


Is Django paging query inefficient? How to avoid full-table scanning of databases?
Django Pagination Query Efficiency is Low? How to Avoid Full Table Scans?
Django's built-in pagination, while convenient, can lead to performance issues with large datasets if not implemented carefully. The primary culprit is the potential for full table scans. When you use Paginator
with a queryset that hasn't been optimized, Django might fetch all rows from the database before slicing them into pages. This is inefficient and drastically slows down the response time, especially with millions of records. To avoid full table scans, you must ensure that your database query only retrieves the necessary rows for the requested page. This involves using database-level pagination features, which means leveraging LIMIT
and OFFSET
clauses in your SQL query. Django's ORM provides ways to do this, most effectively through QuerySet.offset()
and QuerySet.limit()
, or by directly using raw SQL queries with appropriate LIMIT
and OFFSET
clauses if needed for complex scenarios. Properly indexed database columns are also crucial; without them, even limited queries can still be slow. Ensure you have indexes on columns frequently used in WHERE
clauses of your pagination queries.
What are the common causes of slow pagination in Django?
Several factors contribute to slow pagination in Django applications:
- Lack of Database Indexing: Without proper indexes on relevant columns, the database must perform a full table scan to locate the desired rows for each page. This is extremely slow for large datasets.
-
Inefficient QuerySets: Using
QuerySet
methods that force the evaluation of the entire queryset before pagination (e.g., iterating through the entire queryset before applying pagination) defeats the purpose of pagination and leads to performance bottlenecks. - N 1 Problem: If your pagination involves related models and you're not using prefetching or select_related, you'll end up making numerous additional database queries for each object on a page (one query per object to retrieve related data).
- Unoptimized Database Queries: Complex or poorly written queries that don't leverage database indexes effectively can significantly impact performance.
-
Improper Use of
Paginator
: UsingPaginator
without considering the underlying database query can lead to fetching the entire dataset before applying pagination, which is highly inefficient. -
Lack of Database-Level Pagination: Relying solely on Python-side pagination without using
LIMIT
andOFFSET
in the database query will result in fetching all data from the database before slicing it, negating the performance benefits of pagination. - Heavy Data Transfer: Transferring large amounts of data from the database to the application server even after pagination can still cause delays if not handled efficiently.
How can I optimize my Django models and queries for efficient pagination?
Optimizing Django models and queries for efficient pagination involves a multi-pronged approach:
-
Database Indexing: Create indexes on columns frequently used in
WHERE
clauses of your pagination queries, especially those involved in ordering. -
Efficient QuerySets: Use
QuerySet.order_by()
to define the sorting order for your data. UtilizeQuerySet.select_related()
andQuerySet.prefetch_related()
to reduce database queries when dealing with related models. Avoid unnecessaryQuerySet
operations that force early evaluation of the queryset. -
Database-Level Pagination: Employ
QuerySet.offset()
andQuerySet.limit()
methods to leverage the database's built-in pagination capabilities usingLIMIT
andOFFSET
clauses in the generated SQL. This ensures only the necessary data is retrieved. -
Raw SQL (if necessary): For complex pagination scenarios, consider using raw SQL queries with
LIMIT
andOFFSET
for fine-grained control over the database interaction. - Model Optimization: Ensure your models are appropriately designed and normalized to avoid redundancy and improve query efficiency.
- Caching: Implement caching strategies (e.g., using Django's caching framework or a dedicated caching solution like Redis) to store frequently accessed paginated data.
What are the best practices for implementing efficient pagination in Django with large datasets?
For efficient pagination with large datasets in Django, follow these best practices:
-
Always use database-level pagination: Never fetch the entire dataset into memory before paginating. Always use
LIMIT
andOFFSET
to retrieve only the data needed for the current page. - Optimize database queries: Ensure your queries are efficient and use appropriate indexes. Profile your queries to identify bottlenecks.
- Use appropriate data structures: Avoid unnecessary data transformation or manipulation after retrieving data from the database.
- Implement caching: Cache frequently accessed pages to reduce database load.
-
Consider alternative pagination strategies: For extremely large datasets, explore alternative pagination techniques like cursor-based pagination, which avoids the issues associated with
OFFSET
for very large offsets. Cursor-based pagination uses a unique identifier to fetch the next page, making it more efficient for very large datasets. - Use asynchronous tasks: For complex pagination logic or computationally intensive operations, offload the work to asynchronous tasks (e.g., using Celery) to prevent blocking the main thread.
- Monitor performance: Regularly monitor your application's performance to identify and address any emerging pagination issues. Use profiling tools to pinpoint slow queries and optimize them accordingly.
The above is the detailed content of Is Django paging query inefficient? How to avoid full-table scanning of databases?. For more information, please follow other related articles on the PHP Chinese website!
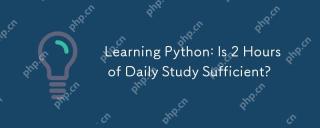
Is it enough to learn Python for two hours a day? It depends on your goals and learning methods. 1) Develop a clear learning plan, 2) Select appropriate learning resources and methods, 3) Practice and review and consolidate hands-on practice and review and consolidate, and you can gradually master the basic knowledge and advanced functions of Python during this period.
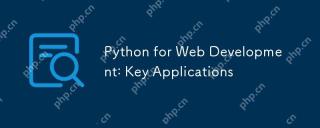
Key applications of Python in web development include the use of Django and Flask frameworks, API development, data analysis and visualization, machine learning and AI, and performance optimization. 1. Django and Flask framework: Django is suitable for rapid development of complex applications, and Flask is suitable for small or highly customized projects. 2. API development: Use Flask or DjangoRESTFramework to build RESTfulAPI. 3. Data analysis and visualization: Use Python to process data and display it through the web interface. 4. Machine Learning and AI: Python is used to build intelligent web applications. 5. Performance optimization: optimized through asynchronous programming, caching and code
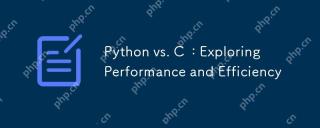
Python is better than C in development efficiency, but C is higher in execution performance. 1. Python's concise syntax and rich libraries improve development efficiency. 2.C's compilation-type characteristics and hardware control improve execution performance. When making a choice, you need to weigh the development speed and execution efficiency based on project needs.
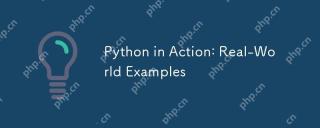
Python's real-world applications include data analytics, web development, artificial intelligence and automation. 1) In data analysis, Python uses Pandas and Matplotlib to process and visualize data. 2) In web development, Django and Flask frameworks simplify the creation of web applications. 3) In the field of artificial intelligence, TensorFlow and PyTorch are used to build and train models. 4) In terms of automation, Python scripts can be used for tasks such as copying files.
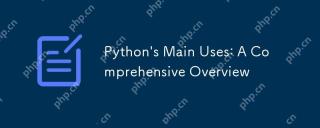
Python is widely used in data science, web development and automation scripting fields. 1) In data science, Python simplifies data processing and analysis through libraries such as NumPy and Pandas. 2) In web development, the Django and Flask frameworks enable developers to quickly build applications. 3) In automated scripts, Python's simplicity and standard library make it ideal.
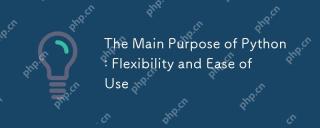
Python's flexibility is reflected in multi-paradigm support and dynamic type systems, while ease of use comes from a simple syntax and rich standard library. 1. Flexibility: Supports object-oriented, functional and procedural programming, and dynamic type systems improve development efficiency. 2. Ease of use: The grammar is close to natural language, the standard library covers a wide range of functions, and simplifies the development process.
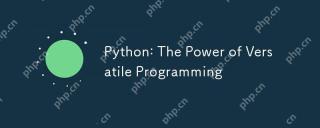
Python is highly favored for its simplicity and power, suitable for all needs from beginners to advanced developers. Its versatility is reflected in: 1) Easy to learn and use, simple syntax; 2) Rich libraries and frameworks, such as NumPy, Pandas, etc.; 3) Cross-platform support, which can be run on a variety of operating systems; 4) Suitable for scripting and automation tasks to improve work efficiency.
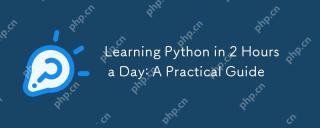
Yes, learn Python in two hours a day. 1. Develop a reasonable study plan, 2. Select the right learning resources, 3. Consolidate the knowledge learned through practice. These steps can help you master Python in a short time.


Hot AI Tools

Undresser.AI Undress
AI-powered app for creating realistic nude photos

AI Clothes Remover
Online AI tool for removing clothes from photos.

Undress AI Tool
Undress images for free

Clothoff.io
AI clothes remover

AI Hentai Generator
Generate AI Hentai for free.

Hot Article

Hot Tools

MinGW - Minimalist GNU for Windows
This project is in the process of being migrated to osdn.net/projects/mingw, you can continue to follow us there. MinGW: A native Windows port of the GNU Compiler Collection (GCC), freely distributable import libraries and header files for building native Windows applications; includes extensions to the MSVC runtime to support C99 functionality. All MinGW software can run on 64-bit Windows platforms.

DVWA
Damn Vulnerable Web App (DVWA) is a PHP/MySQL web application that is very vulnerable. Its main goals are to be an aid for security professionals to test their skills and tools in a legal environment, to help web developers better understand the process of securing web applications, and to help teachers/students teach/learn in a classroom environment Web application security. The goal of DVWA is to practice some of the most common web vulnerabilities through a simple and straightforward interface, with varying degrees of difficulty. Please note that this software

SecLists
SecLists is the ultimate security tester's companion. It is a collection of various types of lists that are frequently used during security assessments, all in one place. SecLists helps make security testing more efficient and productive by conveniently providing all the lists a security tester might need. List types include usernames, passwords, URLs, fuzzing payloads, sensitive data patterns, web shells, and more. The tester can simply pull this repository onto a new test machine and he will have access to every type of list he needs.
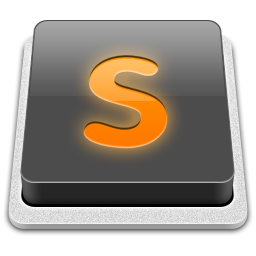
SublimeText3 Mac version
God-level code editing software (SublimeText3)

Notepad++7.3.1
Easy-to-use and free code editor