This tutorial details the creation of an automated NBA statistics data pipeline using AWS services, Python, and DynamoDB. Whether you're a sports data enthusiast or an AWS learner, this hands-on project provides valuable experience in real-world data processing.
Project Overview
This pipeline automatically retrieves NBA statistics from the SportsData API, processes the data, and stores it in DynamoDB. The AWS services used include:
- DynamoDB: Data storage
- Lambda: Serverless execution
- CloudWatch: Monitoring and logging
Prerequisites
Before starting, ensure you have:
- Basic Python skills
- An AWS account
- The AWS CLI installed and configured
- A SportsData API key
Project Setup
Clone the repository and install dependencies:
git clone https://github.com/nolunchbreaks/nba-stats-pipeline.git cd nba-stats-pipeline pip install -r requirements.txt
Environment Configuration
Create a .env
file in the project root with these variables:
<code>SPORTDATA_API_KEY=your_api_key_here AWS_REGION=us-east-1 DYNAMODB_TABLE_NAME=nba-player-stats</code>
Project Structure
The project's directory structure is as follows:
<code>nba-stats-pipeline/ ├── src/ │ ├── __init__.py │ ├── nba_stats.py │ └── lambda_function.py ├── tests/ ├── requirements.txt ├── README.md └── .env</code>
Data Storage and Structure
DynamoDB Schema
The pipeline stores NBA team statistics in DynamoDB using this schema:
- Partition Key: TeamID
- Sort Key: Timestamp
- Attributes: Team statistics (win/loss, points per game, conference standings, division rankings, historical metrics)
AWS Infrastructure
DynamoDB Table Configuration
Configure the DynamoDB table as follows:
- Table Name:
nba-player-stats
- Primary Key:
TeamID
(String) - Sort Key:
Timestamp
(Number) - Provisioned Capacity: Adjust as needed
Lambda Function Configuration (if using Lambda)
- Runtime: Python 3.9
- Memory: 256MB
- Timeout: 30 seconds
- Handler:
lambda_function.lambda_handler
Error Handling and Monitoring
The pipeline includes robust error handling for API failures, DynamoDB throttling, data transformation issues, and invalid API responses. CloudWatch logs all events in structured JSON for performance monitoring, debugging, and ensuring successful data processing.
Resource Cleanup
After completing the project, clean up AWS resources:
git clone https://github.com/nolunchbreaks/nba-stats-pipeline.git cd nba-stats-pipeline pip install -r requirements.txt
Key Takeaways
This project highlighted:
- AWS Service Integration: Effective use of multiple AWS services for a cohesive data pipeline.
- Error Handling: The importance of thorough error handling in production environments.
- Monitoring: Essential role of logging and monitoring in maintaining data pipelines.
- Cost Management: Awareness of AWS resource usage and cleanup.
Future Enhancements
Possible project extensions include:
- Real-time game statistics integration
- Data visualization implementation
- API endpoints for data access
- Advanced data analysis capabilities
Conclusion
This NBA statistics pipeline demonstrates the power of combining AWS services and Python for building functional data pipelines. It's a valuable resource for those interested in sports analytics or AWS data processing. Share your experiences and suggestions for improvement!
Follow for more AWS and Python tutorials! Appreciate a ❤️ and a ? if you found this helpful!
The above is the detailed content of Building an NBA Stats Pipeline with AWS, Python, and DynamoDB. For more information, please follow other related articles on the PHP Chinese website!
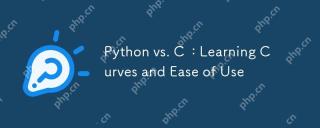
Python is easier to learn and use, while C is more powerful but complex. 1. Python syntax is concise and suitable for beginners. Dynamic typing and automatic memory management make it easy to use, but may cause runtime errors. 2.C provides low-level control and advanced features, suitable for high-performance applications, but has a high learning threshold and requires manual memory and type safety management.
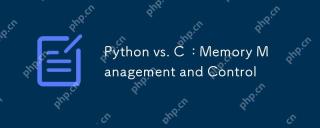
Python and C have significant differences in memory management and control. 1. Python uses automatic memory management, based on reference counting and garbage collection, simplifying the work of programmers. 2.C requires manual management of memory, providing more control but increasing complexity and error risk. Which language to choose should be based on project requirements and team technology stack.
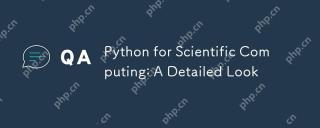
Python's applications in scientific computing include data analysis, machine learning, numerical simulation and visualization. 1.Numpy provides efficient multi-dimensional arrays and mathematical functions. 2. SciPy extends Numpy functionality and provides optimization and linear algebra tools. 3. Pandas is used for data processing and analysis. 4.Matplotlib is used to generate various graphs and visual results.
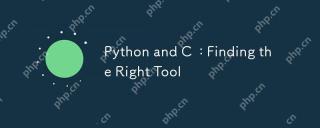
Whether to choose Python or C depends on project requirements: 1) Python is suitable for rapid development, data science, and scripting because of its concise syntax and rich libraries; 2) C is suitable for scenarios that require high performance and underlying control, such as system programming and game development, because of its compilation and manual memory management.
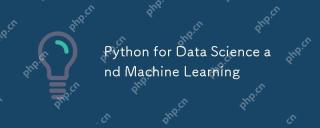
Python is widely used in data science and machine learning, mainly relying on its simplicity and a powerful library ecosystem. 1) Pandas is used for data processing and analysis, 2) Numpy provides efficient numerical calculations, and 3) Scikit-learn is used for machine learning model construction and optimization, these libraries make Python an ideal tool for data science and machine learning.
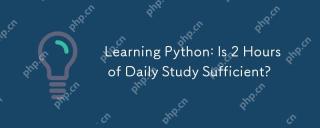
Is it enough to learn Python for two hours a day? It depends on your goals and learning methods. 1) Develop a clear learning plan, 2) Select appropriate learning resources and methods, 3) Practice and review and consolidate hands-on practice and review and consolidate, and you can gradually master the basic knowledge and advanced functions of Python during this period.
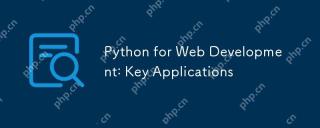
Key applications of Python in web development include the use of Django and Flask frameworks, API development, data analysis and visualization, machine learning and AI, and performance optimization. 1. Django and Flask framework: Django is suitable for rapid development of complex applications, and Flask is suitable for small or highly customized projects. 2. API development: Use Flask or DjangoRESTFramework to build RESTfulAPI. 3. Data analysis and visualization: Use Python to process data and display it through the web interface. 4. Machine Learning and AI: Python is used to build intelligent web applications. 5. Performance optimization: optimized through asynchronous programming, caching and code
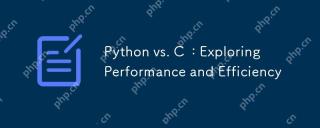
Python is better than C in development efficiency, but C is higher in execution performance. 1. Python's concise syntax and rich libraries improve development efficiency. 2.C's compilation-type characteristics and hardware control improve execution performance. When making a choice, you need to weigh the development speed and execution efficiency based on project needs.


Hot AI Tools

Undresser.AI Undress
AI-powered app for creating realistic nude photos

AI Clothes Remover
Online AI tool for removing clothes from photos.

Undress AI Tool
Undress images for free

Clothoff.io
AI clothes remover

AI Hentai Generator
Generate AI Hentai for free.

Hot Article

Hot Tools

Atom editor mac version download
The most popular open source editor

SublimeText3 Linux new version
SublimeText3 Linux latest version
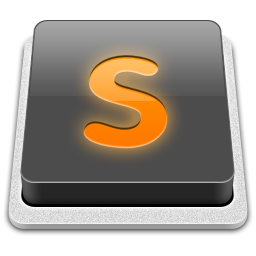
SublimeText3 Mac version
God-level code editing software (SublimeText3)

SublimeText3 English version
Recommended: Win version, supports code prompts!

SAP NetWeaver Server Adapter for Eclipse
Integrate Eclipse with SAP NetWeaver application server.