


Making Python CLIs More Maintainable: A Journey with Dynamic Command Loading
This blog post details a recent improvement to our HyperGraph project's command-line interface (CLI): a dynamic command loading system. Initially, adding new CLI commands was a multi-step manual process, violating DRY principles and the Open/Closed Principle.
The Challenge: Manual Command Registration
Adding a new command involved:
- Creating the command's implementation file.
- Updating imports within
__init__.py
. - Adding the command to a static list in the command loader.
This was tedious, prone to errors, and required modifying existing code for each new feature—far from ideal.
Exploring Solutions: Automation vs. Dynamic Loading
Two solutions were considered:
- An automation script to handle file modifications.
- A dynamic loading system leveraging Python's module discovery capabilities.
While an automation script seemed simpler initially, it would only address the symptoms, not the underlying design flaw.
The Solution: Dynamic Command Discovery
The chosen solution was a dynamic loading system that automatically registers commands. The core code is:
async def load_commands(self) -> None: implementations_package = "hypergraph.cli.commands.implementations" for _, name, _ in pkgutil.iter_modules([str(self.commands_path)]): if name.startswith("_"): # Skip private modules continue module = importlib.import_module(f"{implementations_package}.{name}") for item_name, item in inspect.getmembers(module): if (inspect.isclass(item) and issubclass(item, BaseCommand) and item != BaseCommand): command = item(self.system) self.registry.register_command(command)
This approach offers several advantages:
- Eliminates manual command registration.
- Maintains backward compatibility with existing code.
- Simplifies adding new commands to placing a new file in the
implementations
directory. - Leverages standard Python libraries, adhering to the "batteries included" philosophy.
Key Lessons Learned
- Avoid Quick Fixes: While automation offered short-term relief, dynamic loading provides a more sustainable, long-term solution.
-
Preserve Compatibility: Maintaining original
CommandRegistry
methods ensures existing code continues to function. - Robust Error Handling: Comprehensive error handling and logging are vital for debugging in a dynamic system.
A Minor Setback
A minor issue arose with a missing type import (Any
from typing
), highlighting the importance of thorough type hinting in Python.
Future Steps
While the dynamic system is implemented, an automation script remains a possibility as a development tool for generating command file templates. Future plans include:
- Monitoring production performance.
- Gathering developer feedback.
- Implementing further improvements based on real-world use.
Conclusion
This refactoring demonstrates the benefits of reevaluating approaches for more elegant solutions. Though requiring more initial effort than a quick fix, the result is more maintainable, extensible, and Pythonic code. Prioritizing long-term maintainability simplifies future development.
Tags: #Python #Refactoring #CleanCode #CLI #Programming
For detailed technical information, refer to our Codeberg repository.
The above is the detailed content of Making Python CLIs More Maintainable: A Journey with Dynamic Command Loading. For more information, please follow other related articles on the PHP Chinese website!
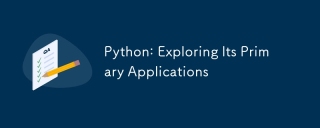
Python is widely used in the fields of web development, data science, machine learning, automation and scripting. 1) In web development, Django and Flask frameworks simplify the development process. 2) In the fields of data science and machine learning, NumPy, Pandas, Scikit-learn and TensorFlow libraries provide strong support. 3) In terms of automation and scripting, Python is suitable for tasks such as automated testing and system management.
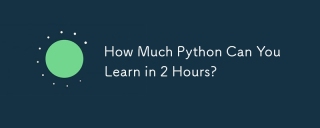
You can learn the basics of Python within two hours. 1. Learn variables and data types, 2. Master control structures such as if statements and loops, 3. Understand the definition and use of functions. These will help you start writing simple Python programs.
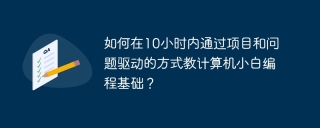
How to teach computer novice programming basics within 10 hours? If you only have 10 hours to teach computer novice some programming knowledge, what would you choose to teach...
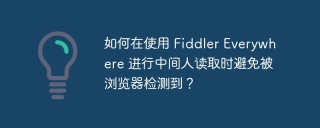
How to avoid being detected when using FiddlerEverywhere for man-in-the-middle readings When you use FiddlerEverywhere...
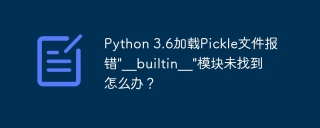
Error loading Pickle file in Python 3.6 environment: ModuleNotFoundError:Nomodulenamed...
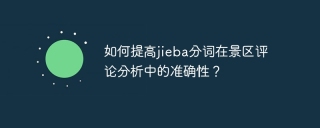
How to solve the problem of Jieba word segmentation in scenic spot comment analysis? When we are conducting scenic spot comments and analysis, we often use the jieba word segmentation tool to process the text...

How to use regular expression to match the first closed tag and stop? When dealing with HTML or other markup languages, regular expressions are often required to...
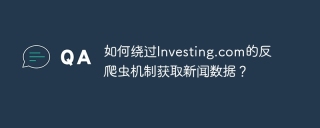
Understanding the anti-crawling strategy of Investing.com Many people often try to crawl news data from Investing.com (https://cn.investing.com/news/latest-news)...


Hot AI Tools

Undresser.AI Undress
AI-powered app for creating realistic nude photos

AI Clothes Remover
Online AI tool for removing clothes from photos.

Undress AI Tool
Undress images for free

Clothoff.io
AI clothes remover

AI Hentai Generator
Generate AI Hentai for free.

Hot Article

Hot Tools

SublimeText3 English version
Recommended: Win version, supports code prompts!

Atom editor mac version download
The most popular open source editor
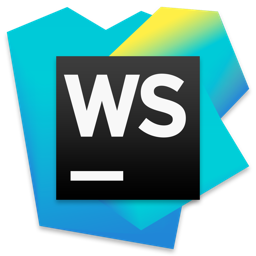
WebStorm Mac version
Useful JavaScript development tools
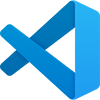
VSCode Windows 64-bit Download
A free and powerful IDE editor launched by Microsoft

MinGW - Minimalist GNU for Windows
This project is in the process of being migrated to osdn.net/projects/mingw, you can continue to follow us there. MinGW: A native Windows port of the GNU Compiler Collection (GCC), freely distributable import libraries and header files for building native Windows applications; includes extensions to the MSVC runtime to support C99 functionality. All MinGW software can run on 64-bit Windows platforms.