Buy Me a Coffee☕
*My post explains Fashion-MNIST.
FashionMNIST() can use Fashion-MNIST dataset as shown below:
*Memos:
- The 1st argument is root(Required-Type:str or pathlib.Path). *An absolute or relative path is possible.
- The 2nd argument is train(Optional-Default:True-Type:bool). *If it's True, train data(60,000 images) is used while if it's False, test data(10,000 images) is used.
- The 3rd argument is transform(Optional-Default:None-Type:callable).
- The 4th argument is target_transform(Optional-Default:None-Type:callable).
- The 5th argument is download(Optional-Default:False-Type:bool):
*Memos:
- If it's True, the dataset is downloaded from the internet and extracted(unzipped) to root.
- If it's True and the dataset is already downloaded, it's extracted.
- If it's True and the dataset is already downloaded and extracted, nothing happens.
- It should be False if the dataset is already downloaded and extracted because it's faster.
- You can manually download and extract the dataset(t10k-images-idx3-ubyte.gz, t10k-labels-idx1-ubyte.gz, train-images-idx3-ubyte.gz and train-labels-idx1-ubyte.gz) from here to data/FashionMNIST/raw/.
from torchvision.datasets import FashionMNIST train_data = FashionMNIST( root="data" ) train_data = FashionMNIST( root="data", train=True, transform=None, target_transform=None, download=False ) test_data = FashionMNIST( root="data", train=False ) len(train_data), len(test_data) # (60000, 10000) train_data # Dataset FashionMNIST # Number of datapoints: 60000 # Root location: data # Split: Train train_data.root # 'data' train_data.train # True print(train_data.transform) # None print(train_data.target_transform) # None train_data.download # <bound method mnist.download of dataset fashionmnist number datapoints: root location: data split: train> len(train_data.classes) # 10 train_data.classes # ['T-shirt/top', 'Trouser', 'Pullover', 'Dress', 'Coat', # 'Sandal', 'Shirt', 'Sneaker', 'Bag', 'Ankle boot'] train_data[0] # (<pil.image.image image mode="L" size="28x28">, 9) train_data[1] # (<pil.image.image image mode="L" size="28x28">, 0) train_data[2] # (<pil.image.image image mode="L" size="28x28">, 0) train_data[3] # (<pil.image.image image mode="L" size="28x28">, 3) train_data[4] # (<pil.image.image image mode="L" size="28x28">, 0) import matplotlib.pyplot as plt def show_images(data, main_title=None): plt.figure(figsize=(8, 4)) plt.suptitle(t=main_title, y=1.0, fontsize=14) for i, (image, label) in enumerate(data, 1): plt.subplot(2, 5, i) plt.tight_layout() plt.title(label) plt.imshow(image) if i == 10: break plt.show() show_images(data=train_data, main_title="train_data") show_images(data=test_data, main_title="test_data") </pil.image.image></pil.image.image></pil.image.image></pil.image.image></pil.image.image></bound>
The above is the detailed content of FashionMNIST in PyTorch. For more information, please follow other related articles on the PHP Chinese website!
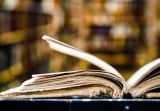
This tutorial demonstrates how to use Python to process the statistical concept of Zipf's law and demonstrates the efficiency of Python's reading and sorting large text files when processing the law. You may be wondering what the term Zipf distribution means. To understand this term, we first need to define Zipf's law. Don't worry, I'll try to simplify the instructions. Zipf's Law Zipf's law simply means: in a large natural language corpus, the most frequently occurring words appear about twice as frequently as the second frequent words, three times as the third frequent words, four times as the fourth frequent words, and so on. Let's look at an example. If you look at the Brown corpus in American English, you will notice that the most frequent word is "th
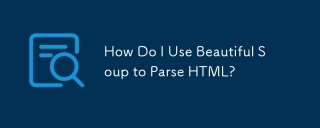
This article explains how to use Beautiful Soup, a Python library, to parse HTML. It details common methods like find(), find_all(), select(), and get_text() for data extraction, handling of diverse HTML structures and errors, and alternatives (Sel
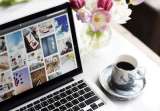
Dealing with noisy images is a common problem, especially with mobile phone or low-resolution camera photos. This tutorial explores image filtering techniques in Python using OpenCV to tackle this issue. Image Filtering: A Powerful Tool Image filter
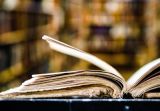
PDF files are popular for their cross-platform compatibility, with content and layout consistent across operating systems, reading devices and software. However, unlike Python processing plain text files, PDF files are binary files with more complex structures and contain elements such as fonts, colors, and images. Fortunately, it is not difficult to process PDF files with Python's external modules. This article will use the PyPDF2 module to demonstrate how to open a PDF file, print a page, and extract text. For the creation and editing of PDF files, please refer to another tutorial from me. Preparation The core lies in using external module PyPDF2. First, install it using pip: pip is P
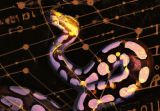
This tutorial demonstrates how to leverage Redis caching to boost the performance of Python applications, specifically within a Django framework. We'll cover Redis installation, Django configuration, and performance comparisons to highlight the bene
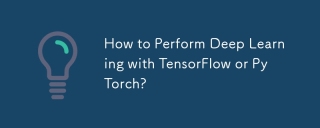
This article compares TensorFlow and PyTorch for deep learning. It details the steps involved: data preparation, model building, training, evaluation, and deployment. Key differences between the frameworks, particularly regarding computational grap
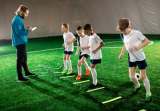
Python, a favorite for data science and processing, offers a rich ecosystem for high-performance computing. However, parallel programming in Python presents unique challenges. This tutorial explores these challenges, focusing on the Global Interprete
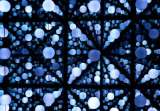
This tutorial demonstrates creating a custom pipeline data structure in Python 3, leveraging classes and operator overloading for enhanced functionality. The pipeline's flexibility lies in its ability to apply a series of functions to a data set, ge


Hot AI Tools

Undresser.AI Undress
AI-powered app for creating realistic nude photos

AI Clothes Remover
Online AI tool for removing clothes from photos.

Undress AI Tool
Undress images for free

Clothoff.io
AI clothes remover

AI Hentai Generator
Generate AI Hentai for free.

Hot Article

Hot Tools

Safe Exam Browser
Safe Exam Browser is a secure browser environment for taking online exams securely. This software turns any computer into a secure workstation. It controls access to any utility and prevents students from using unauthorized resources.
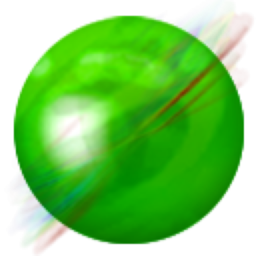
ZendStudio 13.5.1 Mac
Powerful PHP integrated development environment

SublimeText3 English version
Recommended: Win version, supports code prompts!

Zend Studio 13.0.1
Powerful PHP integrated development environment
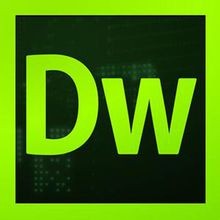
Dreamweaver CS6
Visual web development tools
