Artificial Intelligence (AI) has rapidly transitioned from a futuristic concept to a present-day reality reshaping industries and career paths. From powering chatbots and recommendation systems to enabling autonomous vehicles and predictive analytics, AI has become the backbone of technological innovation. For those eager to start a career in AI, understanding the programming languages that drive AI development is essential.
But before diving into the list of languages, it’s worth noting that building a career in AI requires more than just mastering coding. It involves an understanding of machine learning (ML), data science, and domain-specific applications. A comprehensive guide like roadmap.sh AI Engineer Roadmap can be invaluable in structuring your learning path.
Now, let’s focus on the core tools—programming languages. Here are the best languages for AI development and why they matter:
1. Python:
Python remains the most popular language in AI development for several reasons. Its simplicity and readability make it an excellent choice for beginners, while its extensive libraries—such as TensorFlow, PyTorch, Scikit-learn, and Keras—cater to advanced AI applications. Python’s versatility also means you can seamlessly work on data preprocessing, model building, and deployment in a single ecosystem.
Where Python shines:
- Machine learning and deep learning
- Natural language processing (NLP)
- Computer vision
- Robotics
Why Python?
Its supportive community and vast resources make troubleshooting and innovation much easier.
2. R: The Data Science Specialist
For those with a strong focus on statistics, data analysis, and visualization, R is a go-to language. Its comprehensive packages, such as Caret, RandomForest, and ggplot2, are perfect for training and visualizing machine learning models.
Where R excels:
- Statistical analysis
- Predictive modeling
- Data visualization
While it isn’t as versatile as Python for deployment, R remains a powerful ally for prototyping and research in AI.
3. Java: The Enterprise AI
Java has been a staple in enterprise applications for decades. Its stability, scalability, and portability make it a reliable choice for large-scale AI solutions. Tools like Weka, Deeplearning4j, and MOA enable Java developers to dive into AI and machine learning projects.
Where Java is effective:
- Enterprise-grade AI solutions
- Big data integration
- Cloud-based AI systems
If you’re already familiar with Java, leveraging it for AI development can reduce the learning curve.
4. Julia:
Julia is emerging as a strong contender in AI development, particularly for tasks that require heavy numerical computing. It offers the speed of C while retaining the usability of Python, making it ideal for high-performance AI systems.
Where Julia fits:
- Scientific computing
- High-performance machine learning
- Large-scale simulations
Although its ecosystem is still growing compared to Python or R, Julia’s future in AI looks promising.
5. C :
For applications that require fine-grained control over performance, such as robotics and game AI, C is unparalleled. Its low-level memory management capabilities make it ideal for real-time AI solutions.
Where C leads:
- Robotics
- Game development
- High-performance AI applications
However, C comes with a steep learning curve and may not be beginner-friendly.
6. JavaScript: AI in the Browser
With the rise of TensorFlow.js and Brain.js, JavaScript has entered the AI arena, enabling developers to create AI applications that run directly in web browsers.
Where JavaScript thrives:
- Front-end AI applications
- Interactive data visualizations
- Prototyping AI concepts
This is particularly useful for developers who want to integrate AI features into web applications.
7. MATLAB: The Researcher’s Tool
MATLAB is widely used in academia and research for AI projects involving image processing, signal processing, and neural networks. Its built-in toolbox simplifies many AI workflows, but its proprietary nature makes it less popular in open-source communities.
How to Choose the Right Language?
The best programming language for AI development depends on your goals and the specific application you have in mind:
- For beginners: Start with Python—it’s easy to learn and widely applicable.
- For data-centric projects: Consider R for its statistical prowess.
- For performance-critical applications: Opt for Julia or C .
- For web-based AI: JavaScript might be your best bet.
- For enterprise-level solutions: Java’s scalability is unmatched.
Next Steps
Starting a career in AI Engineering/Development requires more than just mastering a programming language. Here are some additional tips:
- Learn the fundamentals of machine learning: Understand algorithms, data preprocessing, and model evaluation.
- Get comfortable with data: Data wrangling, cleaning, and visualization are foundational skills.
- Experiment with AI frameworks: Libraries like TensorFlow, PyTorch, and Scikit-learn are must-haves.
- Collaborate and innovate: Join AI communities, contribute to open-source projects, and stay updated on industry trends.
The above is the detailed content of These Are the Best Programming Languages for AI Development.. For more information, please follow other related articles on the PHP Chinese website!
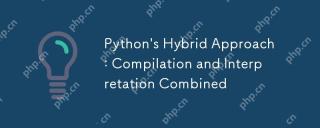
Pythonusesahybridapproach,combiningcompilationtobytecodeandinterpretation.1)Codeiscompiledtoplatform-independentbytecode.2)BytecodeisinterpretedbythePythonVirtualMachine,enhancingefficiencyandportability.
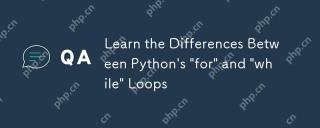
ThekeydifferencesbetweenPython's"for"and"while"loopsare:1)"For"loopsareidealforiteratingoversequencesorknowniterations,while2)"while"loopsarebetterforcontinuinguntilaconditionismetwithoutpredefinediterations.Un
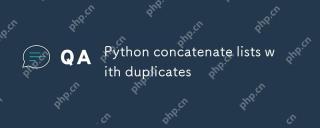
In Python, you can connect lists and manage duplicate elements through a variety of methods: 1) Use operators or extend() to retain all duplicate elements; 2) Convert to sets and then return to lists to remove all duplicate elements, but the original order will be lost; 3) Use loops or list comprehensions to combine sets to remove duplicate elements and maintain the original order.
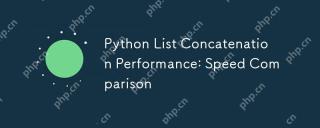
ThefastestmethodforlistconcatenationinPythondependsonlistsize:1)Forsmalllists,the operatorisefficient.2)Forlargerlists,list.extend()orlistcomprehensionisfaster,withextend()beingmorememory-efficientbymodifyinglistsin-place.
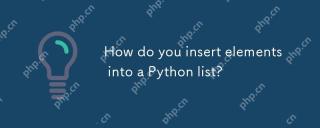
ToinsertelementsintoaPythonlist,useappend()toaddtotheend,insert()foraspecificposition,andextend()formultipleelements.1)Useappend()foraddingsingleitemstotheend.2)Useinsert()toaddataspecificindex,thoughit'sslowerforlargelists.3)Useextend()toaddmultiple
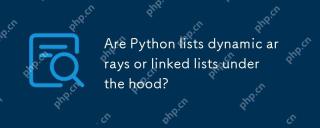
Pythonlistsareimplementedasdynamicarrays,notlinkedlists.1)Theyarestoredincontiguousmemoryblocks,whichmayrequirereallocationwhenappendingitems,impactingperformance.2)Linkedlistswouldofferefficientinsertions/deletionsbutslowerindexedaccess,leadingPytho
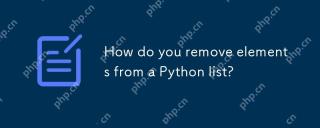
Pythonoffersfourmainmethodstoremoveelementsfromalist:1)remove(value)removesthefirstoccurrenceofavalue,2)pop(index)removesandreturnsanelementataspecifiedindex,3)delstatementremoveselementsbyindexorslice,and4)clear()removesallitemsfromthelist.Eachmetho
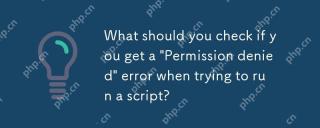
Toresolvea"Permissiondenied"errorwhenrunningascript,followthesesteps:1)Checkandadjustthescript'spermissionsusingchmod xmyscript.shtomakeitexecutable.2)Ensurethescriptislocatedinadirectorywhereyouhavewritepermissions,suchasyourhomedirectory.


Hot AI Tools

Undresser.AI Undress
AI-powered app for creating realistic nude photos

AI Clothes Remover
Online AI tool for removing clothes from photos.

Undress AI Tool
Undress images for free

Clothoff.io
AI clothes remover

Video Face Swap
Swap faces in any video effortlessly with our completely free AI face swap tool!

Hot Article

Hot Tools
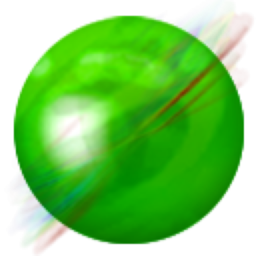
ZendStudio 13.5.1 Mac
Powerful PHP integrated development environment
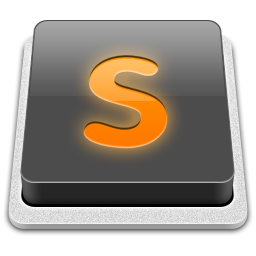
SublimeText3 Mac version
God-level code editing software (SublimeText3)
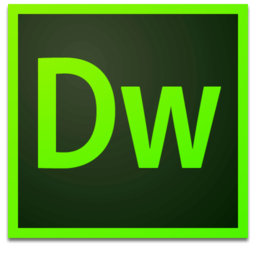
Dreamweaver Mac version
Visual web development tools
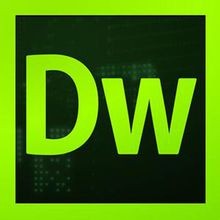
Dreamweaver CS6
Visual web development tools

Safe Exam Browser
Safe Exam Browser is a secure browser environment for taking online exams securely. This software turns any computer into a secure workstation. It controls access to any utility and prevents students from using unauthorized resources.
