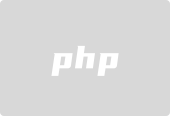
Buy Me a Coffee☕
*Memos:
-
My post explains linspace().
-
My post explains logspace().
arange() can create the 1D tensor of zero or integers or floating-point numbers between start and end-1(start<=x<=end-1) as shown below:
*Memos:
-
arange() can be used with torch but not with a tensor.
- The 1st argument with torch is start(Optional-Default:0-Type:int, float, complex or bool):
*Memos
- It must be lower than or equal to end.
- The 0D tensor of int, float, complex or bool also works.
- The 2nd argument with torch is end(Required-Type:int, float, complex or bool):
*Memos:
- It must be greater than or equal to start.
- The 0D tensor of int, float, complex or bool also works.
- The 3rd argument with torch is step(Optional-Default:1-Type:int, float, complex or bool):
*Memos:
- It must be greater than 0.
- The 0D tensor of int, float, complex or bool also works.
- There is dtype argument with torch(Optional-Default:None-Type:dtype):
*Memos:
- If it's None, it's inferred from start, end or step, then for floating-point numbers, get_default_dtype() is used. *My post explains get_default_dtype() and set_default_dtype().
-
dtype= must be used.
-
My post explains dtype argument.
- There is device argument with torch(Optional-Default:None-Type:str, int or device()):
*Memos:
- If it's None, get_default_device() is used. *My post explains get_default_device() and set_default_device().
-
device= must be used.
-
My post explains device argument.
- There is requires_grad argument with torch(Optional-Default:False-Type:bool):
*Memos:
-
requires_grad= must be used.
-
My post explains requires_grad argument.
- There is out argument with torch(Optional-Default:None-Type:tensor):
*Memos:
-
out= must be used.
-
My post explains out argument.
- There is range() which is similar to arange() but range() is deprecated.
import torch
torch.arange(end=5)
# tensor([0, 1, 2, 3, 4])
torch.arange(start=5, end=15)
# tensor([5, 6, 7, 8, 9, 10, 11, 12, 13, 14])
torch.arange(start=5, end=15, step=3)
# tensor([5, 8, 11, 14])
torch.arange(start=-5, end=5)
# tensor([-5, -4, -3, -2, -1, 0, 1, 2, 3, 4])
torch.arange(start=-5, end=5, step=3)
torch.arange(start=torch.tensor(-5),
end=torch.tensor(5),
step=torch.tensor(3))
# tensor([-5, -2, 1, 4])
torch.arange(start=-5., end=5., step=3.)
torch.arange(start=torch.tensor(-5.),
end=torch.tensor(5.),
step=torch.tensor(3.))
# tensor([-5., -2., 1., 4.])
torch.arange(start=-5.+0.j, end=5.+0.j, step=3.+0.j)
torch.arange(start=torch.tensor(-5.+0.j),
end=torch.tensor(5.+0.j),
step=torch.tensor(3.+0.j))
# tensor([-5., -2., 1., 4.])
torch.arange(start=False, end=True, step=True)
torch.arange(start=torch.tensor(False),
end=torch.tensor(True),
step=torch.tensor(True))
# tensor([0])
The above is the detailed content of arange in PyTorch. For more information, please follow other related articles on the PHP Chinese website!