This tutorial explores DeepChecks for data validation and machine learning model testing, and leverages GitHub Actions for automated testing and artifact creation. We'll cover machine learning testing principles, DeepChecks functionality, and a complete automated workflow.
Image by Author
Understanding Machine Learning Testing
Effective machine learning requires rigorous testing beyond simple accuracy metrics. We must assess fairness, robustness, and ethical considerations, including bias detection, false positives/negatives, performance metrics, throughput, and alignment with AI ethics. This involves techniques like data validation, cross-validation, F1-score calculation, confusion matrix analysis, and drift detection (data and prediction). Data splitting (train/test/validation) is crucial for reliable model evaluation. Automating this process is key to building dependable AI systems.
For beginners, the Machine Learning Fundamentals with Python skill track provides a solid foundation.
DeepChecks, an open-source Python library, simplifies comprehensive machine learning testing. It offers built-in checks for model performance, data integrity, and distribution, supporting continuous validation for reliable model deployment.
Getting Started with DeepChecks
Install DeepChecks using pip:
pip install deepchecks --upgrade -q
Data Loading and Preparation (Loan Dataset)
We'll use the Loan Data dataset from DataCamp.
import pandas as pd loan_data = pd.read_csv("loan_data.csv") loan_data.head()
Create a DeepChecks dataset:
from sklearn.model_selection import train_test_split from deepchecks.tabular import Dataset label_col = 'not.fully.paid' deep_loan_data = Dataset(loan_data, label=label_col, cat_features=["purpose"])
Data Integrity Testing
DeepChecks' data integrity suite performs automated checks.
from deepchecks.tabular.suites import data_integrity integ_suite = data_integrity() suite_result = integ_suite.run(deep_loan_data) suite_result.show_in_iframe() # Use show_in_iframe for DataLab compatibility
This generates a report covering: Feature-Label Correlation, Feature-Feature Correlation, Single Value Checks, Special Character Detection, Null Value Analysis, Data Type Consistency, String Mismatches, Duplicate Detection, String Length Validation, Conflicting Labels, and Outlier Detection.
Save the report:
suite_result.save_as_html()
Individual Test Execution
For efficiency, run individual tests:
from deepchecks.tabular.checks import IsSingleValue, DataDuplicates result = IsSingleValue().run(deep_loan_data) print(result.value) # Unique value counts per column result = DataDuplicates().run(deep_loan_data) print(result.value) # Duplicate sample count
Model Evaluation with DeepChecks
We'll train an ensemble model (Logistic Regression, Random Forest, Gaussian Naive Bayes) and evaluate it using DeepChecks.
pip install deepchecks --upgrade -q
The model evaluation report includes: ROC curves, weak segment performance, unused feature detection, train-test performance comparison, prediction drift analysis, simple model comparisons, model inference time, confusion matrices, and more.
JSON output:
import pandas as pd loan_data = pd.read_csv("loan_data.csv") loan_data.head()
Individual test example (Label Drift):
from sklearn.model_selection import train_test_split from deepchecks.tabular import Dataset label_col = 'not.fully.paid' deep_loan_data = Dataset(loan_data, label=label_col, cat_features=["purpose"])
Automating with GitHub Actions
This section details setting up a GitHub Actions workflow to automate data validation and model testing. The process involves creating a repository, adding data and Python scripts (data_validation.py
, train_validation.py
), and configuring a GitHub Actions workflow (main.yml
) to execute these scripts and save the results as artifacts. Detailed steps and code snippets are provided in the original input. Refer to the kingabzpro/Automating-Machine-Learning-Testing
repository for a complete example. The workflow utilizes the actions/checkout
, actions/setup-python
, and actions/upload-artifact
actions.
Conclusion
Automating machine learning testing using DeepChecks and GitHub Actions significantly improves efficiency and reliability. Early detection of issues enhances model accuracy and fairness. This tutorial provides a practical guide to implementing this workflow, enabling developers to build more robust and trustworthy AI systems. Consider the Machine Learning Scientist with Python career track for further development in this field.
The above is the detailed content of DeepChecks Tutorial: Automating Machine Learning Testing. For more information, please follow other related articles on the PHP Chinese website!
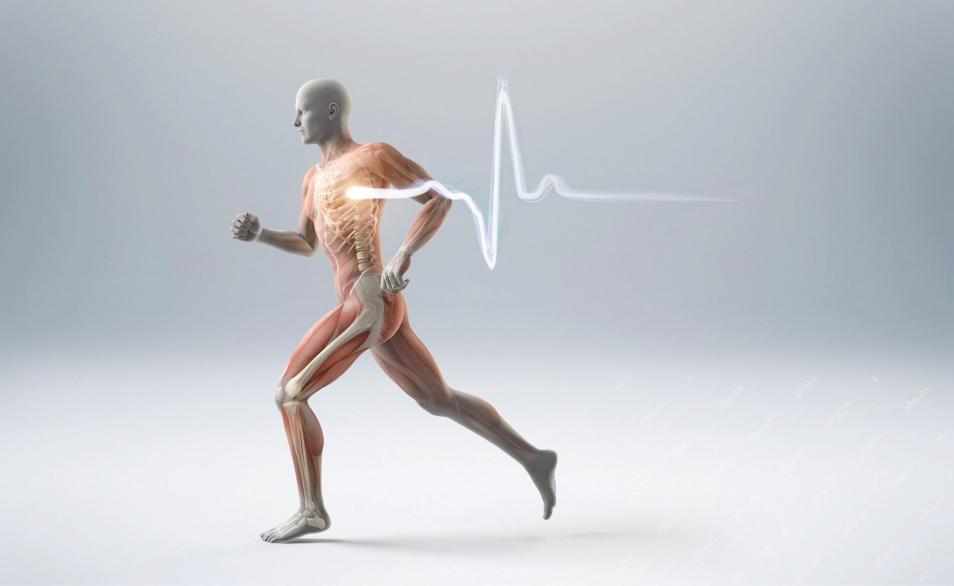
Scientists have extensively studied human and simpler neural networks (like those in C. elegans) to understand their functionality. However, a crucial question arises: how do we adapt our own neural networks to work effectively alongside novel AI s
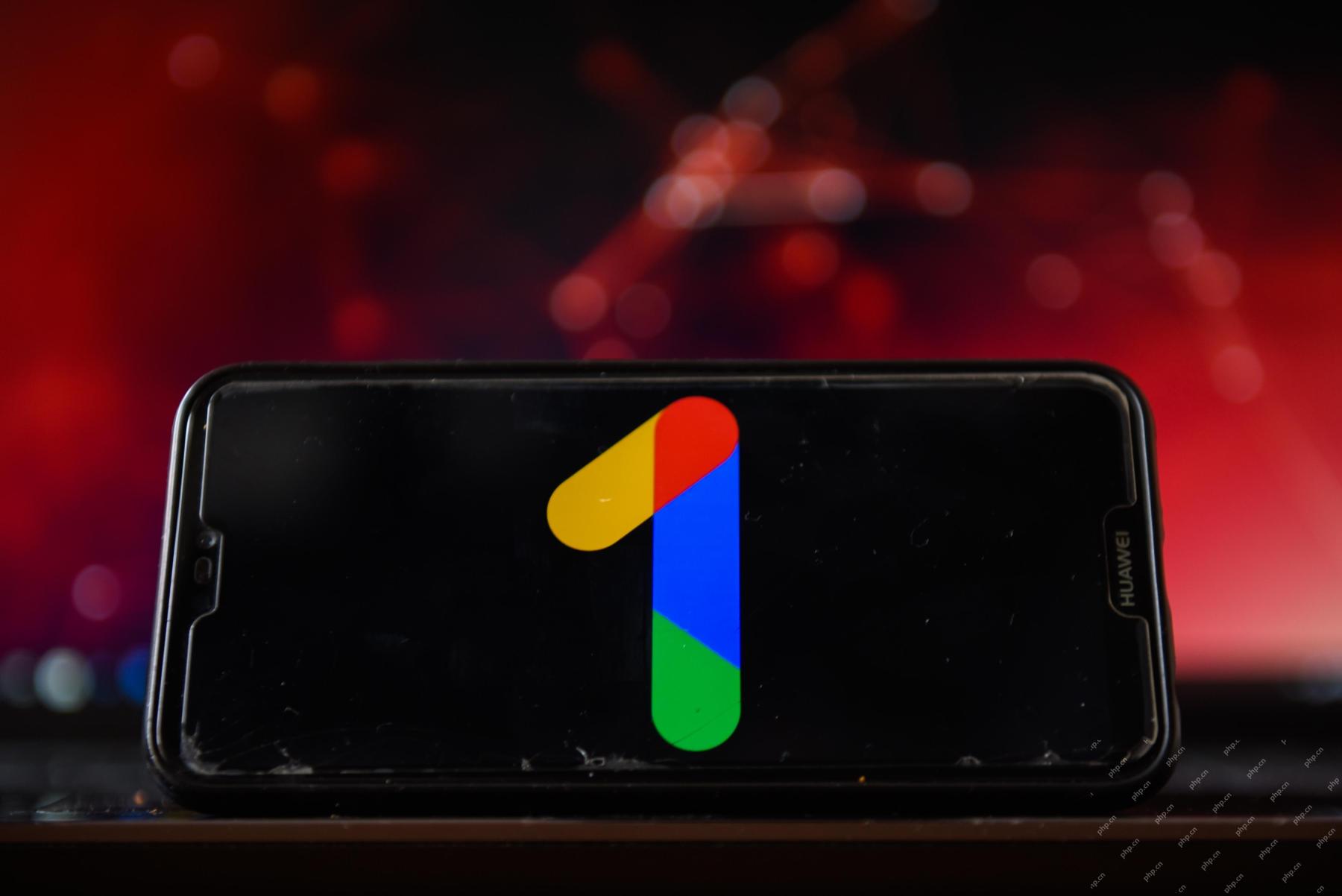
Google's Gemini Advanced: New Subscription Tiers on the Horizon Currently, accessing Gemini Advanced requires a $19.99/month Google One AI Premium plan. However, an Android Authority report hints at upcoming changes. Code within the latest Google P
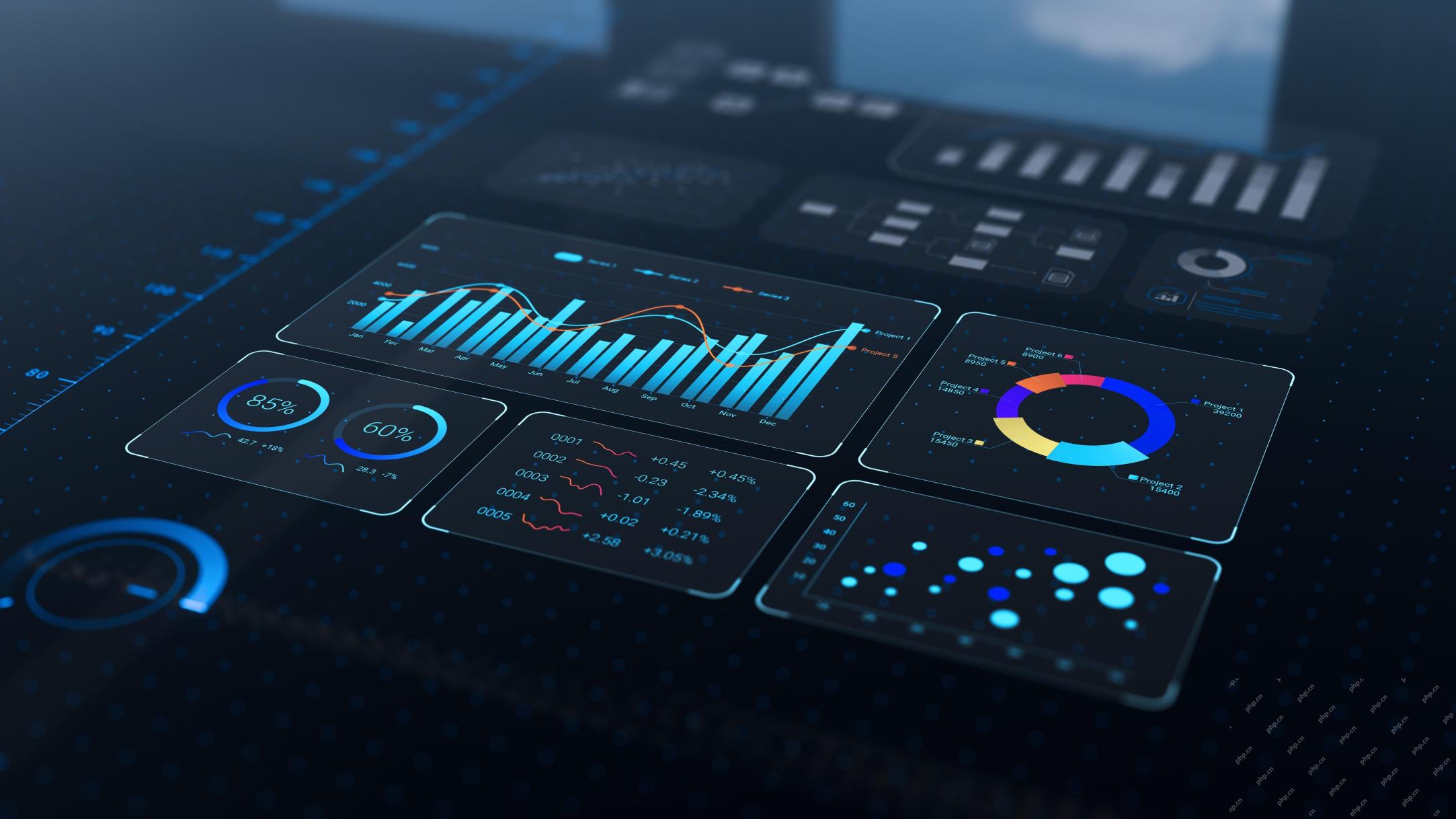
Despite the hype surrounding advanced AI capabilities, a significant challenge lurks within enterprise AI deployments: data processing bottlenecks. While CEOs celebrate AI advancements, engineers grapple with slow query times, overloaded pipelines, a

Handling documents is no longer just about opening files in your AI projects, it’s about transforming chaos into clarity. Docs such as PDFs, PowerPoints, and Word flood our workflows in every shape and size. Retrieving structured
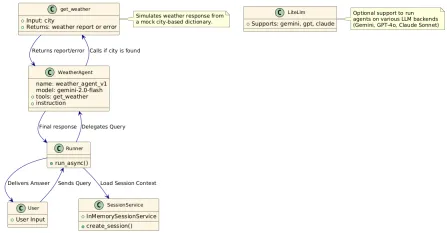
Harness the power of Google's Agent Development Kit (ADK) to create intelligent agents with real-world capabilities! This tutorial guides you through building conversational agents using ADK, supporting various language models like Gemini and GPT. W
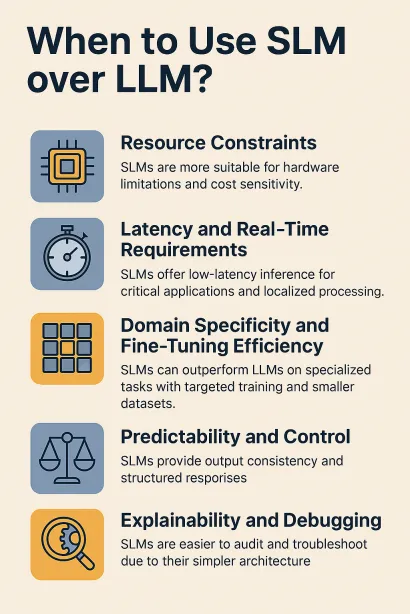
summary: Small Language Model (SLM) is designed for efficiency. They are better than the Large Language Model (LLM) in resource-deficient, real-time and privacy-sensitive environments. Best for focus-based tasks, especially where domain specificity, controllability, and interpretability are more important than general knowledge or creativity. SLMs are not a replacement for LLMs, but they are ideal when precision, speed and cost-effectiveness are critical. Technology helps us achieve more with fewer resources. It has always been a promoter, not a driver. From the steam engine era to the Internet bubble era, the power of technology lies in the extent to which it helps us solve problems. Artificial intelligence (AI) and more recently generative AI are no exception
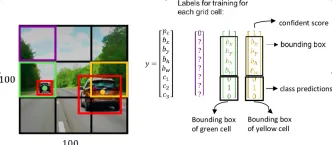
Harness the Power of Google Gemini for Computer Vision: A Comprehensive Guide Google Gemini, a leading AI chatbot, extends its capabilities beyond conversation to encompass powerful computer vision functionalities. This guide details how to utilize
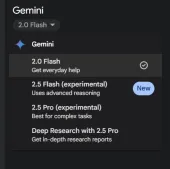
The AI landscape of 2025 is electrifying with the arrival of Google's Gemini 2.0 Flash and OpenAI's o4-mini. These cutting-edge models, launched weeks apart, boast comparable advanced features and impressive benchmark scores. This in-depth compariso


Hot AI Tools

Undresser.AI Undress
AI-powered app for creating realistic nude photos

AI Clothes Remover
Online AI tool for removing clothes from photos.

Undress AI Tool
Undress images for free

Clothoff.io
AI clothes remover

Video Face Swap
Swap faces in any video effortlessly with our completely free AI face swap tool!

Hot Article

Hot Tools

SublimeText3 English version
Recommended: Win version, supports code prompts!

PhpStorm Mac version
The latest (2018.2.1) professional PHP integrated development tool

SecLists
SecLists is the ultimate security tester's companion. It is a collection of various types of lists that are frequently used during security assessments, all in one place. SecLists helps make security testing more efficient and productive by conveniently providing all the lists a security tester might need. List types include usernames, passwords, URLs, fuzzing payloads, sensitive data patterns, web shells, and more. The tester can simply pull this repository onto a new test machine and he will have access to every type of list he needs.

Notepad++7.3.1
Easy-to-use and free code editor

Atom editor mac version download
The most popular open source editor
