


Introduction
Effective code generation hinges on mastering prompt engineering. Well-crafted prompts guide Large Language Models (LLMs) to generate, improve, and optimize application code. This guide explores 15 proven prompting techniques categorized as root, refinement-based, decomposition-based, reasoning-based, and priming techniques. We'll illustrate each using a simple Flask web application, starting with a basic "Hello World" app and progressively enhancing it.
Research Note: We consulted aixrv.org for emerging prompting techniques. At the time of writing, no new approaches beyond those presented here were identified. However, prompt engineering is a rapidly evolving field, so continuous monitoring is recommended.
1. Root Techniques
These fundamental prompting methods provide straightforward paths to simple code outputs.
1.1. Direct Instruction Prompting
-
Overview: A concise command without extra details.
-
Prompt Example: "Create a minimal Python Flask app displaying 'Hello World!' at the root URL."
-
Generated Code (Conceptual): (Code snippet similar to the original example would appear here)
-
Why It Works: Sufficient for smaller tasks. Provides a foundation for subsequent enhancements.
1.2. Query-Based Prompting
-
Overview: Posing a question to elicit an explanatory response and/or code.
-
Prompt Example: "How do I build a basic Flask app that returns 'Hello World!' on the home page?"
-
Generated Response (Conceptual): The model might provide code and an explanation of each step.
-
Why It Works: Encourages more informative responses from the LLM.
1.3. Example-Based Prompting
-
Overview: Providing a sample of the desired style or format.
-
Prompt Example: "Here's a simple Node.js Express 'Hello World' server: [Node.js code]. Create a similar Flask 'Hello World' server."
-
Why It Works: The model mirrors the structure and style, ensuring consistency. More precise than direct instruction.
2. Refinement-Based Techniques
These techniques focus on iteratively improving existing code.
2.1. Iterative Refinement Prompting
-
Overview: Improving an initial solution incrementally.
-
Prompt Sequence:
- "Generate a minimal Flask app returning 'Hello World!'"
- "Modify this app to include a
/hello/<name></name>
endpoint that greets the user by name."
-
Refined Code Snippet (Conceptual): (Code snippet showing the added endpoint would appear here)
-
Why It Works: Builds upon existing code, allowing for incremental improvements.
2.2. Extension Prompting
-
Overview: Adding new features to existing code.
-
Prompt Example: "Add an endpoint to the Flask app that returns a JSON response with a list of sample users."
-
Refined Code Snippet (Conceptual): (Code snippet for the new endpoint would appear here)
-
Why It Works: Targets specific features, allowing for focused model attention.
2.3. Style/Formatting Transformation
-
Overview: Modifying code style (e.g., PEP 8 compliance).
-
Prompt Example: "Refactor the Flask app to adhere to PEP 8 naming conventions and limit line lengths to 79 characters."
-
Why It Works: Systematically applies style preferences.
3. Decomposition-Based Techniques
These techniques break down large tasks into smaller, more manageable steps.
3.1. Function-by-Function Decomposition
-
Overview: Separating tasks into sub-functions or modules.
-
Prompt Example:
- "Create a function
init_db()
to initialize a SQLite database." - "Create
insert_user(name)
to add users to the database." - "Create
get_all_users()
to retrieve all users."
- "Create a function
-
Result (Conceptual): (Code snippets for the three functions would appear here)
-
Why It Works: Organizes large tasks into modular, maintainable components.
3.2. Chunk-Based Prompting
-
Overview: Providing partial code and asking the model to complete missing sections.
-
Prompt Example: "Complete the Flask app below by adding routes to add and retrieve users: [Partial code snippet]"
-
Why It Works: Focuses the model on specific gaps, ensuring code cohesion.
3.3. Step-by-Step Instructions
-
Overview: Enumerating sub-tasks or logical steps.
-
Prompt Example:
- "Import necessary libraries."
- "Set up database initialization."
- "Create a route to add a user using
insert_user()
." - "Create a route to list users using
get_all_users()
."
-
Why It Works: Makes the code generation process transparent and ensures correct operational sequencing.
4. Reasoning-Based Techniques
These prompts encourage the model to articulate its reasoning process before providing code.
4.1. Chain-of-Thought Prompting
-
Overview: Requesting a step-by-step explanation of the reasoning process.
-
Prompt Example: "Explain how to add authentication to a Flask app step-by-step, then provide the code."
-
Why It Works: Encourages a clear path to the solution, resulting in more coherent code.
4.2. Zero-Shot Chain-of-Thought
-
Overview: Asking the model to reason through a problem without examples.
-
Prompt Example: "Explain your choice of password hashing library for Flask and show the code integrating it for user registration."
-
Why It Works: Promotes a thorough approach to library selection and usage.
4.3. Few-Shot Chain-of-Thought
-
Overview: Providing reasoning examples before presenting a new problem.
-
Prompt Example: "[Example of step-by-step reasoning for a login system]. Using this approach, add a
/register
route that securely stores new user credentials." -
Why It Works: Provides a framework for consistent logical application to new problems.
5. Priming Techniques
These techniques use added context to influence code style and domain knowledge.
5.1. Persona-Based Prompting
-
Overview: Instructing the model to adopt a specific role (e.g., security expert).
-
Prompt Example: "You're a senior Python backend developer specializing in security. Generate a secure Flask user registration route."
-
Why It Works: Tailors the solution to the persona's expertise, often including security best practices.
5.2. Skeleton (Template) Priming
-
Overview: Providing a template with placeholders for the model to fill.
-
Prompt Example: "Complete this Flask app template to implement a user login form: [Flask template with placeholders]"
-
Why It Works: Constrains the model to a specific framework.
5.3. Reference-Heavy Priming
-
Overview: Providing documentation or data schemas for the model to utilize.
-
Prompt Example: "Using this SQLAlchemy documentation [link], update the Flask app routes to use SQLAlchemy models instead of raw SQL."
-
Why It Works: Allows for specialized knowledge integration, ensuring accurate and up-to-date code.
Conclusion
These 15 techniques systematically guide code development and optimization using LLMs. Root techniques establish a base, refinement techniques enhance it, decomposition techniques manage complexity, reasoning techniques improve clarity, and priming techniques add context. Experiment with combinations for optimal results. Remember that prompt engineering is an evolving field, so continuous learning and adaptation are key.
The above is the detailed content of Prompting Techniques Every Developer Should Know for Code Generation. For more information, please follow other related articles on the PHP Chinese website!
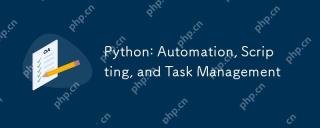
Python excels in automation, scripting, and task management. 1) Automation: File backup is realized through standard libraries such as os and shutil. 2) Script writing: Use the psutil library to monitor system resources. 3) Task management: Use the schedule library to schedule tasks. Python's ease of use and rich library support makes it the preferred tool in these areas.
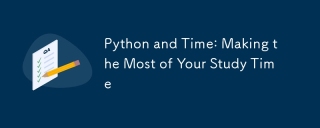
To maximize the efficiency of learning Python in a limited time, you can use Python's datetime, time, and schedule modules. 1. The datetime module is used to record and plan learning time. 2. The time module helps to set study and rest time. 3. The schedule module automatically arranges weekly learning tasks.
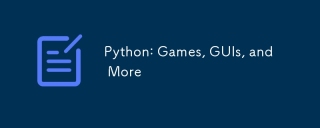
Python excels in gaming and GUI development. 1) Game development uses Pygame, providing drawing, audio and other functions, which are suitable for creating 2D games. 2) GUI development can choose Tkinter or PyQt. Tkinter is simple and easy to use, PyQt has rich functions and is suitable for professional development.
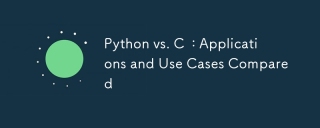
Python is suitable for data science, web development and automation tasks, while C is suitable for system programming, game development and embedded systems. Python is known for its simplicity and powerful ecosystem, while C is known for its high performance and underlying control capabilities.
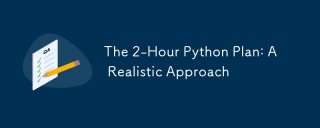
You can learn basic programming concepts and skills of Python within 2 hours. 1. Learn variables and data types, 2. Master control flow (conditional statements and loops), 3. Understand the definition and use of functions, 4. Quickly get started with Python programming through simple examples and code snippets.
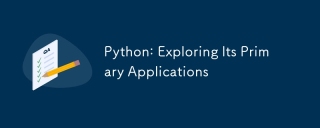
Python is widely used in the fields of web development, data science, machine learning, automation and scripting. 1) In web development, Django and Flask frameworks simplify the development process. 2) In the fields of data science and machine learning, NumPy, Pandas, Scikit-learn and TensorFlow libraries provide strong support. 3) In terms of automation and scripting, Python is suitable for tasks such as automated testing and system management.
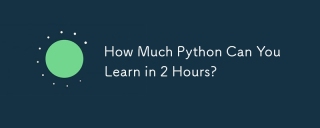
You can learn the basics of Python within two hours. 1. Learn variables and data types, 2. Master control structures such as if statements and loops, 3. Understand the definition and use of functions. These will help you start writing simple Python programs.
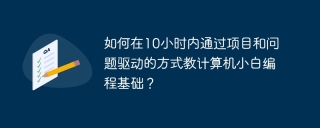
How to teach computer novice programming basics within 10 hours? If you only have 10 hours to teach computer novice some programming knowledge, what would you choose to teach...


Hot AI Tools

Undresser.AI Undress
AI-powered app for creating realistic nude photos

AI Clothes Remover
Online AI tool for removing clothes from photos.

Undress AI Tool
Undress images for free

Clothoff.io
AI clothes remover

AI Hentai Generator
Generate AI Hentai for free.

Hot Article

Hot Tools

SAP NetWeaver Server Adapter for Eclipse
Integrate Eclipse with SAP NetWeaver application server.

mPDF
mPDF is a PHP library that can generate PDF files from UTF-8 encoded HTML. The original author, Ian Back, wrote mPDF to output PDF files "on the fly" from his website and handle different languages. It is slower than original scripts like HTML2FPDF and produces larger files when using Unicode fonts, but supports CSS styles etc. and has a lot of enhancements. Supports almost all languages, including RTL (Arabic and Hebrew) and CJK (Chinese, Japanese and Korean). Supports nested block-level elements (such as P, DIV),
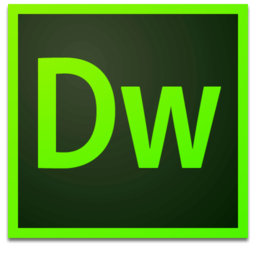
Dreamweaver Mac version
Visual web development tools
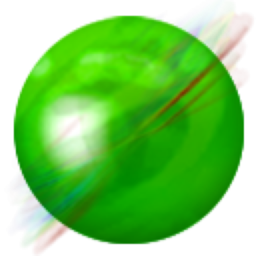
ZendStudio 13.5.1 Mac
Powerful PHP integrated development environment

MinGW - Minimalist GNU for Windows
This project is in the process of being migrated to osdn.net/projects/mingw, you can continue to follow us there. MinGW: A native Windows port of the GNU Compiler Collection (GCC), freely distributable import libraries and header files for building native Windows applications; includes extensions to the MSVC runtime to support C99 functionality. All MinGW software can run on 64-bit Windows platforms.