


In the realm of music and sound, there's a fascinating debate about frequency that has captured the attention of musicians, historians, and scientists alike. At the heart of this discussion lies the number 432 Hz, often referred to as the "natural frequency of the universe." Today, I'll take you through my journey of building a web application that analyzes audio files to determine if they're tuned to this mystical frequency.
The Historical Context
Before we dive into the technical details, let's understand why 432 Hz matters. This frequency wasn't chosen arbitrarily – it has deep historical roots. Musical legends like Bach and Beethoven tuned their instruments to A=432 Hz, considering it the natural tuning that resonates with the universe itself.
However, this changed during World War II when the standard was shifted to 440 Hz. Some argue that 440 Hz creates a subtle sense of tension and anxiety, comparing it to radio static. In contrast, 432 Hz is said to promote harmony and a natural flow in music. Whether you believe in these effects or not, the technical challenge of analyzing audio frequencies remains fascinating.
Technical Overview
Our application is built using modern web technologies and scientific computing libraries:
- Backend: FastAPI (Python)
- Audio Processing: pydub, numpy, scipy
- Frontend: Web interface for file uploads
- Analysis: Fast Fourier Transform (FFT) for frequency detection
The Science Behind Frequency Analysis
At the core of our application lies the Fast Fourier Transform (FFT) algorithm. FFT transforms our audio signal from the time domain to the frequency domain, allowing us to identify the dominant frequencies in a piece of music.
Here's how the analysis works:
- Audio Input Processing
audio = AudioSegment.from_file(io.BytesIO(file_content)).set_channels(1) # Convert to mono samples = np.array(audio.get_array_of_samples()) sample_rate = audio.frame_rate
- Frequency Analysis
fft_vals = rfft(samples) fft_freqs = rfftfreq(len(samples), d=1/sample_rate) dominant_freq = fft_freqs[np.argmax(np.abs(fft_vals))]
- Result Interpretation
tolerance = 5 # Hz result = ( f"The dominant frequency is {dominant_freq:.2f} Hz, " f"{'close to' if abs(dominant_freq - 432) <h2> Technical Implementation Details </h2> <h3> Backend Architecture </h3> <p>Our FastAPI backend handles the heavy lifting of audio processing. Here are the key features:</p> <ol> <li> <p><strong>File Validation</strong></p> <ul> <li>Ensures uploaded files are audio formats</li> <li>Limits file size to 20MB</li> <li>Validates audio stream integrity</li> </ul> </li> <li> <p><strong>Audio Processing Pipeline</strong></p> <ul> <li>Converts audio to mono for consistent analysis</li> <li>Extracts raw samples for FFT processing</li> <li>Applies FFT to identify frequency components</li> </ul> </li> <li> <p><strong>Error Handling</strong></p> <ul> <li>Graceful handling of invalid files</li> <li>Clear error messages for unsupported formats</li> <li>Robust exception handling for processing errors</li> </ul> </li> </ol> <h3> API Design </h3> <p>The API is simple yet effective:<br> </p> <pre class="brush:php;toolbar:false"> audio = AudioSegment.from_file(io.BytesIO(file_content)).set_channels(1) # Convert to mono samples = np.array(audio.get_array_of_samples()) sample_rate = audio.frame_rate
User Experience
The application provides a straightforward interface:
- Upload any supported audio file
- Receive instant analysis of the dominant frequency
- Get clear feedback on how close the frequency is to 432 Hz
- View detailed interpretation of the frequency's meaning and significance
Frequency Interpretation
One of the key features is the intelligent interpretation of frequencies. The application not only tells you the dominant frequency but also explains its significance:
fft_vals = rfft(samples) fft_freqs = rfftfreq(len(samples), d=1/sample_rate) dominant_freq = fft_freqs[np.argmax(np.abs(fft_vals))]
The interpretation system provides context for different frequency ranges:
- 432 Hz (±5 Hz): Explains the historical significance and natural alignment
- 440 Hz (±5 Hz): Details about the modern standard tuning
- Below 432 Hz: Information about lower frequency characteristics
- Above 432 Hz: Insights into higher frequency properties
This feature helps users understand not just the numerical value of the frequency, but also its musical and historical context, making the tool more educational and engaging.
Technical Challenges and Solutions
Challenge 1: Audio Format Compatibility
- Solution: Using pydub for broad format support
- Implemented format validation before processing
Challenge 2: Processing Large Files
- Solution: Implemented file size limits
- Added streaming support for efficient memory usage
Challenge 3: Accuracy vs. Performance
- Solution: Balanced FFT window size
- Implemented tolerance range for practical results
Future Improvements
-
Enhanced Analysis
- Multiple frequency detection
- Harmonic analysis
- Time-based frequency tracking
-
User Features
- Batch file processing
- Frequency visualization
- Audio pitch shifting to 432 Hz
Conclusion
Building this frequency analyzer has been an exciting journey through the intersection of music, history, and technology. Whether you're a musician interested in the 432 Hz phenomenon or a developer curious about audio processing, I hope this project provides valuable insights into how we can analyze and understand the frequencies that make up our musical world.
The complete source code is available on GitHub, and I welcome contributions and suggestions for improvements. Feel free to experiment with different audio files and explore the fascinating world of frequency analysis!
Note: This project is open-source and available for educational purposes. The frequency analysis is meant for experimental use and may not be suitable for professional audio tuning applications.
reyesvicente
/
432Hz-Frequency-Checker
This project checks if the frequency of a song is 432Hz or not.
This project checks if the frequency of a song is 432Hz or not.
Why 432Hz?
432Hz is considered the natural frequency of the universe, embraced by great composers like Bach and Beethoven to craft music that touches the soul. This indicates that the universal music scale utilized 432A for tuning their instruments. However, during World War II, this was altered to 440Hz, which resembles the static of a radio—disorienting and unsettling. In contrast, 432Hz fosters harmony and a sense of flow. It’s the ideal frequency, one that feels organic and uplifting! Nature truly is wonderful!
Run the backend:
audio = AudioSegment.from_file(io.BytesIO(file_content)).set_channels(1) # Convert to mono samples = np.array(audio.get_array_of_samples()) sample_rate = audio.frame_rate
Run the frontend
fft_vals = rfft(samples) fft_freqs = rfftfreq(len(samples), d=1/sample_rate) dominant_freq = fft_freqs[np.argmax(np.abs(fft_vals))]
The above is the detailed content of Exploring the Magic of Hz: Building a Music Frequency Analyzer. For more information, please follow other related articles on the PHP Chinese website!
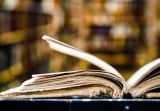
This tutorial demonstrates how to use Python to process the statistical concept of Zipf's law and demonstrates the efficiency of Python's reading and sorting large text files when processing the law. You may be wondering what the term Zipf distribution means. To understand this term, we first need to define Zipf's law. Don't worry, I'll try to simplify the instructions. Zipf's Law Zipf's law simply means: in a large natural language corpus, the most frequently occurring words appear about twice as frequently as the second frequent words, three times as the third frequent words, four times as the fourth frequent words, and so on. Let's look at an example. If you look at the Brown corpus in American English, you will notice that the most frequent word is "th
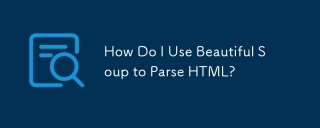
This article explains how to use Beautiful Soup, a Python library, to parse HTML. It details common methods like find(), find_all(), select(), and get_text() for data extraction, handling of diverse HTML structures and errors, and alternatives (Sel
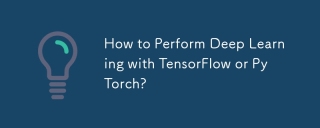
This article compares TensorFlow and PyTorch for deep learning. It details the steps involved: data preparation, model building, training, evaluation, and deployment. Key differences between the frameworks, particularly regarding computational grap
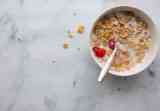
Serialization and deserialization of Python objects are key aspects of any non-trivial program. If you save something to a Python file, you do object serialization and deserialization if you read the configuration file, or if you respond to an HTTP request. In a sense, serialization and deserialization are the most boring things in the world. Who cares about all these formats and protocols? You want to persist or stream some Python objects and retrieve them in full at a later time. This is a great way to see the world on a conceptual level. However, on a practical level, the serialization scheme, format or protocol you choose may determine the speed, security, freedom of maintenance status, and other aspects of the program
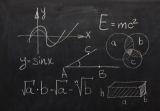
Python's statistics module provides powerful data statistical analysis capabilities to help us quickly understand the overall characteristics of data, such as biostatistics and business analysis. Instead of looking at data points one by one, just look at statistics such as mean or variance to discover trends and features in the original data that may be ignored, and compare large datasets more easily and effectively. This tutorial will explain how to calculate the mean and measure the degree of dispersion of the dataset. Unless otherwise stated, all functions in this module support the calculation of the mean() function instead of simply summing the average. Floating point numbers can also be used. import random import statistics from fracti
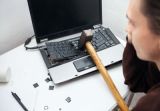
In this tutorial you'll learn how to handle error conditions in Python from a whole system point of view. Error handling is a critical aspect of design, and it crosses from the lowest levels (sometimes the hardware) all the way to the end users. If y
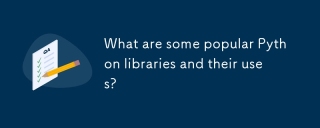
The article discusses popular Python libraries like NumPy, Pandas, Matplotlib, Scikit-learn, TensorFlow, Django, Flask, and Requests, detailing their uses in scientific computing, data analysis, visualization, machine learning, web development, and H
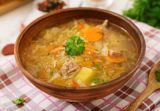
This tutorial builds upon the previous introduction to Beautiful Soup, focusing on DOM manipulation beyond simple tree navigation. We'll explore efficient search methods and techniques for modifying HTML structure. One common DOM search method is ex


Hot AI Tools

Undresser.AI Undress
AI-powered app for creating realistic nude photos

AI Clothes Remover
Online AI tool for removing clothes from photos.

Undress AI Tool
Undress images for free

Clothoff.io
AI clothes remover

AI Hentai Generator
Generate AI Hentai for free.

Hot Article

Hot Tools
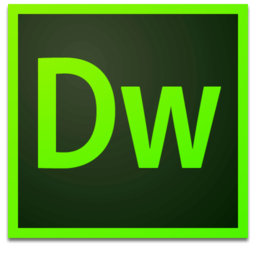
Dreamweaver Mac version
Visual web development tools

MinGW - Minimalist GNU for Windows
This project is in the process of being migrated to osdn.net/projects/mingw, you can continue to follow us there. MinGW: A native Windows port of the GNU Compiler Collection (GCC), freely distributable import libraries and header files for building native Windows applications; includes extensions to the MSVC runtime to support C99 functionality. All MinGW software can run on 64-bit Windows platforms.

MantisBT
Mantis is an easy-to-deploy web-based defect tracking tool designed to aid in product defect tracking. It requires PHP, MySQL and a web server. Check out our demo and hosting services.

Atom editor mac version download
The most popular open source editor

Notepad++7.3.1
Easy-to-use and free code editor
