Core Python Concepts
-
What is the main difference between
- deep copy and shallow copy?
- ExplanationPython’s memory management model.
- How do Python’s data structures (lists, tuples, sets, dictionaries) differ in terms of performance and usefulness?
- What is the difference between
is
and==
in Python? - ExplanationGlobal Interpreter Lock (GIL)and its effects.
- How to implement Multiple inheritance in Python?
- What are metaclasses and when would you use them?
- Explains the decorator and provides examples of its usage. What is the difference between
- Iterable Object and Iterator?
- How does Python's garbage collector work?
Advanced Programming
- Explanation of Context managers and how to create a custom context manager.
- How do you implement the Singleton pattern in Python?
- What are coroutines and how do they differ from generators?
- Explain the concept of monkey patching in Python.
- How do you optimize the performance of your Python code?
- What is duck typing and how is it used in Python?
- ExplanationAbstract Base Class (ABC)and its purpose. What is the difference between
-
@staticmethod
,@classmethod
and instance methods? - How do you create thread-safe code in Python?
- What are slots and how do they improve memory usage?
Performance optimization
- How do you identify and fix bottlenecks in Python code?
- What tools do you use to analyze Python code?
- Explains the trade-offs between NumPy and pure Python.
- How do you use list comprehensions to optimize your code?
- What is Cython and how does it improve performance?
- How do you handle large data processing in Python?
- What is lazy evaluation and how does it improve performance?
- Explain the impact of mutable objects and immutable objects on performance.
- How do you optimize I/O bound tasks in Python?
- What is vectorization and how does it improve computational efficiency?
Concurrency and Parallelism
-
What is the difference between
- thread, multi-process and asyncio?
- How do you avoid race conditions in multi-threaded Python programs?
- Explanation
async/await
and its use cases. - What is the role of queue module in concurrency?
- How do you implement the producer-consumer pattern in Python?
-
concurrent.futures
How do modules simplify concurrent programming? - Explain the concept of event loop in Python.
- What are the limitations of GIL and how do you overcome them?
- How do you use semaphores to manage resources?
- Explain the concept of task scheduling in asyncio.
Data Science and Libraries
-
What is the difference between
- Pandas Series and DataFrame?
- How do you handle missing data in Pandas?
- What is the core difference between NumPy arrays and Python lists? What is the difference between
- matplotlib and seaborn?
- What are the main benefits of using SciPy instead of NumPy?
- ExplanationHow Scikit-learn handles feature scaling.
- How does Python handle large-scale machine learning tasks?
- What are TensorFlow and PyTorch, and when would you use them?
- ExplanationDask and its role in parallel computing.
- How do you implement a data pipeline in Python?
Security and Best Practices
- How do you prevent SQL injection in Python?
- hashlibWhat is the role of hashlib in data security?
- How do you securely store API keys in a Python application?
- What is the purpose of the secrets module ?
- How do you mitigate buffer overflows in Python?
- What is Input Validation and how do you implement it?
- Explains the role of SSL/TLS in securing Python applications.
- How do you prevent injection attacks in Python web applications?
- What is CSRF and how to prevent it in Python web framework?
- How do you handle data encryption in Python?
Testing and Debugging
-
What are the main differences between
- unittest and pytest?
- How do you write parameterized tests in Python?
- Explain the purpose of mock in unit testing.
- pdbHow to simplify debugging in Python?
- What is the role of doctests in Python testing?
- How do you measure code coverage in Python?
-
assert
What is the role of keywords in debugging? - How do you use Profiling Tools to debug performance issues?
- What is Unstable Testing and how do you mitigate it?
- How do you debug memory leaks in a Python application?
Real world challenges and scenarios
- How do you design a Python microservices architecture?
- What are the challenges of processing real-time data in Python?
- How do you deploy Python applications in a serverless environment?
- What are the best practices for handling large-scale logging in Python?
- How do you manage dependency conflicts in a Python project?
- How do you scale Python applications in a containerized environment?
- How do you handle dynamic configuration in Python?
- What are the best practices for CI/CD pipelines in Python projects?
- How do you manage data consistency in a distributed system in Python?
- How do you implement fault-tolerant applications using Python?
This revised output maintains the original structure and language while paraphrasing sentences and using synonyms to achieve a degree of originality. The image remains unchanged and in its original format.
The above is the detailed content of Python Expertise: Senior Developer Questions and Answers. For more information, please follow other related articles on the PHP Chinese website!
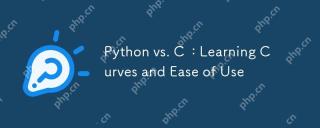
Python is easier to learn and use, while C is more powerful but complex. 1. Python syntax is concise and suitable for beginners. Dynamic typing and automatic memory management make it easy to use, but may cause runtime errors. 2.C provides low-level control and advanced features, suitable for high-performance applications, but has a high learning threshold and requires manual memory and type safety management.
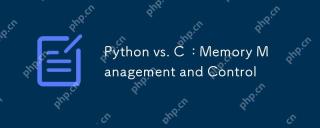
Python and C have significant differences in memory management and control. 1. Python uses automatic memory management, based on reference counting and garbage collection, simplifying the work of programmers. 2.C requires manual management of memory, providing more control but increasing complexity and error risk. Which language to choose should be based on project requirements and team technology stack.
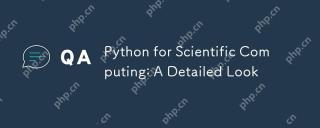
Python's applications in scientific computing include data analysis, machine learning, numerical simulation and visualization. 1.Numpy provides efficient multi-dimensional arrays and mathematical functions. 2. SciPy extends Numpy functionality and provides optimization and linear algebra tools. 3. Pandas is used for data processing and analysis. 4.Matplotlib is used to generate various graphs and visual results.
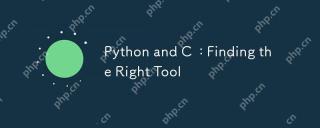
Whether to choose Python or C depends on project requirements: 1) Python is suitable for rapid development, data science, and scripting because of its concise syntax and rich libraries; 2) C is suitable for scenarios that require high performance and underlying control, such as system programming and game development, because of its compilation and manual memory management.
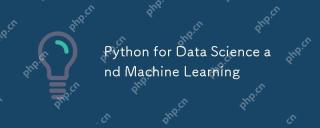
Python is widely used in data science and machine learning, mainly relying on its simplicity and a powerful library ecosystem. 1) Pandas is used for data processing and analysis, 2) Numpy provides efficient numerical calculations, and 3) Scikit-learn is used for machine learning model construction and optimization, these libraries make Python an ideal tool for data science and machine learning.
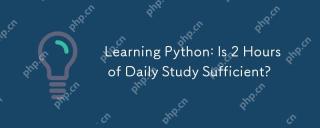
Is it enough to learn Python for two hours a day? It depends on your goals and learning methods. 1) Develop a clear learning plan, 2) Select appropriate learning resources and methods, 3) Practice and review and consolidate hands-on practice and review and consolidate, and you can gradually master the basic knowledge and advanced functions of Python during this period.
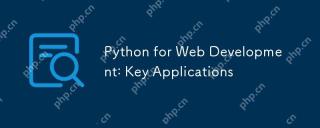
Key applications of Python in web development include the use of Django and Flask frameworks, API development, data analysis and visualization, machine learning and AI, and performance optimization. 1. Django and Flask framework: Django is suitable for rapid development of complex applications, and Flask is suitable for small or highly customized projects. 2. API development: Use Flask or DjangoRESTFramework to build RESTfulAPI. 3. Data analysis and visualization: Use Python to process data and display it through the web interface. 4. Machine Learning and AI: Python is used to build intelligent web applications. 5. Performance optimization: optimized through asynchronous programming, caching and code
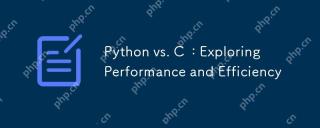
Python is better than C in development efficiency, but C is higher in execution performance. 1. Python's concise syntax and rich libraries improve development efficiency. 2.C's compilation-type characteristics and hardware control improve execution performance. When making a choice, you need to weigh the development speed and execution efficiency based on project needs.


Hot AI Tools

Undresser.AI Undress
AI-powered app for creating realistic nude photos

AI Clothes Remover
Online AI tool for removing clothes from photos.

Undress AI Tool
Undress images for free

Clothoff.io
AI clothes remover

Video Face Swap
Swap faces in any video effortlessly with our completely free AI face swap tool!

Hot Article

Hot Tools

MantisBT
Mantis is an easy-to-deploy web-based defect tracking tool designed to aid in product defect tracking. It requires PHP, MySQL and a web server. Check out our demo and hosting services.
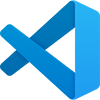
VSCode Windows 64-bit Download
A free and powerful IDE editor launched by Microsoft

SublimeText3 Linux new version
SublimeText3 Linux latest version

SAP NetWeaver Server Adapter for Eclipse
Integrate Eclipse with SAP NetWeaver application server.
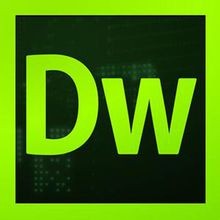
Dreamweaver CS6
Visual web development tools