


Introduction
I am currently maintaining a powerful open source creative drawing board. This drawing board integrates a lot of interesting brushes and auxiliary drawing functions, which allows users to experience a new drawing effect. Whether on mobile or PC, you can enjoy a better interactive experience and effect display.
In this article, I will explain in detail how to combine Transformers.js to achieve background removal and image marking segmentation. The result is as follows
Link: https://songlh.top/paint-board/
Github: https://github.com/LHRUN/paint-board Welcome to Star ⭐️
Transformers.js
Transformers.js is a powerful JavaScript library based on Hugging Face's Transformers that can be run directly in the browser without relying on server-side computation. This means that you can run your models locally, increasing efficiency and reducing deployment and maintenance costs.
Currently Transformers.js has provided 1000 models on Hugging Face, covering various domains, which can satisfy most of your needs, such as image processing, text generation, translation, sentiment analysis and other tasks processing, you can easily achieve through Transformers.js. Search for models as follows.
The current major version of Transformers.js has been updated to V3, which adds a lot of great features, details: Transformers.js v3: WebGPU Support, New Models & Tasks, and More….
Both of the features I've added to this post use WebGpu support, which is only available in V3, and has greatly improved processing speed, with parsing now in the milliseconds. However, it should be noted that there are not many browsers that support WebGPU, so it is recommended to use the latest version of Google to visit.
Function 1: Remove background
To remove the background I use the Xenova/modnet model, which looks like this
The processing logic can be divided into three steps
- initialise the state, and load the model and processor.
- the display of the interface, this is based on your own design, not on mine.
- Show the effect, this is based on your own design, not mine. Nowadays it is more popular to use a border line to dynamically display the contrast effect before and after removing the background.
The code logic is as follows, React TS , see my project's source code for details, the source code is located in src/components/boardOperation/uploadImage/index.tsx
import { useState, FC, useRef, useEffect, useMemo } from 'react' import { env, AutoModel, AutoProcessor, RawImage, PreTrainedModel, Processor } from '@huggingface/transformers' const REMOVE_BACKGROUND_STATUS = { LOADING: 0, NO_SUPPORT_WEBGPU: 1, LOAD_ERROR: 2, LOAD_SUCCESS: 3, PROCESSING: 4, PROCESSING_SUCCESS: 5 } type RemoveBackgroundStatusType = (typeof REMOVE_BACKGROUND_STATUS)[keyof typeof REMOVE_BACKGROUND_STATUS] const UploadImage: FC = ({ url }) => { const [removeBackgroundStatus, setRemoveBackgroundStatus] = useState<removebackgroundstatustype>() const [processedImage, setProcessedImage] = useState('') const modelRef = useRef<pretrainedmodel>() const processorRef = useRef<processor>() const removeBackgroundBtnTip = useMemo(() => { switch (removeBackgroundStatus) { case REMOVE_BACKGROUND_STATUS.LOADING: return 'Remove background function loading' case REMOVE_BACKGROUND_STATUS.NO_SUPPORT_WEBGPU: return 'WebGPU is not supported in this browser, to use the remove background function, please use the latest version of Google Chrome' case REMOVE_BACKGROUND_STATUS.LOAD_ERROR: return 'Remove background function failed to load' case REMOVE_BACKGROUND_STATUS.LOAD_SUCCESS: return 'Remove background function loaded successfully' case REMOVE_BACKGROUND_STATUS.PROCESSING: return 'Remove Background Processing' case REMOVE_BACKGROUND_STATUS.PROCESSING_SUCCESS: return 'Remove Background Processing Success' default: return '' } }, [removeBackgroundStatus]) useEffect(() => { ;(async () => { try { if (removeBackgroundStatus === REMOVE_BACKGROUND_STATUS.LOADING) { return } setRemoveBackgroundStatus(REMOVE_BACKGROUND_STATUS.LOADING) // Checking WebGPU Support if (!navigator?.gpu) { setRemoveBackgroundStatus(REMOVE_BACKGROUND_STATUS.NO_SUPPORT_WEBGPU) return } const model_id = 'Xenova/modnet' if (env.backends.onnx.wasm) { env.backends.onnx.wasm.proxy = false } // Load model and processor modelRef.current ??= await AutoModel.from_pretrained(model_id, { device: 'webgpu' }) processorRef.current ??= await AutoProcessor.from_pretrained(model_id) setRemoveBackgroundStatus(REMOVE_BACKGROUND_STATUS.LOAD_SUCCESS) } catch (err) { console.log('err', err) setRemoveBackgroundStatus(REMOVE_BACKGROUND_STATUS.LOAD_ERROR) } })() }, []) const processImages = async () => { const model = modelRef.current const processor = processorRef.current if (!model || !processor) { return } setRemoveBackgroundStatus(REMOVE_BACKGROUND_STATUS.PROCESSING) // load image const img = await RawImage.fromURL(url) // Pre-processed image const { pixel_values } = await processor(img) // Generate image mask const { output } = await model({ input: pixel_values }) const maskData = ( await RawImage.fromTensor(output[0].mul(255).to('uint8')).resize( img.width, img.height ) ).data // Create a new canvas const canvas = document.createElement('canvas') canvas.width = img.width canvas.height = img.height const ctx = canvas.getContext('2d') as CanvasRenderingContext2D // Draw the original image ctx.drawImage(img.toCanvas(), 0, 0) // Updating the mask area const pixelData = ctx.getImageData(0, 0, img.width, img.height) for (let i = 0; i <button classname="{`btn" btn-primary btn-sm remove_background_status.load_success remove_background_status.processing_success undefined : onclick="{processImages}"> Remove background </button> <div classname="text-xs text-base-content mt-2 flex"> {removeBackgroundBtnTip} </div> <div classname="relative mt-4 border border-base-content border-dashed rounded-lg overflow-hidden"> <img src="/static/imghwm/default1.png" data-src="https://img.php.cn/upload/article/000/000/000/173262759935552.jpg?x-oss-process=image/resize,p_40" class="lazy" classname="{`w-[50vw]" max-w- h- max-h- object-contain alt="Exploring the Canvas Series: combined with Transformers.js to achieve intelligent image processing" > {processedImage && ( <img src="/static/imghwm/default1.png" data-src="https://img.php.cn/upload/article/000/000/000/173262759935552.jpg?x-oss-process=image/resize,p_40" class="lazy" classname="{`w-full" h-full absolute top-0 left-0 z- object-contain alt="Exploring the Canvas Series: combined with Transformers.js to achieve intelligent image processing" > )} </div> ) } export default UploadImage </processor></pretrainedmodel></removebackgroundstatustype>
Function 2: Image Marker Segmentation
The image marker segmentation is implemented using the Xenova/slimsam-77-uniform model. The effect is as follows, you can click on the image after it is loaded, and the segmentation is generated according to the coordinates of your click.
The processing logic can be divided into five steps
- initialise the state, and load the model and processor
- Get the image and load it, then save the image loading data and embedding data.
- listen to the image click event, record the click data, divided into positive markers and negative markers, after each click according to the click data decoded to generate the mask data, and then according to the mask data to draw the segmentation effect.
- interface display, this to your own design arbitrary play, not my prevail
- click to save the image, according to the mask pixel data, match the original image data, and then exported through the canvas drawing
The code logic is as follows, React TS , see my project's source code for details, the source code is located in src/components/boardOperation/uploadImage/imageSegmentation.tsx
import { useState, useRef, useEffect, useMemo, MouseEvent, FC } from 'react' import { SamModel, AutoProcessor, RawImage, PreTrainedModel, Processor, Tensor, SamImageProcessorResult } from '@huggingface/transformers' import LoadingIcon from '@/components/icons/loading.svg?react' import PositiveIcon from '@/components/icons/boardOperation/image-segmentation-positive.svg?react' import NegativeIcon from '@/components/icons/boardOperation/image-segmentation-negative.svg?react' interface MarkPoint { position: number[] label: number } const SEGMENTATION_STATUS = { LOADING: 0, NO_SUPPORT_WEBGPU: 1, LOAD_ERROR: 2, LOAD_SUCCESS: 3, PROCESSING: 4, PROCESSING_SUCCESS: 5 } type SegmentationStatusType = (typeof SEGMENTATION_STATUS)[keyof typeof SEGMENTATION_STATUS] const ImageSegmentation: FC = ({ url }) => { const [markPoints, setMarkPoints] = useState<markpoint>([]) const [segmentationStatus, setSegmentationStatus] = useState<segmentationstatustype>() const [pointStatus, setPointStatus] = useState<boolean>(true) const maskCanvasRef = useRef<htmlcanvaselement>(null) // Segmentation mask const modelRef = useRef<pretrainedmodel>() // model const processorRef = useRef<processor>() // processor const imageInputRef = useRef<rawimage>() // original image const imageProcessed = useRef<samimageprocessorresult>() // Processed image const imageEmbeddings = useRef<tensor>() // Embedding data const segmentationTip = useMemo(() => { switch (segmentationStatus) { case SEGMENTATION_STATUS.LOADING: return 'Image Segmentation function Loading' case SEGMENTATION_STATUS.NO_SUPPORT_WEBGPU: return 'WebGPU is not supported in this browser, to use the image segmentation function, please use the latest version of Google Chrome.' case SEGMENTATION_STATUS.LOAD_ERROR: return 'Image Segmentation function failed to load' case SEGMENTATION_STATUS.LOAD_SUCCESS: return 'Image Segmentation function loaded successfully' case SEGMENTATION_STATUS.PROCESSING: return 'Image Processing...' case SEGMENTATION_STATUS.PROCESSING_SUCCESS: return 'The image has been processed successfully, you can click on the image to mark it, the green mask area is the segmentation area.' default: return '' } }, [segmentationStatus]) // 1. load model and processor useEffect(() => { ;(async () => { try { if (segmentationStatus === SEGMENTATION_STATUS.LOADING) { return } setSegmentationStatus(SEGMENTATION_STATUS.LOADING) if (!navigator?.gpu) { setSegmentationStatus(SEGMENTATION_STATUS.NO_SUPPORT_WEBGPU) return }const model_id = 'Xenova/slimsam-77-uniform' modelRef.current ??= await SamModel.from_pretrained(model_id, { dtype: 'fp16', // or "fp32" device: 'webgpu' }) processorRef.current ??= await AutoProcessor.from_pretrained(model_id) setSegmentationStatus(SEGMENTATION_STATUS.LOAD_SUCCESS) } catch (err) { console.log('err', err) setSegmentationStatus(SEGMENTATION_STATUS.LOAD_ERROR) } })() }, []) // 2. process image useEffect(() => { ;(async () => { try { if ( !modelRef.current || !processorRef.current || !url || segmentationStatus === SEGMENTATION_STATUS.PROCESSING ) { return } setSegmentationStatus(SEGMENTATION_STATUS.PROCESSING) clearPoints() imageInputRef.current = await RawImage.fromURL(url) imageProcessed.current = await processorRef.current( imageInputRef.current ) imageEmbeddings.current = await ( modelRef.current as any ).get_image_embeddings(imageProcessed.current) setSegmentationStatus(SEGMENTATION_STATUS.PROCESSING_SUCCESS) } catch (err) { console.log('err', err) } })() }, [url, modelRef.current, processorRef.current]) // Updating the mask effect function updateMaskOverlay(mask: RawImage, scores: Float32Array) { const maskCanvas = maskCanvasRef.current if (!maskCanvas) { return } const maskContext = maskCanvas.getContext('2d') as CanvasRenderingContext2D // Update canvas dimensions (if different) if (maskCanvas.width !== mask.width || maskCanvas.height !== mask.height) { maskCanvas.width = mask.width maskCanvas.height = mask.height } // Allocate buffer for pixel data const imageData = maskContext.createImageData( maskCanvas.width, maskCanvas.height ) // Select best mask const numMasks = scores.length // 3 let bestIndex = 0 for (let i = 1; i scores[bestIndex]) { bestIndex = i } } // Fill mask with colour const pixelData = imageData.data for (let i = 0; i { if ( !modelRef.current || !imageEmbeddings.current || !processorRef.current || !imageProcessed.current ) { return }// No click on the data directly clears the segmentation effect if (!markPoints.length && maskCanvasRef.current) { const maskContext = maskCanvasRef.current.getContext( '2d' ) as CanvasRenderingContext2D maskContext.clearRect( 0, 0, maskCanvasRef.current.width, maskCanvasRef.current.height ) return } // Prepare inputs for decoding const reshaped = imageProcessed.current.reshaped_input_sizes[0] const points = markPoints .map((x) => [x.position[0] * reshaped[1], x.position[1] * reshaped[0]]) .flat(Infinity) const labels = markPoints.map((x) => BigInt(x.label)).flat(Infinity) const num_points = markPoints.length const input_points = new Tensor('float32', points, [1, 1, num_points, 2]) const input_labels = new Tensor('int64', labels, [1, 1, num_points]) // Generate the mask const { pred_masks, iou_scores } = await modelRef.current({ ...imageEmbeddings.current, input_points, input_labels }) // Post-process the mask const masks = await (processorRef.current as any).post_process_masks( pred_masks, imageProcessed.current.original_sizes, imageProcessed.current.reshaped_input_sizes ) updateMaskOverlay(RawImage.fromTensor(masks[0][0]), iou_scores.data) } const clamp = (x: number, min = 0, max = 1) => { return Math.max(Math.min(x, max), min) } const clickImage = (e: MouseEvent) => { if (segmentationStatus !== SEGMENTATION_STATUS.PROCESSING_SUCCESS) { return } const { clientX, clientY, currentTarget } = e const { left, top } = currentTarget.getBoundingClientRect() const x = clamp( (clientX - left currentTarget.scrollLeft) / currentTarget.scrollWidth ) const y = clamp( (clientY - top currentTarget.scrollTop) / currentTarget.scrollHeight ) const existingPointIndex = markPoints.findIndex( (point) => Math.abs(point.position[0] - x) { setMarkPoints([]) decode([]) } return ( <div classname="card shadow-xl overflow-auto"> <div classname="flex items-center gap-x-3"> <button classname="btn btn-primary btn-sm" onclick="{clearPoints}"> Clear Points </button> <button classname="btn btn-primary btn-sm" onclick="{()"> setPointStatus(true)} > {pointStatus ? 'Positive' : 'Negative'} </button> </div> <div classname="text-xs text-base-content mt-2">{segmentationTip}</div> <div> <h2> Conclusion </h2> <p>Thank you for reading. This is the whole content of this article, I hope this article is helpful to you, welcome to like and favourite. If you have any questions, please feel free to discuss in the comment section!</p> </div> </div></tensor></samimageprocessorresult></rawimage></processor></pretrainedmodel></htmlcanvaselement></boolean></segmentationstatustype></markpoint>
The above is the detailed content of Exploring the Canvas Series: combined with Transformers.js to achieve intelligent image processing. For more information, please follow other related articles on the PHP Chinese website!
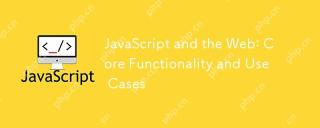
The main uses of JavaScript in web development include client interaction, form verification and asynchronous communication. 1) Dynamic content update and user interaction through DOM operations; 2) Client verification is carried out before the user submits data to improve the user experience; 3) Refreshless communication with the server is achieved through AJAX technology.
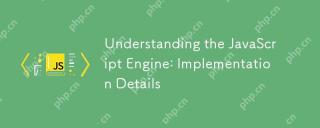
Understanding how JavaScript engine works internally is important to developers because it helps write more efficient code and understand performance bottlenecks and optimization strategies. 1) The engine's workflow includes three stages: parsing, compiling and execution; 2) During the execution process, the engine will perform dynamic optimization, such as inline cache and hidden classes; 3) Best practices include avoiding global variables, optimizing loops, using const and lets, and avoiding excessive use of closures.

Python is more suitable for beginners, with a smooth learning curve and concise syntax; JavaScript is suitable for front-end development, with a steep learning curve and flexible syntax. 1. Python syntax is intuitive and suitable for data science and back-end development. 2. JavaScript is flexible and widely used in front-end and server-side programming.
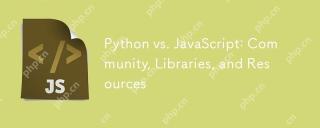
Python and JavaScript have their own advantages and disadvantages in terms of community, libraries and resources. 1) The Python community is friendly and suitable for beginners, but the front-end development resources are not as rich as JavaScript. 2) Python is powerful in data science and machine learning libraries, while JavaScript is better in front-end development libraries and frameworks. 3) Both have rich learning resources, but Python is suitable for starting with official documents, while JavaScript is better with MDNWebDocs. The choice should be based on project needs and personal interests.
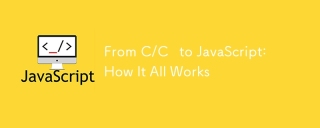
The shift from C/C to JavaScript requires adapting to dynamic typing, garbage collection and asynchronous programming. 1) C/C is a statically typed language that requires manual memory management, while JavaScript is dynamically typed and garbage collection is automatically processed. 2) C/C needs to be compiled into machine code, while JavaScript is an interpreted language. 3) JavaScript introduces concepts such as closures, prototype chains and Promise, which enhances flexibility and asynchronous programming capabilities.
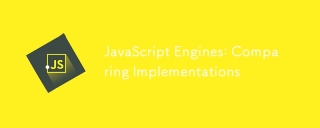
Different JavaScript engines have different effects when parsing and executing JavaScript code, because the implementation principles and optimization strategies of each engine differ. 1. Lexical analysis: convert source code into lexical unit. 2. Grammar analysis: Generate an abstract syntax tree. 3. Optimization and compilation: Generate machine code through the JIT compiler. 4. Execute: Run the machine code. V8 engine optimizes through instant compilation and hidden class, SpiderMonkey uses a type inference system, resulting in different performance performance on the same code.
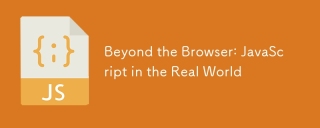
JavaScript's applications in the real world include server-side programming, mobile application development and Internet of Things control: 1. Server-side programming is realized through Node.js, suitable for high concurrent request processing. 2. Mobile application development is carried out through ReactNative and supports cross-platform deployment. 3. Used for IoT device control through Johnny-Five library, suitable for hardware interaction.
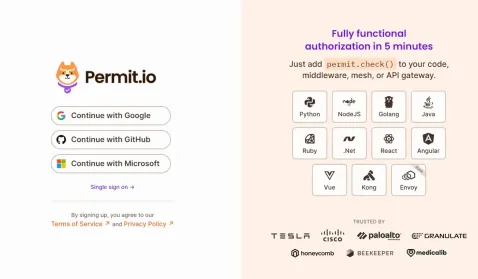
I built a functional multi-tenant SaaS application (an EdTech app) with your everyday tech tool and you can do the same. First, what’s a multi-tenant SaaS application? Multi-tenant SaaS applications let you serve multiple customers from a sing


Hot AI Tools

Undresser.AI Undress
AI-powered app for creating realistic nude photos

AI Clothes Remover
Online AI tool for removing clothes from photos.

Undress AI Tool
Undress images for free

Clothoff.io
AI clothes remover

AI Hentai Generator
Generate AI Hentai for free.

Hot Article

Hot Tools

MinGW - Minimalist GNU for Windows
This project is in the process of being migrated to osdn.net/projects/mingw, you can continue to follow us there. MinGW: A native Windows port of the GNU Compiler Collection (GCC), freely distributable import libraries and header files for building native Windows applications; includes extensions to the MSVC runtime to support C99 functionality. All MinGW software can run on 64-bit Windows platforms.

Notepad++7.3.1
Easy-to-use and free code editor
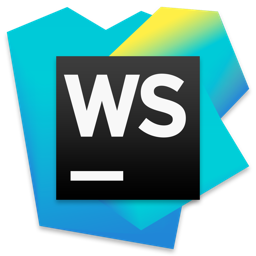
WebStorm Mac version
Useful JavaScript development tools
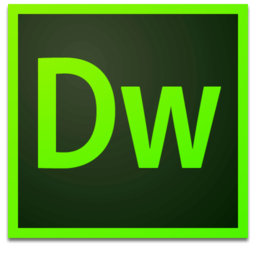
Dreamweaver Mac version
Visual web development tools
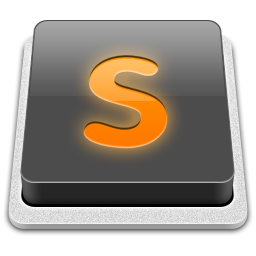
SublimeText3 Mac version
God-level code editing software (SublimeText3)