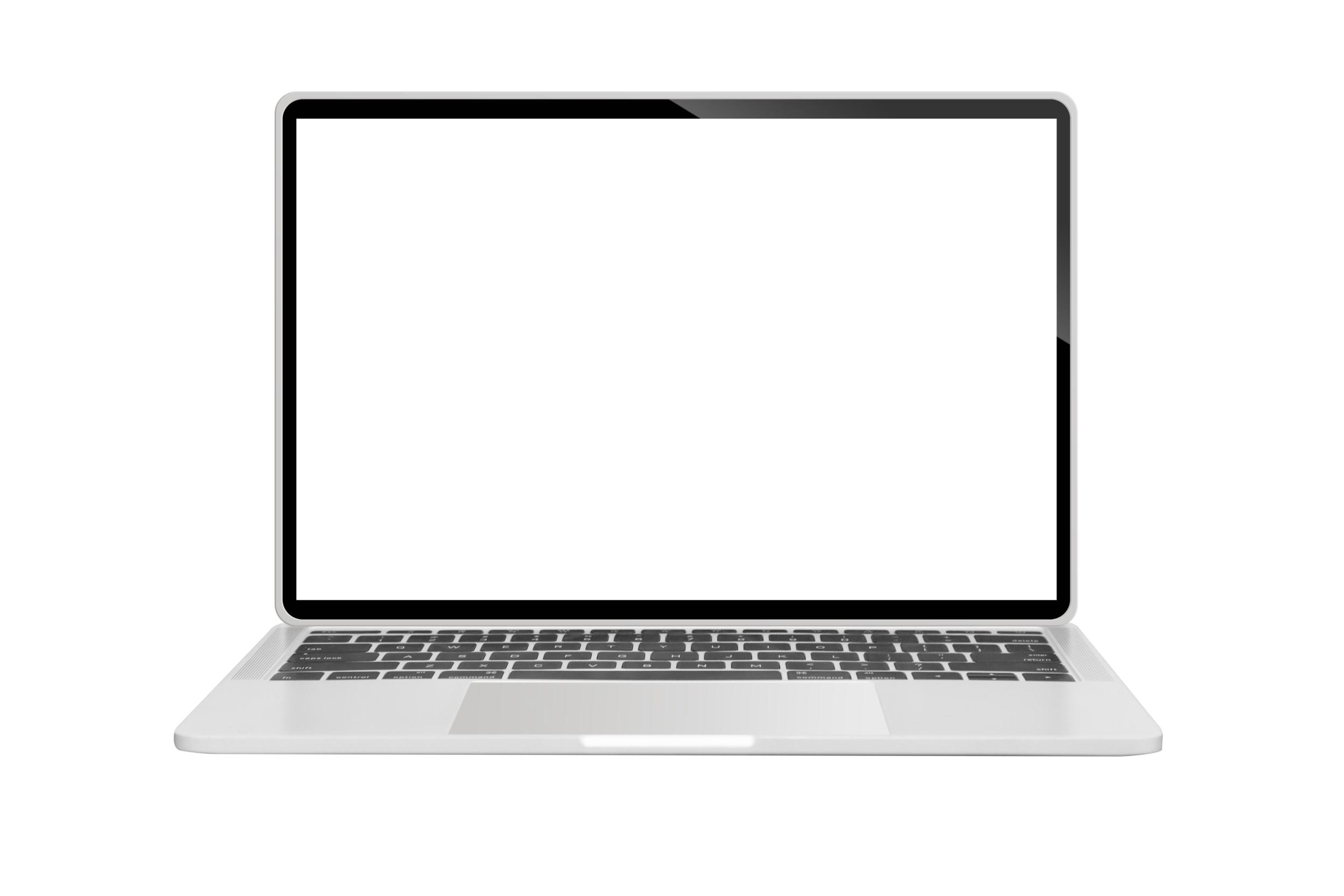
Google is leading this shift. Its "AI Overviews" feature already serves more than one billion users, providing complete answers before anyone clicks a link.[^2] Other players are also gaining ground fast. ChatGPT, Microsoft Copilot, and Pe


Hot AI Tools

Undresser.AI Undress
AI-powered app for creating realistic nude photos

AI Clothes Remover
Online AI tool for removing clothes from photos.

Undress AI Tool
Undress images for free

Clothoff.io
AI clothes remover

Video Face Swap
Swap faces in any video effortlessly with our completely free AI face swap tool!

Hot Article

Hot Tools

Notepad++7.3.1
Easy-to-use and free code editor

DVWA
Damn Vulnerable Web App (DVWA) is a PHP/MySQL web application that is very vulnerable. Its main goals are to be an aid for security professionals to test their skills and tools in a legal environment, to help web developers better understand the process of securing web applications, and to help teachers/students teach/learn in a classroom environment Web application security. The goal of DVWA is to practice some of the most common web vulnerabilities through a simple and straightforward interface, with varying degrees of difficulty. Please note that this software
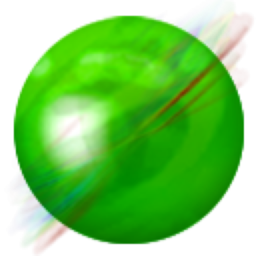
ZendStudio 13.5.1 Mac
Powerful PHP integrated development environment
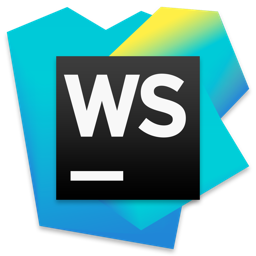
WebStorm Mac version
Useful JavaScript development tools
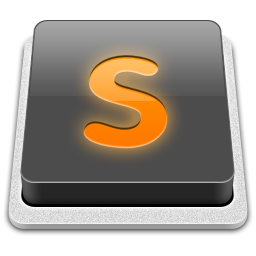
SublimeText3 Mac version
God-level code editing software (SublimeText3)
