


Quantization for Large Language Models (LLMs): Reduce AI Model Sizes Efficiently
Run Your Own ChatGPT on Your Laptop: A Guide to LLM Quantization
Ever dreamed of running your own ChatGPT directly on your laptop? Thanks to advancements in Large Language Models (LLMs), this is becoming a reality. The key is quantization—a technique that shrinks these massive models to fit on consumer hardware with minimal performance loss (when done right!). This guide explains quantization, its methods, and shows you how to quantize a model using Hugging Face's Quanto library in two easy steps. Follow along using the DataCamp DataLab.
The Ever-Growing Size of LLMs
LLMs have exploded in complexity. GPT-1 (2018) had 0.11 billion parameters; GPT-2 (2019), 1.5 billion; GPT-3 (2020), a whopping 175 billion; and GPT-4 boasts over 1 trillion. This massive growth creates a memory bottleneck, hindering both training and inference, and limiting accessibility. Quantization solves this by reducing the model's size while preserving performance.
Understanding Quantization
Quantization is a model compression technique that reduces the precision of a model's weights and activations. This involves converting data from a higher-precision type (e.g., 32-bit floating-point) to a lower-precision type (e.g., 8-bit integer). Fewer bits mean a smaller model, consuming less memory, storage, and energy.
Think of image compression: High-resolution images are compressed for web use, reducing size and loading time at the cost of some detail. Similarly, quantizing an LLM reduces computational demands, enabling it to run on less powerful hardware.
Image compression for faster web loading.
Quantization introduces noise (quantization error), but research focuses on minimizing this to maintain performance.
The Theory Behind Quantization
Quantization typically targets model weights—the parameters determining the strength of connections between neurons. These weights are initially random and adjusted during training. A simple example is rounding weights to fewer decimal places.
Example: A weight matrix (left) and its quantized version (right).
The difference between the original and quantized matrices is the quantization error.
Quantization error matrix.
In practice, quantization involves changing the data type (downcasting). For example, converting from float32 (4 bytes per parameter) to int8 (1 byte per parameter) significantly reduces memory usage.
Brain Floating Point (BF16) and Downcasting
BF16, developed by Google, offers a balance between float32's dynamic range and float16's efficiency. Downcasting—converting from a higher-precision to a lower-precision data type—increases speed but can lead to data loss and error propagation, especially with smaller data types.
Types of Quantization
Several quantization types exist:
- Linear Quantization: Maps floating-point values to a fixed-point range evenly. It involves calculating minimum/maximum values, scale, zero-point, quantizing, and dequantizing (during inference).
Linear quantization equations.
Example: Linear quantization of a weight matrix.
Dequantization and quantization error.
-
Blockwise Quantization: Quantizes weights in smaller blocks, handling non-uniform distributions more effectively.
-
Weight vs. Activation Quantization: Quantization can be applied to both weights (static) and activations (dynamic). Activation quantization is more complex.
-
Post-Training Quantization (PTQ) vs. Quantization-Aware Training (QAT): PTQ quantizes a pre-trained model; QAT modifies training to simulate quantization effects, leading to better accuracy but increased training time.
Calibration Techniques
Some methods require calibration—running inference on a dataset to optimize quantization parameters. Techniques include percentile calibration and mean/standard deviation calibration. Methods like QLoRA avoid calibration.
Tools for Quantization
Several Python libraries support quantization, including PyTorch and TensorFlow. Hugging Face's Quanto library simplifies the process for PyTorch models.
Quantizing a Model with Hugging Face's Quanto
Here's a step-by-step guide using the Pythia 410M model:
- Load the Model: Load the pre-trained model and tokenizer.
from transformers import AutoModelForCausalLM, AutoTokenizer model_name = "EleutherAI/pythia-410m" model = AutoModelForCausalLM.from_pretrained(model_name, low_cpu_mem_usage=True) tokenizer = AutoTokenizer.from_pretrained(model_name)
-
Quantize: Use
quantize()
to convert the model.
from quanto import quantize, freeze quantize(model, weights=torch.int8, activations=None)
-
Freeze: Use
freeze()
to apply quantization to the weights.
freeze(model)
-
Check Results: Verify the reduced model size and test inference. (Note:
compute_module_sizes()
is a custom function; see DataCamp DataLab for implementation).
Conclusion
Quantization makes LLMs more accessible and efficient. By understanding its techniques and using tools like Hugging Face's Quanto, you can run powerful models on less powerful hardware. For larger models, consider upgrading your resources.
LLM Quantization FAQs
- QAT vs. PTQ: QAT generally performs better but requires more resources during training.
-
Quanto Library: Supports both PTQ and QAT.
quantize()
includes implicit calibration; acalibration()
method is available for custom calibration. - Precision: Int4 and Int2 quantization is possible.
-
Accessing Hugging Face Models: Change the
model_name
variable to the desired model. Remember to accept Hugging Face's terms and conditions.
The above is the detailed content of Quantization for Large Language Models (LLMs): Reduce AI Model Sizes Efficiently. For more information, please follow other related articles on the PHP Chinese website!
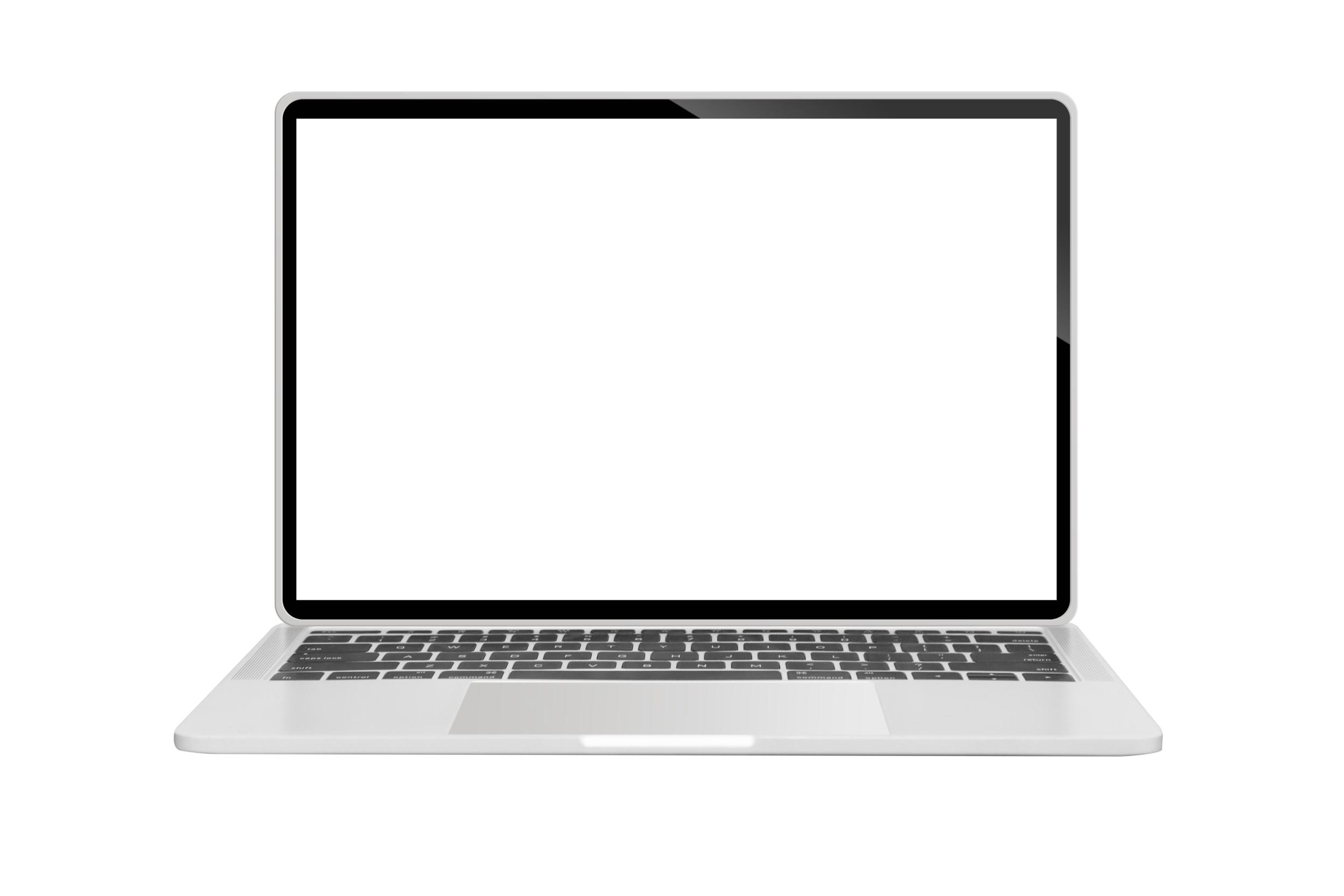
Google is leading this shift. Its "AI Overviews" feature already serves more than one billion users, providing complete answers before anyone clicks a link.[^2] Other players are also gaining ground fast. ChatGPT, Microsoft Copilot, and Pe
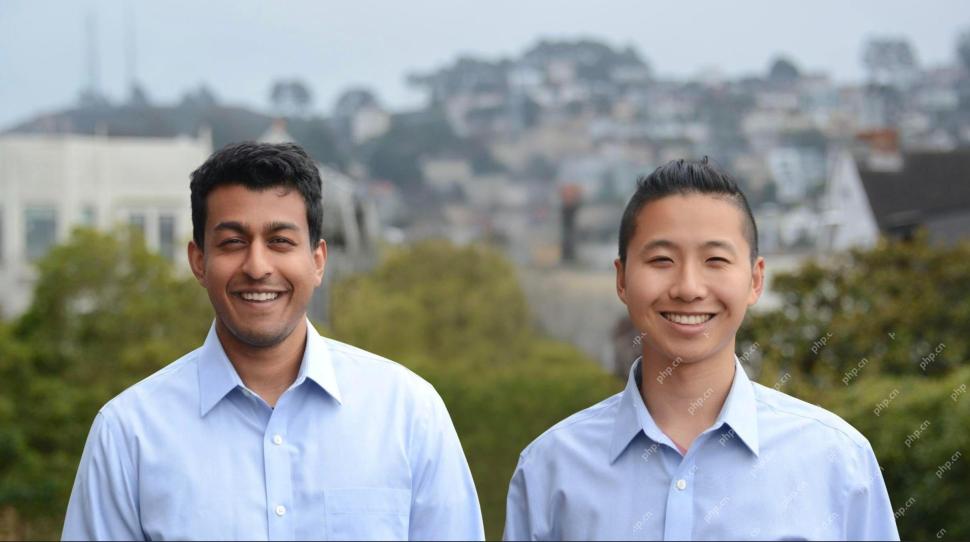
In 2022, he founded social engineering defense startup Doppel to do just that. And as cybercriminals harness ever more advanced AI models to turbocharge their attacks, Doppel’s AI systems have helped businesses combat them at scale— more quickly and
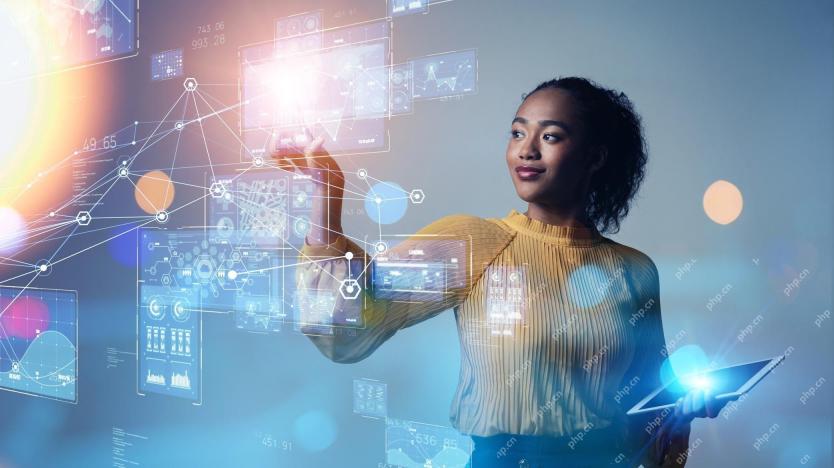
Voila, via interacting with suitable world models, generative AI and LLMs can be substantively boosted. Let’s talk about it. This analysis of an innovative AI breakthrough is part of my ongoing Forbes column coverage on the latest in AI, including
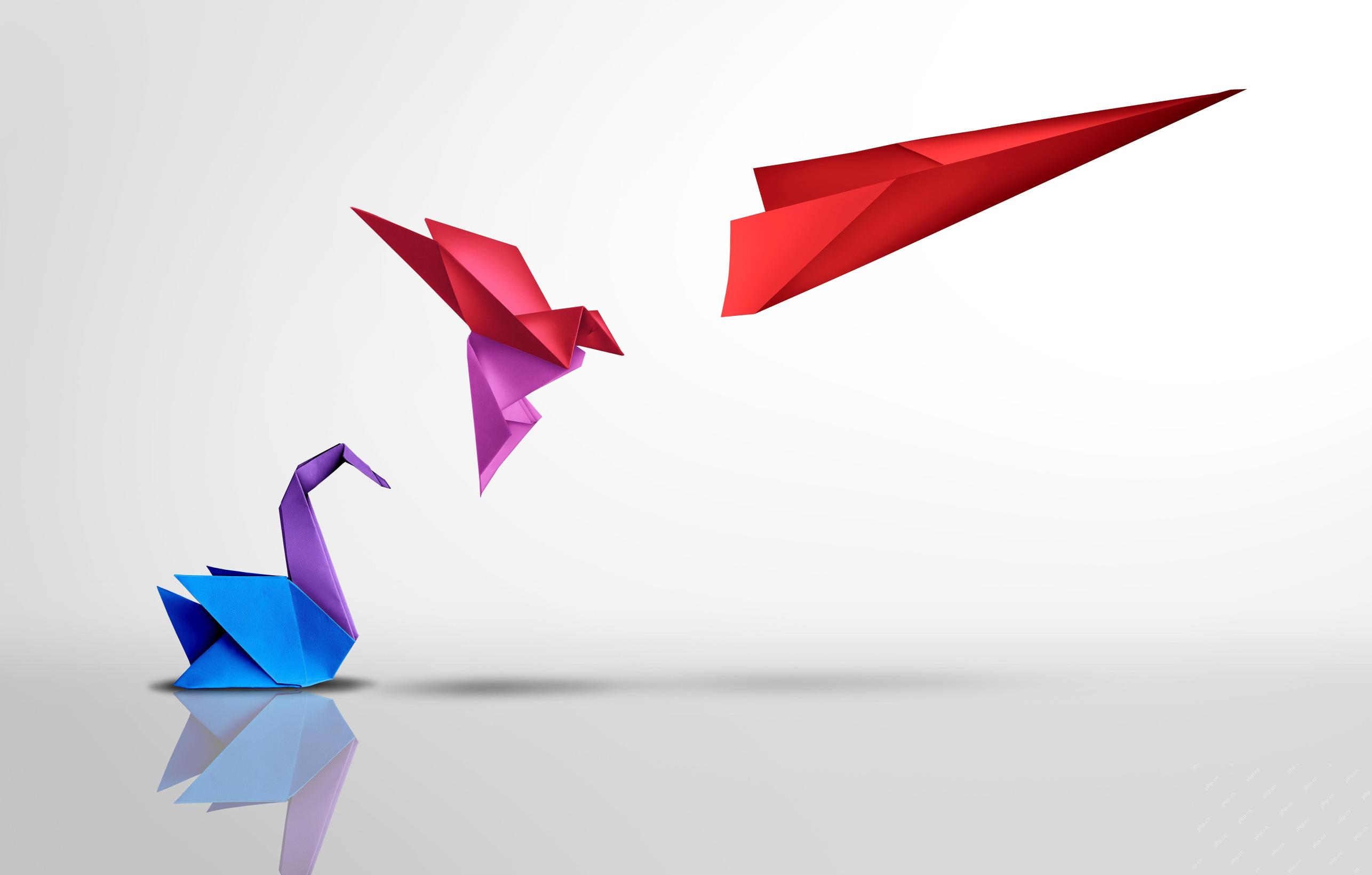
Labor Day 2050. Parks across the nation fill with families enjoying traditional barbecues while nostalgic parades wind through city streets. Yet the celebration now carries a museum-like quality — historical reenactment rather than commemoration of c
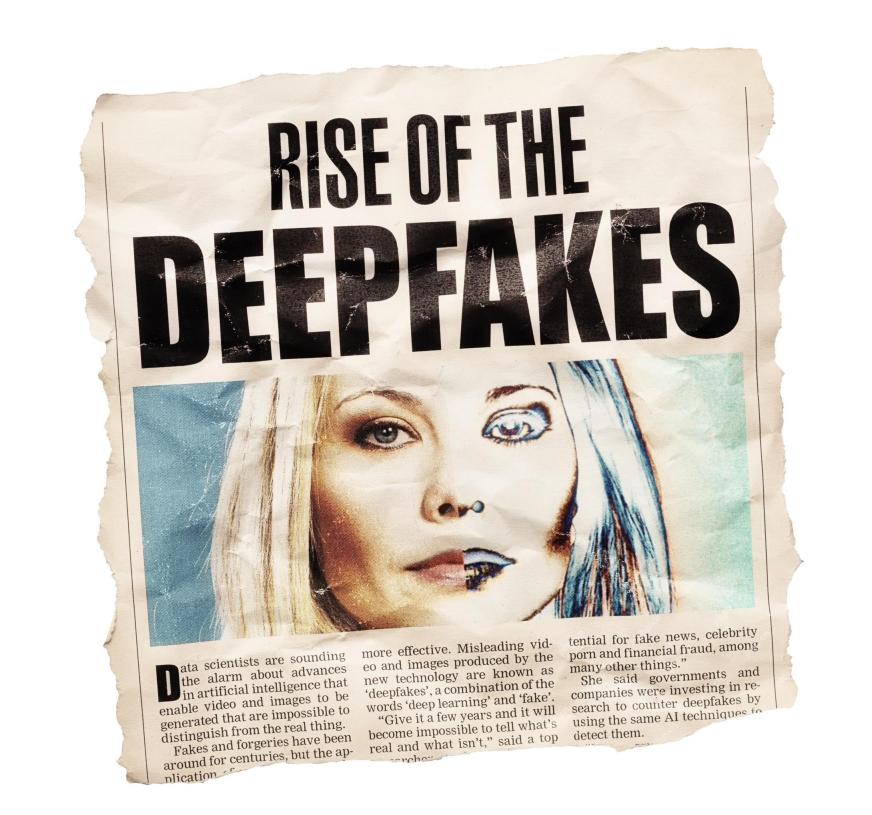
To help address this urgent and unsettling trend, a peer-reviewed article in the February 2025 edition of TEM Journal provides one of the clearest, data-driven assessments as to where that technological deepfake face off currently stands. Researcher
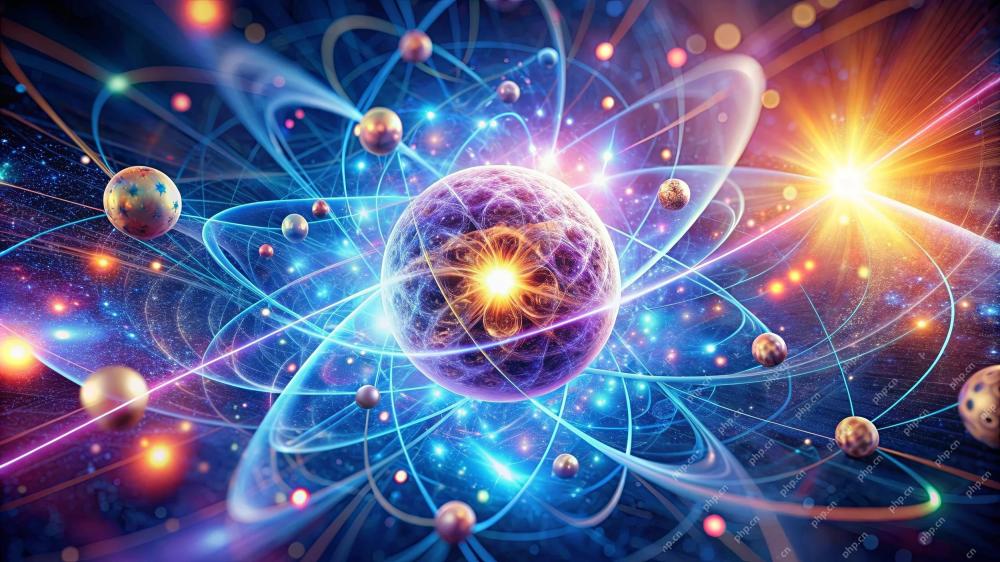
From vastly decreasing the time it takes to formulate new drugs to creating greener energy, there will be huge opportunities for businesses to break new ground. There’s a big problem, though: there’s a severe shortage of people with the skills busi
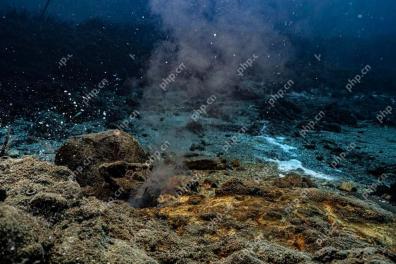
Years ago, scientists found that certain kinds of bacteria appear to breathe by generating electricity, rather than taking in oxygen, but how they did so was a mystery. A new study published in the journal Cell identifies how this happens: the microb
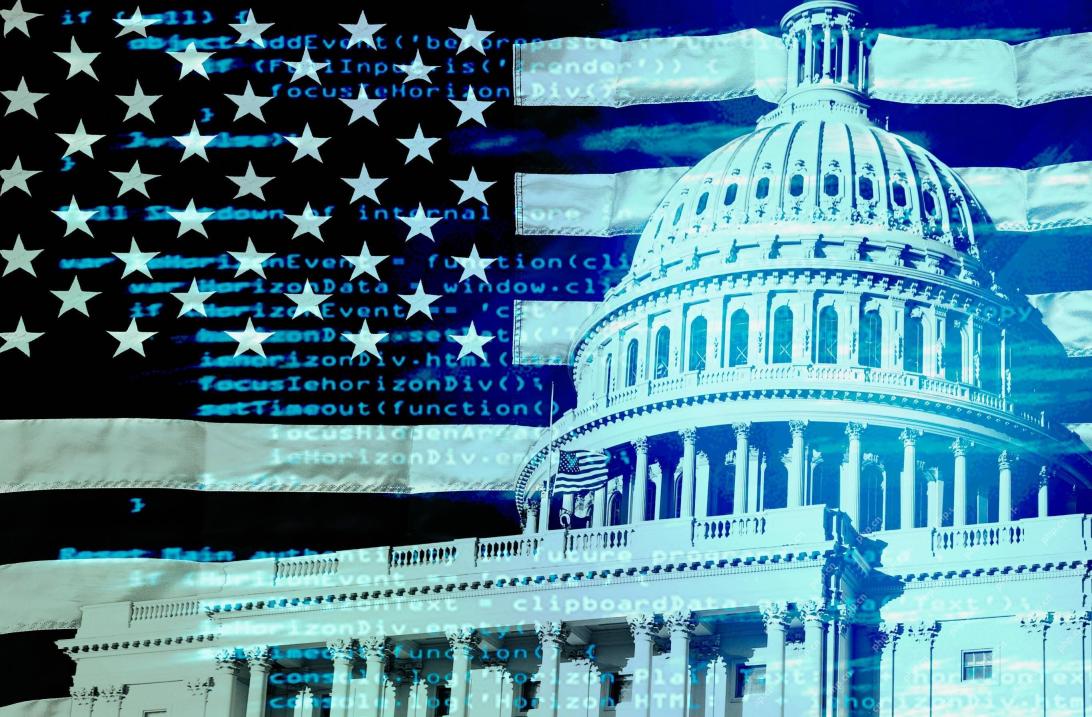
At the RSAC 2025 conference this week, Snyk hosted a timely panel titled “The First 100 Days: How AI, Policy & Cybersecurity Collide,” featuring an all-star lineup: Jen Easterly, former CISA Director; Nicole Perlroth, former journalist and partne


Hot AI Tools

Undresser.AI Undress
AI-powered app for creating realistic nude photos

AI Clothes Remover
Online AI tool for removing clothes from photos.

Undress AI Tool
Undress images for free

Clothoff.io
AI clothes remover

Video Face Swap
Swap faces in any video effortlessly with our completely free AI face swap tool!

Hot Article

Hot Tools

SublimeText3 Linux new version
SublimeText3 Linux latest version

SAP NetWeaver Server Adapter for Eclipse
Integrate Eclipse with SAP NetWeaver application server.

SublimeText3 English version
Recommended: Win version, supports code prompts!

PhpStorm Mac version
The latest (2018.2.1) professional PHP integrated development tool
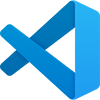
VSCode Windows 64-bit Download
A free and powerful IDE editor launched by Microsoft
