Imagine you’re a chef in a bustling kitchen. You have a recipe—a function, if you will. Over time, you find that most of your dishes require a drizzle of olive oil, a pinch of salt, or a sprinkle of herbs before they’re served. Instead of manually adding these finishing touches to every dish, wouldn’t it be convenient to have an assistant who applies them automatically? That’s precisely what Python decorators can do for your code—add functionality in an elegant, reusable, and expressive way.
In this article, we’ll explore the world of advanced Python decorators. We’ll go beyond the basics, diving into parameterized decorators, stackable decorators, and even decorators with classes. We’ll also highlight best practices and pitfalls to avoid. Ready? Let’s start cooking!
The Basics Revisited
Before diving into the deep end, let’s revisit the foundation. A decorator in Python is simply a function that takes another function (or method) as an argument, augments it, and returns a new function. Here’s an example:
# Basic decorator example def simple_decorator(func): def wrapper(*args, **kwargs): print(f"Calling {func.__name__}...") result = func(*args, **kwargs) print(f"{func.__name__} finished.") return result return wrapper @simple_decorator def say_hello(): print("Hello, world!") say_hello()
Output:
Calling say_hello... Hello, world! say_hello finished.
Now, let’s graduate to the advanced use cases.
Parameterized Decorators
Sometimes, a decorator needs to accept its own arguments. For instance, what if we want a decorator that logs messages at different levels (INFO, DEBUG, ERROR)?
# Parameterized decorator example def log(level): def decorator(func): def wrapper(*args, **kwargs): print(f"[{level}] Calling {func.__name__}...") result = func(*args, **kwargs) print(f"[{level}] {func.__name__} finished.") return result return wrapper return decorator @log("INFO") def process_data(): print("Processing data...") process_data()
Output:
[INFO] Calling process_data... Processing data... [INFO] process_data finished.
This layered structure—a function returning a decorator—is key to creating flexible, parameterized decorators.
Stackable Decorators
Python allows multiple decorators to be applied to a single function. Let’s create two decorators and stack them.
# Stackable decorators def uppercase(func): def wrapper(*args, **kwargs): result = func(*args, **kwargs) return result.upper() return wrapper def exclaim(func): def wrapper(*args, **kwargs): result = func(*args, **kwargs) return result + "!!!" return wrapper @uppercase @exclaim def greet(): return "hello" print(greet())
Output:
HELLO!!!
Here, the decorators are applied in a bottom-up manner: @exclaim wraps greet, and @uppercase wraps the result.
Using Classes as Decorators
A lesser-known feature of Python is that classes can be used as decorators. This can be particularly useful when you need to maintain state.
# Class-based decorator class CountCalls: def __init__(self, func): self.func = func self.call_count = 0 def __call__(self, *args, **kwargs): self.call_count += 1 print(f"Call {self.call_count} to {self.func.__name__}") return self.func(*args, **kwargs) @CountCalls def say_hello(): print("Hello!") say_hello() say_hello()
Output:
Call 1 to say_hello Hello! Call 2 to say_hello Hello!
Here, the call method enables the class to behave like a function, allowing it to wrap the target function seamlessly.
Decorators for Methods
Decorators work just as well with methods in classes. However, handling self correctly is essential.
# Method decorator example def log_method(func): def wrapper(self, *args, **kwargs): print(f"Method {func.__name__} called on {self}") return func(self, *args, **kwargs) return wrapper class Greeter: @log_method def greet(self, name): print(f"Hello, {name}!") obj = Greeter() obj.greet("Alice")
Output:
Method greet called on <__main__.greeter object at> Hello, Alice! </__main__.greeter>
Combining Decorators with Context Managers
Sometimes, you’ll need to integrate decorators with resource management. For instance, let’s create a decorator that times the execution of a function.
import time # Timing decorator def time_it(func): def wrapper(*args, **kwargs): start = time.time() result = func(*args, **kwargs) end = time.time() print(f"{func.__name__} took {end - start:.2f} seconds") return result return wrapper @time_it def slow_function(): time.sleep(2) print("Done sleeping!") slow_function()
Output:
# Basic decorator example def simple_decorator(func): def wrapper(*args, **kwargs): print(f"Calling {func.__name__}...") result = func(*args, **kwargs) print(f"{func.__name__} finished.") return result return wrapper @simple_decorator def say_hello(): print("Hello, world!") say_hello()
Best Practices
When working with decorators, keeping readability and maintainability in mind is crucial. Here are some tips:
- Use functools.wraps: This preserves metadata of the original function.
Calling say_hello... Hello, world! say_hello finished.
Test Thoroughly: Decorators can introduce subtle bugs, especially when chaining multiple decorators.
Document Decorators: Clearly document what each decorator does and its expected parameters.
Avoid Overuse: While decorators are powerful, overusing them can make code difficult to follow.
Wrapping Up
Decorators are one of Python’s most expressive features. They allow you to extend and modify behavior in a clean, reusable manner. From parameterized decorators to class-based implementations, the possibilities are endless. As you hone your skills, you’ll find yourself leveraging decorators to write cleaner, more Pythonic code—and perhaps, like a great chef, creating your signature touches in every recipe you craft.
note: AI assisted content
The above is the detailed content of Advanced Python Decorators: Elevating Your Code. For more information, please follow other related articles on the PHP Chinese website!
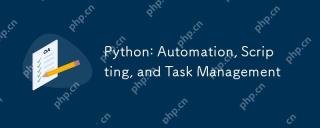
Python excels in automation, scripting, and task management. 1) Automation: File backup is realized through standard libraries such as os and shutil. 2) Script writing: Use the psutil library to monitor system resources. 3) Task management: Use the schedule library to schedule tasks. Python's ease of use and rich library support makes it the preferred tool in these areas.
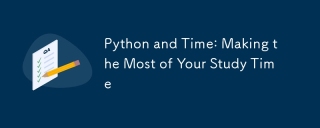
To maximize the efficiency of learning Python in a limited time, you can use Python's datetime, time, and schedule modules. 1. The datetime module is used to record and plan learning time. 2. The time module helps to set study and rest time. 3. The schedule module automatically arranges weekly learning tasks.
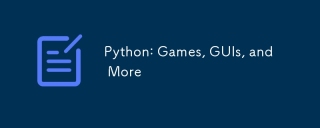
Python excels in gaming and GUI development. 1) Game development uses Pygame, providing drawing, audio and other functions, which are suitable for creating 2D games. 2) GUI development can choose Tkinter or PyQt. Tkinter is simple and easy to use, PyQt has rich functions and is suitable for professional development.
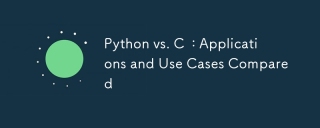
Python is suitable for data science, web development and automation tasks, while C is suitable for system programming, game development and embedded systems. Python is known for its simplicity and powerful ecosystem, while C is known for its high performance and underlying control capabilities.
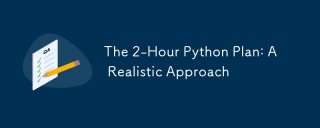
You can learn basic programming concepts and skills of Python within 2 hours. 1. Learn variables and data types, 2. Master control flow (conditional statements and loops), 3. Understand the definition and use of functions, 4. Quickly get started with Python programming through simple examples and code snippets.
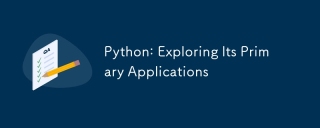
Python is widely used in the fields of web development, data science, machine learning, automation and scripting. 1) In web development, Django and Flask frameworks simplify the development process. 2) In the fields of data science and machine learning, NumPy, Pandas, Scikit-learn and TensorFlow libraries provide strong support. 3) In terms of automation and scripting, Python is suitable for tasks such as automated testing and system management.
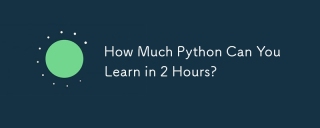
You can learn the basics of Python within two hours. 1. Learn variables and data types, 2. Master control structures such as if statements and loops, 3. Understand the definition and use of functions. These will help you start writing simple Python programs.
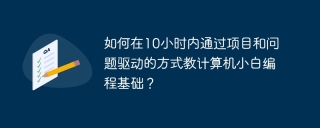
How to teach computer novice programming basics within 10 hours? If you only have 10 hours to teach computer novice some programming knowledge, what would you choose to teach...


Hot AI Tools

Undresser.AI Undress
AI-powered app for creating realistic nude photos

AI Clothes Remover
Online AI tool for removing clothes from photos.

Undress AI Tool
Undress images for free

Clothoff.io
AI clothes remover

AI Hentai Generator
Generate AI Hentai for free.

Hot Article

Hot Tools

Atom editor mac version download
The most popular open source editor

MinGW - Minimalist GNU for Windows
This project is in the process of being migrated to osdn.net/projects/mingw, you can continue to follow us there. MinGW: A native Windows port of the GNU Compiler Collection (GCC), freely distributable import libraries and header files for building native Windows applications; includes extensions to the MSVC runtime to support C99 functionality. All MinGW software can run on 64-bit Windows platforms.
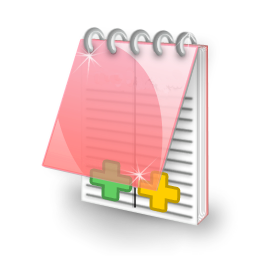
EditPlus Chinese cracked version
Small size, syntax highlighting, does not support code prompt function
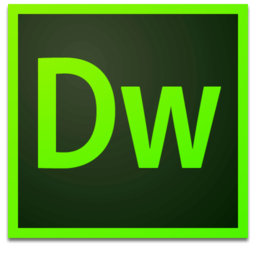
Dreamweaver Mac version
Visual web development tools

Notepad++7.3.1
Easy-to-use and free code editor