


Sorting Algoritms
1. Bubble Sort
In this, we swap the higher element with its neighbor until we reach the end of the array. Now the highest element is at the last position. So, we change the boundary and decrease it by 1 from the last. At worst, we have to iterate n times to sort the array.
def bubble_sort(arr): n = len(arr) for i in range(n): swapped = False # Last i elements are already in place, so we don't need to check them for j in range(0, n-i-1): # Swap if the element found is greater than the next element if arr[j] > arr[j+1]: arr[j], arr[j+1] = arr[j+1], arr[j] # Swap the elements swapped = True if not swapped: break return arr
Algorithm -
- Iterate over the array and find the maximum element, then swap it to the last.
- Compare all adjacent elements and swap if the larger element is before the smaller element. Keep doing this until you reach the end of the array.
- Maintain a flag: if no element is swapped, then we can break the loop, as the array is sorted.
Time Complexity :
- Best Case - If the array is already sorted then only one iteration is required. Time complexity - O(n)
- Average Case - if the array is randomly sorted, then time complexity - O(n2)
- Worst Case - if the array is in decreasing order then we will require n*2 iterations.
Space Complexity - O(1), No extra memory required.
Advantages -
No extra memory required.
Stable as elements keep their relative order.
Disadvantages -
- Time complexity - O(n2), which is very high for large datasets.
Applications-
- Can be used only when the dataset is very small, and simplicity outweighs inefficiency due to high time complexity.
2. Selection Sort
In this, we find the smallest element in the array and replace it with the first element. Then, we increase the boundary by 1 and repeat the same steps until we reach the end of the array.
def selectionSort(a): i = 0 while iAlgorithm -
Iterate over the array and find the minimum element.
Swap it with the first element and increase the pointer by 1.
Repeat this process until we reach the end of the array.
Time Complexity : It has a time complexity of O(n2) in all three cases: best, average, and worst. This is because we have to select the minimum element and swap it every time, regardless of whether the array is already sorted or not.
Space Complexity - O(1), No extra memory required.
Advantages -
No extra memory required.
Fewer swaps are done than in bubble sort.
Disadvantages -
Time complexity - O(n2), which is very high for large datasets.
Not Stable, as it does not maintain the relative order of equal elements.
Applications -
It can be used in systems with limited memory as it does not require additional storage.
It is used in systems where minimizing the number of swaps is critical, such as in systems with slow write operations.
3. Insertion Sort
It is an algorithm that works by inserting an unsorted element into its correct position by iteratively checking backwards from the position of the element to the start of the array.
def bubble_sort(arr): n = len(arr) for i in range(n): swapped = False # Last i elements are already in place, so we don't need to check them for j in range(0, n-i-1): # Swap if the element found is greater than the next element if arr[j] > arr[j+1]: arr[j], arr[j+1] = arr[j+1], arr[j] # Swap the elements swapped = True if not swapped: break return arrAlgorithm -
Start from the second element of the array and compare it with the first element. If the current element is smaller than the first element, then swap them.
Now increase the pointer and do this for the third element: compare it with the second and first elements.
Repeat the same process for the rest of the elements, comparing them with all the previous elements, and insert them at the suitable position.
Time Complexity :
- Best Case - If the array is already sorted then only one iteration is required. Time complexity is O(n)
- Average Case - if the array is randomly sorted, then time complexity is O(n2)
- Worst Case - if the array is in decreasing order then we will require n2 iterations.Space Complexity - O(1), No extra memory required.
Advantages -
- No extra memory required.
- Stable, as elements keep their relative order.
Disadvantages -
Time complexity - O(n2), which is very high for large datasets.
Not Stable, as it does not maintain the relative order of equal elements.
Applications-
- It is efficient for scenarios where elements arrive in real-time and need to be sorted, such as live data streams.
4. Merge Sort
Merge Sort is an algorithm that follows the divide and conquer approach. It has two main steps: first, dividing the array recursively, and second, merging the divided arrays in sorted order.
def selectionSort(a): i = 0 while iAlgorithm -
Divide the array into two halves by calculating the mid-point.
Continue dividing until the length of each sub-array is 1.
Call the merge function on both halves: the left half and the right half.
Use three pointers for the merging process:
- The first pointer for the left half array.
- The second pointer for the right half array.
- The third pointer for the sorted array.
Iterate through both halves and compare their elements. Insert the smaller element into the sorted array and increment the corresponding pointer by 1.
Repeat this process recursively until the entire array is sorted.
Time Complexity : Merge Sort has a time complexity of O(n log n) in all three cases: best, average, and worst. This is because, irrespective of whether the array is already sorted or not, the same steps are followed for each division and merge.
O( log n ) - The array size is halved at each step during the divide phase.
O(n) - During merging process we have to iterate over all the elements once.
So the total time complexity is O (n) * O (log n) = O (n log n)
Space Complexity - O(n), Extra memory is required during the merging process to store the temporary arrays.
Advantages -
Stable, as elements keep their relative order.
Time complexity is O (n log n), even for the large datasets.
Suitable for parallel processing because sub-arrays are merged independently.
Disadvantages -
- Time complexity - O(n2), which is very high for large datasets.
- Extra memory is required for the merging process.
- Not in place as extra memory is required.
- Slower than QuickSort in general for most datasets.
Applications -
- It is used in situations where data is too large to fit in memory, such as merging large files.
- It is used to sort linked lists because random access is not required.
5. Quick Sort
Quick Sort is an algorithm that follows the divide-and-conquer approach. We choose a pivot element and partition the array around the pivot element after placing the pivot in its correct sorted position.
The first step is to choose the pivot element and then partition the array around the pivot. All elements smaller than the pivot will be on the left, and all elements greater than the pivot will be on its right. The pivot is then in its correct sorted position. Recursively, the same process is applied by dividing the array into two halves: the first half contains the elements before the pivot, and the second half contains the elements after the pivot. This process is repeated until the length of each sub-array reaches 1.
def bubble_sort(arr): n = len(arr) for i in range(n): swapped = False # Last i elements are already in place, so we don't need to check them for j in range(0, n-i-1): # Swap if the element found is greater than the next element if arr[j] > arr[j+1]: arr[j], arr[j+1] = arr[j+1], arr[j] # Swap the elements swapped = True if not swapped: break return arrAlgorithm -
- Divide the array into two halves by calculating the mid-point.
- Choose a pivot; any element can be chosen as the pivot.
- Iterate over the array and compare each element with the pivot.
- Place all elements smaller than the pivot on the left and all elements greater than the pivot on the right.
- Swap the pivot with the left pointer so that the pivot is at its correct sorted position.
- Repeat this process recursively until the length of the partition is greater than 1.
Time Complexity :
1. Best Case - Time complexity - O(n log n), when the pivot divides the array into two equal halves.
2. Average Case - Time complexity - O(n log n), when the pivot divides the array into two equal halves. But not necessarily equal.
3. Worst case - Time complexity - O(n2) , When -
The smallest element is chosen as the pivot in an already sorted array.
The largest element is chosen as the pivot in an array sorted in decreasing order.
O( log n ) - The array size is halved at each step during the divide phase.
O(n) - During the ordering of elements.
So, the total time complexity is O (n) * O (log n) = O (n log n)
Space Complexity :
Best and Average case - O( log n) - for the recursive stack.
Worst Case - O(n) - for the recursive stack.
Advantages -
- Efficient for large datasets unless the pivot is chosen bad.
- It is cache friendly as we work on the same array to sort and do not copy data to to any auxiliary array.
- One of the fastest general purpose algorithms for large data when stability is not required.
Disadvantages -
- Time complexity - O(n2), which is very high for large datasets.
- Not Stable, as it does not maintain the relative order of equal elements.
Applications -
- It is used in programming libraries and frameworks. For example - Python’s sorted() function and Java’s Array.sort() is based on quick sort.
- It is used indDatabase query optimization by efficiently sorting rows during query execution.
- It works well for in-memory sorting of large datasets due to its cache-friendly properties.
6.Heap Sort
Heap Sort is a comparison-based sorting algorithm. It is an extension of Selection Sort. In Heap Sort, we create a Binary Heap and swap the maximum or minimum element with the last element. Then, we reduce the heap size by 1. This process is repeated until the length of the heap is greater than 1.
def bubble_sort(arr): n = len(arr) for i in range(n): swapped = False # Last i elements are already in place, so we don't need to check them for j in range(0, n-i-1): # Swap if the element found is greater than the next element if arr[j] > arr[j+1]: arr[j], arr[j+1] = arr[j+1], arr[j] # Swap the elements swapped = True if not swapped: break return arrAlgorithm -
- Build a max heap using the heapify process. Heapify is a method used to maintain the heap property of a binary heap data structure.
- If the array is of size n, it contains n//2 parent nodes.
- For a parent at index i:
a.Its left child is at index 2i 1
b. Its right child is at index 2i 2
- Iterate over all subtrees with parents from index n//2 to 0 and call the heapify function on them.
- Now, the array becomes a max heap, with the largest element at index 0.
- Swap the first element with the last element of the heap and decrease the size of the heap by 1.
- Repeat this process until the length of the heap is greater than 1
Time Complexity : Heap Sort has a time complexity of O(n log n) in all three cases: best, average, and worst. This is because, irrespective of whether the array is already sorted or not, the same steps are followed each time a max heap is created and an element is swapped.
O( log n ) - To create max heap
O(n) - As the max heap is created and an element is swapped n times.
So the total time complexity is O (n) * O (log n) = O (n log n)
Space Complexity : For all cases - O( log n) - for the recursive stack.
Advantages -
- Time complexity is O (n log n), even for the large datasets.
- Memory usage is almost constant.
Disadvantages -
- Not Stable, as it does not maintain the relative order of equal elements.
- Many swaps are required as compared to merge sort.
Applications -
- It is useful for implementing priority queues where extraction of the maximum or minimum element is frequent.
- Useful in systems where in-place sorting is necessary and memory usage is critical.
- It is used in scenarios where rankings are needed.
7.Counting Sort and Radix Sort
Counting Sort is a non-comparison-based sorting algorithm. It is particularly efficient when the range of input values is small compared to the number of elements to be sorted. The basic idea behind Counting Sort is to count the frequency of each distinct element in the input array and use that information to place the elements in their correct sorted positions.
Radix Sort uses Counting Sort as a subroutine. It applies Counting Sort to each digit place of a number and repeatedly sorts until it processes all the digits of the largest number in the array.
def bubble_sort(arr): n = len(arr) for i in range(n): swapped = False # Last i elements are already in place, so we don't need to check them for j in range(0, n-i-1): # Swap if the element found is greater than the next element if arr[j] > arr[j+1]: arr[j], arr[j+1] = arr[j+1], arr[j] # Swap the elements swapped = True if not swapped: break return arrdef selectionSort(a): i = 0 while i<len smallest="min(a[i:])" index_of_smallest="a.index(smallest)" a i="i+1" return> <p><strong>Algorithm -</strong></p> <ol> <li><p>Find the maximum number in the array and determine the number of digits (d) in it. If the length of the number is d, Counting Sort is called d times on the array.</p></li> <li><p>Call Counting Sort for each digit place in the array, starting from the ones place, then tens place, and so on.</p></li> <li><p>In Counting sort:</p></li> </ol> <ul> <li>First, create an index array and map the elements based on their values.For example, if a digit is 4, increment the value at the 4th index of the index array.</li> <li>Create a prefix sum array from the index array.</li> <li>Using the prefix sum array, create a new sorted array equal to the length of the input array</li> <li>For example, if the prefix sum array has a value of 2 at index 1, place the value 1 at position 2 in the sorted array and decrement the value in the prefix sum array by 1</li> </ul> <p><strong>Time Complexity :</strong></p> <p><strong>Counting Sort</strong> has a time complexity of O(n k), where n is the number of elements to sort and k is the range of values (size of the index array).This complexity is valid for all three cases: best, average, and worst.</p> <p>This is because, irrespective of whether the array is already sorted or not, the same steps are followed each time.</p> <p><strong>Radix Sort’s</strong> time complexity increases by a factor of d, where d is the number of digits in the largest number in the array. Time complexity is O (d * (n k))</p> <p>So the total time complexity is O (d) * O(n k) = O (d * (n k))</p> <p>Space Complexity : For all cases - O(n k), where n is the length of the input array and k is the range of values in the index array.</p> <p><strong>Advantages -</strong></p> <ol> <li>Stable as elements keep their relative order.</li> <li>Counting sort generally performs faster than all comparison-based sorting algorithms, such as merge sort and quicksort, if the range of input is of the order of the number of input.</li> </ol> <p><strong>Disadvantages -</strong></p> <ol> <li>Counting sort doesn’t work on decimal values.</li> <li>Counting sort is inefficient if the range of values to be sorted is very large.</li> <li>Not in place sorting algorithm, as it uses extra space O(O(n m)).</li> </ol> <p><strong>Applications -</strong></p> <ol> <li>It is used in applications like counting character occurrences in strings.</li> <li>Useful for sorting integers with a large range of values, such as IDs or phone numbers.</li> <li>Efficient for sorting data with multiple keys, like dates or tuples.</li> </ol> </len>
The above is the detailed content of Sorting Algorithms || Python || Data Structures and Algorithms. For more information, please follow other related articles on the PHP Chinese website!
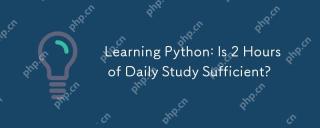
Is it enough to learn Python for two hours a day? It depends on your goals and learning methods. 1) Develop a clear learning plan, 2) Select appropriate learning resources and methods, 3) Practice and review and consolidate hands-on practice and review and consolidate, and you can gradually master the basic knowledge and advanced functions of Python during this period.
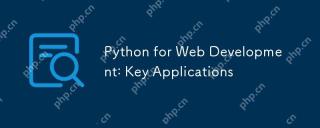
Key applications of Python in web development include the use of Django and Flask frameworks, API development, data analysis and visualization, machine learning and AI, and performance optimization. 1. Django and Flask framework: Django is suitable for rapid development of complex applications, and Flask is suitable for small or highly customized projects. 2. API development: Use Flask or DjangoRESTFramework to build RESTfulAPI. 3. Data analysis and visualization: Use Python to process data and display it through the web interface. 4. Machine Learning and AI: Python is used to build intelligent web applications. 5. Performance optimization: optimized through asynchronous programming, caching and code
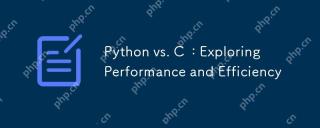
Python is better than C in development efficiency, but C is higher in execution performance. 1. Python's concise syntax and rich libraries improve development efficiency. 2.C's compilation-type characteristics and hardware control improve execution performance. When making a choice, you need to weigh the development speed and execution efficiency based on project needs.
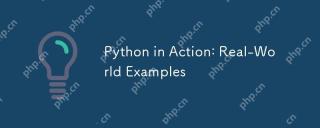
Python's real-world applications include data analytics, web development, artificial intelligence and automation. 1) In data analysis, Python uses Pandas and Matplotlib to process and visualize data. 2) In web development, Django and Flask frameworks simplify the creation of web applications. 3) In the field of artificial intelligence, TensorFlow and PyTorch are used to build and train models. 4) In terms of automation, Python scripts can be used for tasks such as copying files.
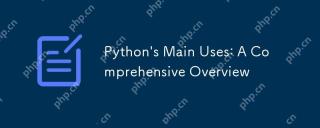
Python is widely used in data science, web development and automation scripting fields. 1) In data science, Python simplifies data processing and analysis through libraries such as NumPy and Pandas. 2) In web development, the Django and Flask frameworks enable developers to quickly build applications. 3) In automated scripts, Python's simplicity and standard library make it ideal.
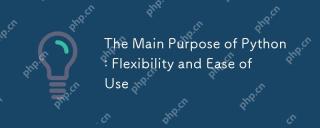
Python's flexibility is reflected in multi-paradigm support and dynamic type systems, while ease of use comes from a simple syntax and rich standard library. 1. Flexibility: Supports object-oriented, functional and procedural programming, and dynamic type systems improve development efficiency. 2. Ease of use: The grammar is close to natural language, the standard library covers a wide range of functions, and simplifies the development process.
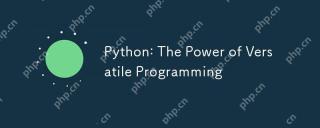
Python is highly favored for its simplicity and power, suitable for all needs from beginners to advanced developers. Its versatility is reflected in: 1) Easy to learn and use, simple syntax; 2) Rich libraries and frameworks, such as NumPy, Pandas, etc.; 3) Cross-platform support, which can be run on a variety of operating systems; 4) Suitable for scripting and automation tasks to improve work efficiency.
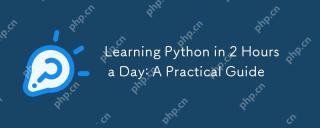
Yes, learn Python in two hours a day. 1. Develop a reasonable study plan, 2. Select the right learning resources, 3. Consolidate the knowledge learned through practice. These steps can help you master Python in a short time.


Hot AI Tools

Undresser.AI Undress
AI-powered app for creating realistic nude photos

AI Clothes Remover
Online AI tool for removing clothes from photos.

Undress AI Tool
Undress images for free

Clothoff.io
AI clothes remover

AI Hentai Generator
Generate AI Hentai for free.

Hot Article

Hot Tools
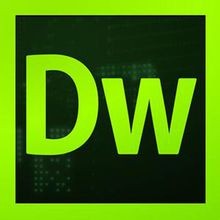
Dreamweaver CS6
Visual web development tools

Atom editor mac version download
The most popular open source editor

Zend Studio 13.0.1
Powerful PHP integrated development environment
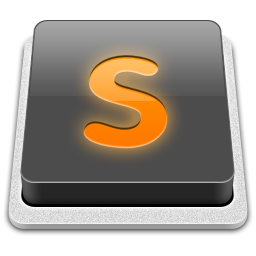
SublimeText3 Mac version
God-level code editing software (SublimeText3)

DVWA
Damn Vulnerable Web App (DVWA) is a PHP/MySQL web application that is very vulnerable. Its main goals are to be an aid for security professionals to test their skills and tools in a legal environment, to help web developers better understand the process of securing web applications, and to help teachers/students teach/learn in a classroom environment Web application security. The goal of DVWA is to practice some of the most common web vulnerabilities through a simple and straightforward interface, with varying degrees of difficulty. Please note that this software