As part of #OpenSourceWeek Day 4, DeepSeek introduces 2 new tools to make deep learning faster and more efficient: DualPipe and EPLB. These tools help improve how computers handle calculations and communication during training, making the process smoother and quicker. In the fast-changing world of deep learning, finding ways to train models better while using fewer resources is key. DualPipe and EPLB are big steps forward in solving these challenges. This article explains how these tools work and how they can make a difference in deep learning.
? Day 4 of #OpenSourceWeek: Optimized Parallelism Strategies
— DeepSeek (@deepseek_ai) February 27, 2025
✅ DualPipe – a bidirectional pipeline parallelism algorithm for computation-communication overlap in V3/R1 training.
? https://t.co/GBtxSvWLT4
✅ EPLB – an expert-parallel load balancer for V3/R1.
?…
This release marks Day 4 of our Open Source Week celebrations, following the successful launches of FlashML on Day 1, DeepEP on Day 2, and DeepGEMM on Day 3.
Table of contents
- Understanding Pipeline Parallelism
- DualPipe: Bidirectional Pipeline Parallelism
- Key Features
- Technical Details
- EPLB: Expert-Parallel Load Balancer
- Key Features
- Technical Details
- Hierarchical Load Balancing
- Global Load Balancing
- Profiling Data: Analyzing Computation-Communication Overlap
- Key Features
- Training Profiling data
- Real-World Applications
- Future Directions
- Conclusion
Understanding Pipeline Parallelism
Pipeline parallelism is an approach that facilitates the concurrent processing of various segments of a model’s training sequence. By partitioning the model and handling multiple inputs at once, pipeline parallelism can markedly abbreviate the training period. Yet, traditional pipeline methodologies are prone to inefficiencies, including idle intervals or “bubbles,” that impair performance. Innovations like DualPipe are introduced to ameliorate these inefficiencies and augment overall efficiency.
Within deep learning, the expression “bubbles in a pipeline” characterizes intervals of inactivity on GPUs during pipeline parallel training, where a segment of the pipeline is stalled, pending data from an antecedent segment. This generates a “gap” or “bubble” in the computational progression, culminating in inefficient GPU resource management.
DualPipe: Bidirectional Pipeline Parallelism
DualPipe is a sophisticated bidirectional pipeline parallelism algorithm that aims to maximize the overlap between forward and backward computation-communication phases. This approach is particularly beneficial in reducing pipeline bubbles, which can significantly hinder training efficiency.
Key Features
- Full Overlap: Achieves complete overlap of forward and backward phases, ensuring that resources are utilized effectively.
- Reduced Pipeline Bubbles: Minimizes idle time during training, leading to enhanced resource utilization and faster training times.
Technical Details
The algorithm’s performance can be illustrated through a scheduling example involving 8 PP ranks and 20 micro-batches. The micro-batches in the reverse direction are symmetric to those in the forward direction, simplifying the illustration.
|
Bubble | Parameter | Activation | ||||||||||||||||
1F1B | (PP-1)(? ?) | 1× | PP | ||||||||||||||||
ZB1P | (PP-1)(? ?-2?) | 1× | PP | ||||||||||||||||
DualPipe | (PP/2-1)(?&? ?-3?) | 2× | PP 1 |
Where:
- ?: Execution time of a forward chunk
- ?: Execution time of a full backward chunk
- ?: Execution time of a “backward for weights” chunk
- ?&?: Execution time of two mutually overlapped forward and backward chunks
Example DualPipe scheduling configuration for 8 PP (Pipeline Parallelism) ranks and 20 micro-batches, with a focus on two directions. The micro-batches processed in the reverse direction mirror those in the forward direction, allowing us to omit their batch identifiers for the sake of simplifying the illustration. Two cells that share a common black border are involved in overlapping computation and communication tasks.
For more information visit DualPipe Github Repository
EPLB: Expert-Parallel Load Balancer
EPLB, or Expert-Parallel Load Balancer, optimizes load balancing in V3/R1 training. It efficiently distributes workloads across multiple processing units, boosting overall performance.
Key Features
- Expert Parallelism: Utilizes expert models to balance the load effectively, ensuring that each processing unit is utilized to its full potential.
- Dynamic Load Balancing: Adapts to varying workloads during training, allowing for real-time adjustments to maintain optimal performance.
Technical Details
EPLB (Efficient Pipeline Load Distribution) aims at the judicious assignment of tasks to accessible resources to diminish idle intervals and enhance throughput. This methodology is of heightened significance in contexts where varying models or tasks necessitate distinct levels of computational power.
The load balancing algorithm employs two distinct policies, tailored to varying circumstances:
Hierarchical Load Balancing
The hierarchical load balancing policy activates when the number of server nodes divides evenly into the expert group count. This strategy leverages group-limited expert routing by initially organizing expert groups onto nodes in a manner that promotes balanced load distribution. Subsequently, expert replication occurs within each node to maintain load equilibrium. Ultimately, these replicated experts are assigned to individual GPUs, thereby achieving load balance across different GPUs. The hierarchical load balancing policy is particularly suited for the prefilling stage when dealing with smaller expert-parallel sizes.
Global Load Balancing
Conversely, when the server nodes’ count does not divide the expert groups, the global load balancing policy is implemented. This approach involves the global replication of experts, irrespective of their grouping within expert groups. Following replication, the experts are evenly distributed to individual GPUs, ensuring load balance is maintained across the GPUs. The global load balancing policy is applicable in the decoding stage when handling larger expert-parallel sizes.
Example Code:
import torch import eplb weight = torch.tensor([[ 90, 132, 40, 61, 104, 165, 39, 4, 73, 56, 183, 86], [ 20, 107, 104, 64, 19, 197, 187, 157, 172, 86, 16, 27]]) num_replicas = 16 num_groups = 4 num_nodes = 2 num_gpus = 8 phy2log, log2phy, logcnt = eplb.rebalance_experts(weight, num_replicas, num_groups, num_nodes, num_gpus) print(phy2log)
Output:
tensor([[ 5, 6, 5, 7, 8, 4, 3, 4, 10, 9, 10, 2, 0, 1, 11, 1], [ 7, 10, 6, 8, 6, 11, 8, 9, 2, 4, 5, 1, 5, 0, 3, 1]])
The visual representation illustrates a dual-tiered Configuration of Mixture of Experts (MoE), with each tier comprising 12 specialized experts. To boost the model’s robustness and create backup mechanisms, we introduce an extra 4 experts in each tier. This modification leads to a cumulative total of 16 experts per tier serving as backups. The system replicates and distributes these experts across 2 computational nodes, with each node containing 4 GPUs. It applies the hierarchical load balancing policy and demonstrates the strategic replication and allocation of experts according to the plan.
For detailed implementation instructions, refer to the EPLB GitHub repository.
Profiling Data: Analyzing Computation-Communication Overlap
To effectively analyze the computation-communication overlap in V3/R1, the profiling data provides essential insights. The bottlenecks of the performance and the optimization of training process can be understood using this data.
Key Features
- Comprehensive Analysis: This approach provides an extensive evaluation of computation and communication phases, facilitating a deep understanding of system performance metrics.
- Performance Insights: It pinpoints opportunities for enhancing training efficiency, equipping developers with critical information to guide optimization efforts.
Training Profiling data
The training profile data illustrates the strategy for overlapping individual forward and backward chunks within DualPipe. Each chunk incorporates 4 layers of Mixture of Experts (MoE). The parallel configuration matches the settings used in DeepSeek-V3 pretraining, specifically using EP64 (Epoch 64) and TP1 (Temporal Padding with 1 token) configurations, with a sequence length of 4K. To keep things simple, we exclude PP (Pipeline Parallelism) communication during profiling.
For more information and to access the profiling data, visit the Profiling Data GitHub repository.
Real-World Applications
The practical application of DualPipe and EPLB has demonstrated encouraging outcomes across diverse fields such as natural language processing, computer vision, and reinforcement learning. By refining the training process, these methodologies facilitate expedited model convergence and heightened precision, proving to be indispensable instruments for both researchers and practitioners.
Future Directions
As the field of deep learning progresses, the demand for more efficient training methodologies will likely escalate. Future investigations may concentrate on amplifying the effectiveness of DualPipe and EPLB, possibly by investigating hybrid models that amalgamate the advantages of both. Moreover, the integration of these strategies with cutting-edge technologies, including quantum computing, might pave novel pathways for optimization.
Conclusion
The progress in parallelism strategies via DualPipe and EPLB marks considerable strides in refining deep learning training procedures. By harnessing these algorithms, both researchers and practitioners can attain superior resource utilization and accelerated training durations, culminating in more efficient model creation. The assimilation of profiling data augments the capacity to calibrate these processes, guaranteeing that deep learning’s trajectory of rapid advancement persists.
The above is the detailed content of Optimized Parallelism Strategies Released by DeepSeek. For more information, please follow other related articles on the PHP Chinese website!
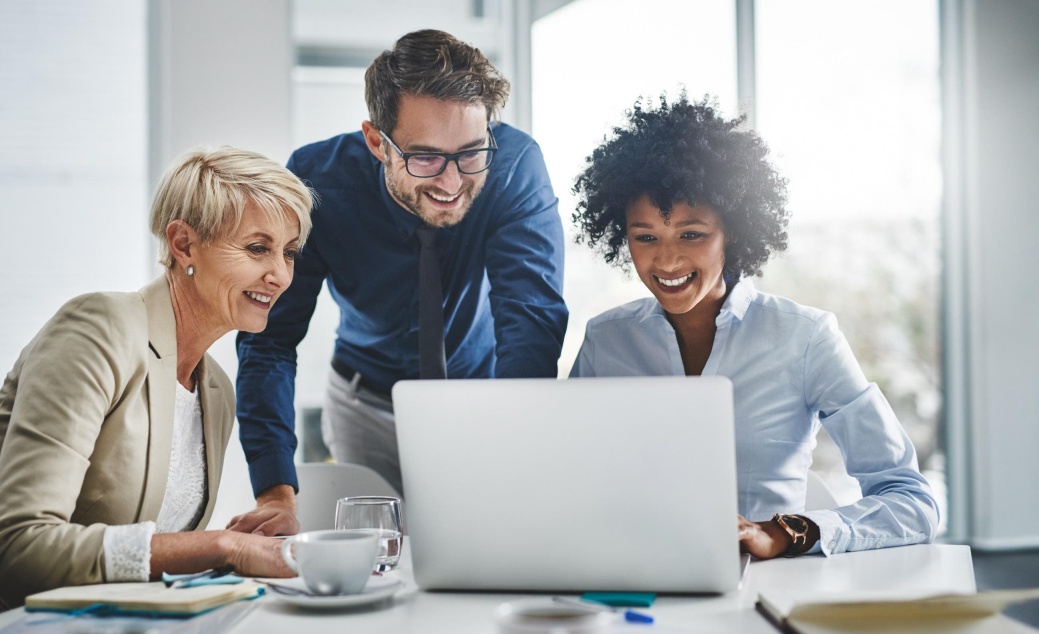
For those of you who might be new to my column, I broadly explore the latest advances in AI across the board, including topics such as embodied AI, AI reasoning, high-tech breakthroughs in AI, prompt engineering, training of AI, fielding of AI, AI re
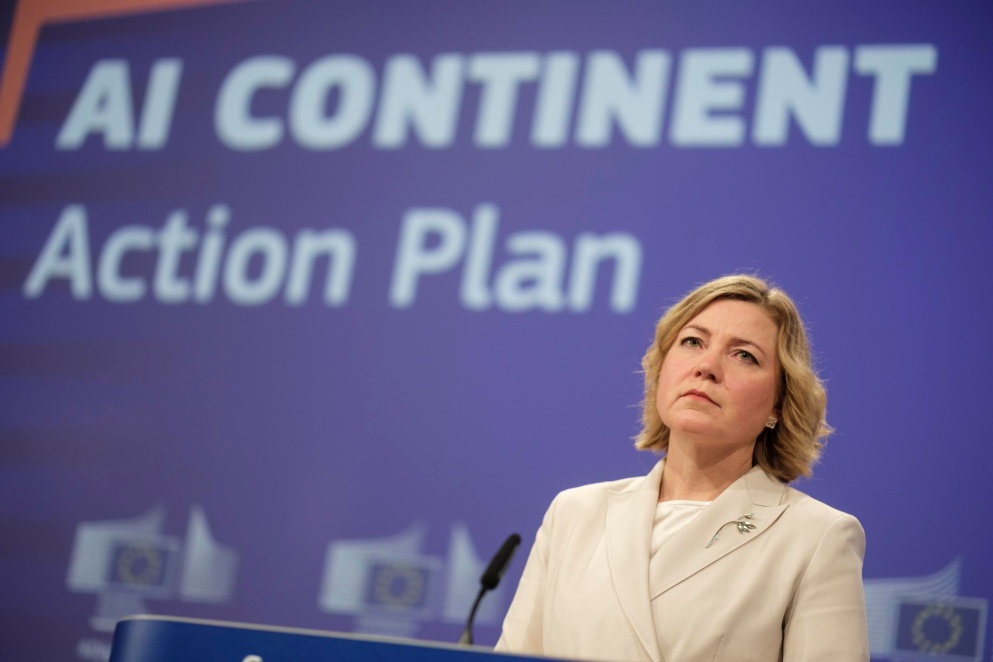
Europe's ambitious AI Continent Action Plan aims to establish the EU as a global leader in artificial intelligence. A key element is the creation of a network of AI gigafactories, each housing around 100,000 advanced AI chips – four times the capaci
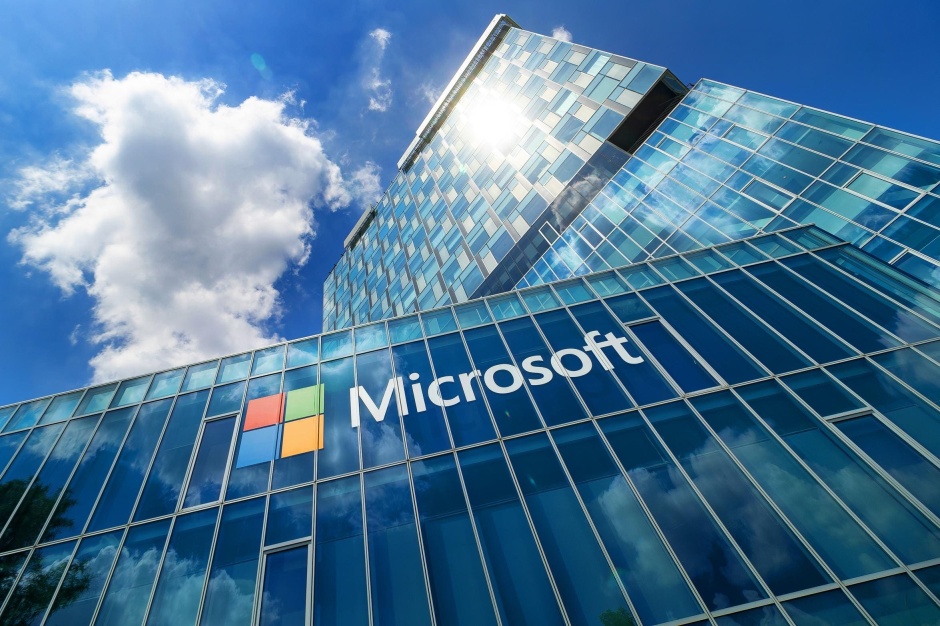
Microsoft's Unified Approach to AI Agent Applications: A Clear Win for Businesses Microsoft's recent announcement regarding new AI agent capabilities impressed with its clear and unified presentation. Unlike many tech announcements bogged down in te
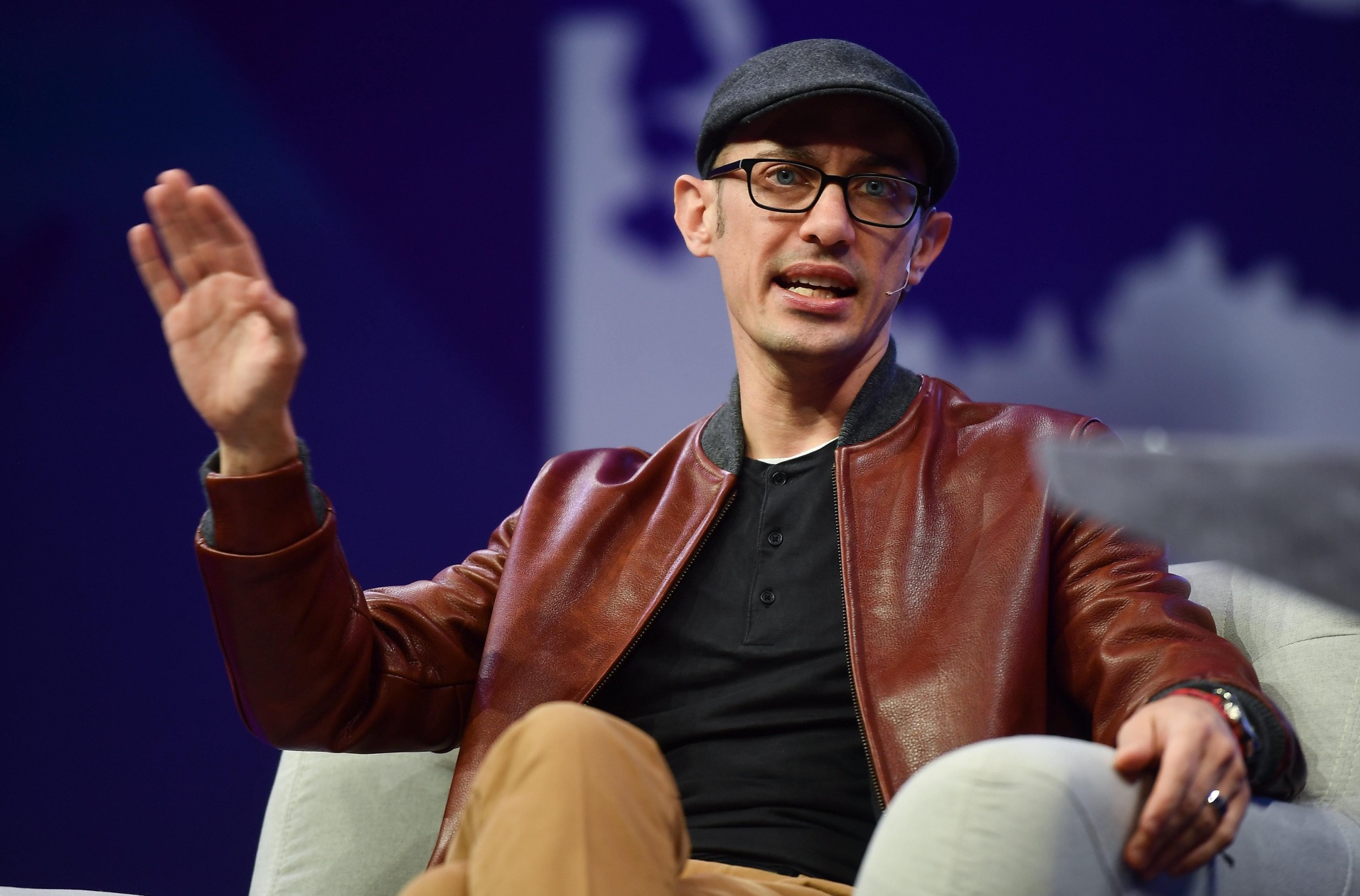
Shopify CEO Tobi Lütke's recent memo boldly declares AI proficiency a fundamental expectation for every employee, marking a significant cultural shift within the company. This isn't a fleeting trend; it's a new operational paradigm integrated into p
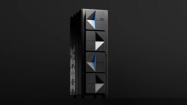
IBM's z17 Mainframe: Integrating AI for Enhanced Business Operations Last month, at IBM's New York headquarters, I received a preview of the z17's capabilities. Building on the z16's success (launched in 2022 and demonstrating sustained revenue grow
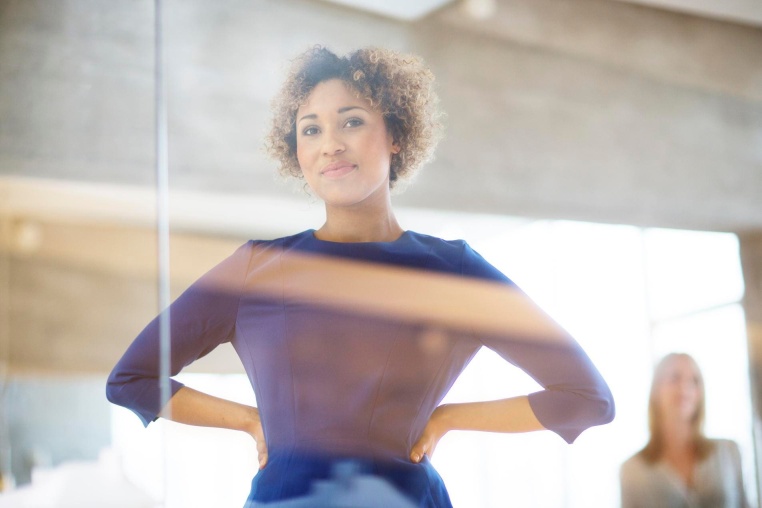
Unlock unshakeable confidence and eliminate the need for external validation! These five ChatGPT prompts will guide you towards complete self-reliance and a transformative shift in self-perception. Simply copy, paste, and customize the bracketed in
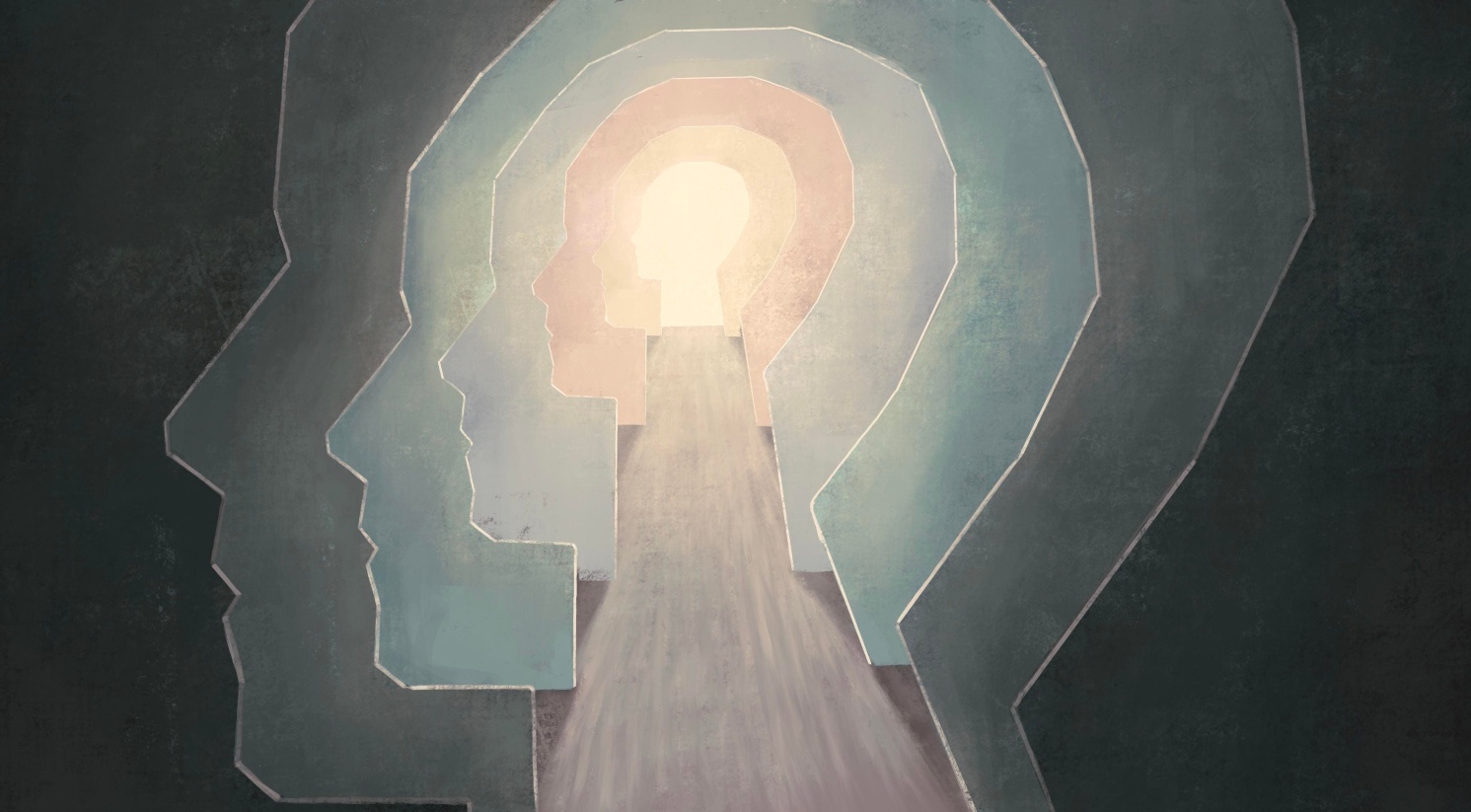
A recent [study] by Anthropic, an artificial intelligence security and research company, begins to reveal the truth about these complex processes, showing a complexity that is disturbingly similar to our own cognitive domain. Natural intelligence and artificial intelligence may be more similar than we think. Snooping inside: Anthropic Interpretability Study The new findings from the research conducted by Anthropic represent significant advances in the field of mechanistic interpretability, which aims to reverse engineer internal computing of AI—not just observe what AI does, but understand how it does it at the artificial neuron level. Imagine trying to understand the brain by drawing which neurons fire when someone sees a specific object or thinks about a specific idea. A
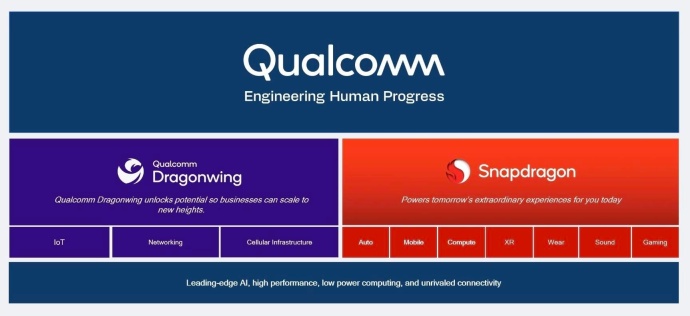
Qualcomm's Dragonwing: A Strategic Leap into Enterprise and Infrastructure Qualcomm is aggressively expanding its reach beyond mobile, targeting enterprise and infrastructure markets globally with its new Dragonwing brand. This isn't merely a rebran


Hot AI Tools

Undresser.AI Undress
AI-powered app for creating realistic nude photos

AI Clothes Remover
Online AI tool for removing clothes from photos.

Undress AI Tool
Undress images for free

Clothoff.io
AI clothes remover

AI Hentai Generator
Generate AI Hentai for free.

Hot Article

Hot Tools

MantisBT
Mantis is an easy-to-deploy web-based defect tracking tool designed to aid in product defect tracking. It requires PHP, MySQL and a web server. Check out our demo and hosting services.
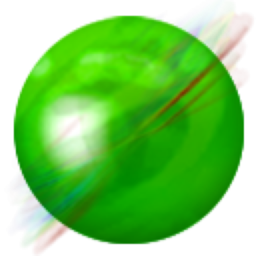
ZendStudio 13.5.1 Mac
Powerful PHP integrated development environment

SublimeText3 Chinese version
Chinese version, very easy to use

PhpStorm Mac version
The latest (2018.2.1) professional PHP integrated development tool

SecLists
SecLists is the ultimate security tester's companion. It is a collection of various types of lists that are frequently used during security assessments, all in one place. SecLists helps make security testing more efficient and productive by conveniently providing all the lists a security tester might need. List types include usernames, passwords, URLs, fuzzing payloads, sensitive data patterns, web shells, and more. The tester can simply pull this repository onto a new test machine and he will have access to every type of list he needs.