DeepSeek's groundbreaking open-source release: FlashMLA, a CUDA kernel accelerating LLMs. This optimized multi-latent attention (MLA) decoding kernel, specifically designed for Hopper GPUs, significantly boosts the speed and efficiency of AI model hosting. Key improvements include BF16 support and a paged KV cache (64-block size), resulting in impressive performance benchmarks.
? Day 1 of #OpenSourceWeek: FlashMLA
DeepSeek proudly unveils FlashMLA, a high-efficiency MLA decoding kernel for Hopper GPUs. Optimized for variable-length sequences and now in production.
✅ BF16 support
✅ Paged KV cache (block size 64)
⚡ 3000 GB/s memory-bound & 580 TFLOPS…— DeepSeek (@deepseek_ai) February 24, 2025
Key Features:
- BF16 Precision: Enables efficient computation while maintaining numerical stability.
- Paged KV Cache (64-block size): Enhances memory efficiency and reduces latency, especially crucial for large models.
These optimizations achieve up to 3000 GB/s memory bandwidth and 580 TFLOPS in computation-bound scenarios on H800 SXM5 GPUs using CUDA 12.6. This dramatically improves AI inference performance. Previously used in DeepSeek Models, FlashMLA now accelerates DeepSeek AI's R1 V3.
Table of Contents:
- What is FlashMLA?
- Understanding Multi-head Latent Attention (MLA)
- Standard Multi-Head Attention Limitations
- MLA's Memory Optimization Strategy
- Key-Value Caching and Autoregressive Decoding
- KV Caching Mechanics
- Addressing Memory Challenges
- FlashMLA's Role in DeepSeek Models
- NVIDIA Hopper Architecture
- Performance Analysis and Implications
- Conclusion
What is FlashMLA?
FlashMLA is a highly optimized MLA decoding kernel built for NVIDIA Hopper GPUs. Its design prioritizes speed and efficiency, reflecting DeepSeek's commitment to scalable AI model acceleration.
Hardware and Software Requirements:
- Hopper architecture GPUs (e.g., H800 SXM5)
- CUDA 12.3
- PyTorch 2.0
Performance Benchmarks:
FlashMLA demonstrates exceptional performance:
- Memory Bandwidth: Up to 3000 GB/s (approaching the H800 SXM5 theoretical peak).
- Computational Throughput: Up to 580 TFLOPS for BF16 matrix multiplication (significantly exceeding the H800's theoretical peak).
This superior performance makes FlashMLA ideal for demanding AI workloads.
Understanding Multi-head Latent Attention (MLA)
MLA, introduced with DeepSeek-V2, addresses the memory limitations of standard multi-head attention (MHA) by using a low-rank factorized projection matrix. Unlike methods like Group-Query Attention, MLA improves performance while reducing memory overhead.
Standard Multi-Head Attention Limitations:
MHA's KV cache scales linearly with sequence length, creating a memory bottleneck for long sequences. The cache size is calculated as: seq_len * n_h * d_h
(where n_h
is the number of attention heads and d_h
is the head dimension).
MLA's Memory Optimization:
MLA compresses keys and values into a smaller latent vector (c_t
), reducing the KV cache size to seq_len * d_c
(where d_c
is the latent vector dimension). This significantly reduces memory usage (up to 93.3% reduction in DeepSeek-V2).
Key-Value Caching and Autoregressive Decoding
KV caching accelerates autoregressive decoding by reusing previously computed key-value pairs. However, this increases memory usage.
Addressing Memory Challenges:
Techniques like Multi-Query Attention (MQA) and Grouped-Query Attention (GQA) mitigate the memory issues associated with KV caching.
FlashMLA's Role in DeepSeek Models:
FlashMLA powers DeepSeek's R1 and V3 models, enabling efficient large-scale AI applications.
NVIDIA Hopper Architecture
NVIDIA Hopper is a high-performance GPU architecture designed for AI and HPC workloads. Its innovations, such as the Transformer Engine and second-generation MIG, enable exceptional speed and scalability.
Performance Analysis and Implications
FlashMLA achieves 580 TFLOPS for BF16 matrix multiplication, more than double the H800 GPU's theoretical peak. This demonstrates highly efficient utilization of GPU resources.
Conclusion
FlashMLA represents a major advancement in AI inference efficiency, particularly for Hopper GPUs. Its MLA optimization, combined with BF16 support and paged KV caching, delivers remarkable performance improvements. This makes large-scale AI models more accessible and cost-effective, setting a new benchmark for model efficiency.
The above is the detailed content of DeepSeek Launches FlashMLA. For more information, please follow other related articles on the PHP Chinese website!
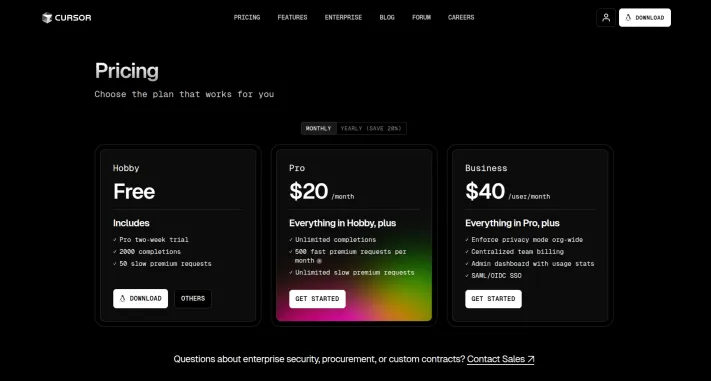
Vibe coding is reshaping the world of software development by letting us create applications using natural language instead of endless lines of code. Inspired by visionaries like Andrej Karpathy, this innovative approach lets dev

DALL-E 3: A Generative AI Image Creation Tool Generative AI is revolutionizing content creation, and DALL-E 3, OpenAI's latest image generation model, is at the forefront. Released in October 2023, it builds upon its predecessors, DALL-E and DALL-E 2
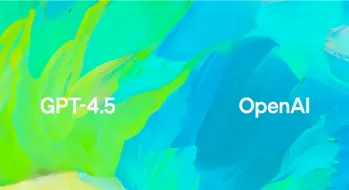
February 2025 has been yet another game-changing month for generative AI, bringing us some of the most anticipated model upgrades and groundbreaking new features. From xAI’s Grok 3 and Anthropic’s Claude 3.7 Sonnet, to OpenAI’s G
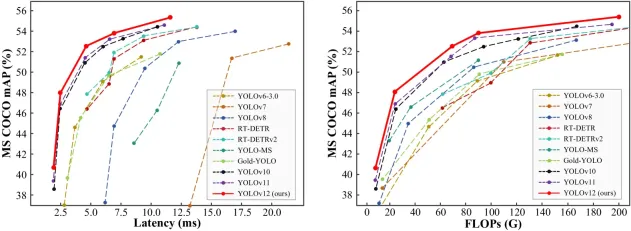
YOLO (You Only Look Once) has been a leading real-time object detection framework, with each iteration improving upon the previous versions. The latest version YOLO v12 introduces advancements that significantly enhance accuracy
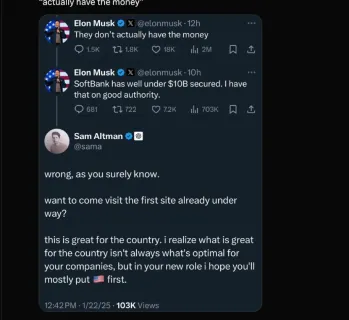
The $500 billion Stargate AI project, backed by tech giants like OpenAI, SoftBank, Oracle, and Nvidia, and supported by the U.S. government, aims to solidify American AI leadership. This ambitious undertaking promises a future shaped by AI advanceme
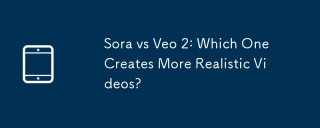
Google's Veo 2 and OpenAI's Sora: Which AI video generator reigns supreme? Both platforms generate impressive AI videos, but their strengths lie in different areas. This comparison, using various prompts, reveals which tool best suits your needs. T
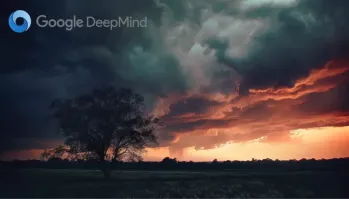
Google DeepMind's GenCast: A Revolutionary AI for Weather Forecasting Weather forecasting has undergone a dramatic transformation, moving from rudimentary observations to sophisticated AI-powered predictions. Google DeepMind's GenCast, a groundbreak
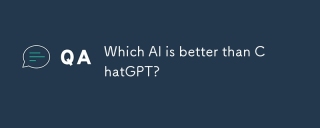
The article discusses AI models surpassing ChatGPT, like LaMDA, LLaMA, and Grok, highlighting their advantages in accuracy, understanding, and industry impact.(159 characters)


Hot AI Tools

Undresser.AI Undress
AI-powered app for creating realistic nude photos

AI Clothes Remover
Online AI tool for removing clothes from photos.

Undress AI Tool
Undress images for free

Clothoff.io
AI clothes remover

AI Hentai Generator
Generate AI Hentai for free.

Hot Article

Hot Tools

SublimeText3 English version
Recommended: Win version, supports code prompts!

Zend Studio 13.0.1
Powerful PHP integrated development environment

Atom editor mac version download
The most popular open source editor

MinGW - Minimalist GNU for Windows
This project is in the process of being migrated to osdn.net/projects/mingw, you can continue to follow us there. MinGW: A native Windows port of the GNU Compiler Collection (GCC), freely distributable import libraries and header files for building native Windows applications; includes extensions to the MSVC runtime to support C99 functionality. All MinGW software can run on 64-bit Windows platforms.
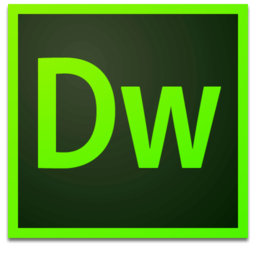
Dreamweaver Mac version
Visual web development tools