I recently tried to implement a DQN based Chess Agent.
Now, anyone who knows how DQNs and Chess works would tell you that's a dumb idea.
And...it was, but as a beginner I enjoyed it nevertheless. In this article I'll share the insights I learned while working on this.
Understanding the Environment.
Before I started implementing the Agent itself, I had to familiarize myself with the environment I'll be using and make a custom wrapper on top of it so that it can interact with the Agent during training.
-
I used the chess environment from the kaggle_environments library.
from kaggle_environments import make env = make("chess", debug=True)
-
I also used Chessnut, which is a lightweight python library that helps parse and validate chess games.
from Chessnut import Game initial_fen = env.state[0]['observation']['board'] game=Game(env.state[0]['observation']['board'])
In this environment, the state of the board is stored in the FEN format.
It provides a compact way to represent all the pieces on the board and the currently active player. However, since I planned on feeding the input to a neural network, I had to modify the representation of the state.
Converting FEN to Matrix format
Since there are 12 different types of pieces on a board, I created 12 channels of 8x8 grids to represent the state of each of those types on the board.
Creating a Wrapper for the Environment
class EnvCust: def __init__(self): self.env = make("chess", debug=True) self.game=Game(env.state[0]['observation']['board']) print(self.env.state[0]['observation']['board']) self.action_space=game.get_moves(); self.obs_space=(self.env.state[0]['observation']['board']) def get_action(self): return Game(self.env.state[0]['observation']['board']).get_moves(); def get_obs_space(self): return fen_to_board(self.env.state[0]['observation']['board']) def step(self,action): reward=0 g=Game(self.env.state[0]['observation']['board']); if(g.board.get_piece(Game.xy2i(action[2:4]))=='q'): reward=7 elif g.board.get_piece(Game.xy2i(action[2:4]))=='n' or g.board.get_piece(Game.xy2i(action[2:4]))=='b' or g.board.get_piece(Game.xy2i(action[2:4]))=='r': reward=4 elif g.board.get_piece(Game.xy2i(action[2:4]))=='P': reward=2 g=Game(self.env.state[0]['observation']['board']); g.apply_move(action) done=False if(g.status==2): done=True reward=10 elif g.status == 1: done = True reward = -5 self.env.step([action,'None']) self.action_space=list(self.get_action()) if(self.action_space==[]): done=True else: self.env.step(['None',random.choice(self.action_space)]) g=Game(self.env.state[0]['observation']['board']); if g.status==2: reward=-10 done=True self.action_space=list(self.get_action()) return self.env.state[0]['observation']['board'],reward,done
The point of this wrapper was to provide a reward policy for the agent and a step function which is used to interact with the environment during training.
Chessnut was useful in getting information like the legal moves possible at current state of the board and also to recognize Checkmates during the game.
I tried to create a reward policy to give positive points for checkmates and taking out enemy pieces while negative points for losing the game.
Creating a Replay Buffer
Replay Buffer is used during the training period to save the (state,action,reward,next state) output by the Q-Network and later used randomly for backpropagation of the Target Network
Auxiliary Functions
Chessnut returns legal action in UCI format which looks like 'a2a3', however to interact with the Neural Network I converted each action into a distinct index using a basic pattern. There are total 64 Squares, so I decided to have 64*64 unique indexes for each move.
I know that not all of the 64*64 moves would be legal, but I could handle legality using Chessnut and the pattern was simple enough.
Neural Network Structure
from kaggle_environments import make env = make("chess", debug=True)
This Neural Network uses the Convolutional Layers to take in the 12 channel input and also uses the valid action indexes to filter out the reward output prediction.
Implementing the Agent
from Chessnut import Game initial_fen = env.state[0]['observation']['board'] game=Game(env.state[0]['observation']['board'])
This was obviously a very basic model which had no chance of actually performing well (And it didn't), but it did help me understand how DQNs work a little better.
The above is the detailed content of Building a Chess Agent using DQN. For more information, please follow other related articles on the PHP Chinese website!
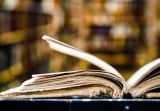
This tutorial demonstrates how to use Python to process the statistical concept of Zipf's law and demonstrates the efficiency of Python's reading and sorting large text files when processing the law. You may be wondering what the term Zipf distribution means. To understand this term, we first need to define Zipf's law. Don't worry, I'll try to simplify the instructions. Zipf's Law Zipf's law simply means: in a large natural language corpus, the most frequently occurring words appear about twice as frequently as the second frequent words, three times as the third frequent words, four times as the fourth frequent words, and so on. Let's look at an example. If you look at the Brown corpus in American English, you will notice that the most frequent word is "th
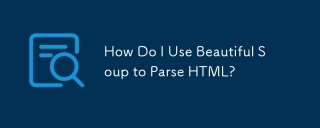
This article explains how to use Beautiful Soup, a Python library, to parse HTML. It details common methods like find(), find_all(), select(), and get_text() for data extraction, handling of diverse HTML structures and errors, and alternatives (Sel
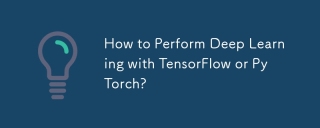
This article compares TensorFlow and PyTorch for deep learning. It details the steps involved: data preparation, model building, training, evaluation, and deployment. Key differences between the frameworks, particularly regarding computational grap
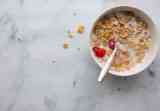
Serialization and deserialization of Python objects are key aspects of any non-trivial program. If you save something to a Python file, you do object serialization and deserialization if you read the configuration file, or if you respond to an HTTP request. In a sense, serialization and deserialization are the most boring things in the world. Who cares about all these formats and protocols? You want to persist or stream some Python objects and retrieve them in full at a later time. This is a great way to see the world on a conceptual level. However, on a practical level, the serialization scheme, format or protocol you choose may determine the speed, security, freedom of maintenance status, and other aspects of the program
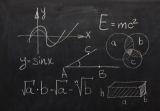
Python's statistics module provides powerful data statistical analysis capabilities to help us quickly understand the overall characteristics of data, such as biostatistics and business analysis. Instead of looking at data points one by one, just look at statistics such as mean or variance to discover trends and features in the original data that may be ignored, and compare large datasets more easily and effectively. This tutorial will explain how to calculate the mean and measure the degree of dispersion of the dataset. Unless otherwise stated, all functions in this module support the calculation of the mean() function instead of simply summing the average. Floating point numbers can also be used. import random import statistics from fracti
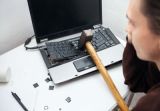
In this tutorial you'll learn how to handle error conditions in Python from a whole system point of view. Error handling is a critical aspect of design, and it crosses from the lowest levels (sometimes the hardware) all the way to the end users. If y
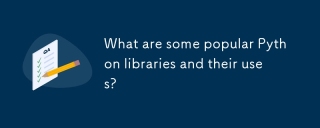
The article discusses popular Python libraries like NumPy, Pandas, Matplotlib, Scikit-learn, TensorFlow, Django, Flask, and Requests, detailing their uses in scientific computing, data analysis, visualization, machine learning, web development, and H
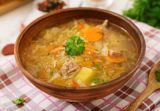
This tutorial builds upon the previous introduction to Beautiful Soup, focusing on DOM manipulation beyond simple tree navigation. We'll explore efficient search methods and techniques for modifying HTML structure. One common DOM search method is ex


Hot AI Tools

Undresser.AI Undress
AI-powered app for creating realistic nude photos

AI Clothes Remover
Online AI tool for removing clothes from photos.

Undress AI Tool
Undress images for free

Clothoff.io
AI clothes remover

AI Hentai Generator
Generate AI Hentai for free.

Hot Article

Hot Tools
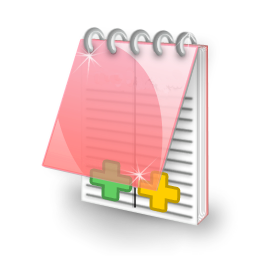
EditPlus Chinese cracked version
Small size, syntax highlighting, does not support code prompt function

Safe Exam Browser
Safe Exam Browser is a secure browser environment for taking online exams securely. This software turns any computer into a secure workstation. It controls access to any utility and prevents students from using unauthorized resources.

MantisBT
Mantis is an easy-to-deploy web-based defect tracking tool designed to aid in product defect tracking. It requires PHP, MySQL and a web server. Check out our demo and hosting services.

SublimeText3 English version
Recommended: Win version, supports code prompts!
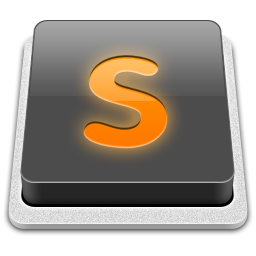
SublimeText3 Mac version
God-level code editing software (SublimeText3)
