The Challenge: Moving Beyond Traditional Prompting
When working with Language Models (LLMs), developers face a common set of challenges. We spend countless hours crafting perfect prompts, only to find that our carefully engineered solutions break when we switch models or when the input slightly changes. The traditional approach of prompt engineering is manual, time-consuming, and often unpredictable.
The Solution: Stanford's DSPy Framework
DSPy (Declarative Self-improving Python) emerges as Stanford NLP's answer to these challenges. As described on their website (dspy.ai), it's "the open-source framework for programming - rather than prompting - language models." It enables fast iteration on building modular AI systems and provides algorithms for optimizing prompts and weights, whether you're building simple classifiers, sophisticated RAG pipelines, or Agent loops.
How It Works: The Core Components
1. Getting Started
First, install the framework:
pip install -U dspy import dspy lm = dspy.LM('openai/gpt-4-mini', api_key='YOUR_OPENAI_API_KEY') dspy.configure(lm=lm)
2. Understanding Signatures
Signatures are the foundation of DSPy's declarative approach. They define the semantic roles for inputs and outputs in a simple format:
# Simple question answering "question -> answer" # Retrieval-based QA "context: list[str], question: str -> answer: str" # Multiple-choice with reasoning "question, choices: list[str] -> reasoning: str, selection: int"
3. Working with Modules
DSPy provides several key modules for different use cases:
- Predict: Direct LLM responses
- ChainOfThought: Step-by-step reasoning
- ProgramOfThought: Code-based solutions
- ReAct: Agent-based interactions
- MultiChainComparison: Compare multiple reasoning paths
4. Real-World Applications
Mathematical Problem Solving
math = dspy.ChainOfThought("question -> answer: float") math(question="Two dice are tossed. What is the probability that the sum equals two?")
Retrieval-Augmented Generation (RAG)
def search_wikipedia(query: str) -> list[str]: results = dspy.ColBERTv2(url='http://20.102.90.50:2017/wiki17_abstracts')(query, k=3) return [x['text'] for x in results] rag = dspy.ChainOfThought('context, question -> response')
Beyond the Basics
DSPy supports various advanced use cases:
- Classification tasks
- Information extraction
- Agent-based systems with tools
- Complex RAG pipelines
The framework's self-improving nature means your applications can optimize their performance over time, learning from interactions and results.
Ready to Start?
You can find complete examples and explore more use cases in the DSPy documentation and the community repository at https://github.com/gabrielvanderlei/DSPy-examples.
DSPy represents a paradigm shift from traditional prompt engineering to declarative programming with language models. It brings structure, reliability, and predictability to LLM development, making it easier to build and maintain AI-powered applications.
The above is the detailed content of DSPy: A New Approach to Language Model Programming. For more information, please follow other related articles on the PHP Chinese website!
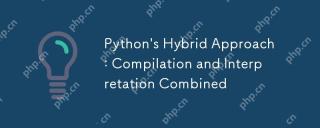
Pythonusesahybridapproach,combiningcompilationtobytecodeandinterpretation.1)Codeiscompiledtoplatform-independentbytecode.2)BytecodeisinterpretedbythePythonVirtualMachine,enhancingefficiencyandportability.
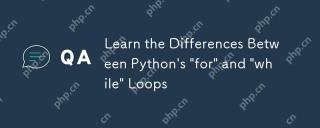
ThekeydifferencesbetweenPython's"for"and"while"loopsare:1)"For"loopsareidealforiteratingoversequencesorknowniterations,while2)"while"loopsarebetterforcontinuinguntilaconditionismetwithoutpredefinediterations.Un
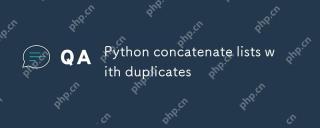
In Python, you can connect lists and manage duplicate elements through a variety of methods: 1) Use operators or extend() to retain all duplicate elements; 2) Convert to sets and then return to lists to remove all duplicate elements, but the original order will be lost; 3) Use loops or list comprehensions to combine sets to remove duplicate elements and maintain the original order.
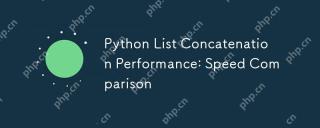
ThefastestmethodforlistconcatenationinPythondependsonlistsize:1)Forsmalllists,the operatorisefficient.2)Forlargerlists,list.extend()orlistcomprehensionisfaster,withextend()beingmorememory-efficientbymodifyinglistsin-place.
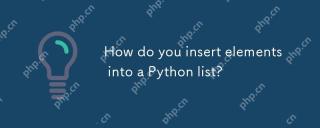
ToinsertelementsintoaPythonlist,useappend()toaddtotheend,insert()foraspecificposition,andextend()formultipleelements.1)Useappend()foraddingsingleitemstotheend.2)Useinsert()toaddataspecificindex,thoughit'sslowerforlargelists.3)Useextend()toaddmultiple
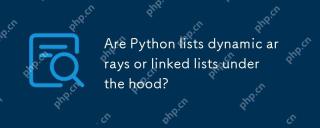
Pythonlistsareimplementedasdynamicarrays,notlinkedlists.1)Theyarestoredincontiguousmemoryblocks,whichmayrequirereallocationwhenappendingitems,impactingperformance.2)Linkedlistswouldofferefficientinsertions/deletionsbutslowerindexedaccess,leadingPytho
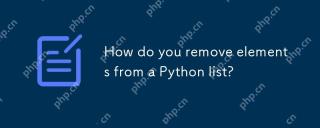
Pythonoffersfourmainmethodstoremoveelementsfromalist:1)remove(value)removesthefirstoccurrenceofavalue,2)pop(index)removesandreturnsanelementataspecifiedindex,3)delstatementremoveselementsbyindexorslice,and4)clear()removesallitemsfromthelist.Eachmetho
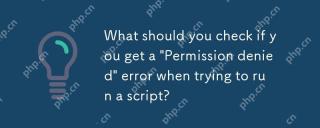
Toresolvea"Permissiondenied"errorwhenrunningascript,followthesesteps:1)Checkandadjustthescript'spermissionsusingchmod xmyscript.shtomakeitexecutable.2)Ensurethescriptislocatedinadirectorywhereyouhavewritepermissions,suchasyourhomedirectory.


Hot AI Tools

Undresser.AI Undress
AI-powered app for creating realistic nude photos

AI Clothes Remover
Online AI tool for removing clothes from photos.

Undress AI Tool
Undress images for free

Clothoff.io
AI clothes remover

Video Face Swap
Swap faces in any video effortlessly with our completely free AI face swap tool!

Hot Article

Hot Tools
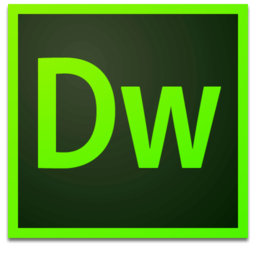
Dreamweaver Mac version
Visual web development tools
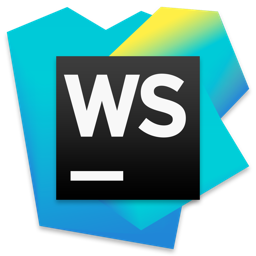
WebStorm Mac version
Useful JavaScript development tools
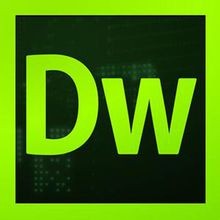
Dreamweaver CS6
Visual web development tools

SublimeText3 English version
Recommended: Win version, supports code prompts!

MinGW - Minimalist GNU for Windows
This project is in the process of being migrated to osdn.net/projects/mingw, you can continue to follow us there. MinGW: A native Windows port of the GNU Compiler Collection (GCC), freely distributable import libraries and header files for building native Windows applications; includes extensions to the MSVC runtime to support C99 functionality. All MinGW software can run on 64-bit Windows platforms.
